windows10上搭建caffe以及踩到的坑
对动作捕捉的几篇论文感兴趣,想复现一下,需要caffe环境就折腾了下!转模型需要python 2.7环境,我顺便也弄了!!!
1. 环境
Windows10
RTX2080TI 11G
Anaconda Python2.7
visual studio 2013
cuda 11.1
cudnn 8.2.0
cmake 3.26.1
git
2. 具体步骤
2.1 安装cuda和cudnn
略 这部分看其他博客
cuda_11.1.0_456.43_win10.exe
cudnn-11.3-windows-x64-v8.2.0.53.zip
2.2 安装caffe
1.下载源码
git clone https://github.com/BVLC/caffe.git
cd caffe
git checkout windows
2.修改build_win.cmd 这一步决定着后面能不能编译成功
path/caffe/scripts/build_win.cmd
a.需要确定visual studio的版本
MSVC版本号对应关系 我在这边踩了坑,因为我看的那个博客这边弄错了。这个链接没问题。MSVC_VERSION = 12表示VS2013,MSVC_VERSION = 14表示VS2015
b.确定GPU的显卡架构 架构表 一定要看
下面开始修改文件:
1.修改caffe源码中./scripts/build_win.cmd
修改caffe源码中./cmake/Cuda.cmake:
我的显卡是2080ti,对应着75,我只要保证有75就行!
这里的80需要根据我的显卡情况改成75
这里面是一定要改的,不然找不到cudnn!因为caffe之类的代码很久不更新了,只支持到了使用cudnn7.x,在使用了cudnn8的环境下编译caffe时,会在src/caffe/layers/cudnn_conv_layer.cpp等文件里出错!
报错信息是这样的:
error: identifier "CUDNN_CONVOLUTION_FWD_SPECIFY_WORKSPACE_LIMIT" is undefined
error: identifier "cudnnGetConvolutionForwardAlgorithm" is undefined
这是因为cudnn8里没有cudnnGetConvolutionForwardAlgorithm()这个函数了,改成了cudnnGetConvolutionForwardAlgorithm_v7(),也没了CUDNN_CONVOLUTION_FWD_SPECIFY_WORKSPACE_LIMIT这个宏定义,这些都是API不兼容,但是NVIDIA声明cudnn8不支持了,caffe的代码也没人去更新了,所以不能指望NVIDIA或者berkeley,只能自行修改。将cudnn_conv_layer.cpp文件替换成如下:
#ifdef USE_CUDNN
#include <algorithm>
#include <vector>#include "caffe/layers/cudnn_conv_layer.hpp"namespace caffe {// Set to three for the benefit of the backward pass, which
// can use separate streams for calculating the gradient w.r.t.
// bias, filter weights, and bottom data for each group independently
#define CUDNN_STREAMS_PER_GROUP 3/*** TODO(dox) explain cuDNN interface*/
template <typename Dtype>
void CuDNNConvolutionLayer<Dtype>::LayerSetUp(const vector<Blob<Dtype>*>& bottom, const vector<Blob<Dtype>*>& top) {ConvolutionLayer<Dtype>::LayerSetUp(bottom, top);// Initialize CUDA streams and cuDNN.stream_ = new cudaStream_t[this->group_ * CUDNN_STREAMS_PER_GROUP];handle_ = new cudnnHandle_t[this->group_ * CUDNN_STREAMS_PER_GROUP];// Initialize algorithm arraysfwd_algo_ = new cudnnConvolutionFwdAlgo_t[bottom.size()];bwd_filter_algo_= new cudnnConvolutionBwdFilterAlgo_t[bottom.size()];bwd_data_algo_ = new cudnnConvolutionBwdDataAlgo_t[bottom.size()];// initialize size arraysworkspace_fwd_sizes_ = new size_t[bottom.size()];workspace_bwd_filter_sizes_ = new size_t[bottom.size()];workspace_bwd_data_sizes_ = new size_t[bottom.size()];// workspace dataworkspaceSizeInBytes = 0;workspaceData = NULL;workspace = new void*[this->group_ * CUDNN_STREAMS_PER_GROUP];for (size_t i = 0; i < bottom.size(); ++i) {// initialize all to default algorithmsfwd_algo_[i] = (cudnnConvolutionFwdAlgo_t)0;bwd_filter_algo_[i] = (cudnnConvolutionBwdFilterAlgo_t)0;bwd_data_algo_[i] = (cudnnConvolutionBwdDataAlgo_t)0;// default algorithms don't require workspaceworkspace_fwd_sizes_[i] = 0;workspace_bwd_data_sizes_[i] = 0;workspace_bwd_filter_sizes_[i] = 0;}for (int g = 0; g < this->group_ * CUDNN_STREAMS_PER_GROUP; g++) {CUDA_CHECK(cudaStreamCreate(&stream_[g]));CUDNN_CHECK(cudnnCreate(&handle_[g]));CUDNN_CHECK(cudnnSetStream(handle_[g], stream_[g]));workspace[g] = NULL;}// Set the indexing parameters.bias_offset_ = (this->num_output_ / this->group_);// Create filter descriptor.const int* kernel_shape_data = this->kernel_shape_.cpu_data();const int kernel_h = kernel_shape_data[0];const int kernel_w = kernel_shape_data[1];cudnn::createFilterDesc<Dtype>(&filter_desc_,this->num_output_ / this->group_, this->channels_ / this->group_,kernel_h, kernel_w);// Create tensor descriptor(s) for data and corresponding convolution(s).for (int i = 0; i < bottom.size(); i++) {cudnnTensorDescriptor_t bottom_desc;cudnn::createTensor4dDesc<Dtype>(&bottom_desc);bottom_descs_.push_back(bottom_desc);cudnnTensorDescriptor_t top_desc;cudnn::createTensor4dDesc<Dtype>(&top_desc);top_descs_.push_back(top_desc);cudnnConvolutionDescriptor_t conv_desc;cudnn::createConvolutionDesc<Dtype>(&conv_desc);conv_descs_.push_back(conv_desc);}// Tensor descriptor for bias.if (this->bias_term_) {cudnn::createTensor4dDesc<Dtype>(&bias_desc_);}handles_setup_ = true;
}template <typename Dtype>
void CuDNNConvolutionLayer<Dtype>::Reshape(const vector<Blob<Dtype>*>& bottom, const vector<Blob<Dtype>*>& top) {ConvolutionLayer<Dtype>::Reshape(bottom, top);CHECK_EQ(2, this->num_spatial_axes_)<< "CuDNNConvolution input must have 2 spatial axes "<< "(e.g., height and width). "<< "Use 'engine: CAFFE' for general ND convolution.";bottom_offset_ = this->bottom_dim_ / this->group_;top_offset_ = this->top_dim_ / this->group_;const int height = bottom[0]->shape(this->channel_axis_ + 1);const int width = bottom[0]->shape(this->channel_axis_ + 2);const int height_out = top[0]->shape(this->channel_axis_ + 1);const int width_out = top[0]->shape(this->channel_axis_ + 2);const int* pad_data = this->pad_.cpu_data();const int pad_h = pad_data[0];const int pad_w = pad_data[1];const int* stride_data = this->stride_.cpu_data();const int stride_h = stride_data[0];const int stride_w = stride_data[1];
#if CUDNN_VERSION_MIN(8, 0, 0)int RetCnt;bool found_conv_algorithm;size_t free_memory, total_memory;cudnnConvolutionFwdAlgoPerf_t fwd_algo_pref_[4];cudnnConvolutionBwdDataAlgoPerf_t bwd_data_algo_pref_[4];//get memory sizescudaMemGetInfo(&free_memory, &total_memory);
#else// Specify workspace limit for kernels directly until we have a// planning strategy and a rewrite of Caffe's GPU memory mangagementsize_t workspace_limit_bytes = 8*1024*1024;
#endiffor (int i = 0; i < bottom.size(); i++) {cudnn::setTensor4dDesc<Dtype>(&bottom_descs_[i],this->num_,this->channels_ / this->group_, height, width,this->channels_ * height * width,height * width, width, 1);cudnn::setTensor4dDesc<Dtype>(&top_descs_[i],this->num_,this->num_output_ / this->group_, height_out, width_out,this->num_output_ * this->out_spatial_dim_,this->out_spatial_dim_, width_out, 1);cudnn::setConvolutionDesc<Dtype>(&conv_descs_[i], bottom_descs_[i],filter_desc_, pad_h, pad_w,stride_h, stride_w);#if CUDNN_VERSION_MIN(8, 0, 0)// choose forward algorithm for filter// in forward filter the CUDNN_CONVOLUTION_FWD_ALGO_WINOGRAD_NONFUSED is not implemented in cuDNN 8CUDNN_CHECK(cudnnGetConvolutionForwardAlgorithm_v7(handle_[0],bottom_descs_[i],filter_desc_,conv_descs_[i],top_descs_[i],4,&RetCnt,fwd_algo_pref_));found_conv_algorithm = false;for(int n=0;n<RetCnt;n++){if (fwd_algo_pref_[n].status == CUDNN_STATUS_SUCCESS &&fwd_algo_pref_[n].algo != CUDNN_CONVOLUTION_FWD_ALGO_WINOGRAD_NONFUSED &&fwd_algo_pref_[n].memory < free_memory){found_conv_algorithm = true;fwd_algo_[i] = fwd_algo_pref_[n].algo;workspace_fwd_sizes_[i] = fwd_algo_pref_[n].memory;break;}}if(!found_conv_algorithm) LOG(ERROR) << "cuDNN did not return a suitable algorithm for convolution.";else{// choose backward algorithm for filter// for better or worse, just a fixed constant due to the missing // cudnnGetConvolutionBackwardFilterAlgorithm in cuDNN version 8.0bwd_filter_algo_[i] = CUDNN_CONVOLUTION_BWD_FILTER_ALGO_0;//twice the amount of the forward search to be save workspace_bwd_filter_sizes_[i] = 2*workspace_fwd_sizes_[i];}// choose backward algo for dataCUDNN_CHECK(cudnnGetConvolutionBackwardDataAlgorithm_v7(handle_[0],filter_desc_, top_descs_[i], conv_descs_[i], bottom_descs_[i],4,&RetCnt,bwd_data_algo_pref_));found_conv_algorithm = false;for(int n=0;n<RetCnt;n++){if (bwd_data_algo_pref_[n].status == CUDNN_STATUS_SUCCESS &&bwd_data_algo_pref_[n].algo != CUDNN_CONVOLUTION_BWD_DATA_ALGO_WINOGRAD &&bwd_data_algo_pref_[n].algo != CUDNN_CONVOLUTION_BWD_DATA_ALGO_WINOGRAD_NONFUSED &&bwd_data_algo_pref_[n].memory < free_memory){found_conv_algorithm = true;bwd_data_algo_[i] = bwd_data_algo_pref_[n].algo;workspace_bwd_data_sizes_[i] = bwd_data_algo_pref_[n].memory;break;}}if(!found_conv_algorithm) LOG(ERROR) << "cuDNN did not return a suitable algorithm for convolution.";
#else// choose forward and backward algorithms + workspace(s)CUDNN_CHECK(cudnnGetConvolutionForwardAlgorithm(handle_[0],bottom_descs_[i],filter_desc_,conv_descs_[i],top_descs_[i],CUDNN_CONVOLUTION_FWD_SPECIFY_WORKSPACE_LIMIT,workspace_limit_bytes,&fwd_algo_[i]));CUDNN_CHECK(cudnnGetConvolutionForwardWorkspaceSize(handle_[0],bottom_descs_[i],filter_desc_,conv_descs_[i],top_descs_[i],fwd_algo_[i],&(workspace_fwd_sizes_[i])));// choose backward algorithm for filterCUDNN_CHECK(cudnnGetConvolutionBackwardFilterAlgorithm(handle_[0],bottom_descs_[i], top_descs_[i], conv_descs_[i], filter_desc_,CUDNN_CONVOLUTION_BWD_FILTER_SPECIFY_WORKSPACE_LIMIT,workspace_limit_bytes, &bwd_filter_algo_[i]) );// get workspace for backwards filter algorithmCUDNN_CHECK(cudnnGetConvolutionBackwardFilterWorkspaceSize(handle_[0],bottom_descs_[i], top_descs_[i], conv_descs_[i], filter_desc_,bwd_filter_algo_[i], &workspace_bwd_filter_sizes_[i]));// choose backward algo for dataCUDNN_CHECK(cudnnGetConvolutionBackwardDataAlgorithm(handle_[0],filter_desc_, top_descs_[i], conv_descs_[i], bottom_descs_[i],CUDNN_CONVOLUTION_BWD_DATA_SPECIFY_WORKSPACE_LIMIT,workspace_limit_bytes, &bwd_data_algo_[i]));// get workspace sizeCUDNN_CHECK(cudnnGetConvolutionBackwardDataWorkspaceSize(handle_[0],filter_desc_, top_descs_[i], conv_descs_[i], bottom_descs_[i],bwd_data_algo_[i], &workspace_bwd_data_sizes_[i]) );
#endif}// reduce over all workspace sizes to get a maximum to allocate / reallocatesize_t total_workspace_fwd = 0;size_t total_workspace_bwd_data = 0;size_t total_workspace_bwd_filter = 0;for (size_t i = 0; i < bottom.size(); i++) {total_workspace_fwd = std::max(total_workspace_fwd,workspace_fwd_sizes_[i]);total_workspace_bwd_data = std::max(total_workspace_bwd_data,workspace_bwd_data_sizes_[i]);total_workspace_bwd_filter = std::max(total_workspace_bwd_filter,workspace_bwd_filter_sizes_[i]);}// get max over all operationssize_t max_workspace = std::max(total_workspace_fwd,total_workspace_bwd_data);max_workspace = std::max(max_workspace, total_workspace_bwd_filter);// ensure all groups have enough workspacesize_t total_max_workspace = max_workspace *(this->group_ * CUDNN_STREAMS_PER_GROUP);// this is the total amount of storage needed over all groups + streamsif (total_max_workspace > workspaceSizeInBytes) {DLOG(INFO) << "Reallocating workspace storage: " << total_max_workspace;workspaceSizeInBytes = total_max_workspace;// free the existing workspace and allocate a new (larger) onecudaFree(this->workspaceData);cudaError_t err = cudaMalloc(&(this->workspaceData), workspaceSizeInBytes);if (err != cudaSuccess) {// force zero memory pathfor (int i = 0; i < bottom.size(); i++) {workspace_fwd_sizes_[i] = 0;workspace_bwd_filter_sizes_[i] = 0;workspace_bwd_data_sizes_[i] = 0;fwd_algo_[i] = CUDNN_CONVOLUTION_FWD_ALGO_IMPLICIT_GEMM;bwd_filter_algo_[i] = CUDNN_CONVOLUTION_BWD_FILTER_ALGO_0;bwd_data_algo_[i] = CUDNN_CONVOLUTION_BWD_DATA_ALGO_0;}// NULL out all workspace pointersfor (int g = 0; g < (this->group_ * CUDNN_STREAMS_PER_GROUP); g++) {workspace[g] = NULL;}// NULL out underlying dataworkspaceData = NULL;workspaceSizeInBytes = 0;}// if we succeed in the allocation, set pointer aliases for workspacesfor (int g = 0; g < (this->group_ * CUDNN_STREAMS_PER_GROUP); g++) {workspace[g] = reinterpret_cast<char *>(workspaceData) + g*max_workspace;}}// Tensor descriptor for bias.if (this->bias_term_) {cudnn::setTensor4dDesc<Dtype>(&bias_desc_,1, this->num_output_ / this->group_, 1, 1);}
}template <typename Dtype>
CuDNNConvolutionLayer<Dtype>::~CuDNNConvolutionLayer() {// Check that handles have been setup before destroying.if (!handles_setup_) { return; }for (int i = 0; i < bottom_descs_.size(); i++) {cudnnDestroyTensorDescriptor(bottom_descs_[i]);cudnnDestroyTensorDescriptor(top_descs_[i]);cudnnDestroyConvolutionDescriptor(conv_descs_[i]);}if (this->bias_term_) {cudnnDestroyTensorDescriptor(bias_desc_);}cudnnDestroyFilterDescriptor(filter_desc_);for (int g = 0; g < this->group_ * CUDNN_STREAMS_PER_GROUP; g++) {cudaStreamDestroy(stream_[g]);cudnnDestroy(handle_[g]);}cudaFree(workspaceData);delete [] stream_;delete [] handle_;delete [] fwd_algo_;delete [] bwd_filter_algo_;delete [] bwd_data_algo_;delete [] workspace_fwd_sizes_;delete [] workspace_bwd_data_sizes_;delete [] workspace_bwd_filter_sizes_;
}INSTANTIATE_CLASS(CuDNNConvolutionLayer);} // namespace caffe
#endif
在命令行窗口运行 scripts/build_win.cmd,会下载libraries_v120_x64_py27_1.1.0.tar.bz2文件,最好挂个梯子,我这边下的很快。这个文件是caffe相关的依赖库,此过程中编译的时候会报一个boost相关的错误,对C:\Users\qiao\.caffe\dependencies\libraries_v120_x64_py27_1.1.0\libraries\include\boost-1_61\boost\config\compiler
路径下的 nvcc.hpp 作如下修改,因为RTX2080ti的编译器nvcc版本大于7.5:
之后删除之前编译的build文件夹,重新编译一次,编译过程中会出现较多警告可以不用理会,稍等一段时间后,最终会出现:
最后在build文件夹下找到Caffe.sln文件,用VS2013打开,然后右键ALL_BUILD进行生成,等几分钟后编译完,
release版本
debug版本
将caffe源码下中python中的caffe文件夹粘贴到上面配置的python路径中D:\Anaconda3\envs\pycaffe27\Lib\site-packages
再将E:\caffe\build\install\bin
路径添加到环境变量中,在终端中测试一下caffe命令是否正常,然后pip安装一些必要的库
pip install numpy scipy protobuf six scikit-image pyyaml pydotplus graphviz
最后打开python,测试一下
参考文献:
Windows10 下RTX30系列Caffe安装教程
Windows10下搭建caffe过程记录
相关文章:
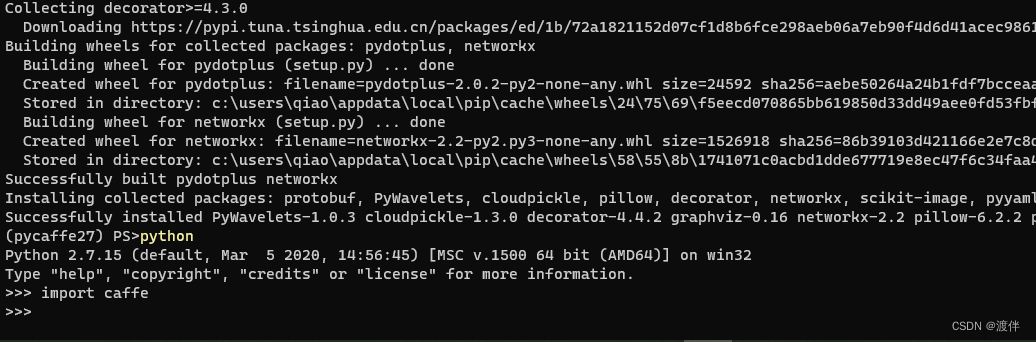
windows10上搭建caffe以及踩到的坑
对动作捕捉的几篇论文感兴趣,想复现一下,需要caffe环境就折腾了下!转模型需要python 2.7环境,我顺便也弄了!!! 1. 环境 Windows10 RTX2080TI 11G Anaconda Python2.7 visual studio 2013 cuda…...
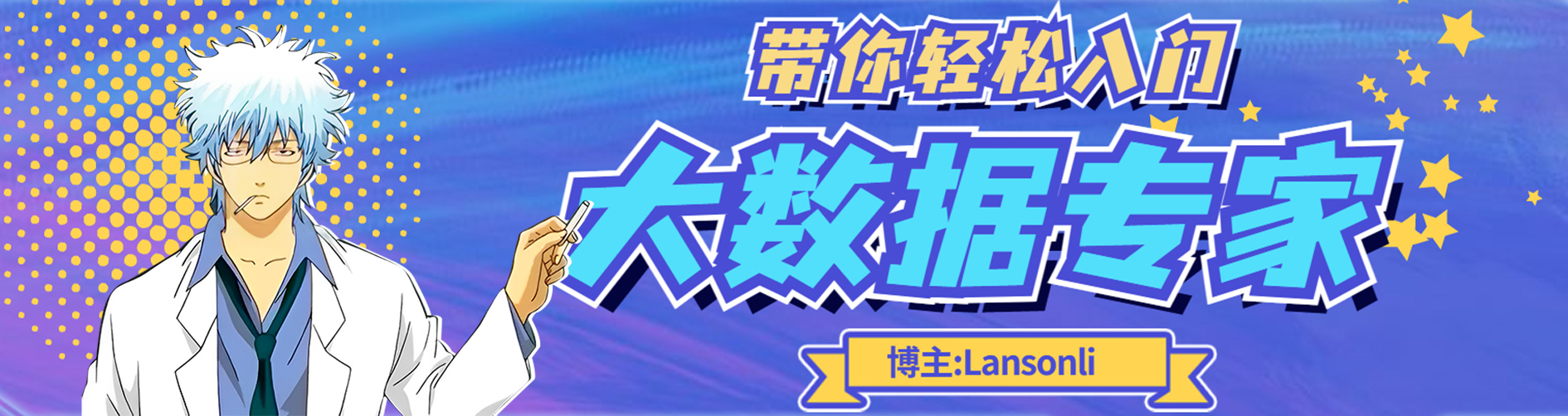
大数据Flink(七十):SQL 动态表 连续查询
文章目录 SQL 动态表 & 连续查询 一、SQL 应用于流处理的思路...

「MySQL-04」Linux环境下使用C/C++连接并操纵MySQL
目录 一、准备mysql库:Connector/C 1. 查看是否有mysql相关的库和头文件 2. 安装devel(开发库) 3.到官网下载开发包,并上传到Linux 3.0 须知 3.1 到官网下载开发包 3.2 上传安装包至Linux 二、mysql库:Connector/C 的使用 1. 创建并初始化mys…...

【力扣】两数相除(c/c++)
目录 题目 注意: 示例 1: 示例 2: 提示: 题目解析 题目思路 代码思路 数据处理 注意 减法函数 第一次使用的函数 问题 第二次改良后的代码 处理i的值并且返回 总代码 力扣的代码 注意 题目 给你两个整数,被除数 dividend 和…...
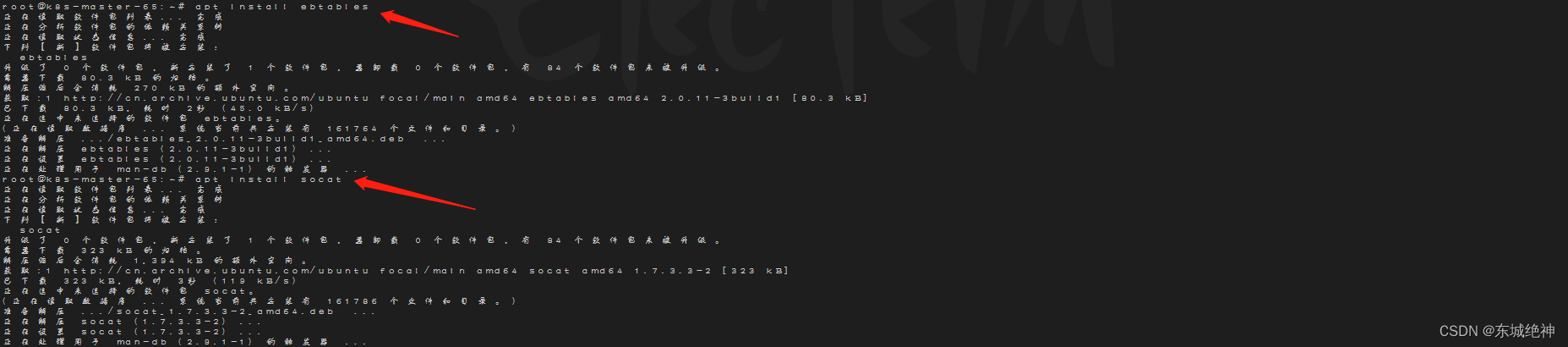
《Kubernetes部署篇:Ubuntu20.04基于二进制安装安装kubeadm、kubelet和kubectl》
一、背景 由于客户网络处于专网环境下, 使用kubeadm工具安装K8S集群,由于无法连通互联网,所有无法使用apt工具安装kubeadm、kubelet、kubectl,当然你也可以使用apt-get工具在一台能够连通互联网环境的服务器上下载kubeadm、kubele…...
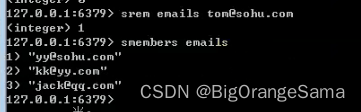
go学习part21 Redis
300_尚硅谷_Redis的基本介绍和原理示意_哔哩哔哩_bilibili Redis 命令 | 菜鸟教程 (runoob.com) 1.基本介绍 2.基本操作 Redis的基本使用: 说明:Redis安装好后,默认有16个数据库,初始默认使用0号库,编号是0...15 1.添加key-val [set] 2.查看当前redi…...
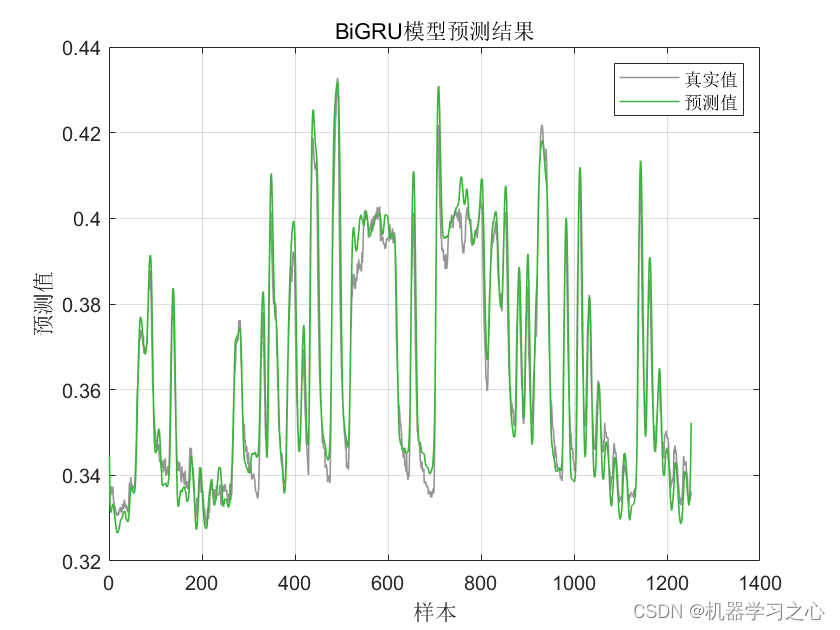
时序预测 | MATLAB实现基于PSO-BiGRU、BiGRU时间序列预测对比
时序预测 | MATLAB实现基于PSO-BiGRU、BiGRU时间序列预测对比 目录 时序预测 | MATLAB实现基于PSO-BiGRU、BiGRU时间序列预测对比效果一览基本描述程序设计参考资料 效果一览 基本描述 1.时序预测 | MATLAB实现基于PSO-BiGRU、BiGRU时间序列预测; 2.单变量时间序列数…...
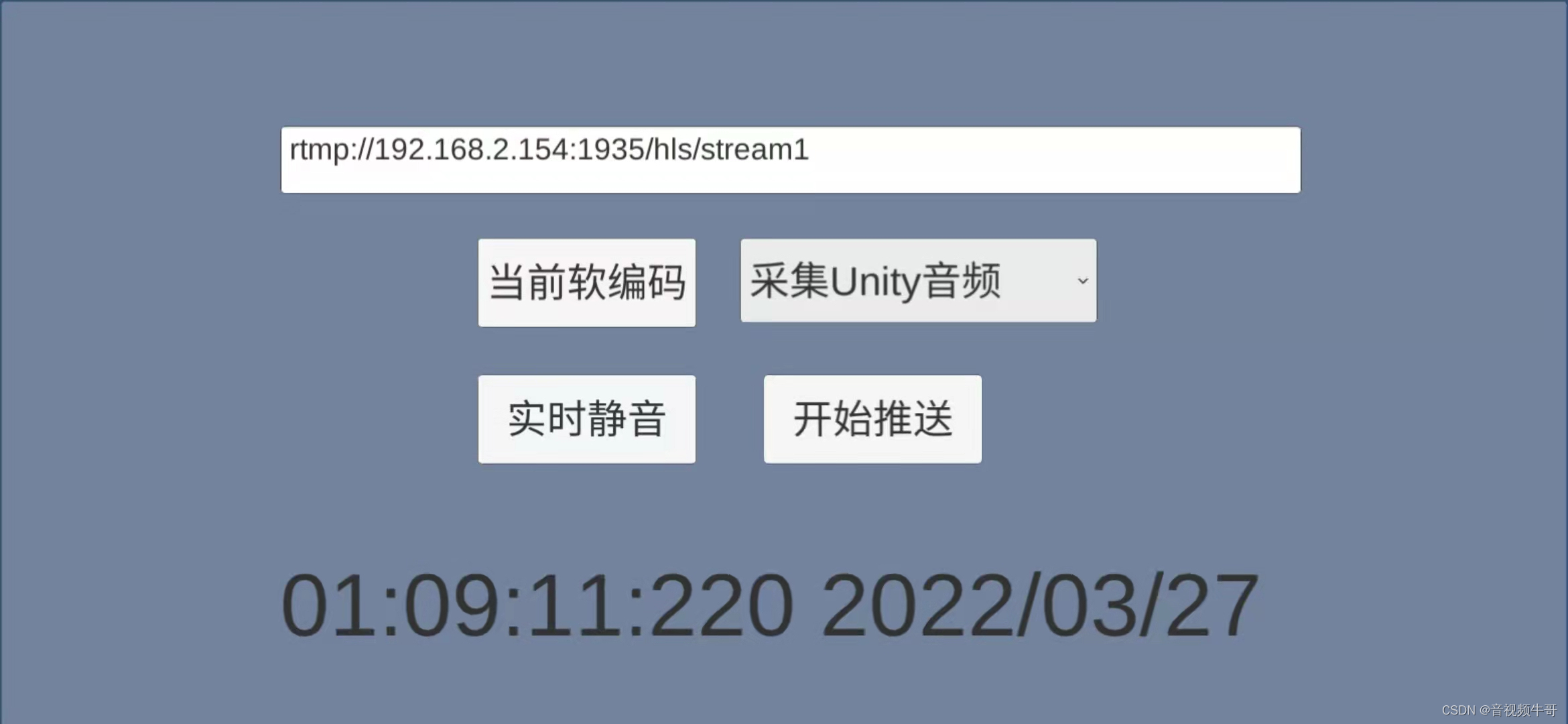
Unity3D下如何采集camera场景数据并推送RTMP服务?
Unity3D使用场景 Unity3D是非常流行的游戏开发引擎,可以创建各种类型的3D和2D游戏或其他互动应用程序。常见使用场景如下: 游戏开发:Unity3D是一个广泛用于游戏开发的环境,适用于创建各种类型的游戏,包括动作游戏、角…...
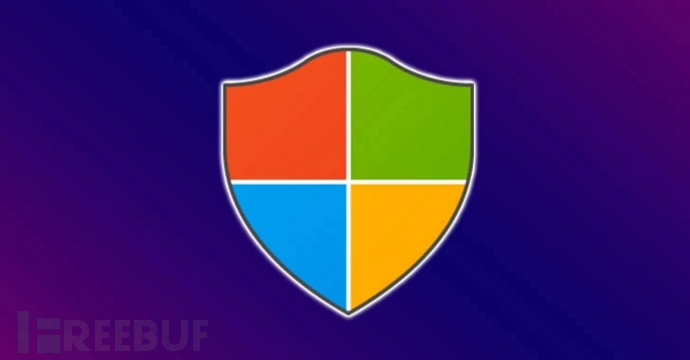
黑客可利用 Windows 容器隔离框架绕过端点安全系统
新的研究结果表明,攻击者可以利用一种隐匿的恶意软件检测规避技术,并通过操纵 Windows 容器隔离框架来绕过端点安全的解决方案。 Deep Instinct安全研究员丹尼尔-阿维诺姆(Daniel Avinoam)在本月初举行的DEF CON安全大会上公布了…...

STM32注入通道
什么是注入通道? 注入通道是ADC的一种采样方式,主要用于在规则通道转换期间并行处理快速变化信号的采样。注入通道的转换可以在规则通道转换时强行插入,相当于一个“中断通道”。当有注入通道需要转换时,规则通道的转换会停止,优先执行注入通道的转换,当注入通道的转换执…...
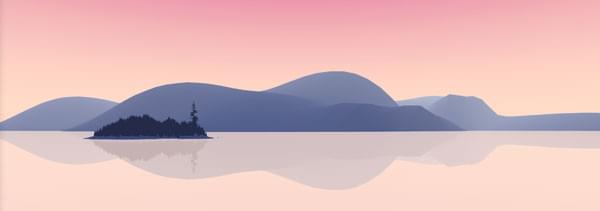
WebVR — 网络虚拟现实
推荐:使用 NSDT编辑器 快速搭建3D应用场景 虚拟现实设备 随着Oculus Rift和许多其他生产设备即将上市,未来看起来很光明——我们已经有足够的技术来使VR体验“足够好”,可以玩游戏。有许多设备可供选择:像Oculus Rift或HTC Vive这…...

ASP.NET Core 的 Routing
ASP.NET Core 的 Routing ASP.NET Core 的 controllers 使用Routing 中间件匹配客户端的 url 请求,然后映射到对应的 controller 的处理方法(Action)上。 Actions 可以是 常规路由 或 属性路由 的映射。 MVC App一般使用常规路由。 REST API…...
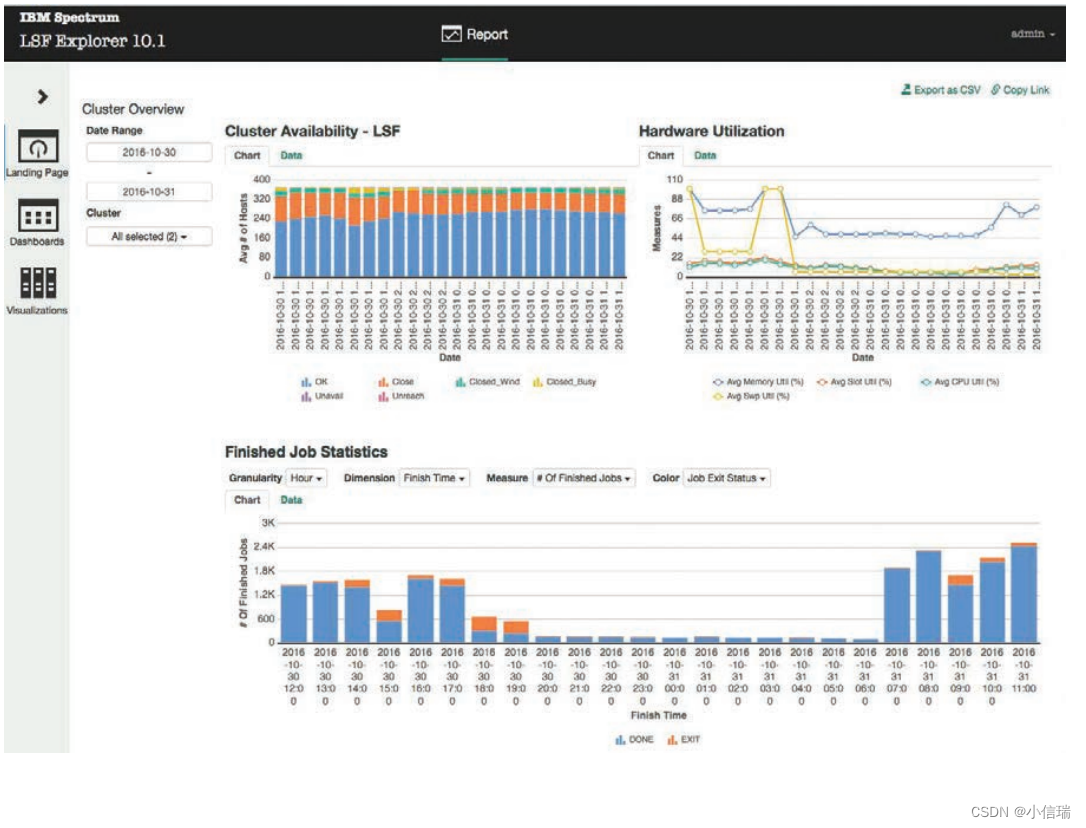
IBM Spectrum LSF Explorer 为要求苛刻的分布式和任务关键型高性能技术计算环境提供强大的工作负载管理
IBM Spectrum LSF Explorer 适用于 IBM Spectrum LSF 集群的强大、轻量级报告解决方案 亮点 ● 允许不同的业务和技术用户使用单一解决方案快速创建和查看报表和仪表板 ● 利用可扩展的库提供预构建的报告 ● 自定义并生成性能、工作负载和资源使用情况的报…...
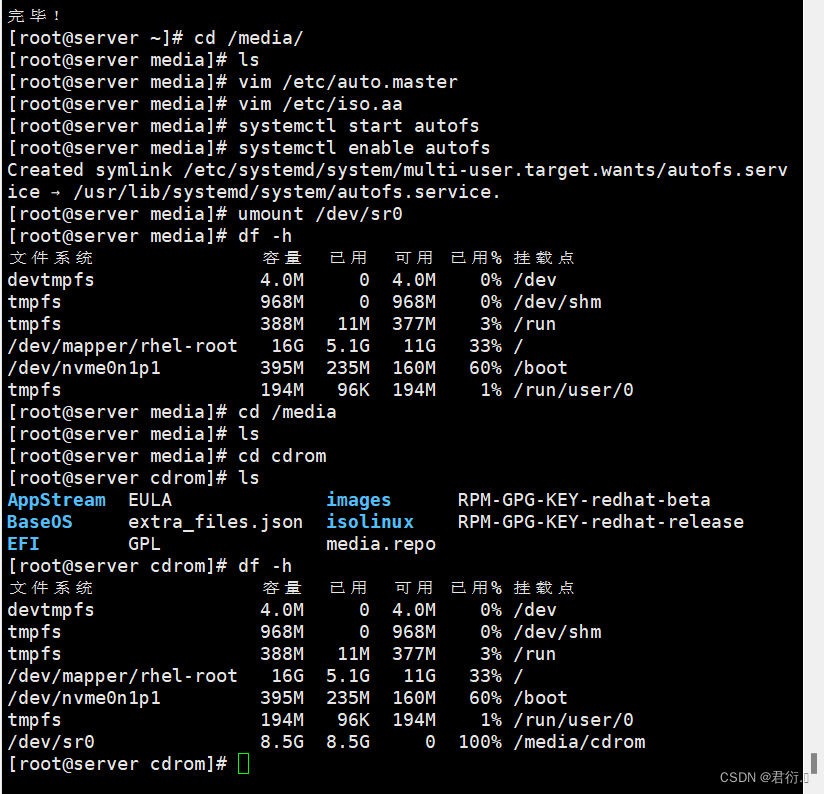
RHCE——十一、NFS服务器
NFS服务器 一、简介1、NFS背景介绍2、生产应用场景 二、NFS工作原理1、示例图2、流程 三、NFS的使用1、安装2、配置文件3、主配置文件分析3.1 实验1 4、NFS账户映射4.1 实验24.2 实验3 四、autofs自动挂载服务1、产生原因2、安装3、配置文件分析4、实验45、实验5 一、简介 1、…...

Python编程练习与解答 练习100:随机密码
编写一个生成最忌密码的函数,密码的长度应该在7-10个字符之间。每个字符应该从ASCII表的第33位到126位中随机选择。函数不接受任何参数,返回随机生成的密码作为位移结果。在文件的main程序中显示随机生成的密码。main程序只在解答没有被导入另一个文件时…...
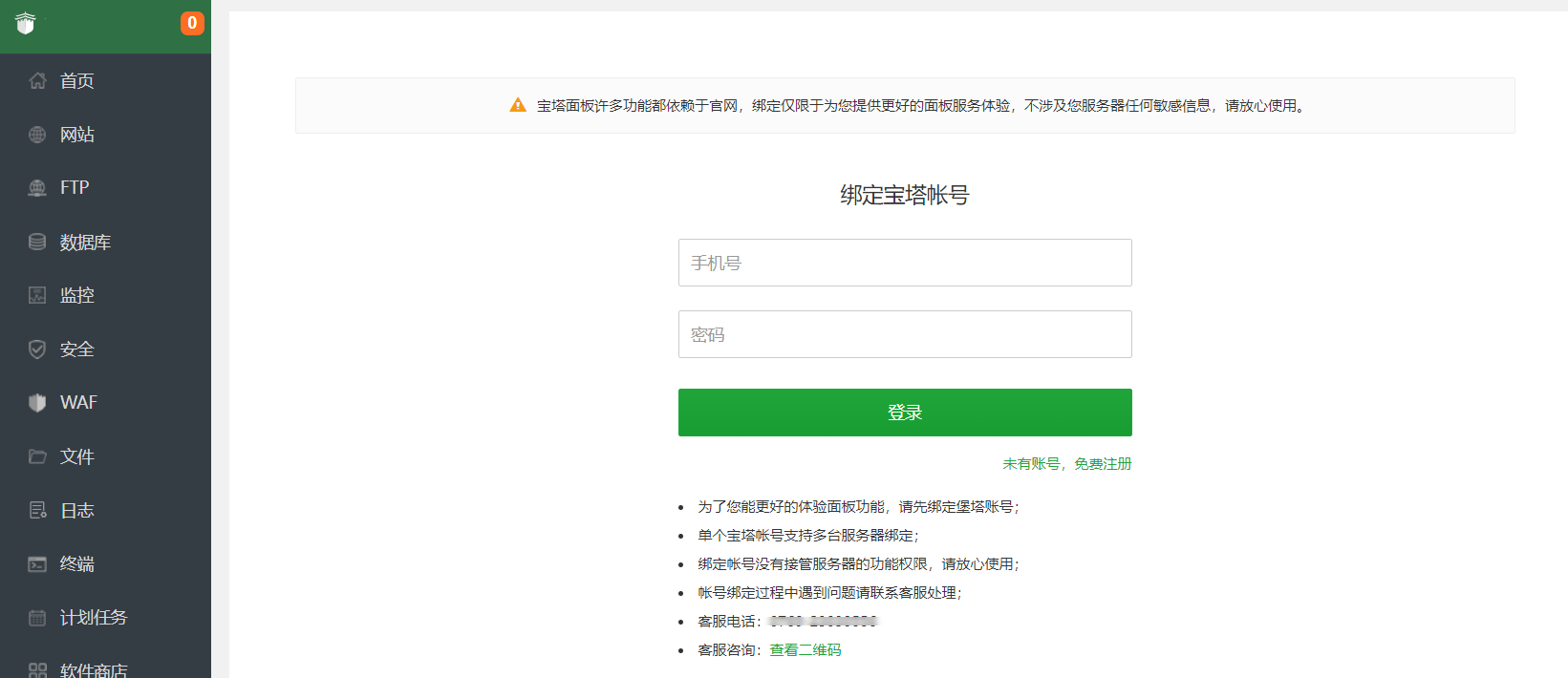
华为云云服务器评测 | 从零开始:云耀云服务器L实例的全面使用解析指南
文章目录 一、前言二、云耀云服务器L实例要点介绍2.1 什么是云耀云服务器L实例2.1.1 浅析云耀云服务器L实例 2.2 云耀云服务器L实例的产品定位2.3 云耀云服务器L实例优势2.4 云耀云服务器L实例支持的镜像与应用场景2.5 云耀云服务器L实例与弹性云服务器(ECS…...
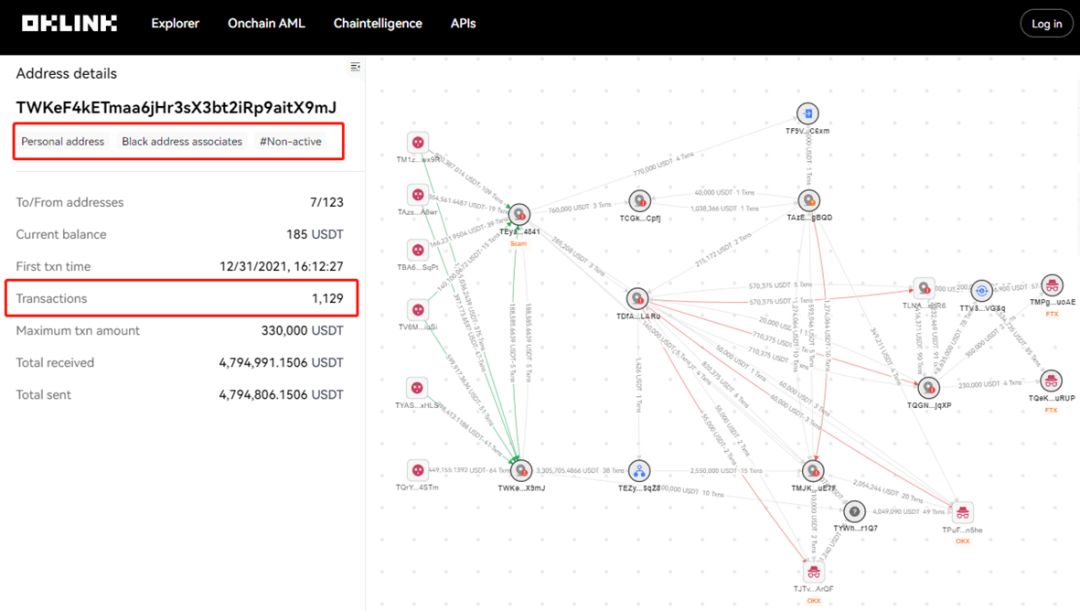
欧科云链研究院探析Facebook稳定币发行经历会不会在PayPal重演
引言 作者最近的报告-探析PayPal发行稳定币是否会重蹈Facebook覆辙-近期被英国的金融时报(中文版)刊登。由于该报告在欧科云链研究院内部反响较好,下面就带大家简单的剖析这篇报告的主要内容。 *这篇文章主要由对比分析(已删减&a…...

docker 容器pip、git安装异常;容器内web对外端口ping不通
1、docker 容器pip、git安装异常 错误信息: git clone https://github.com/vllm-project/vllm.git Cloning into ‘vllm’… fatal: unable to access ‘https://github.com/vllm-project/vllm.git/’: Failed to connect to 127.0.0.1 port 10808: Connection ref…...
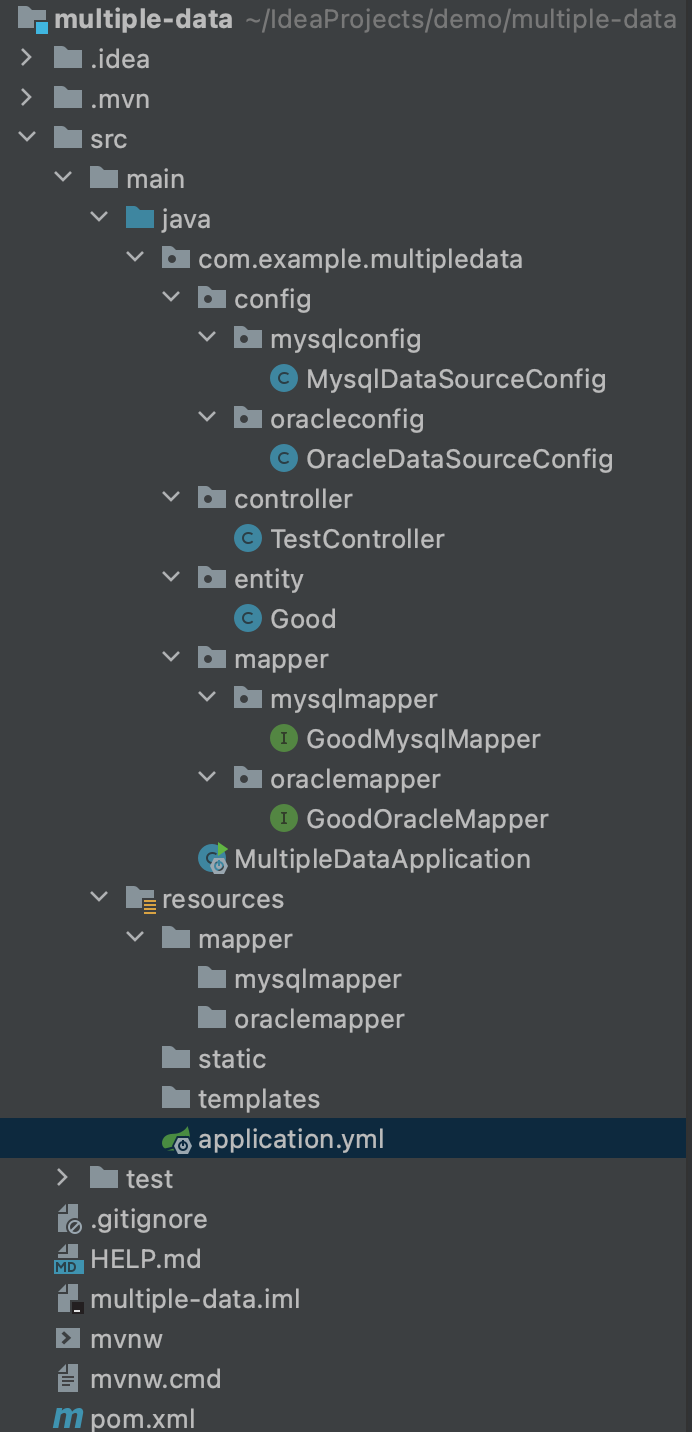
SpringBoot Mybatis 多数据源 MySQL+Oracle+Redis
一、背景 在SpringBoot Mybatis 项目中,需要连接 多个数据源,连接多个数据库,需要连接一个MySQL数据库和一个Oracle数据库和一个Redis 二、依赖 pom.xml <dependencies><dependency><groupId>org.springframework.boot&l…...

【JavaScript 16】对象继承 原型对象属性 原型链 构造函数属性 instanceof运算符 继承 多重继承 模块
对象继承 原型对象概述instanceof运算符构造函数的继承多重继承模块 A 对象通过继承 B 对象,就能 直接拥有 B 对象的所有属性和方法(利于代码复用) 大部分面向对象的编程语言都是通过类(class)实现对象的继承 但 传统…...
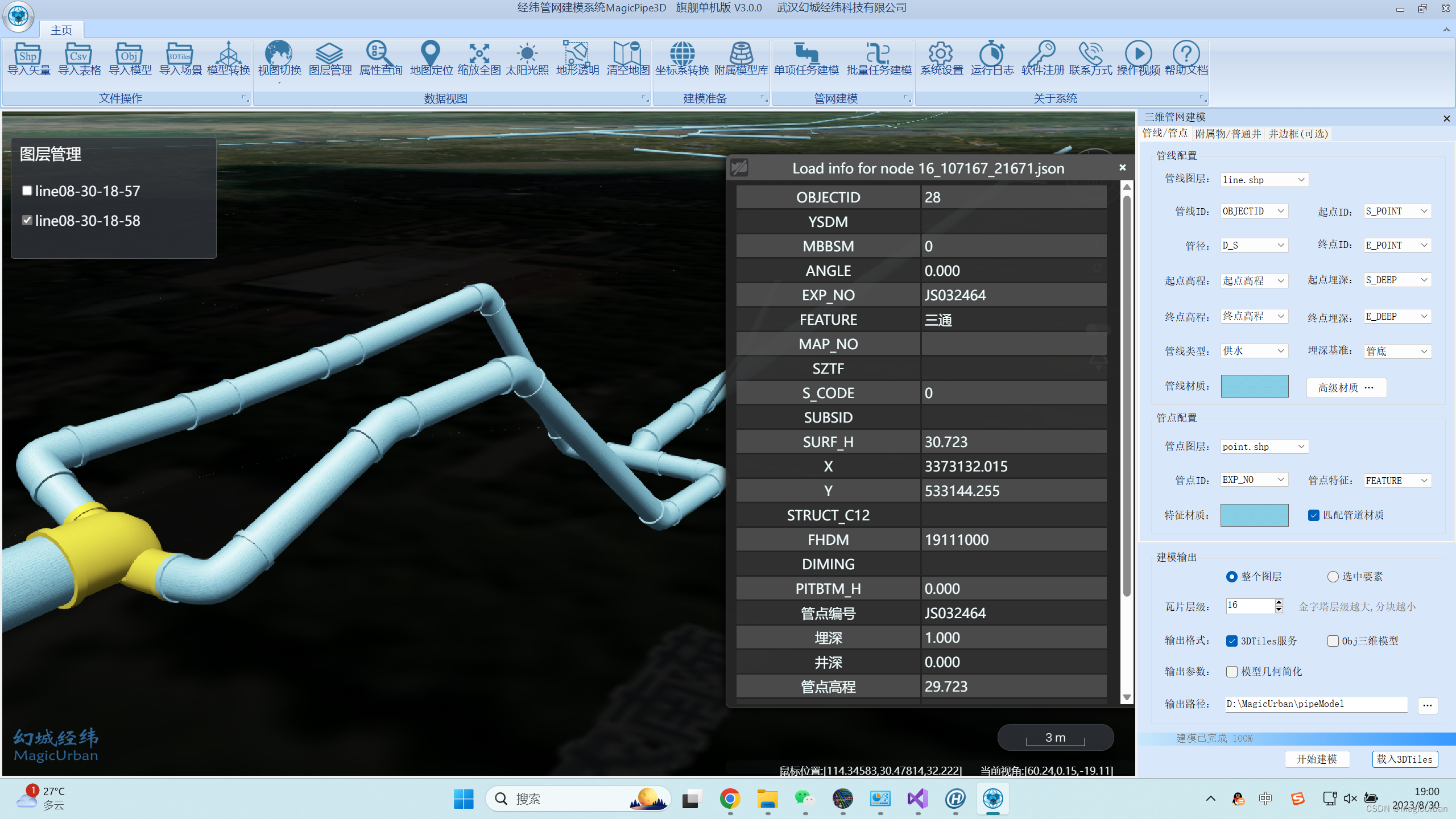
地下管线三维自动建模软件MagicPipe3D V3.0发布
2023年9月1日经纬管网建模系统MagicPipe3D V3.0正式发布,该版本经过众多用户应用和反馈,在三维地下管线建模效果、效率、适配性等方面均有显著提升!MagicPipe3D本地离线参数化构建地下管网模型(包括管道、接头、附属设施等&#x…...
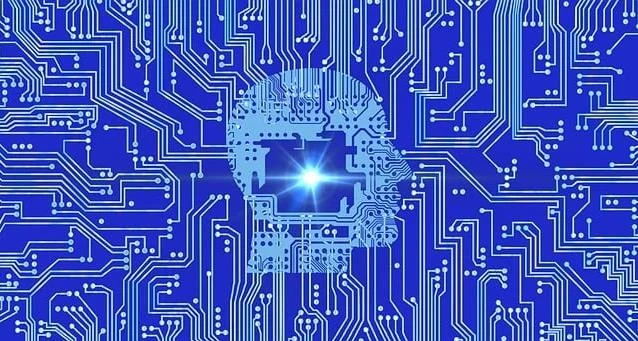
百度等8家企业首批上线大模型服务;大语言模型微调之道
🦉 AI新闻 🚀 百度等8家企业首批上线大模型服务 摘要:百度、字节、中科院旗下8家企业/机构的大模型通过备案,正式面向公众提供服务。百度旗下AI大模型产品文心一言率先开放,用户可下载App或登录官网体验。百川智能也…...
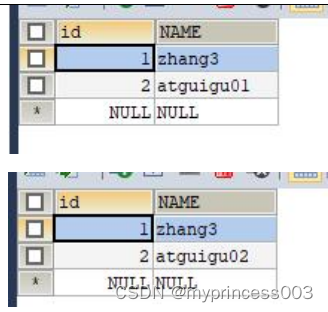
二、Mycat2 相关概念及读写分离
第三章 Mycat2 相关概念 3.1 概念描述 1、分库分表 按照一定规则把数据库中的表拆分为多个带有数据库实例,物理库,物理表访问路 径的分表。 解读:分库:一个电商项目,分为用户库、订单库等等。 分表:一张订单表数据数百万ÿ…...
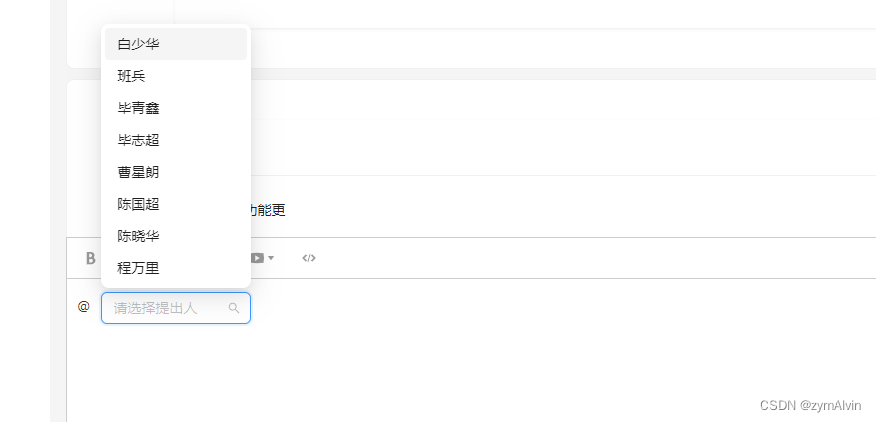
react利用wangEditor写评论和@功能
先引入wangeditor写评论功能 import React, { useEffect, useState, useRef, forwardRef, useImperativeHandle } from react; import wangeditor/editor/dist/css/style.css; import { Editor, Toolbar } from wangeditor/editor-for-react; import { Button, Card, Col, For…...
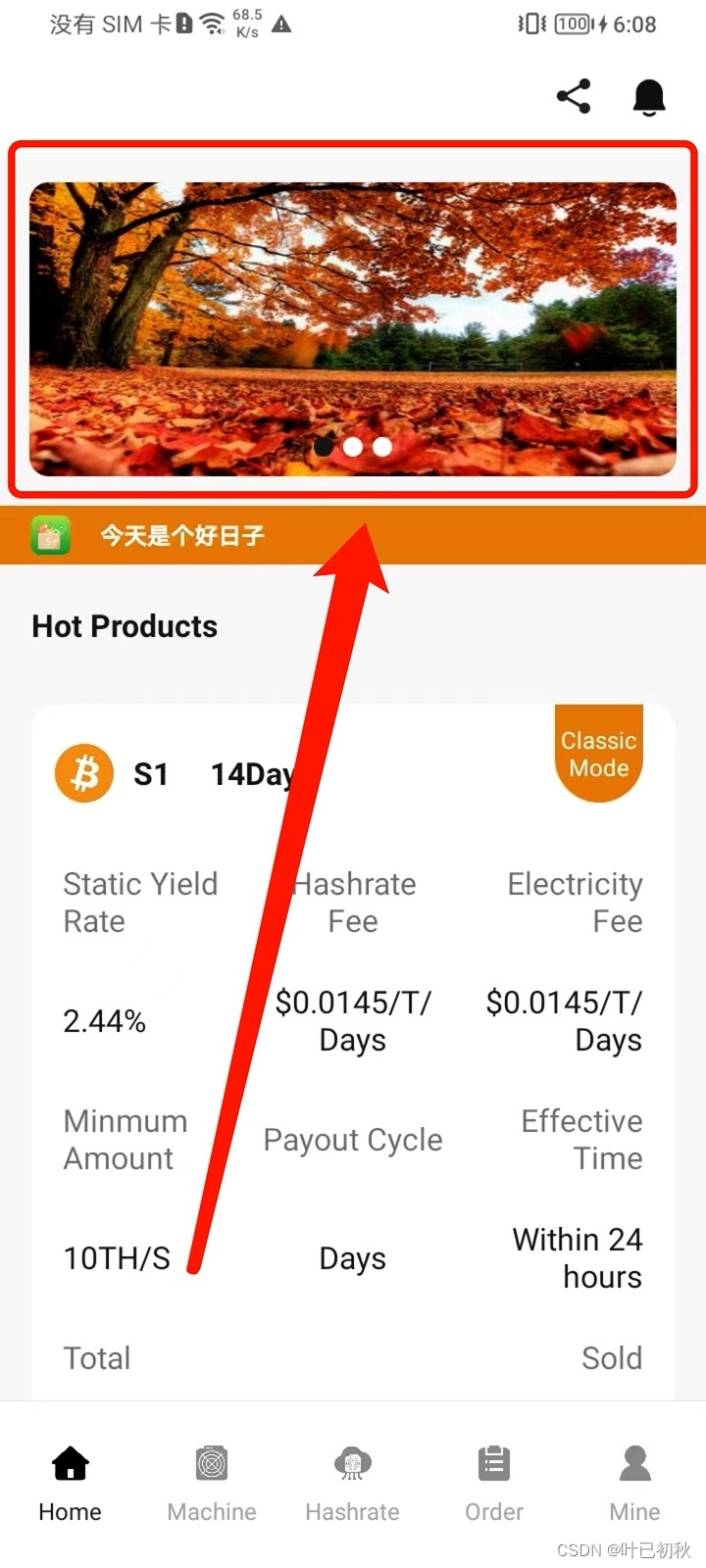
Android之布局转圆角
Android之布局转圆角 文章目录 Android之布局转圆角说明一、效果图二、实现步骤1.自定义RoundRelativeLayout2.使用 总结 说明 很多需求比较无语,需要某个布局转圆角,像个显眼包一样,所以为了满足显眼包,必须整呐提示:…...

Linux的目录结构特点
Linux的目录结构特点 1、使用树形目录结构来组织和管理文件。 2、整个系统只有一个根目录(树根),Linux的根目录用“/”表示。 3、其他所有分区以及外部设备(如硬盘,光驱等)都是以根目录为起点࿰…...
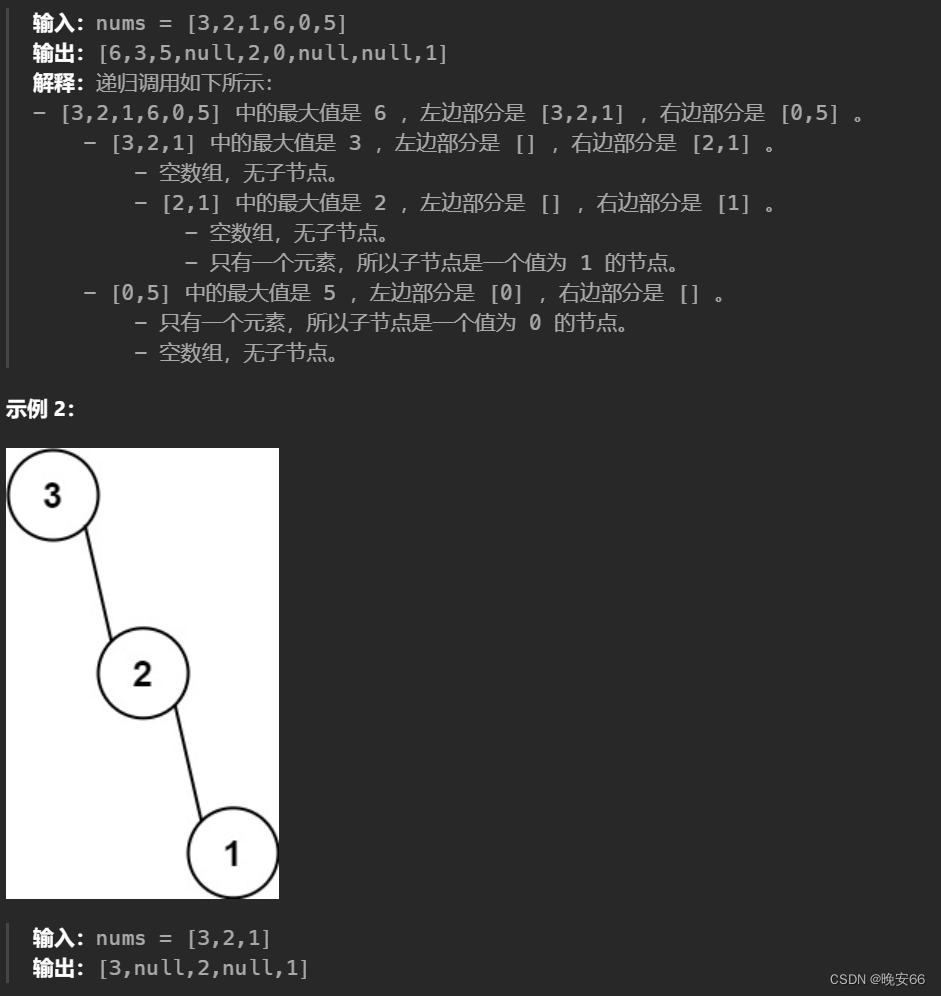
【算法与数据结构】654、LeetCode最大二叉树
文章目录 一、题目二、解法三、完整代码 所有的LeetCode题解索引,可以看这篇文章——【算法和数据结构】LeetCode题解。 一、题目 二、解法 思路分析:【算法与数据结构】106、LeetCode从中序与后序遍历序列构造二叉树这两道题有些类似,相关代…...
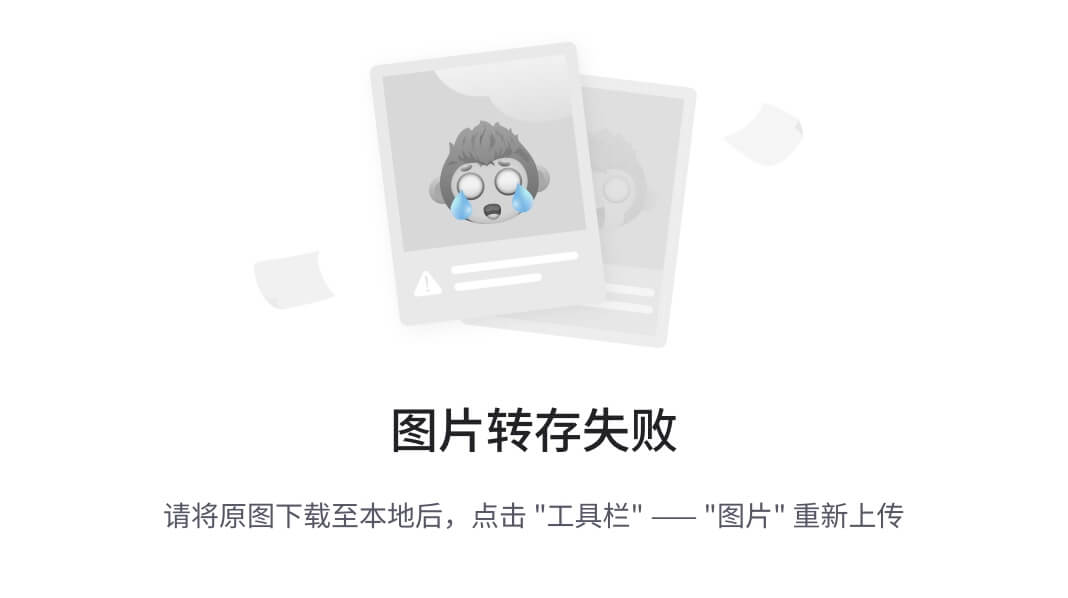
您必须尝试的 4 种经典特征提取技术!
一、说明 特征提取如何实现?其手段并不是很多,有四个基本方法,作为AI工程师不能不知。因此,本篇将对四种特征提取给出系统的方法。 二、概述 图像分类长期以来一直是计算机视觉领域的热门话题,并希望能够保持这种状态。…...
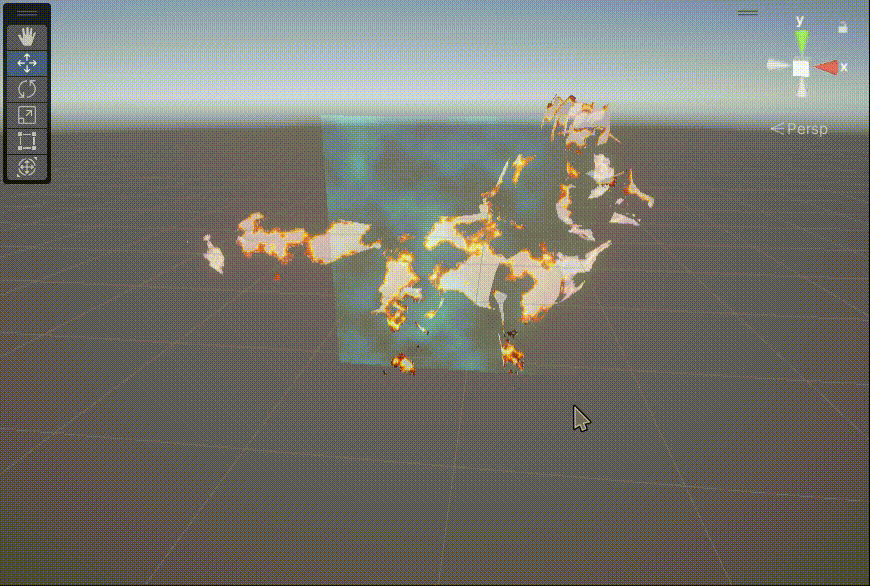
Unity中Shader的遮罩的实现
文章目录 前言一、遮罩效果的实现主要是使用对应的纹理实现的,在属性中暴露对应的遮罩纹理,对其进行采样后,最后相乘输出即可二、如果需要像和主要纹理一样流动,则需要使用和_Time篇一样的方法实现流动即可 前言 Unity中Shader的…...

架构师成长之路|Redis key过期清除策略
Eviction policies maxmemory 100mb 当我们设置的内存达到指定的内存量时,清除策略的配置方式决定了默认行为。Redis可以为可能导致使用更多内存的命令返回错误,也可以在每次添加新数据时清除一些旧数据以返回到指定的限制。 当达到最大内存限制时,Redis所遵循的确切行为是…...