【入门Flink】- 11Flink实现动态TopN
基本处理函数(ProcessFunction)
stream.process(new MyProcessFunction())
方法需要传入一个 ProcessFunction
作为参数,ProcessFunction 不是接口 , 而是一个抽象类 ,继承了AbstractRichFunction,所有的处理函数,都是富函数(RichFunction),拥有富函数所有功能。
// 泛型:
// Type parameters:<I> – Type of the input elements. 输入类型
// <O> – Type of the output elements. 输出类型
public abstract class ProcessFunction<I, O> extends AbstractRichFunction {public abstract void processElement(I value, Context ctx, Collector<O> out) throws Exception;public void onTimer(long timestamp, OnTimerContext ctx, Collector<O> out) throws Exception {}
}
1)抽象方法.processElement()
“处理元素”,定义了处理的核心逻辑。这个方法对于流中的每个元素都会调用一次
,参数包括三个:输入数据值 value,上下文 ctx,以及“收集器”(Collector)out。
value
:当前流中的输入元素ctx
:类型是 ProcessFunction 中定义的内部抽象类 Context,表示当前运行的上下文,可以获取到当前的时间戳
,并提供了用于查询时间和注册定时器的“定时服务”(TimerService)
,以及可以将数据发送到“侧输出流”(side output)
的方法.output()。out
:“收集器”(类型为 Collector),用于返回输出数据。调用 out.collect()方法就可以向下游发出一个数据。这个方法可以多次调用,也可以不调用
。
ProcessFunction 可以轻松实现flatMap、map、filter 这样的基本转换功能;而通过富函数提供的获取上下文方法.getRuntimeContext(),也可以自定义状态(state)进行处理。
2)非抽象方法.onTimer()
只有在注册好的定时器触发的时候才会调用
,而定时器是通过“定时服务”TimerService 来注册的。
三个参数:时间戳(timestamp),上下文(ctx),以及收集器(out)。
-
timestamp
:指设定好的触发时间
,事件时间语义下是水位线 -
ctx:同样可以调用定时服务(TimerService)
-
采集器:任意输出处理之后的数据
.onTimer()方法定时触发,因此ProcessFunction可以自定义数据按照时间分组 、 定时触发计算输出结果;这 就实现了**窗口(window )**的功能。所以说
ProcessFunction 可以实现一切功能
。
注意
:在 Flink 中,只有**“按键分区流”KeyedStream 才支持设置定时器的操作**。
处理函数的分类(8大处理函数)
1)ProcessFunction
最基本的处理函数,基于 DataStream 直接调用.process()时作为参数传入。
2)KeyedProcessFunction
对流按键分区
后的处理函数,基于 KeyedStream 调用.process()时作为参数传入。要想使用定时器,必须基于 KeyedStream
。
3)ProcessWindowFunction
开窗之后
的处理函数,也是全窗口函数的代表。基于 WindowedStream调用.process()时作为参数传入。
4)ProcessAllWindowFunction
同样是开窗之后的处理函数,基于 AllWindowedStream 调用.process()时作为参数传入
5)CoProcessFunction
合并(connect)两条流
之后的处理函数,基于 ConnectedStreams 调用.process()时作为参数传入
6)ProcessJoinFunction
间隔连接(interval join)两条流
之后的处理函数,基于 IntervalJoined 调用.process()时作为参数传入。
7)BroadcastProcessFunction
广播连接流
处理函数,基于 BroadcastConnectedStream 调用.process()时作为参数传入。
“广播连接流”BroadcastConnectedStream,是一个未 keyBy 的普通DataStream与一个广播流(BroadcastStream)做连接(conncet)
之后的产物。
8)KeyedBroadcastProcessFunction
按键分区的广播
连接流处理函数,同样是基于 BroadcastConnectedStream调用.process()时作为参数传 入 。 一个KeyedStream 与广播流(BroadcastStream)做连接
之后的产物。
按键分区处理函数(KeyedProcessFunction)
定时器(Timer)和定时服务(TimerService)
ProcessFunction 的上下文(Context)中提供了.timerService()方法
,可以直接返回一个 TimerService 对象。
TimerService包含以下六个方法:
// 获取当前的处理时间
long currentProcessingTime();
// 获取当前的水位线(事件时间)
long currentWatermark();
// 注册处理时间定时器,当处理时间超过 time 时触发
void registerProcessingTimeTimer(long time);
// 注册事件时间定时器,当水位线超过 time 时触发
void registerEventTimeTimer(long time);
// 删除触发时间为 time 的处理时间定时器
void deleteProcessingTimeTimer(long time);
// 删除触发时间为 time 的处理时间定时器
void deleteEventTimeTimer(long time);
六个方法可以分成两大类:基于处理时间和基于事件时间
。
TimerService 会以键(key)和时间戳为标准,对定时器进行去重;
每个key和时间戳,最多只有一个定时器,如果注册了多次,onTimer()方法也将只被调用一次
。
案例
public class KeyedProcessTimerDemo {public static void main(String[] args) throws Exception {StreamExecutionEnvironment env = StreamExecutionEnvironment.getExecutionEnvironment();env.setParallelism(1);SingleOutputStreamOperator<WaterSensor> sensorDS = env.socketTextStream("124.222.253.33", 7777).map(new WaterSensorMapFunction()).assignTimestampsAndWatermarks(WatermarkStrategy.<WaterSensor>forBoundedOutOfOrderness(Duration.ofSeconds(3)).withTimestampAssigner((element, ts) -> element.getTs() * 1000L));// 传感器Id keyByKeyedStream<WaterSensor, String> sensorKS = sensorDS.keyBy(WaterSensor::getId);sensorKS.process(new KeyedProcessFunction<String, WaterSensor, String>() {/*** 来一条数据调用一次*/@Overridepublic void processElement(WaterSensor value, KeyedProcessFunction<String, WaterSensor, String>.Context ctx, Collector<String> out) throws Exception {// 获取当前数据的 keyString currentKey = ctx.getCurrentKey();// TODO 1.定时器注册TimerService timerService = ctx.timerService();// 1、事件时间的案例Long currentEventTime = ctx.timestamp();//数据中提取出来的事件时间timerService.registerEventTimeTimer(5000L);System.out.println(" 当前key=" + currentKey + ",当前时间=" + currentEventTime + ",注册了一个5s 的定时器");// 2、处理时间的案例// long currentTs = timerService.currentProcessingTime();// timerService.registerProcessingTimeTimer(currentTs + 5000L);// System.out.println(" 当前key="+currentKey + ",当前时间=" + currentTs + ",注册了一个5s 后的定时器");// 3、获取 process 的 当前watermark// long currentWatermark = timerService.currentWatermark();// System.out.println("当前数据=" +value+",当前 watermark=" + currentWatermark);// 注册定时器: 处理时间、事件时间// timerService.registerProcessingTimeTimer();// timerService.registerEventTimeTimer();// 删除定时器: 处理时间、事件时间// timerService.deleteEventTimeTimer();// timerService.deleteProcessingTimeTimer();// 获取当前时间进展: 处理时间-当前系统时间,事件时间-当前 watermark// long currentTs = timerService.currentProcessingTime();}/*** .时间进展到定时器注册的时间,调用该方法* @param timestamp 当前时间进展,就是定时器被触发时的时间*/@Overridepublic void onTimer(long timestamp, KeyedProcessFunction<String, WaterSensor, String>.OnTimerContext ctx, Collector<String> out) throws Exception {super.onTimer(timestamp, ctx, out);String currentKey = ctx.getCurrentKey();System.out.println("key=" + currentKey + "现在时间是" + timestamp + "定时器触发");}}).print();env.execute();}
}
测试结果:
注册多个定时器,但是时间到了只触发一次。
窗口处理函数
ProcessWindowFunction 和 ProcessAllWindowFunction
(ProcessAllWindowFunction,没有 keyBy 的数据流直接开窗并调用.process()方法)
stream.keyBy( t -> t.f0 )
.window( TumblingEventTimeWindows.of(Time.seconds(10))).process(new MyProcessWindowFunction())
/* 泛型* Type parameters:* <IN> – The type of the input value. 输入类型* <OUT> – The type of the output value. 输出类型* <KEY> – The type of the key. key类型* <W> – The type of Window that this window function can be applied on. 窗口类型*/
public abstract class ProcessWindowFunction<IN, OUT, KEY, W extends Window>extends AbstractRichFunction {public abstract void process(KEY key, Context context, Iterable<IN> elements, Collector<OUT> out) throws Exception;public void clear(Context context) throws Exception {}
}
抽象方法process
- key:窗口做统计计算基于的键,也就是之前 keyBy 用来分区的字段。
- context:当前窗口进行计算的上下文,它的类型就是ProcessWindowFunction内部定义的抽象类 Context。
- elements:窗口收集到用来计算的所有数据,这是一个可迭代的集合类型。
- out:收集器
上下文调用函数:
public abstract class Context implements java.io.Serializable {public abstract W window();public abstract long currentProcessingTime();public abstract long currentWatermark();// 窗口状态public abstract KeyedStateStore windowState();// 全局状态public abstract KeyedStateStore globalState();// 定义侧输出流public abstract <X> void output(OutputTag<X> outputTag, X value);}
TopN
需求:实时统计一段时间内的出现次数最多的水位。例如,统计最近10 秒钟内出现次数最多的两个水位,并且每 5 秒钟更新一次。
创建实体类:
public class WaterSensor {/*** 传感器Id*/public String id;/*** 时间戳*/public Long ts;/*** 水位*/public Integer vc;
}
方法一:使用 ProcessAllWindowFunction
public class ProcessAllWindowTopNDemo {public static void main(String[] args) {StreamExecutionEnvironment env = StreamExecutionEnvironment.getExecutionEnvironment();env.setParallelism(1);SingleOutputStreamOperator<WaterSensor> sensorDS = env.socketTextStream("124.222.253.33", 7777).map(new WaterSensorMapFunction()).assignTimestampsAndWatermarks(WatermarkStrategy.<WaterSensor>forBoundedOutOfOrderness(Duration.ofSeconds(3)).withTimestampAssigner((element, ts) -> element.getTs() * 1000L));// 滑动窗口sensorDS.windowAll(SlidingEventTimeWindows.of(Time.seconds(10), Time.seconds(5))).process(new MyTopNPAWF()).print();}
}// 抽取窗口函数
public class MyTopNPAWF extends ProcessAllWindowFunction<WaterSensor, String, TimeWindow> {@Overridepublic void process(ProcessAllWindowFunction<WaterSensor, String, TimeWindow>.Context context, Iterable<WaterSensor> elements, Collector<String> out) throws Exception {Map<Integer, Integer> vcCountMap = new HashMap<>();for (WaterSensor element : elements) {// 统计不同水位出现次数vcCountMap.put(element.getVc(), vcCountMap.getOrDefault(element.getVc(), 0) + 1);}// 对 count 值进行排序: 利用 List 来实现排序List<Tuple2<Integer, Integer>> datas = new ArrayList<>();for (Integer vc : vcCountMap.keySet()) {datas.add(Tuple2.of(vc, vcCountMap.get(vc)));}// 对 List 进行排序,根据 count 值 降序datas.sort(new Comparator<Tuple2<Integer, Integer>>() {@Overridepublic int compare(Tuple2<Integer, Integer> o1, Tuple2<Integer, Integer> o2) {// 降序, 后 减 前return o2.f1 - o1.f1;}});StringBuilder outStr = new StringBuilder();outStr.append("================================\n");// 遍历 排序后的 List,取出前 2 个, 考虑可能List 不够2个的情况==》 List 中元素的个数 和 2 取最小值for (int i = 0; i < Math.min(2, datas.size()); i++) {Tuple2<Integer, Integer> vcCount = datas.get(i);outStr.append("Top").append(i + 1).append("\n");outStr.append("vc=").append(vcCount.f0).append("\n");outStr.append("count=").append(vcCount.f1).append("\n");outStr.append(" 窗 口 结束时间=").append(DateFormatUtils.format(context.window().getEnd(), "yyyy-MM-ddHH:mm:ss.SSS")).append("\n");outStr.append("================================\n");}out.collect(outStr.toString());}
}
无论并行度如何设置,并行度只为1。效率不高
方法二:使用 KeyedProcessFunction ☆
从两个方面去做优化:一是对数据进行按键分区
,分别统计vc 的出现次数;二是进行增量聚合
,得到结果最后再做排序输出。
public class KeyedProcessFunctionTopNDemo {public static void main(String[] args) throws Exception {StreamExecutionEnvironment env = StreamExecutionEnvironment.getExecutionEnvironment();env.setParallelism(1);SingleOutputStreamOperator<WaterSensor> sensorDS = env.socketTextStream("124.222.253.33", 7777).map(new WaterSensorMapFunction()).assignTimestampsAndWatermarks(WatermarkStrategy.<WaterSensor>forBoundedOutOfOrderness(Duration.ofSeconds(3)).withTimestampAssigner((element, ts) -> element.getTs() * 1000L));// 【水位分组】KeyedStream<WaterSensor, Integer> keyedStream = sensorDS.keyBy(WaterSensor::getVc);/*思路二: 使用 KeyedProcessFunction 实现1、按照 vc 做 keyby,开窗,分别 count==》 增量聚合,计算 count==》 全窗口,对计算结果 count 值封装,带上窗口结束时间的标签==》 为了让同一个窗口时间范围的计算结果到一起去2、对同一个窗口范围的 count 值进行处理:排序、取前N 个=》 按照 windowEnd 做 keyby=》 使用 process, 来一条调用一次,需要先存,分开存,用HashMap,key=windowEnd,value=List=》 使用定时器,对 存起来的结果 进行排序、取前N个*/// 1. 按照 vc 分组、开窗、聚合(增量计算+全量打标签)// 开窗聚合后,就是普通的流,没有了窗口信息,需要自己打上窗口的标记windowEndSingleOutputStreamOperator<Tuple3<Integer, Integer, Long>> windowAgg = keyedStream.window(SlidingEventTimeWindows.of(Time.seconds(10), Time.seconds(5))).aggregate(new VcCountAgg(),new WindowResult());// 2. 按照窗口标签(窗口结束时间)keyby,保证同一个窗口时间范围的结果,到一起去。排序、取 TopNwindowAgg.keyBy(r -> r.f2).process(new TopN(2)).print();env.execute();}// 【同水位累加】public static class VcCountAgg implements AggregateFunction<WaterSensor, Integer, Integer>{@Overridepublic Integer createAccumulator() {return 0;}@Overridepublic Integer add(WaterSensor value, Integer accumulator) {return accumulator + 1;}@Overridepublic Integer getResult(Integer accumulator) {return accumulator;}@Overridepublic Integer merge(Integer a, Integer b) {return null;}}/*** 【打时间标签】* 泛型如下:* 第一个:输入类型 = 增量函数的输出 count 值,Integer* 第二个:输出类型 = Tuple3(vc,count,windowEnd) ,带上窗口结束时间的标签* 第三个:key 类型 , vc,Integer* 第四个:窗口类型*/public static class WindowResult extends ProcessWindowFunction<Integer, Tuple3<Integer, Integer, Long>, Integer, TimeWindow> {@Overridepublic void process(Integer key, Context context, Iterable<Integer> elements, Collector<Tuple3<Integer, Integer, Long>> out) throws Exception {// 迭代器里面只有一条数据,next 一次即可Integer count = elements.iterator().next();long windowEnd = context.window().getEnd();out.collect(Tuple3.of(key, count, windowEnd));}}public static class TopN extends KeyedProcessFunction<Long, Tuple3<Integer, Integer, Long>, String> {// 存不同窗口的 统计结果,key=windowEnd,value=list 数据private Map<Long, List<Tuple3<Integer, Integer, Long>>> dataListMap;// 要取的 Top 数量private int threshold;public TopN(int threshold) {this.threshold = threshold;dataListMap = new HashMap<>();}@Overridepublic void processElement(Tuple3<Integer, Integer, Long> value, Context ctx, Collector<String> out) throws Exception {// 进入这个方法,只是一条数据,要排序,得到齐才行===》存起来,不同窗口分开存// 1. 存到 HashMap 中Long windowEnd = value.f2;if (dataListMap.containsKey(windowEnd)) {// 1.1 包含 vc,不是该 vc 的第一条,直接添加到List中List<Tuple3<Integer, Integer, Long>> dataList = dataListMap.get(windowEnd);dataList.add(value);} else {// 1.1 不包含 vc,是该 vc 的第一条,需要初始化listList<Tuple3<Integer, Integer, Long>> dataList = new ArrayList<>();dataList.add(value);dataListMap.put(windowEnd, dataList);}// 2. 注册一个定时器, windowEnd+1ms 即可 延迟1ms 触发即可,及时性ctx.timerService().registerEventTimeTimer(windowEnd + 1);}@Overridepublic void onTimer(long timestamp, OnTimerContext ctx, Collector<String> out) throws Exception {super.onTimer(timestamp, ctx, out);// 定时器触发,同一个窗口范围的计算结果攒齐了,开始排序、取TopNLong windowEnd = ctx.getCurrentKey();// 1. 排序List<Tuple3<Integer, Integer, Long>> dataList = dataListMap.get(windowEnd);dataList.sort(new Comparator<Tuple3<Integer, Integer, Long>>() {@Overridepublic int compare(Tuple3<Integer, Integer, Long> o1, Tuple3<Integer, Integer, Long> o2) {return o2.f1 - o1.f1;}});// 2. 取 TopNStringBuilder outStr = new StringBuilder();outStr.append("================================\n");for (int i = 0; i < Math.min(threshold, dataList.size()); i++) {Tuple3<Integer, Integer, Long> vcCount = dataList.get(i);outStr.append("Top").append(i + 1).append("\n");outStr.append("vc=").append(vcCount.f0).append("\n");outStr.append("count=").append(vcCount.f1).append("\n");outStr.append("窗口结束时间=").append(vcCount.f2).append("\n");outStr.append("================================\n");}// 用完的 List,及时清理,节省资源dataList.clear();out.collect(outStr.toString());}}
}
增量聚合、开窗处理
- 水位线分组
- 增量聚合,相同水位线数量+1
- 窗口函数打时间标签
- 按上述打的时间标签分组,排序获取topN(process)
侧输出流
process函数带侧输出流
案例:对每个传感器,水位超过 10 的输出告警信息
public class SideOutputDemo {public static void main(String[] args) throws Exception {StreamExecutionEnvironment env = StreamExecutionEnvironment.getExecutionEnvironment();env.setParallelism(1);SingleOutputStreamOperator<WaterSensor> sensorDS = env.socketTextStream("124.222.253.33", 7777).map(new WaterSensorMapFunction());OutputTag<String> warnTag = new OutputTag<>("warn", Types.STRING);// 传感器分组SingleOutputStreamOperator<WaterSensor> process = sensorDS.keyBy(WaterSensor::getId).process(new KeyedProcessFunction<String, WaterSensor, WaterSensor>() {@Overridepublic void processElement(WaterSensor value, Context ctx, Collector<WaterSensor> out) throws Exception {// 使用侧输出流告警String currentKey = ctx.getCurrentKey();if (value.getVc() > 10) {ctx.output(warnTag, "当前传感器=" + currentKey + ",当前水位=" + value.getVc() + ",大于阈值 10!!!");}// 主流正常 发送数据out.collect(value);}});process.print("主流");process.getSideOutput(warnTag).printToErr("warn");env.execute();}
}
测流输出的同时不影响主流
相关文章:
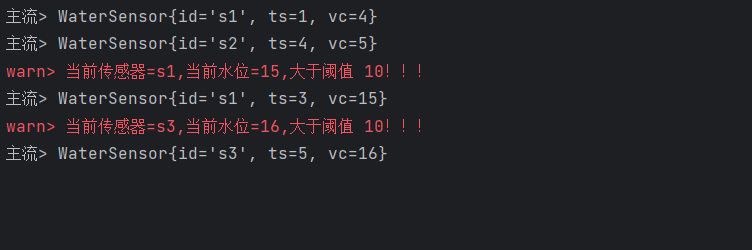
【入门Flink】- 11Flink实现动态TopN
基本处理函数(ProcessFunction) stream.process(new MyProcessFunction())方法需要传入一个 ProcessFunction 作为参数,ProcessFunction 不是接口 , 而是一个抽象类 ,继承了AbstractRichFunction,所有的处…...
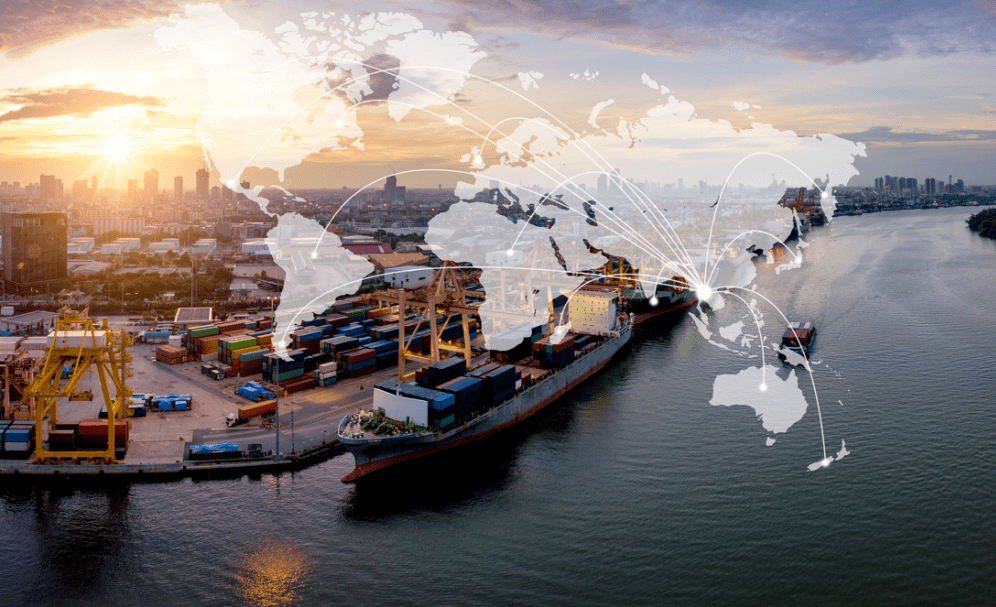
现在做跨境电商还需要全球代理IP吗?全球代理IP哪家靠谱?
随着全球互联网的发展,电商平台的发展和跨境贸易的便利化,跨境电商在过去几年中也一直呈现增长趋势,吸引了越来越多的企业和个体创业者入行。 然而,行业竞争也在不断加剧,跨境电商面临更多的越来越多的挑战࿰…...

微服务的常用组件
1、注册中心组件 Eureka、Nacos、Zookeeper、Consul 2、负载均衡组件 Ribbon 3、远程调用组件 OpenFeign 4、网关组件 Zuul、Gateway 5、服务保护组件 Hystrix、Sentinel 6、服务配置管理组件 SpringCloudConfig、Nacos、Apollo(阿波罗)配置中…...
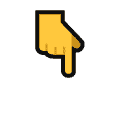
Android问题笔记四十五:解决SeekBar操作thumb周围产生的圆形阴影/灰色阴影/白色圆圈的解决办法
点击跳转专栏>Unity3D特效百例点击跳转专栏>案例项目实战源码点击跳转专栏>游戏脚本-辅助自动化点击跳转专栏>Android控件全解手册点击跳转专栏>Scratch编程案例点击跳转>软考全系列点击跳转>蓝桥系列点击跳转>ChatGPT和AIGC 👉关于作者 专…...

AI从入门到精通,什么是LLMs大型语言模型?
大型语言模型是指由大量文本数据训练而成的自然语言处理模型,它可以生成高质量的文本内容,并能够理解和回答人类提出的问题。 这种模型通常基于深度学习技术,如循环神经网络(RNN)或变换器(Transformer&…...

优测云测试平台 | 有效的单元测试(下)
接着上一篇内容,我们继续~ 四、测试的目标之三:快速反馈 测试的快速反馈有两个方面的含义: 1.测试运行要快速出结果。 2.当测试失败时,要能快速定位失败原因。 测试运行效率决定了开发的工作周期运转的快慢。在理想的 TDD 模型中&#x…...
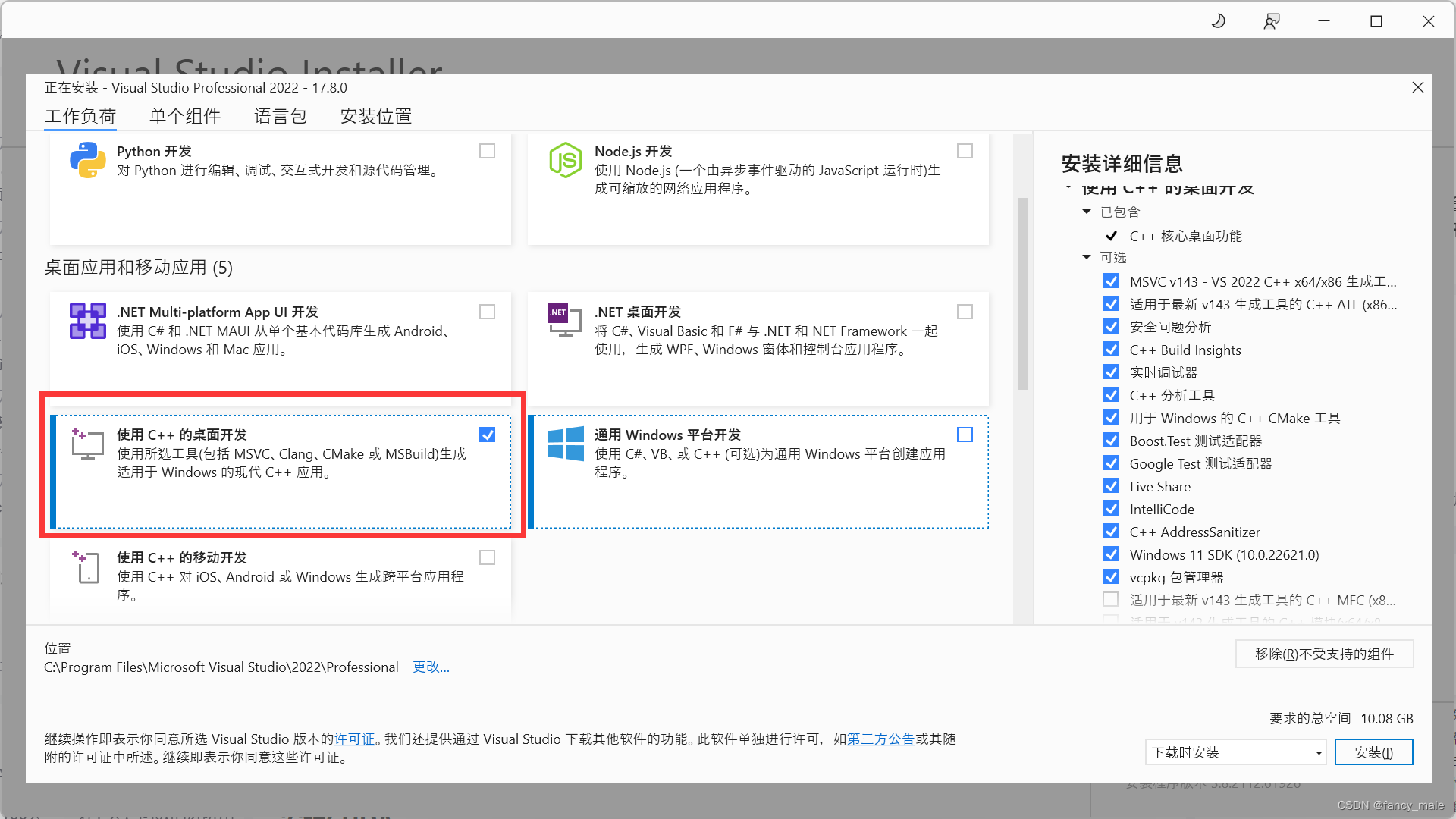
CUDA安装
在cmd中输入nvidia-smi。显示CUDA Version:12.3,所以只能下载小于等于12.3的版本。如下图: 进这个网址:https://developer.nvidia.com/cuda-toolkit-archive 选择一个版本下载。 选择完后之后这样选择: 最后点击下载即…...

【XTDrone Ubuntu18.04】XTDrone + Ubuntu18.04 + PX4 安装过程
重新配置所有的软件 卸载之前安装的ROS GAZEBO 记得把/home下的.ros和.gazebo也删除,删就删干净 参考链接:ROS的卸载与安装 血泪总结!亲测有效 卸载ROS方法 正式安装 安装依赖 sudo apt install ninja-build exiftool ninja-build protobuf…...
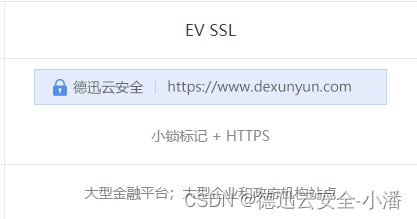
网站使用什么协议比较好
网站协议大多数使用HTTP和HTTPS HTTP协议,超文本传输协议(Hypertext Transfer Protocol,HTTP)是一个简单的请求-响应协议。 HTTP是应用层协议,同其他应用层协议一样,是为了实现某一类具体应用的协议&…...

18. 机器学习——集成学习
机器学习面试题汇总与解析——集成学习 本章讲解知识点 什么是集成学习AdaBoost梯度提升树(Gradient Boosting Decision Tree, GBDT)随机森林(Random Forest,简称RF)XGBoostLightGBM本专栏适合于Python已经入门的学生或人士,有一定的编程基础。 本专栏适合于算法工程师、机器…...
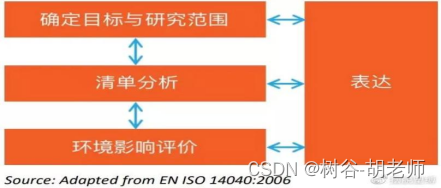
SimaPro生命周期评估建模与碳足迹分析流程
SimaPro以系统和透明的方式轻松建模和分析复杂的生命周期,通过确定供应链中每个环节的热点,从原材料的提取到制造,分销,使用和处置,衡量所有生命周期阶段的产品和服务对环境的影响。SimaPro是过去25年评估生命周期的最…...

我的项目分享(不喜勿喷)
我要分享的项目是大喇叭C2C电商平台系统,一个面向移动端的电子商务平台,为个体消费者和商家提供直接交易和沟通的便利,丰富了人们的生活。 主要功能模块: 该项目的主要功能包括: 1. 用户注册功能:使用正则…...
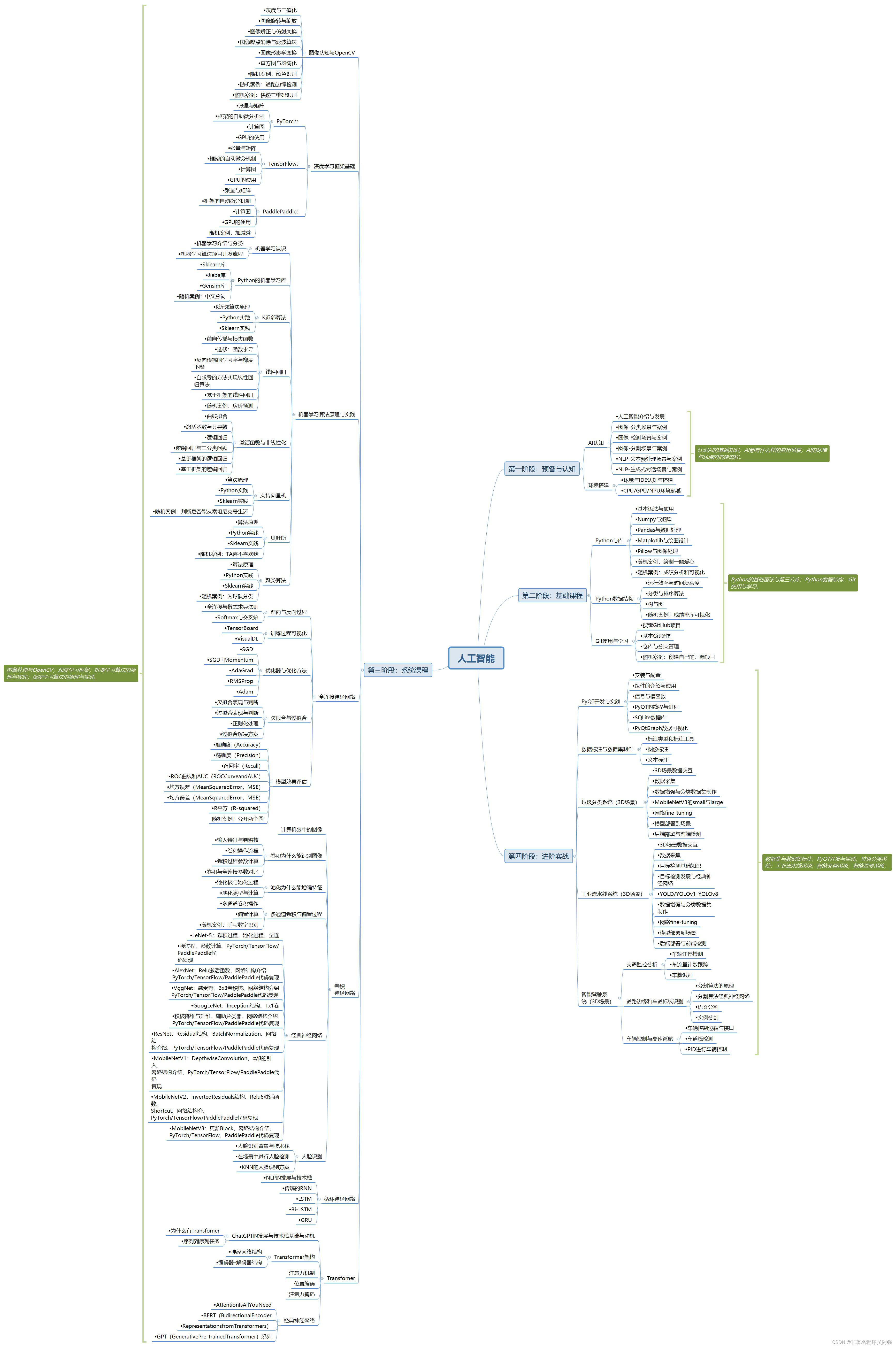
PyTorch:张量与矩阵
PyTorch 是一个基于 Python 的科学计算包,专门针对深度学习研究,提供了丰富的工具和库。在 PyTorch 中,张量(tensor)是深度学习的核心数据结构,它可以看作是可以进行自动微分的多维数组。张量不仅可以代表标…...
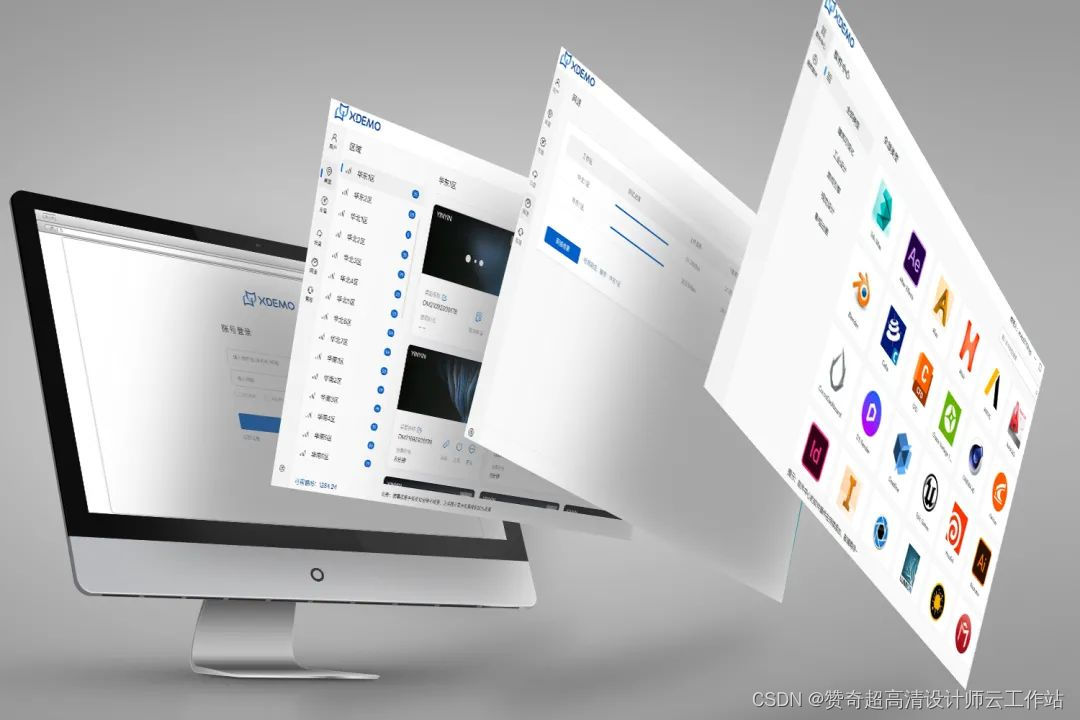
传统广电媒体为何选择上云?有何优势?
随着现在互联网和科技的发展,现在更多的行业都搭上了科技这辆快车快速的完成了转型,那么在传统的广电媒资行业他们目前有哪些痛点呢?传统广电媒体转型发展现状是什么?企业如何数字化转型?企业上云的优势有哪些…...
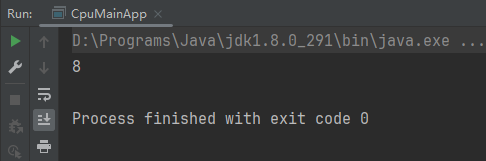
系列十、堆参数调优
一、堆内存调优参数 -Xms堆空间的最小值,默认为物理内存的1/64-Xmx堆空间的最大值,默认为物理内存的1/4-XX:PrintGCDetails输出详细的GC处理日志 二、获取堆内存的默认物理内存 /*** Author : 一叶浮萍归大海* Date: 2023/11/16 14:50* Description: 获…...

sqlite3简单使用
为什么要使用sqlite3? sqlite3轻量简介,无需单独的数据库服务,只需访问磁盘上的.db的文件。在某些情况下很有用。 下面是一些简单的使用代码: import sqlite3 from uuid import uuid1# 连接数据库文件,如果不存在会创建 with…...

实测文心一言4.0,真的比GPT-4毫不逊色吗?
10月17日,李彦宏在百度世界2023上表示。当天,李彦宏以《手把手教你做AI原生应用》为主题发表演讲,发布文心大模型4.0版本。 今天,咱们就开门见山啊。这一回要测一测,昨天才发布的文心一言大模型 4.0。 之所以要测它&…...
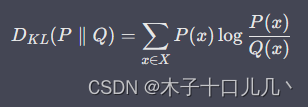
损失函数——KL散度(Kullback-Leibler Divergence,KL Divergence)
KL散度(Kullback-Leibler Divergence,简称KL散度)是一种度量两个概率分布之间差异的指标,也被称为相对熵(Relative Entropy)。KL散度被广泛应用于信息论、统计学、机器学习和数据科学等领域。 KL散度衡量的…...
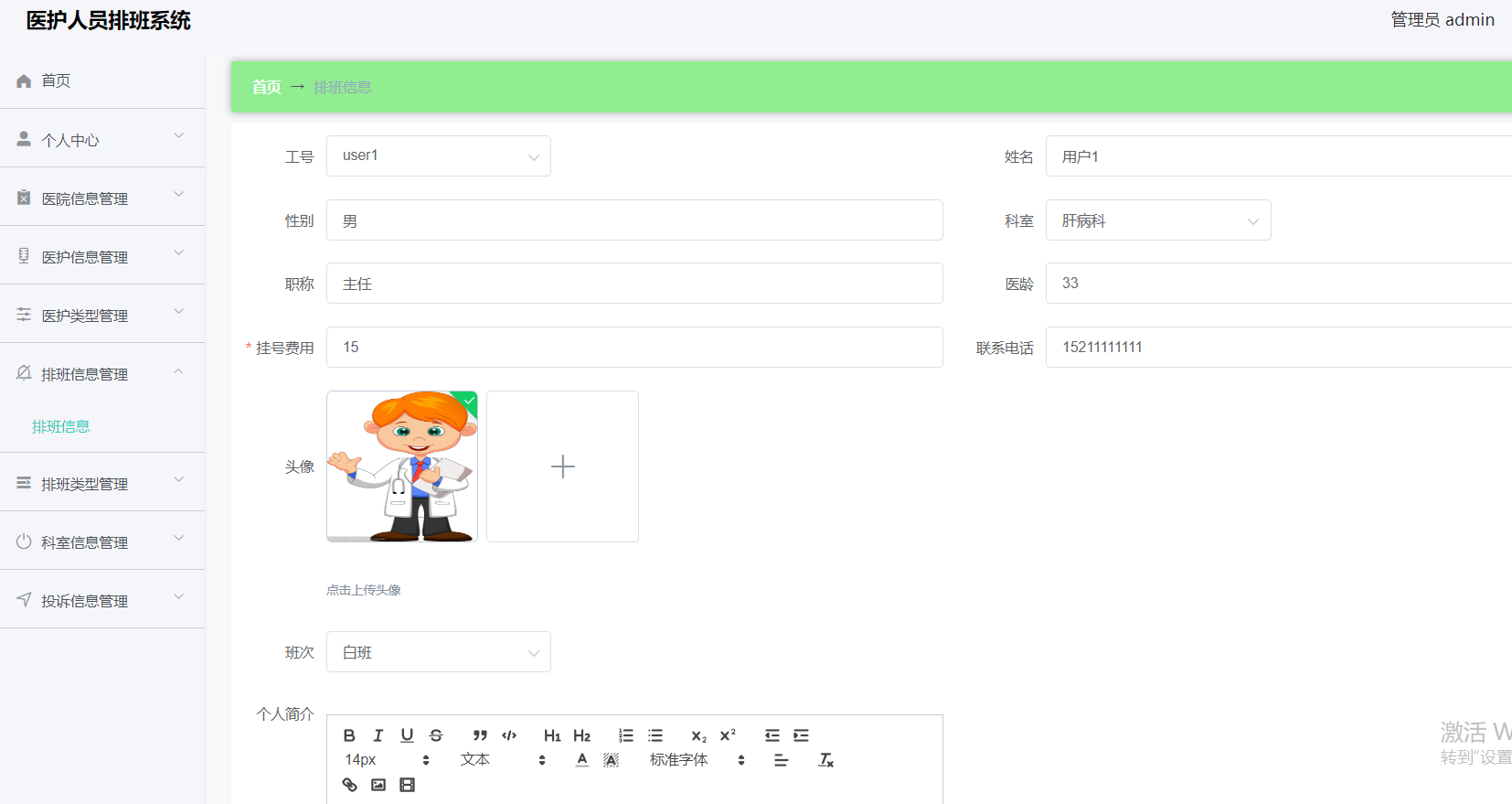
基于springboot的医护人员排班系统 全套代码 全套文档
基于springboot的医护人员排班系统,springboot vue mysql (毕业论文10411字以上,共27页,程序代码,MySQL数据库) 代码下载链接:https://pan.baidu.com/s/177HdCGtTvqiHP4O7qWAgxA?pwd0jlf 提取码:0jlf 【运行环境】 IDEA, JDK1.8, Mysql, Node, Vue …...
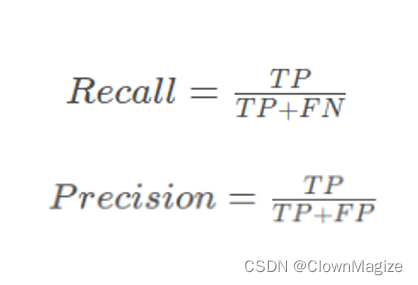
【YOLOX简述】
YOLOX的简述 一、 原因1. 背景2. 概念 二、 算法介绍2.1 YOLOX算法结构图:2.2 算法独特点2.3 Focus网络结构2.4 FPN,PAN2.5 BaseConv2.6 SPP2.7 CSPDarknet2.8 YOlO Head 三、预测曲线3.1 曲线 一、 原因 1. 背景 工业的缺陷检测是计算机视觉中不可缺少…...

一文带你深入浅出Web的自动化测试工具Selenium【建议收藏】
文章目录 前言第01节 Selenium概述第02节 安装浏览器驱动(以Google为例)第03节 定位页面元素1. 打开指定页面2. id 定位3. name 定位4. class 定位5. tag 定位6. xpath 定位7. css 选择器8. link 定位9. 示例 有道翻译 第04节 浏览器控制1. 修改浏览器窗…...
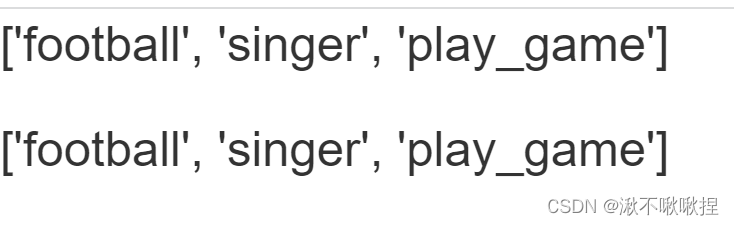
Django模版层
解析: forloop内置对象:运行结果解析 counter0: 从0开始计数 counter : 从1开始计数 first: True,判断循环的开始 last : Tues,判断循环的结束模版变量的书写 我们可以在html中编写python代码。 演示: {{ 填写变量 }}{% 填写类的 %}{{ d.0 }} {{ d.1 }…...

同一个IP地址可有不同的写法?
每个人在上网的时候,都会被分配一个IP地址,这是互联网世界中的“身份证号码”。IP地址是以数字形式呈现的,例如192.168.1.1。然而,你是否知道,尽管一个IP地址的数字串唯一标识一个设备,但它可以有不同的写法…...

《Effective C++》条款13
以对象管理资源 有这样一段代码: class A { public:A* create(){...}}; class B :public A { public:A* ptr create();...delete ptr; }; 我们定义了ptr去接收create()函数的返回值,并且在最后进行了回收资源。看似是没问题的。但是实际上有很多隐患&am…...
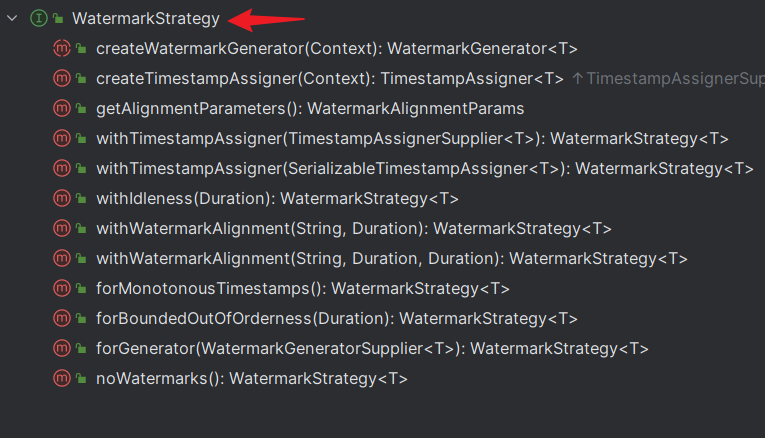
【入门Flink】- 09Flink水位线Watermark
在窗口的处理过程中,基于数据的时间戳,自定义一个“逻辑时钟”。这个时钟的时间不会自动流逝;它的时间进展,就是靠着新到数据的时间戳来推动的。 什么是水位线 用来衡量事件时间进展的标记,就被称作“水位线”&#x…...

华为交换机的基本配置,看完秒懂
一、 交换机的基本配置 交换机连接方式 本地:计算机COM口/USB口 --> Console线 --> 交换机Console口 远程:Putty、SecureCRT、Xshell远程管理工具 华为VRP网络操作系统 1)华为的视图模式 <Huawei> //用户视图&#x…...
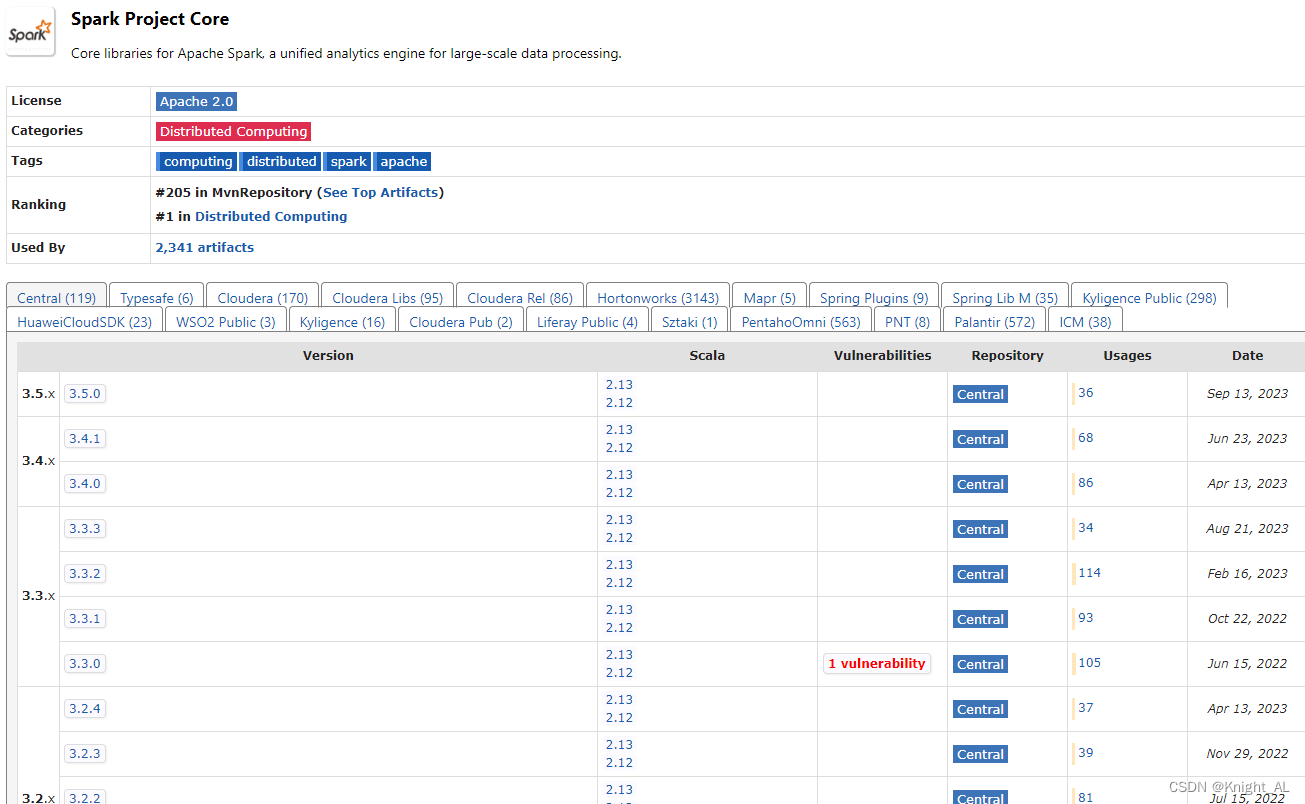
spark与scala的对应版本查看
仓库地址 https://mvnrepository.com/artifact/org.apache.spark/spark-core 总结 spark3.0 以后,不再支持 scala2.11spark3.0 以后,只能用 scala2.12以上...
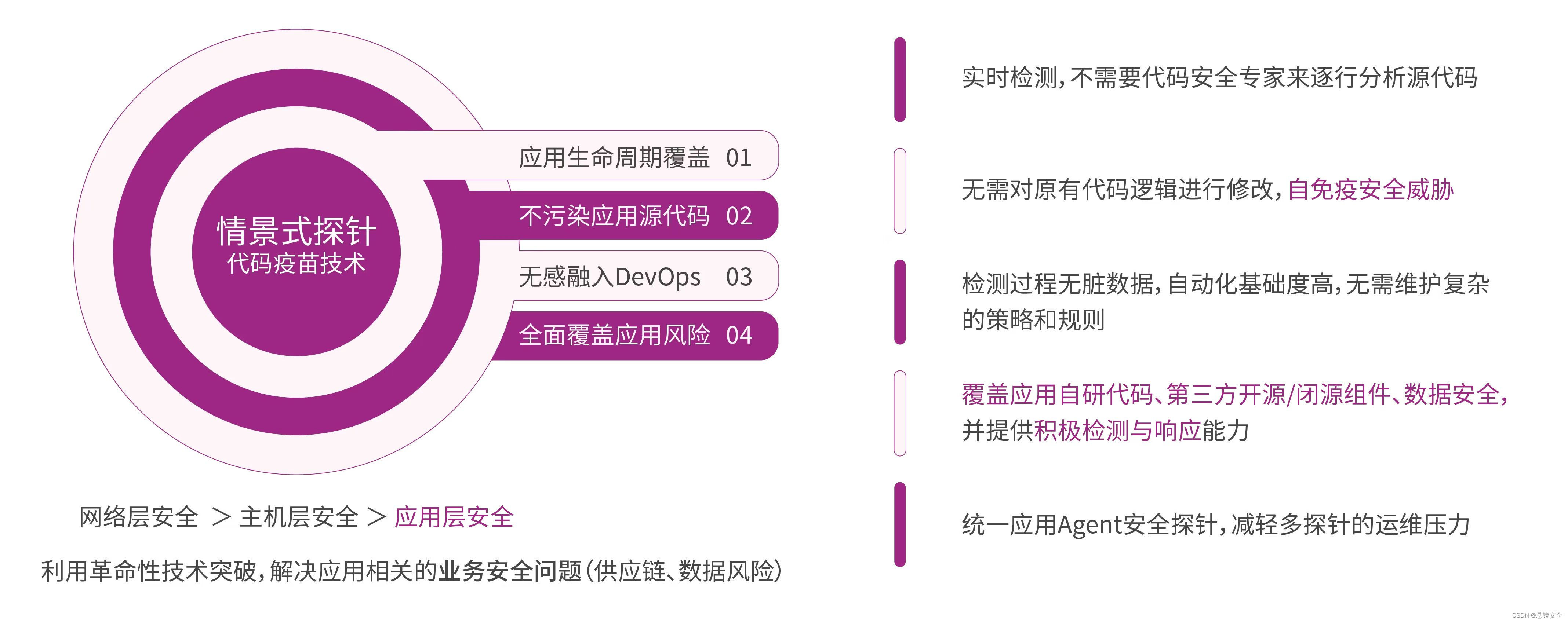
影响力|子芽首创代码疫苗技术获评“年度技术突破者”
近日,由业界权威机构嘶吼产业研究院主办的2023网络安全“金帽子”年度评选结果已正式公布。本届评选由网安产业监管机构及相关委办局领导坐镇、行业资深专家、头部网安企业负责人权威加持。凭借首创的专利级代码疫苗技术创新突破了数字供应链安全领域关键核心技术&a…...
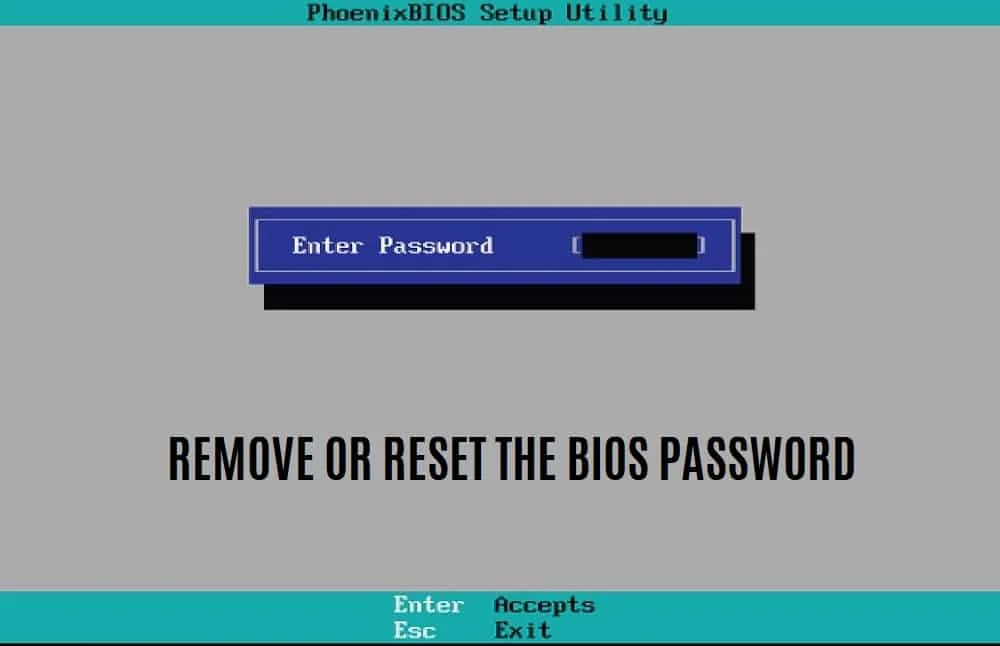
还在为忘记BIOS密码担心?至少有五种方法可以重置或删除BIOS密码
忘记密码是一个我们都非常熟悉的问题。虽然在大多数情况下,只需单击“忘记密码”选项,然后按照几个简单的步骤即可恢复访问权限,但情况并非总是如此。忘记BIOS密码(通常为避免进入BIOS设置或避免个人计算机启动而设置的密码)意味着你将无法完全启动系统。 幸运的是,就像…...

部署百川大语言模型Baichuan2
Baichuan2是百川智能推出的新一代开源大语言模型,采用 2.6 万亿 Tokens 的高质量语料训练。在多个权威的中文、英文和多语言的通用、领域 benchmark 上取得同尺寸最佳的效果。包含有 7B、13B 的 Base 和 Chat 版本,并提供了 Chat 版本的 4bits 量化。 模…...