NLP 项目:维基百科文章爬虫和分类【01】 - 语料库阅读器
自然语言处理是机器学习和人工智能的一个迷人领域。这篇博客文章启动了一个具体的 NLP 项目,涉及使用维基百科文章进行聚类、分类和知识提取。灵感和一般方法源自《Applied Text Analysis with Python》一书。
一、说明
该文是系列文章,揭示如何对爬取文本进行文本处理的全过程。在接下来的文章中,我将展示如何实现维基百科文章爬虫,如何将文章收集到语料库中,如何应用文本预处理、标记化、编码和矢量化,以及最后应用机器学习算法进行聚类和分类。
本文的技术背景是Python v3.11
和几个附加库,其中最重要的nltk v3.8.1
是 和wikipedia-api v0.6.0
。所有示例也应该适用于较新的版本。
二、项目概要
该项目的目标是下载、处理和应用维基百科文章上的机器学习算法。首先,下载并存储来自维基百科的选定文章。其次,生成一个语料库,即所有文本文档的总和。第三,对每个文档文本进行预处理,例如通过删除停用词和符号,然后进行标记化。第四,将标记化文本转换为向量以接收数字表示。最后,应用不同的机器学习算法。
在第一篇文章中,解释了步骤一和步骤二。
2.1 先决条件
我喜欢在Jupyter Notebook中工作并使用优秀的依赖管理器Poetry。在您选择的项目文件夹中运行以下命令以安装所有必需的依赖项并在浏览器中启动 Jupyter 笔记本。
# Complete the interactive project creation
poetry init# Add core dependencies
poetry add nltk@^3.8.1 jupyterlab@^4.0.0 scikit-learn@^1.2.2 wikipedia-api@^0.5.8 matplotlib@^3.7.1 numpy@^1.24.3 pandas@^2.0.1# Add NLTK dependencies
python3 -c "import nltk; \nltk.download('punkt'); \nltk.download('averaged_perceptron_tagger'); \nltk.download('reuters'); \nltk.download('stopwords');"# Start jupyterhub
poetry run jupyterlab
浏览器中应该会打开一个新的 Jupyter Notebook。
2.2 Python 库
在这篇博文中,将使用以下 Python 库:
- 维基百科-API:
Page
代表维基百科文章及其标题、文本、类别和相关页面的对象。
- NLTK
PlaintextCorpusReader
用于提供对文档的访问、提供标记化方法并计算有关所有文件的统计信息的可遍历对象sent_tokenizer
并word_tokenizer
用于生成令牌
三、第 1 部分:维基百科文章爬虫
该项目从创建自定义维基百科爬虫开始。尽管我们可以使用来自各种来源的维基百科语料库数据集(例如 NLTK 中的内置语料库),但自定义爬虫提供了对文件格式、内容和内容现实的最佳控制。
下载和处理原始 HTML 可能非常耗时,尤其是当我们还需要从中确定相关链接和类别时。一个非常方便的图书馆可以帮助您。wikipedia -api为我们完成了所有这些繁重的工作。在此基础上,我们逐步开发核心功能。
首先,我们创建一个基类,定义它自己的 Wikipedia 对象并确定存储文章的位置。
import os
import re
import wikipediaapi as wiki_apiclass WikipediaReader():def __init__(self, dir = "articles"):self.pages = set()self.article_path = os.path.join("./", dir)self.wiki = wiki_api.Wikipedia(language = 'en',extract_format=wiki_api.ExtractFormat.WIKI)try:os.mkdir(self.article_path)except Exception as e:pass
这还定义了pages
爬虫访问的一组页面对象。该page
对象非常有用,因为它可以访问文章标题、文本、类别和其他页面的链接。
其次,我们需要接收文章名称的辅助方法,如果存在,它将page
向集合中添加一个新对象。我们需要将调用包装在一个try except
块中,因为某些包含特殊字符的文章无法正确处理,例如Add article 699/1000 Tomasz Imieliński
. 此外,还有一些我们不需要存储的元文章。
def add_article(self, article):try:page = self.wiki.page(self._get_page_title(article))if page.exists():self.pages.add(page)return(page)except Exception as e:print(e)
第三,我们要提取一篇文章的类别。每篇维基百科文章都在页面底部的两个可见部分(请参阅以下屏幕截图)以及未呈现为 HTML 的元数据中定义类别。因此,最初的类别列表可能听起来令人困惑。看一下这个例子:
wr = WikipediaReader()
wr.add_article("Machine Learning")
ml = wr.list().pop()print(ml.categories)
# {'Category:All articles with unsourced statements': Category:All articles with unsourced statements (id: ??, ns: 14),
# 'Category:Articles with GND identifiers': Category:Articles with GND identifiers (id: ??, ns: 14),
# 'Category:Articles with J9U identifiers': Category:Articles with J9U identifiers (id: ??, ns: 14),
# 'Category:Articles with LCCN identifiers': Category:Articles with LCCN identifiers (id: ??, ns: 14),
# 'Category:Articles with NDL identifiers': Category:Articles with NDL identifiers (id: ??, ns: 14),
# 'Category:Articles with NKC identifiers': Category:Articles with NKC identifiers (id: ??, ns: 14),
# 'Category:Articles with short description': Category:Articles with short description (id: ??, ns: 14),
# 'Category:Articles with unsourced statements from May 2022': Category:Articles with unsourced statements from May 2022 (id: ??, ns: 14),
# 'Category:Commons category link from Wikidata': Category:Commons category link from Wikidata (id: ??, ns: 14),
# 'Category:Cybernetics': Category:Cybernetics (id: ??, ns: 14),
# 'Category:Learning': Category:Learning (id: ??, ns: 14),
# 'Category:Machine learning': Category:Machine learning (id: ??, ns: 14),
# 'Category:Short description is different from Wikidata': Category:Short description is different from Wikidata (id: ??, ns: 14),
# 'Category:Webarchive template wayback links': Category:Webarchive template wayback links (id: ??, ns: 14)}
因此,我们根本不通过应用多个正则表达式过滤器来存储这些特殊类别。
def get_categories(self, title):page = self.add_article(title)if page:if (list(page.categories.keys())) and (len(list(page.categories.keys())) > 0):categories = [c.replace('Category:','').lower() for c in list(page.categories.keys())if c.lower().find('articles') == -1and c.lower().find('pages') == -1and c.lower().find('wikipedia') == -1and c.lower().find('cs1') == -1and c.lower().find('webarchive') == -1and c.lower().find('dmy dates') == -1and c.lower().find('short description') == -1and c.lower().find('commons category') == -1]return dict.fromkeys(categories, 1)return {}
第四,我们现在定义抓取方法。这是一种可定制的广度优先搜索,从一篇文章开始,获取所有相关页面,将这些页面广告到页面对象,然后再次处理它们,直到文章总数耗尽或达到深度级别。说实话:我只用它爬过 1000 篇文章。
def crawl_pages(self, article, depth = 3, total_number = 1000):print(f'Crawl {total_number} :: {article}')page = self.add_article(article)childs = set()if page:for child in page.links.keys():if len(self.pages) < total_number:print(f'Add article {len(self.pages)}/{total_number} {child}')self.add_article(child)childs.add(child)depth -= 1if depth > 0:for child in sorted(childs):if len(self.pages) < total_number:self.crawl_pages(child, depth, len(self.pages))
让我们开始爬取机器学习文章:
reader = WikipediaReader()
reader.crawl_pages("Machine Learning")print(reader.list())
# Crawl 1000 :: Machine Learning
# Add article 1/1000 AAAI Conference on Artificial Intelligence
# Add article 2/1000 ACM Computing Classification System
# Add article 3/1000 ACM Computing Surveys
# Add article 4/1000 ADALINE
# Add article 5/1000 AI boom
# Add article 6/1000 AI control problem
# Add article 7/1000 AI safety
# Add article 8/1000 AI takeover
# Add article 9/1000 AI winter
最后,当一组page
对象可用时,我们提取它们的文本内容并将它们存储在文件中,其中文件名代表其标题的清理版本。需要注意的是:文件名需要保留其文章名称的投降,否则我们无法再次获取页面对象,因为使用小写文章名称的搜索不会返回结果。
def process(self, update=False):for page in self.pages:filename = re.sub('\s+', '_', f'{page.title}')filename = re.sub(r'[\(\):]','', filename)file_path = os.path.join(self.article_path, f'{filename}.txt')if update or not os.path.exists(file_path):print(f'Downloading {page.title} ...')content = page.textwith open(file_path, 'w') as file:file.write(content)else:print(f'Not updating {page.title} ...')
这是类WikipediaReader
的完整源代码。
import os
import re
import wikipediaapi as wiki_apiclass WikipediaReader():def __init__(self, dir = "articles"):self.pages = set()self.article_path = os.path.join("./", dir)self.wiki = wiki_api.Wikipedia(language = 'en',extract_format=wiki_api.ExtractFormat.WIKI)try:os.mkdir(self.article_path)except Exception as e:passdef _get_page_title(self, article):return re.sub(r'\s+','_', article)def add_article(self, article):try:page = self.wiki.page(self._get_page_title(article))if page.exists():self.pages.add(page)return(page)except Exception as e:print(e)def list(self):return self.pagesdef process(self, update=False):for page in self.pages:filename = re.sub('\s+', '_', f'{page.title}')filename = re.sub(r'[\(\):]','', filename)file_path = os.path.join(self.article_path, f'{filename}.txt')if update or not os.path.exists(file_path):print(f'Downloading {page.title} ...')content = page.textwith open(file_path, 'w') as file:file.write(content)else:print(f'Not updating {page.title} ...')def crawl_pages(self, article, depth = 3, total_number = 1000):print(f'Crawl {total_number} :: {article}')page = self.add_article(article)childs = set()if page:for child in page.links.keys():if len(self.pages) < total_number:print(f'Add article {len(self.pages)}/{total_number} {child}')self.add_article(child)childs.add(child)depth -= 1if depth > 0:for child in sorted(childs):if len(self.pages) < total_number:self.crawl_pages(child, depth, len(self.pages))def get_categories(self, title):page = self.add_article(title)if page:if (list(page.categories.keys())) and (len(list(page.categories.keys())) > 0):categories = [c.replace('Category:','').lower() for c in list(page.categories.keys())if c.lower().find('articles') == -1and c.lower().find('pages') == -1and c.lower().find('wikipedia') == -1and c.lower().find('cs1') == -1and c.lower().find('webarchive') == -1and c.lower().find('dmy dates') == -1and c.lower().find('short description') == -1and c.lower().find('commons category') == -1]return dict.fromkeys(categories, 1)return {}
让我们使用维基百科爬虫来下载与机器学习相关的文章。
reader = WikipediaReader()
reader.crawl_pages("Machine Learning")
print(reader.list())
# Downloading The Register ...
# Not updating Bank ...
# Not updating Boosting (machine learning) ...
# Not updating Ian Goodfellow ...
# Downloading Statistical model ...
# Not updating Self-driving car ...
# Not updating Behaviorism ...
# Not updating Statistical classification ...
# Downloading Search algorithm ...
# Downloading Support vector machine ...
# Not updating Deep learning speech synthesis ...
# Not updating Expert system ...s
四、第 2 部分:维基百科语料库
所有文章均以文本文件形式下载到article
文件夹中。为了提供所有这些单独文件的抽象,NLTK 库提供了不同的语料库阅读器对象。该对象不仅提供对单个文件的快速访问,还可以生成统计信息,例如词汇量、单个标记的总数或单词量最多的文档。
让我们使用该类PlaintextCorpusReader
作为起点,然后初始化它,使其指向文章:
import nltk
from nltk.corpus.reader.plaintext import PlaintextCorpusReader
from time import timeclass WikipediaCorpus(PlaintextCorpusReader):passcorpus = WikipediaCorpus('articles', r'[^\.ipynb].*', cat_pattern=r'[.*]')
print(corpus.fileids())# ['2001_A_Space_Odyssey.txt',
# '2001_A_Space_Odyssey_film.txt',
# '2001_A_Space_Odyssey_novel.txt',
# '3D_optical_data_storage.txt',
# 'A*_search_algorithm.txt',
# 'A.I._Artificial_Intelligence.txt',
# 'AAAI_Conference_on_Artificial_Intelligence.txt',
# 'ACM_Computing_Classification_System.txt',
好的,这已经足够好了。让我们用两种方法来扩展它来计算词汇量和最大单词数。对于词汇,我们将使用 NLTK 辅助类FreqDist
,它是一个包含所有单词出现的字典对象,此方法使用简单辅助类消耗所有文本corpus.words()
,从中删除非文本和非数字。
def vocab(self):return nltk.FreqDist(re.sub('[^A-Za-z0-9,;\.]+', ' ', word).lower() for word in corpus.words())
为了得到最大单词数,我们遍历所有带有 的文档fileids()
,然后确定 的长度words(doc)
,并记录最高值
def max_words(self):max = 0for doc in self.fileids():l = len(self.words(doc))max = l if l > max else maxreturn max
最后,我们添加一个describe
生成统计信息的方法(这个想法也源于上面提到的《Applied Text Analysis with Python》一书)。
该方法启动一个计时器来记录校园处理持续了多长时间,然后使用语料库阅读器对象的内置方法和刚刚创建的方法来计算文件数、段落数、句子数、单词数、词汇量和文档中的最大字数。
def describe(self, fileids=None, categories=None):started = time()return {'files': len(self.fileids()),'paras': len(self.paras()),'sents': len(self.sents()),'words': len(self.words()),'vocab': len(self.vocab()),'max_words': self.max_words(),'time': time()-started}pass
这是最后一WikipediaCorpus
堂课:
import nltk
from nltk.corpus.reader.plaintext import PlaintextCorpusReader
from time import timeclass WikipediaCorpus(PlaintextCorpusReader):def vocab(self):return nltk.FreqDist(re.sub('[^A-Za-z0-9,;\.]+', ' ', word).lower() for word in corpus.words())def max_words(self):max = 0for doc in self.fileids():l = len(self.words(doc))max = l if l > max else maxreturn maxdef describe(self, fileids=None, categories=None):started = time()return {'files': len(self.fileids()),'paras': len(self.paras()),'sents': len(self.sents()),'words': len(self.words()),'vocab': len(self.vocab()),'max_words': self.max_words(),'time': time()-started}pass
在撰写本文时,爬取维基百科有关人工智能和机器学习的文章后,可以获得以下统计数据:
corpus = WikipediaCorpus('articles', r'[^\.ipynb].*', cat_pattern=r'[.*]')
corpus.describe()
{'files': 1163,'paras': 96049,'sents': 238961,'words': 4665118,'vocab': 92367,'max_words': 46528,'time': 32.60307598114014}
五、结论
本文是 NLP 项目在维基百科文章上下载、处理和应用机器学习算法的起点。本文涵盖了两个方面。首先,创建WikipediaReader
通过名称查找文章的类,并可以提取其标题、内容、类别和提到的链接。爬虫由两个变量控制:爬取的文章总数和爬取的深度。其次,WikipediaCorpus在
NLTK 的扩展PlaintextCorpusReader
。该对象可以方便地访问单个文件、句子和单词,以及总语料库数据,例如文件数量或词汇、唯一标记的数量。下一篇文章将继续构建文本处理管道。
相关文章:
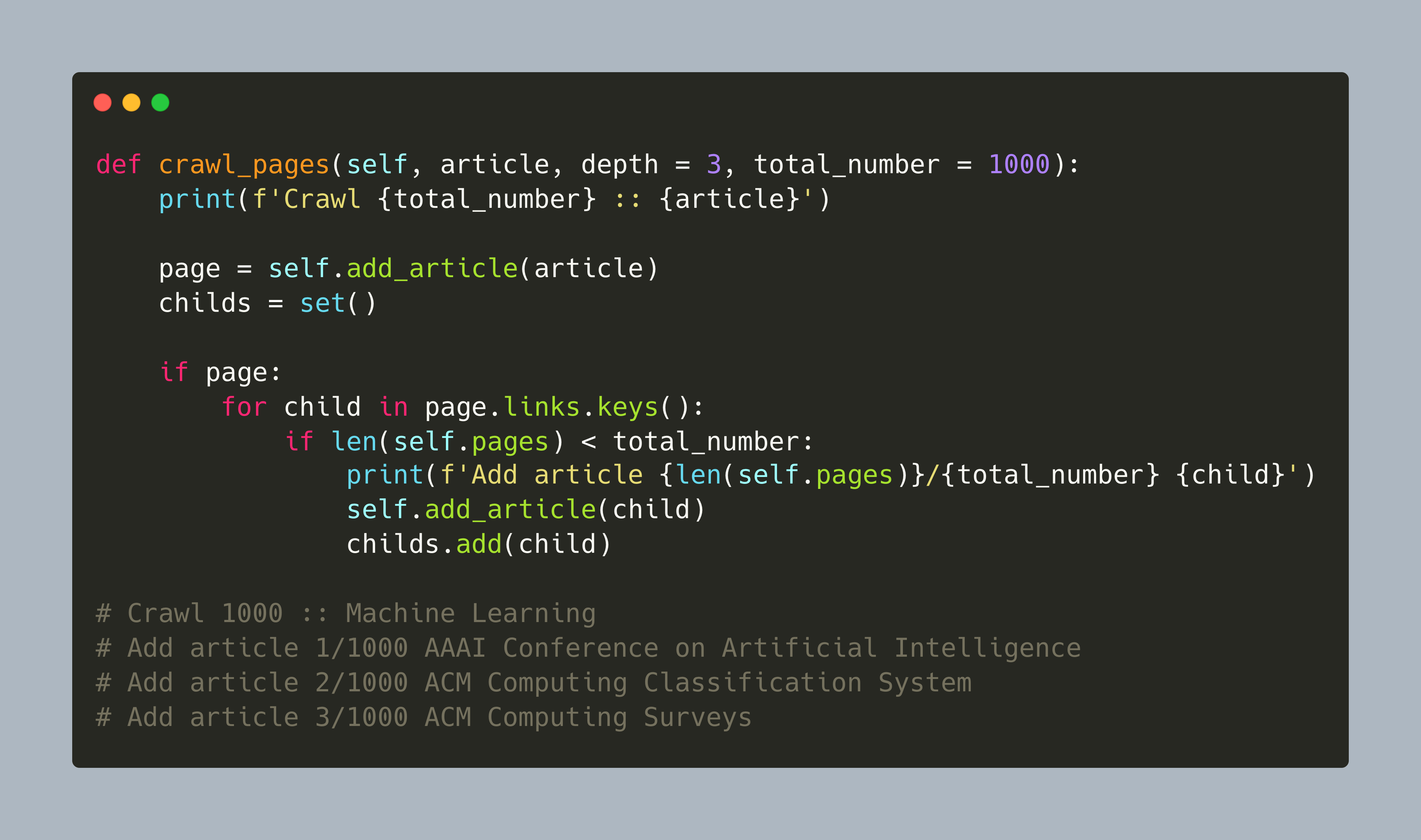
NLP 项目:维基百科文章爬虫和分类【01】 - 语料库阅读器
自然语言处理是机器学习和人工智能的一个迷人领域。这篇博客文章启动了一个具体的 NLP 项目,涉及使用维基百科文章进行聚类、分类和知识提取。灵感和一般方法源自《Applied Text Analysis with Python》一书。 一、说明 该文是系列文章,揭示如何对爬取文…...

QT sqlite的简单用法
1、相关头文件 #include <QSqlDatabase> #include <QSqlError> #include <QSqlQuery> #include <QSqlRecord> #include <QSqlIndex> #include <QSqlField> #include <QFile> #include <QDebug> 2、数据库对象 QSqlDatabas…...
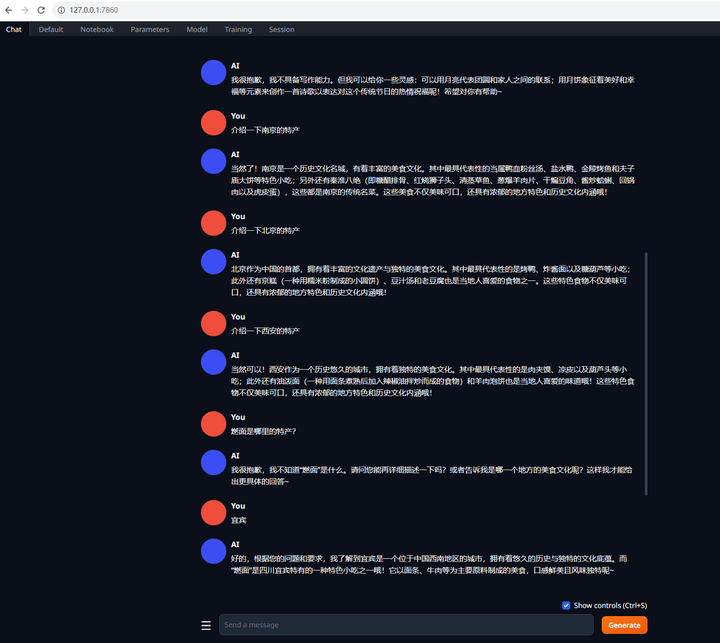
大模型部署手记(12)LLaMa2+Chinese-LLaMA-Plus-2-7B+Windows+text-gen+中文对话
1.简介: 组织机构:Meta(Facebook) 代码仓:https://github.com/facebookresearch/llama 模型:chinese-alpaca-2-7b-hf 下载:使用百度网盘下载 硬件环境:暗影精灵7Plus Windows版…...

C#导出本机Win32native dll
C# 使用 "3f/DllExport" 工具导出C风格的本机函数 [文 / 张赐荣] 首先,让我们来了解一下什么是争渡读屏软件,以及什么是争渡文本预处理API。争渡读屏软件是一款屏幕朗读软件,用于协助视力障碍人士操作电脑。 争渡文本预处理API是一…...
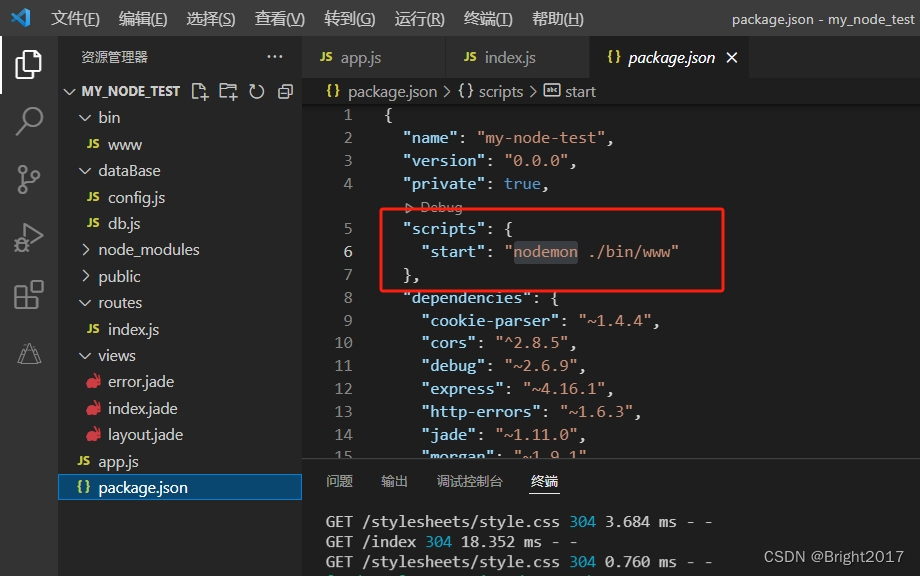
express-generator快速构建node后端项目
express-generator是express官方团队开发者准备的一个快速生成工具,可以非常快速的生成一个基于express开发的框架基础应用。 npm安装 npm install express-generator -g初始化应用 express my_node_test 创建了一个名为 my_node_test 的express骨架项目通过 Exp…...
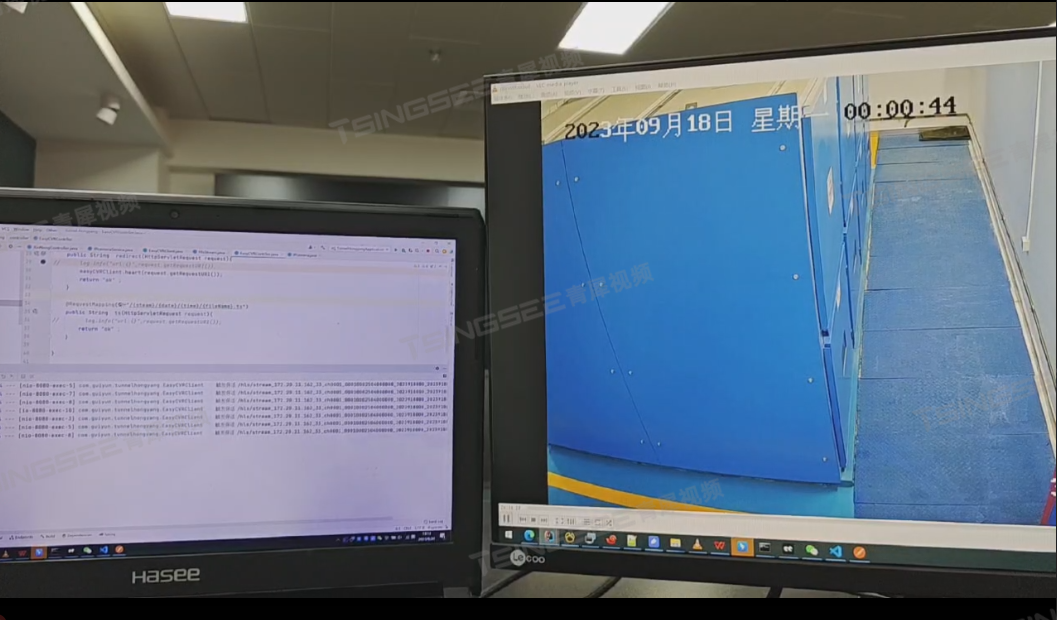
视频监控系统/视频汇聚平台EasyCVR如何反向代理进行后端保活?
安防视频监控/视频集中存储/云存储/磁盘阵列EasyCVR平台可拓展性强、视频能力灵活、部署轻快,可支持的主流标准协议有国标GB28181、RTSP/Onvif、RTMP等,以及支持厂家私有协议与SDK接入,包括海康Ehome、海大宇等设备的SDK等。平台既具备传统安…...
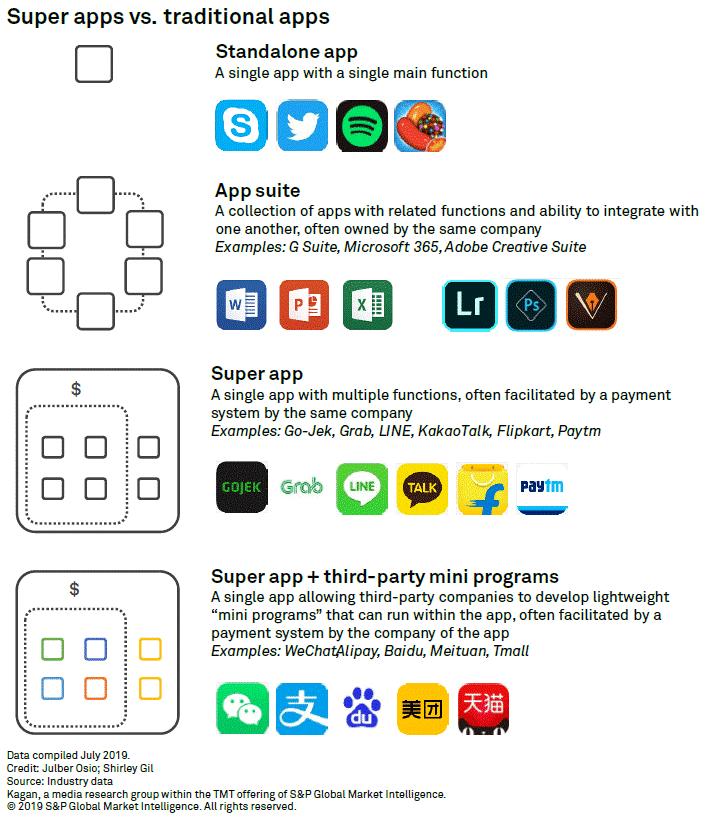
金融信创黄金三年:小程序生态+跨端技术框架构建
小程序应用场景生态的发展,受益于开源技术的发展,以及响应快速开发的实际业务需求,一些跨端框架如:Electron、wxPython、FinClip、Tauri、Flutter等发展也非常迅速,小程序生态跨端技术框架,不仅能满足自有超…...
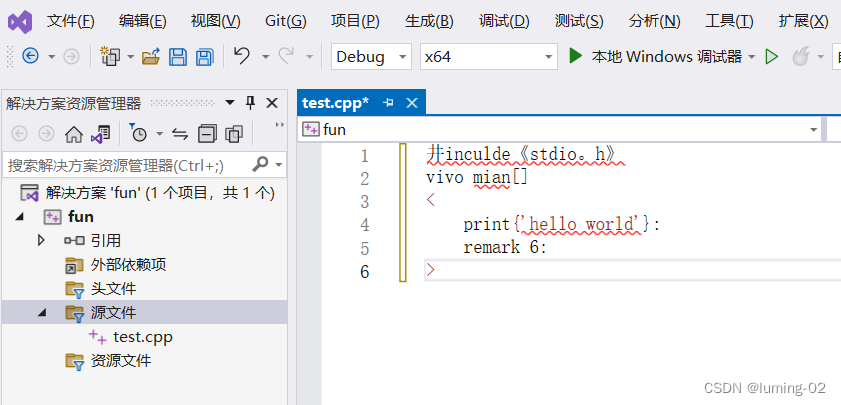
这短短 6 行代码你能数出几个bug?
前言:本文仅仅只是分享笔者一年前见到的诡异代码,大家可以看看乐子,随便数一数一共有多少个bug,这数bug多少还是要点水平的 在初学编程的时候,写的第一个代码大多都是 hello world,可是就算是 hello world…...
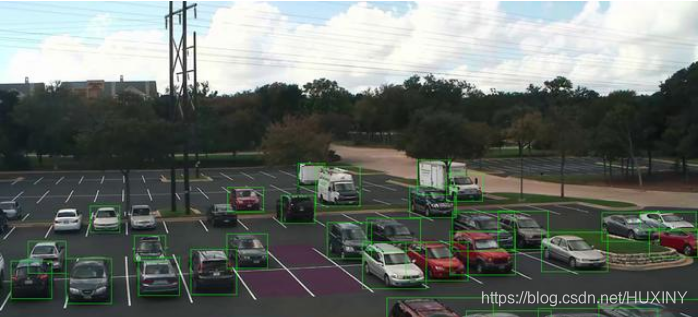
【毕设选题】深度学习 机器视觉 车位识别车道线检测 - python opencv
0 前言 🔥 这两年开始毕业设计和毕业答辩的要求和难度不断提升,传统的毕设题目缺少创新和亮点,往往达不到毕业答辩的要求,这两年不断有学弟学妹告诉学长自己做的项目系统达不到老师的要求。 为了大家能够顺利以及最少的精力通过…...
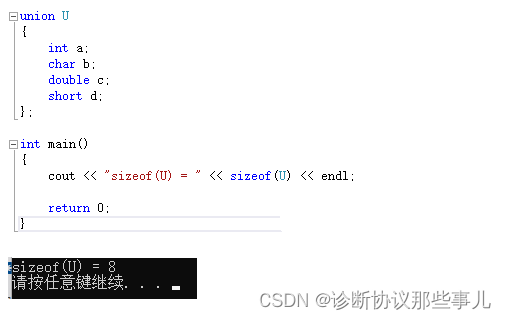
不同数据类型在单片机内存中占多少字节?
文章目录 前言一、不同编译器二、C51* 指针型 三、sizeof结构体联合体 前言 在C语言中,数据类型指的是用于声明不同类型的变量或者函数的一个广泛的系统。变量的类型决定了变量存储占用的空间 一、不同编译器 类型16位编译器大小32位编译器大小64位编译器大小char…...

安卓LinearLayout让控件居中的办法
在控件属性上,是处理不了的。必须是在LinearLayout处理: 垂直居中 <?xml version"1.0" encoding"utf-8"?> <LinearLayout xmlns:android"http://schemas.android.com/apk/res/android"android:layout_width&…...

uniapp下拉刷新
为什么要使用uniapp的下拉刷新呢 跨平台兼容性: Uniapp 允许你一次编写代码,然后在多个平台(如微信小程序、H5、iOS 和 Android 等)上运行。使用 Uniapp 的下拉刷新功能,可以确保在不同平台上都能提供一致的用户体验&a…...

【工作记录】css3 grid布局笔记
概述 Grid 布局即网格布局,是一种新的 CSS 布局模型,比较擅长将一个页面划分为几个主要区域,以及定义这些区域的大小、位置、层次等关系。号称是最强大的的 CSS 布局方案,是目前唯一一种 CSS 二维布局 参数配置说明 属性说明可…...
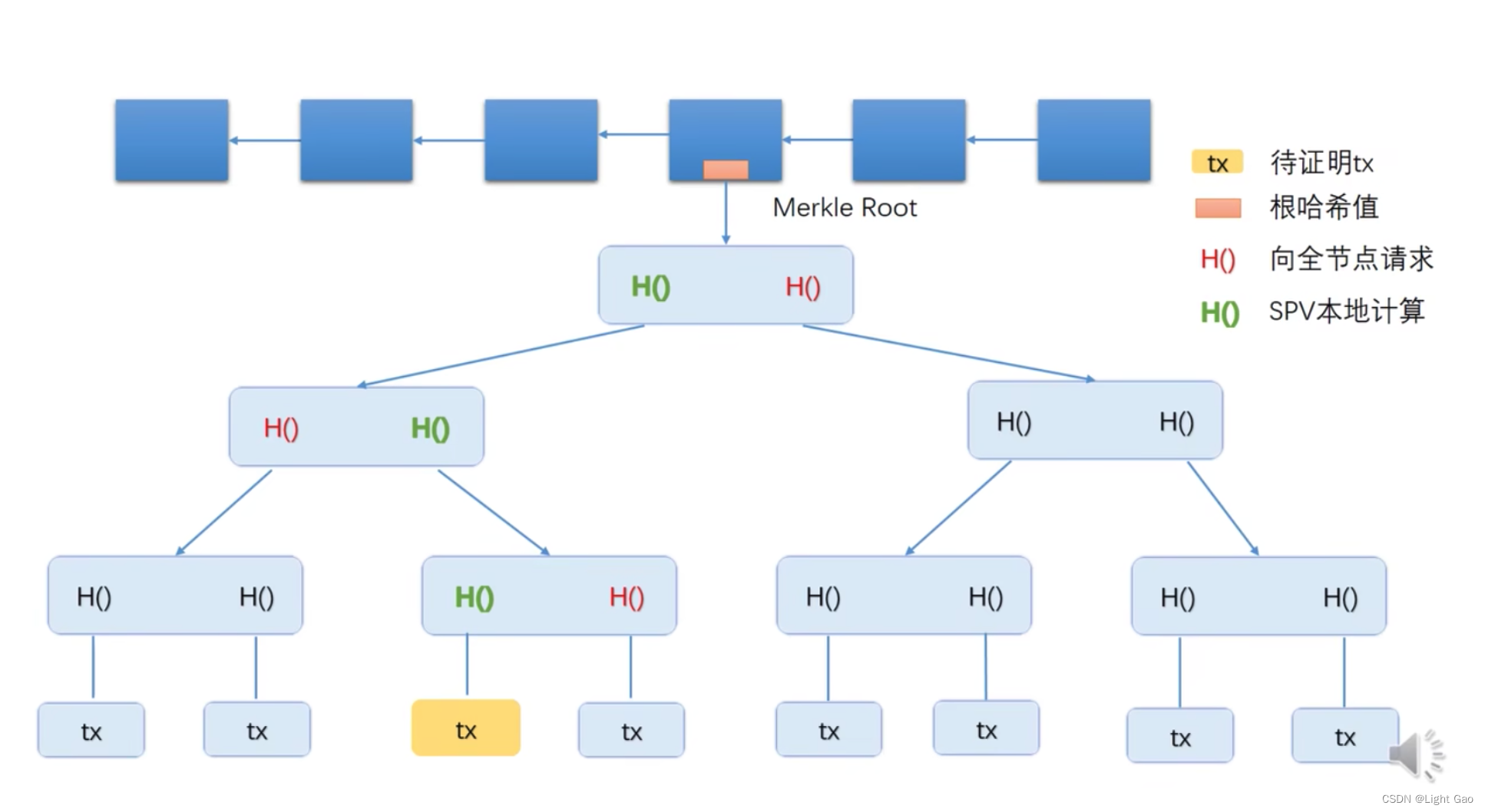
区块链技术-比特币数据结构
背景 随着近几年区块链技术的迅速发展,越来越多的行业正在将区块链技术应用到实际中去。例如,金融、物流、交易所等行业都开始尝试使用区块链技术来替代传统技术。伴随着区块链迅速发展的期间,诞生了比特币(BTC)、以太…...
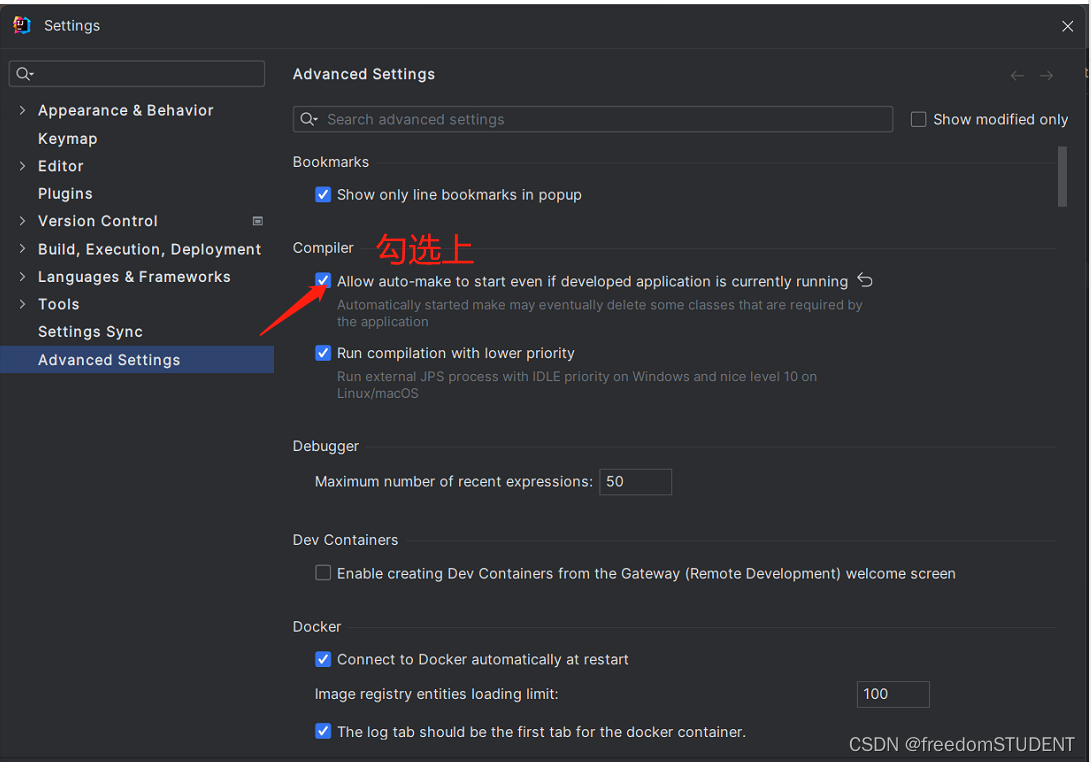
SpringBoot结合dev-tool 实现IDEA项目热部署
什么是热部署? 应用正在运行的时候升级功能, 不需要重新启动应用对于Java应用程序来说, 热部署就是在运行时更新Java类文件 通俗的来讲,应用在运行状态下,修改项目源码后,不用重启应用,会把编译的内容部署到服务器上…...

flink中使用外部定时器实现定时刷新
背景: 我们经常会使用到比如数据库中的配置表信息,而我们不希望每次都去查询db,那么我们就想定时把db配置表的数据定时加载到flink的本地内存中,那么如何实现呢? 外部定时器定时加载实现 1.在open函数中进行定时器的…...
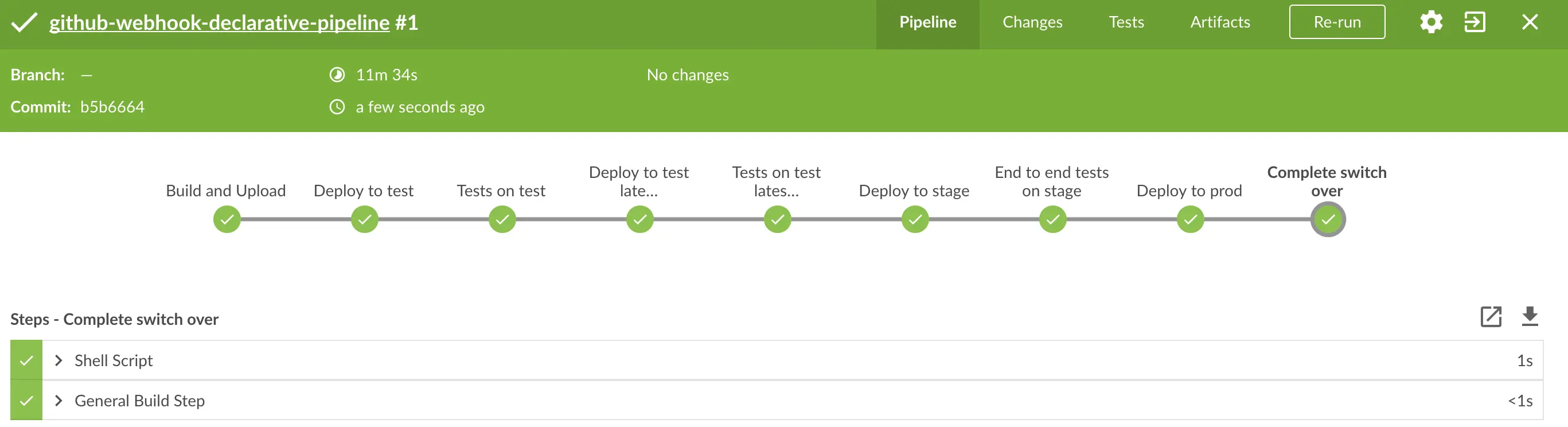
Spring Cloud Pipelines 入门实践
文章目录 1. 前言2. Spring Cloud Pipelines 是做什么的2.1. 预定义的流程2.2. 集成测试和契约测试2.3.部署策略 4. Spring Cloud Pipelines的使用示例4.1. 创建一个Spring Boot应用4.2. 将代码托管到GitHub仓库4.3. 添加Spring Cloud Pipelines依赖4.4. 配置Spring Cloud Pipe…...
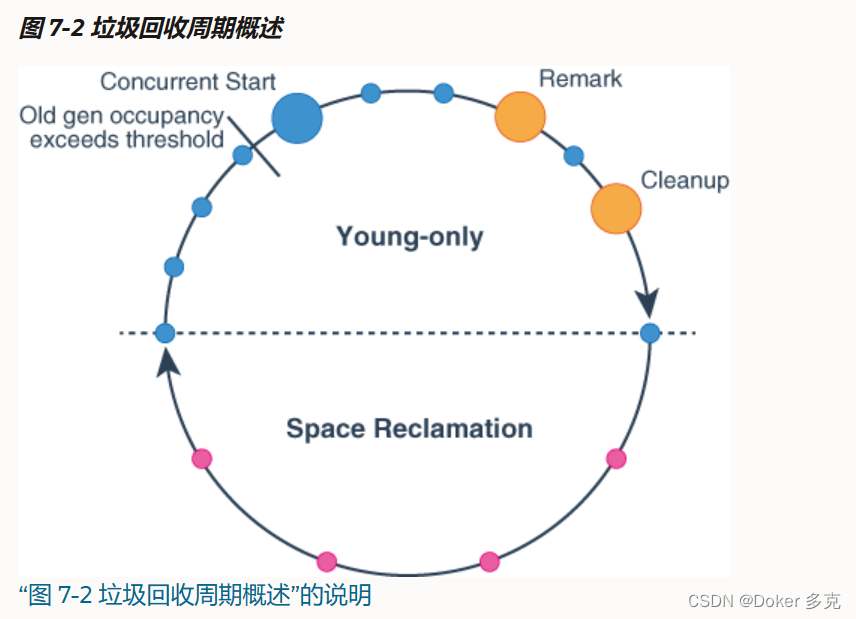
G1 GC详解及设置
一、概述 G1 GC,全称Garbage-First Garbage Collector,在JDK1.7中引入了G1 GC,从JAVA 9开始,G1 GC是默认的GC算法。通过-XX:UseG1GC参数来启用。G1收集器是工作在堆内不同分区上的收集器,分区既可以是年轻代也可以是老…...

GitHub详细教程
将代码推送到GitHub仓库涉及一系列的步骤。以下是详细的步骤说明: 创建一个新的仓库(如果还没有的话): 访问 GitHub。登录您的帐户。点击页面右上角的图标,然后选择“New repository”。填写仓库名称、描述等信息&…...

【小沐学Python】Python实现Web图表功能(Dash)
文章目录 1、简介2、安装3、功能示例3.1 Hello World3.2 连接到数据3.3 可视化数据3.4 控件和回调3.5 设置应用的样式3.5.1 HTML and CSS3.5.2 Dash Design Kit (DDK)3.5.3 Dash Bootstrap Components3.5.4 Dash Mantine Components 4、更多示例4.1 Basic Dashboard4.2 Using C…...
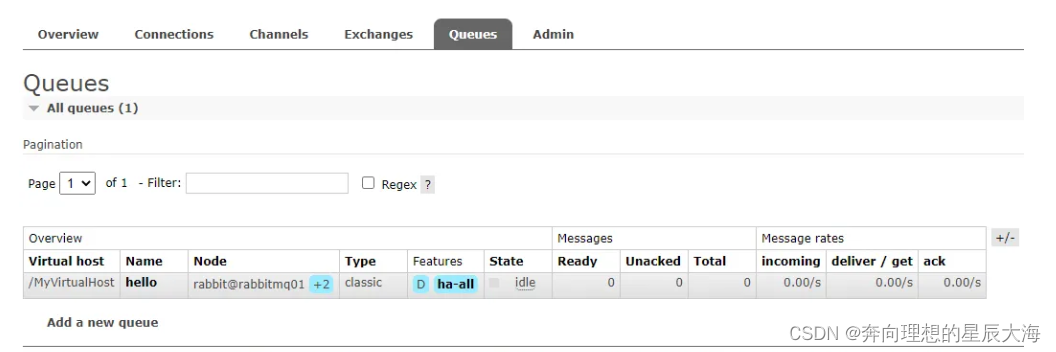
【RabbitMQ】docker rabbitmq集群 docker搭建rabbitmq集群
docker rabbitmq集群 docker搭建rabbitmq集群 RabbitMQ提供了两种常用的集群模式 1.普通集群模式 2.镜像集群模式 普通集群模式只能同步主节点上的交换机和队列信息,但对于队列中的消息不做同步,主节点宕机也不能进行切换(故障转移ÿ…...

Linux 网络驱动实验
本文章对Linux 网络驱动实验中的设备树进行介绍,Linux网络驱动程序比较复杂,只要学会应用。 1、I.MX6ULL 网络外设设备树 I.MX6ULL 有两个 10/100M 的网络 MAC 外设,因此 I.MX6ULL 网络驱动主要就是这两个网络 MAC 外设的驱动。 fec1…...
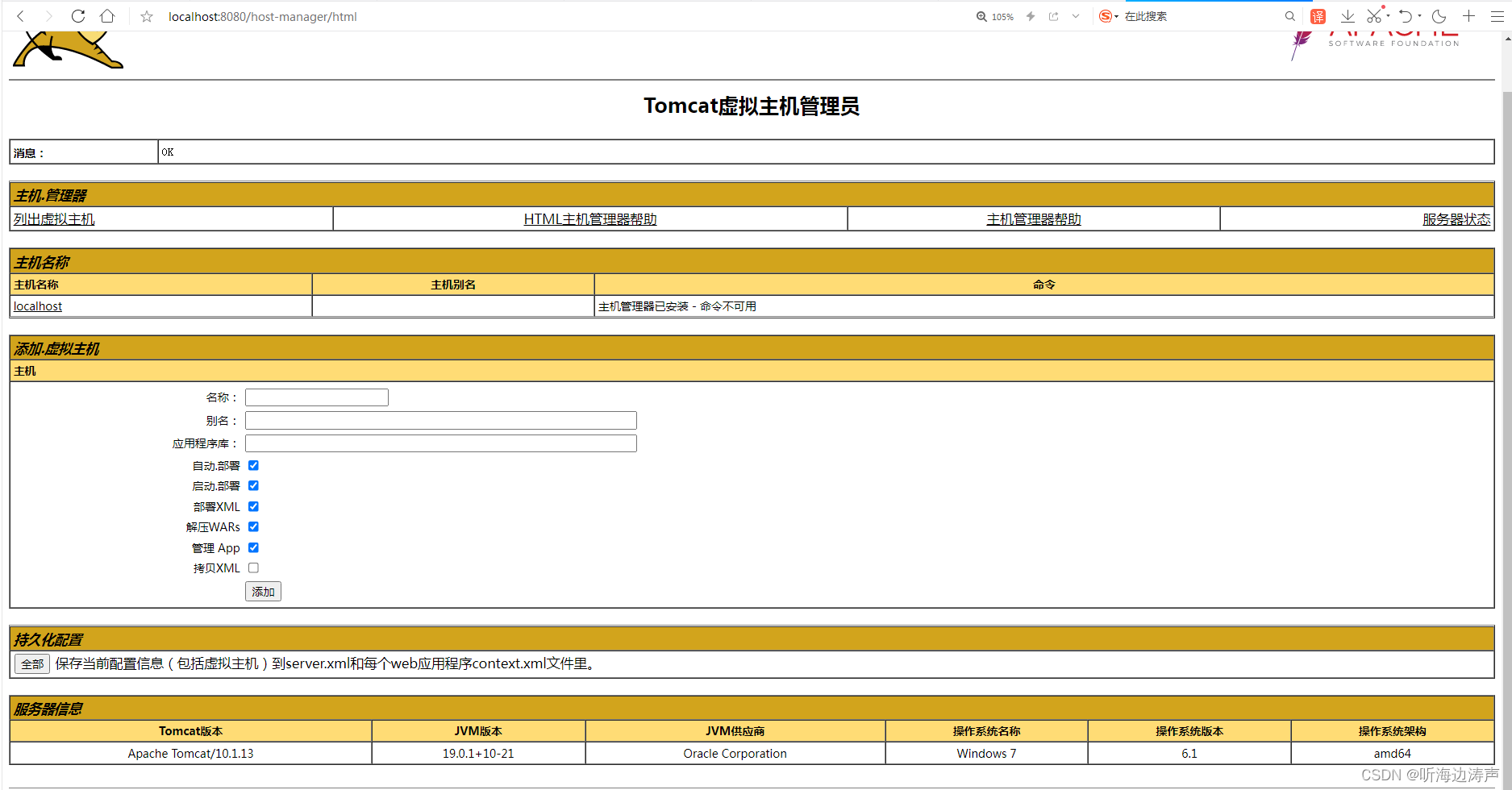
访问Apache Tomcat的虚拟主机管理页面
介绍 通过Tomcat Host Manager应用可以创建、删除、管理Tomcat内的虚拟主机(virtual hosts)。该应用是Tomcat安装的一部分,默认在<Tomcat安装目录>/webapps/host-manager: 配置用户名、密码、角色 要访问Host Manager应…...
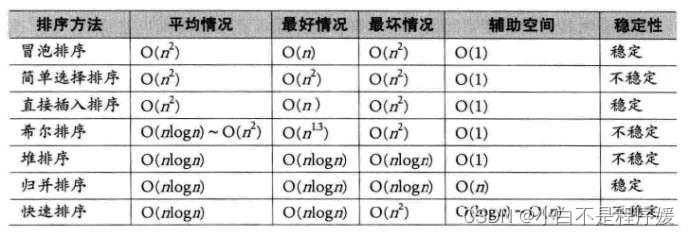
【算法】排序——归并排序和计数排序
主页点击直达:个人主页 我的小仓库:代码仓库 C语言偷着笑:C语言专栏 数据结构挨打小记:初阶数据结构专栏 Linux被操作记:Linux专栏 LeetCode刷题掉发记:LeetCode刷题 算法头疼记:算法专栏…...
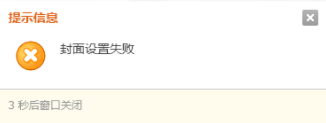
discuz封面设置失败的解决办法(centos系统+windows系统)
discuz封面设置失败的解决办法(centos系统windows系统) centos系统:1、开启/var/www/html 这个目录的读写权限chmod -R 777 /var/www/html然后重启httpd:service httpd restart如果discuz论坛发布帖子,还是显示封面设置失败的话…...
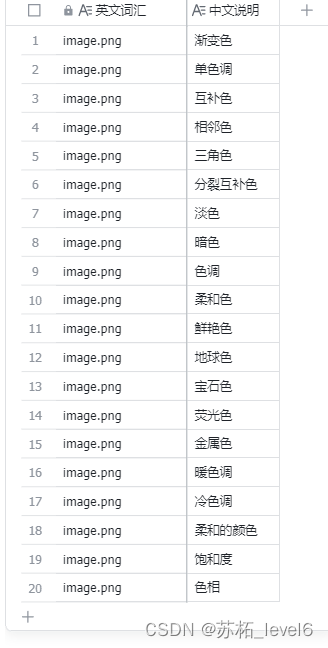
AI绘画-Stable Diffusion笔记
软件:Stable Diffusion 视频教程来自 https://www.bilibili.com/video/BV1As4y127HW/?spm_id_from333.337.search-card.all.click 提示词 提示词类别 内容型提示词 人物主题特征: 服饰穿搭:white dress 发型发色:blonde hair,l…...

中值滤波算法及例程
中值滤波是一种常用的非线性图像滤波算法,它能够有效去除图像中的椒盐噪声(即孤立的亮或暗像素点),同时保持图像边缘和细节的清晰度。中值滤波的主要思想是使用一个滑动窗口,在窗口内对像素值进行排序,并将…...
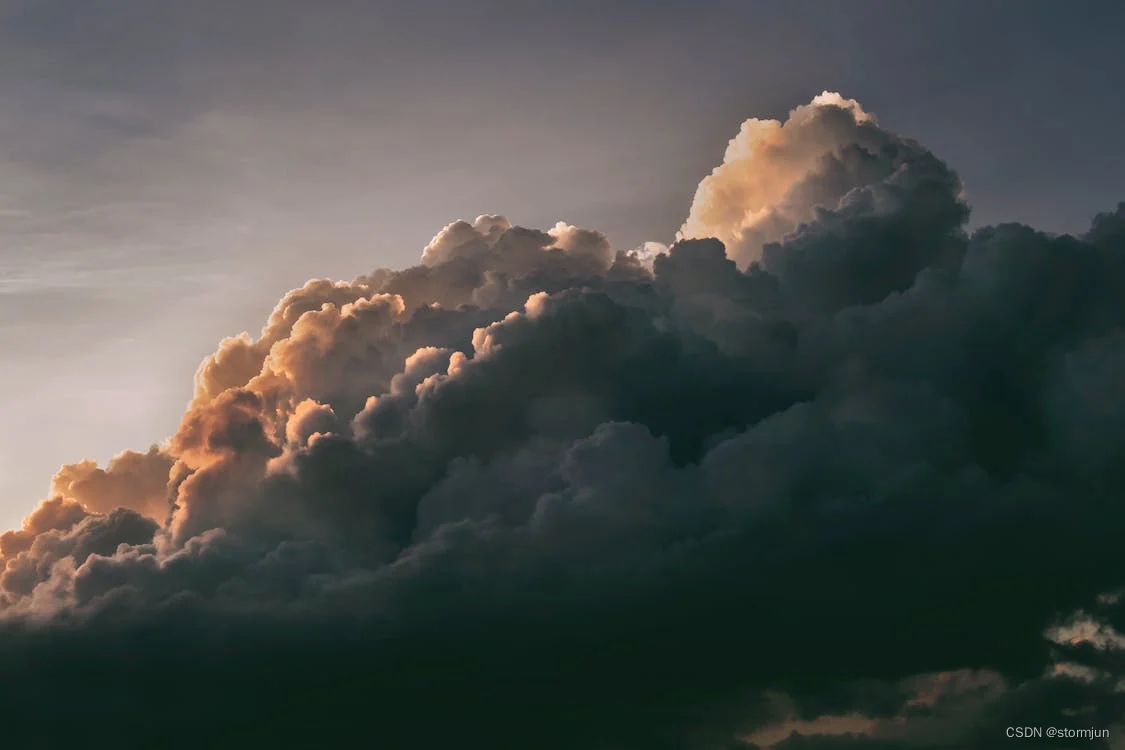
SpringBoot 如何使用 Ehcache 作为缓存
使用Spring Boot Sleuth进行分布式跟踪 在现代分布式应用程序中,跟踪请求和了解应用程序的性能是至关重要的。Spring Boot Sleuth是一个分布式跟踪解决方案,它可以帮助您在分布式系统中跟踪请求并分析性能问题。本文将介绍如何在Spring Boot应用程序中使…...
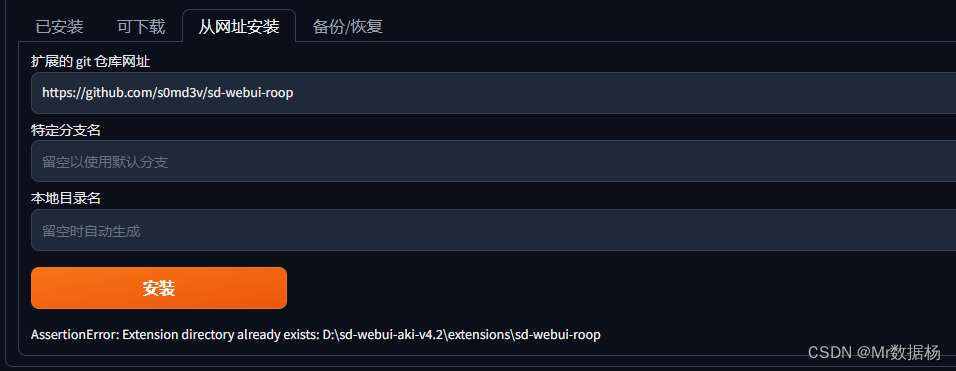
Stable Diffusion 图片换脸插件Roop保姆教程 附错误解决办法和API使用
换脸技术已经不是新鲜事物,但如何实现简单、快速、高效的换脸操作呢?Roop插件正是为解决这一问题而生的。 sd-webui-roop 插件适用于已经本地部署了SD的用户。相较于传统的换脸技术,Roop插件几乎不需要训练,只需一张照片,即可在10秒内完成换脸。 但是要注意到是必须注意…...

华为OD机试 - 组成最大数(Java 2023 B卷 100分)
目录 专栏导读一、题目描述二、输入描述三、输出描述四、解题思路五、Java算法源码六、效果展示1、输入2、输出 华为OD机试 2023B卷题库疯狂收录中,刷题点这里 专栏导读 本专栏收录于《华为OD机试(JAVA)真题(A卷B卷)》…...