R可视化:ggpubr包学习
欢迎大家关注全网生信学习者系列:
-
WX公zhong号:生信学习者
-
Xiao hong书:生信学习者
-
知hu:生信学习者
-
CDSN:生信学习者2
介绍
ggpubr是我经常会用到的R包,它傻瓜式的画图方式对很多初次接触R绘图的人来讲是很友好的。该包有个stat_compare_means函数可以做组间假设检验分析。
安装R包
install.packages("ggpubr") devtools::devtools::install_github("kassambara/ggpubr") library(ggpubr) plotdata <- data.frame(sex = factor(rep(c("F", "M"), each=200)),weight = c(rnorm(200, 55), rnorm(200, 58)))
密度图density
ggdensity(plotdata, x = "weight",add = "mean", rug = TRUE, # x轴显示分布密度color = "sex", fill = "sex",palette = c("#00AFBB", "#E7B800"))
柱状图histogram
gghistogram(plotdata, x = "weight",bins = 30,add = "mean", rug = TRUE,color = "sex", fill = "sex",palette = c("#00AFBB", "#E7B800"))
箱线图boxplot
df <- ToothGrowth head(df) my_comparisons <- list( c("0.5", "1"), c("1", "2"), c("0.5", "2") ) ggboxplot(df, x = "dose", y = "len",color = "dose", palette =c("#00AFBB", "#E7B800", "#FC4E07"),add = "jitter", shape = "dose")+stat_compare_means(comparisons = my_comparisons)+ # Add pairwise comparisons p-valuestat_compare_means(label.y = 50)
小提琴图violin
ggviolin(df, x = "dose", y = "len", fill = "dose",palette = c("#00AFBB", "#E7B800", "#FC4E07"),add = "boxplot", add.params = list(fill = "white"))+stat_compare_means(comparisons = my_comparisons, label = "p.signif")+ # Add significance levelsstat_compare_means(label.y = 50)
点图dotplot
ggdotplot(ToothGrowth, x = "dose", y = "len",color = "dose", palette = "jco", binwidth = 1)
有序条形图 ordered bar plots
data("mtcars") dfm <- mtcars dfm$cyl <- as.factor(dfm$cyl) dfm$name <- rownames(dfm) head(dfm[, c("name", "wt", "mpg", "cyl")]) ggbarplot(dfm, x = "name", y = "mpg",fill = "cyl", # change fill color by cylcolor = "white", # Set bar border colors to whitepalette = "jco", # jco journal color palett. see ?ggparsort.val = "asc", # Sort the value in dscending ordersort.by.groups = TRUE, # Sort inside each groupx.text.angle = 90) # Rotate vertically x axis texts
偏差图Deviation graphs
dfm$mpg_z <- (dfm$mpg -mean(dfm$mpg))/sd(dfm$mpg) dfm$mpg_grp <- factor(ifelse(dfm$mpg_z < 0, "low", "high"), levels = c("low", "high")) # Inspect the data head(dfm[, c("name", "wt", "mpg", "mpg_z", "mpg_grp", "cyl")]) ggbarplot(dfm, x = "name", y = "mpg_z",fill = "mpg_grp", # change fill color by mpg_levelcolor = "white", # Set bar border colors to whitepalette = "jco", # jco journal color palett. see ?ggparsort.val = "asc", # Sort the value in ascending ordersort.by.groups = FALSE, # Don't sort inside each groupx.text.angle = 90, # Rotate vertically x axis textsylab = "MPG z-score",rotate = FALSE,xlab = FALSE,legend.title = "MPG Group")
棒棒糖图 lollipop chart
ggdotchart(dfm, x = "name", y = "mpg",color = "cyl", # Color by groupspalette = c("#00AFBB", "#E7B800", "#FC4E07"), # Custom color palettesorting = "descending", # Sort value in descending orderadd = "segments", # Add segments from y = 0 to dotsrotate = TRUE, # Rotate verticallygroup = "cyl", # Order by groupsdot.size = 6, # Large dot sizelabel = round(dfm$mpg), # Add mpg values as dot labelsfont.label = list(color = "white", size = 9, vjust = 0.5), # Adjust label parametersggtheme = theme_pubr()) # ggplot2 theme
偏差图Deviation graph
ggdotchart(dfm, x = "name", y = "mpg_z",color = "cyl", # Color by groupspalette = c("#00AFBB", "#E7B800", "#FC4E07"), # Custom color palettesorting = "descending", # Sort value in descending orderadd = "segments", # Add segments from y = 0 to dotsadd.params = list(color = "lightgray", size = 2), # Change segment color and sizegroup = "cyl", # Order by groupsdot.size = 6, # Large dot sizelabel = round(dfm$mpg_z,1), # Add mpg values as dot labelsfont.label = list(color = "white", size = 9, vjust = 0.5), # Adjust label parametersggtheme = theme_pubr())+ # ggplot2 themegeom_hline(yintercept = 0, linetype = 2, color = "lightgray")
散点图scatterplot
df <- datasets::iris head(df) ggscatter(df, x = 'Sepal.Width', y = 'Sepal.Length', palette = 'jco', shape = 'Species', add = 'reg.line',color = 'Species', conf.int = TRUE)
-
添加回归线的系数
ggscatter(df, x = 'Sepal.Width', y = 'Sepal.Length', palette = 'jco', shape = 'Species', add = 'reg.line',color = 'Species', conf.int = TRUE)+stat_cor(aes(color=Species),method = "pearson", label.x = 3)
-
添加聚类椭圆 concentration ellipses
data("mtcars") dfm <- mtcars dfm$cyl <- as.factor(dfm$cyl) dfm$name <- rownames(dfm) p1 <- ggscatter(dfm, x = "wt", y = "mpg",color = "cyl", palette = "jco",shape = "cyl",ellipse = TRUE) p2 <- ggscatter(dfm, x = "wt", y = "mpg",color = "cyl", palette = "jco",shape = "cyl",ellipse = TRUE,ellipse.type = "convex") cowplot::plot_grid(p1, p2, align = "hv", nrow = 1)
-
添加mean和stars
ggscatter(dfm, x = "wt", y = "mpg",color = "cyl", palette = "jco",shape = "cyl",ellipse = TRUE, mean.point = TRUE,star.plot = TRUE)
-
显示点标签
dfm$name <- rownames(dfm) p3 <- ggscatter(dfm, x = "wt", y = "mpg",color = "cyl", palette = "jco",label = "name",repel = TRUE) p4 <- ggscatter(dfm, x = "wt", y = "mpg",color = "cyl", palette = "jco",label = "name",repel = TRUE,label.select = c("Toyota Corolla", "Merc 280", "Duster 360")) cowplot::plot_grid(p3, p4, align = "hv", nrow = 1)
气泡图bubble plot
ggscatter(dfm, x = "wt", y = "mpg",color = "cyl",palette = "jco",size = "qsec", alpha = 0.5)+scale_size(range = c(0.5, 15)) # Adjust the range of points size
连线图 lineplot
p1 <- ggbarplot(ToothGrowth, x = "dose", y = "len", add = "mean_se",color = "supp", palette = "jco", position = position_dodge(0.8))+stat_compare_means(aes(group = supp), label = "p.signif", label.y = 29) p2 <- ggline(ToothGrowth, x = "dose", y = "len", add = "mean_se",color = "supp", palette = "jco")+stat_compare_means(aes(group = supp), label = "p.signif", label.y = c(16, 25, 29)) cowplot::plot_grid(p1, p2, ncol = 2, align = "hv")
添加边沿图 marginal plots
library(ggExtra) p <- ggscatter(iris, x = "Sepal.Length", y = "Sepal.Width",color = "Species", palette = "jco",size = 3, alpha = 0.6) ggMarginal(p, type = "boxplot")
-
第二种添加方式: 分别画出三个图,然后进行组合
sp <- ggscatter(iris, x = "Sepal.Length", y = "Sepal.Width",color = "Species", palette = "jco",size = 3, alpha = 0.6, ggtheme = theme_bw()) xplot <- ggboxplot(iris, x = "Species", y = "Sepal.Length", color = "Species", fill = "Species", palette = "jco",alpha = 0.5, ggtheme = theme_bw())+ rotate() yplot <- ggboxplot(iris, x = "Species", y = "Sepal.Width",color = "Species", fill = "Species", palette = "jco",alpha = 0.5, ggtheme = theme_bw()) sp <- sp + rremove("legend") yplot <- yplot + clean_theme() + rremove("legend") xplot <- xplot + clean_theme() + rremove("legend") cowplot::plot_grid(xplot, NULL, sp, yplot, ncol = 2, align = "hv", rel_widths = c(2, 1), rel_heights = c(1, 2))
-
上图主图和边沿图之间的space太大,第三种方法能克服这个缺点
library(cowplot) # Main plot pmain <- ggplot(iris, aes(x = Sepal.Length, y = Sepal.Width, color = Species))+geom_point()+ggpubr::color_palette("jco") # Marginal densities along x axis xdens <- axis_canvas(pmain, axis = "x")+geom_density(data = iris, aes(x = Sepal.Length, fill = Species),alpha = 0.7, size = 0.2)+ggpubr::fill_palette("jco") # Marginal densities along y axis # Need to set coord_flip = TRUE, if you plan to use coord_flip() ydens <- axis_canvas(pmain, axis = "y", coord_flip = TRUE)+geom_boxplot(data = iris, aes(x = Sepal.Width, fill = Species),alpha = 0.7, size = 0.2)+coord_flip()+ggpubr::fill_palette("jco") p1 <- insert_xaxis_grob(pmain, xdens, grid::unit(.2, "null"), position = "top") p2 <- insert_yaxis_grob(p1, ydens, grid::unit(.2, "null"), position = "right") ggdraw(p2)
-
第四种方法,通过grob设置
# Scatter plot colored by groups ("Species") #:::::::::::::::::::::::::::::::::::::::::::::::::::::::::: sp <- ggscatter(iris, x = "Sepal.Length", y = "Sepal.Width",color = "Species", palette = "jco",size = 3, alpha = 0.6) # Create box plots of x/y variables #:::::::::::::::::::::::::::::::::::::::::::::::::::::::::: # Box plot of the x variable xbp <- ggboxplot(iris$Sepal.Length, width = 0.3, fill = "lightgray") +rotate() +theme_transparent() # Box plot of the y variable ybp <- ggboxplot(iris$Sepal.Width, width = 0.3, fill = "lightgray") +theme_transparent() # Create the external graphical objects # called a "grop" in Grid terminology xbp_grob <- ggplotGrob(xbp) ybp_grob <- ggplotGrob(ybp) # Place box plots inside the scatter plot #:::::::::::::::::::::::::::::::::::::::::::::::::::::::::: xmin <- min(iris$Sepal.Length); xmax <- max(iris$Sepal.Length) ymin <- min(iris$Sepal.Width); ymax <- max(iris$Sepal.Width) yoffset <- (1/15)*ymax; xoffset <- (1/15)*xmax # Insert xbp_grob inside the scatter plot sp + annotation_custom(grob = xbp_grob, xmin = xmin, xmax = xmax, ymin = ymin-yoffset, ymax = ymin+yoffset) +# Insert ybp_grob inside the scatter plotannotation_custom(grob = ybp_grob,xmin = xmin-xoffset, xmax = xmin+xoffset, ymin = ymin, ymax = ymax)
二维密度图 2d density
sp <- ggscatter(iris, x = "Sepal.Length", y = "Sepal.Width",color = "lightgray") p1 <- sp + geom_density_2d() # Gradient color p2 <- sp + stat_density_2d(aes(fill = ..level..), geom = "polygon") # Change gradient color: custom p3 <- sp + stat_density_2d(aes(fill = ..level..), geom = "polygon")+gradient_fill(c("white", "steelblue")) # Change the gradient color: RColorBrewer palette p4 <- sp + stat_density_2d(aes(fill = ..level..), geom = "polygon") +gradient_fill("YlOrRd") cowplot::plot_grid(p1, p2, p3, p4, ncol = 2, align = "hv")
混合图
混合表、字体和图
# Density plot of "Sepal.Length" #:::::::::::::::::::::::::::::::::::::: density.p <- ggdensity(iris, x = "Sepal.Length", fill = "Species", palette = "jco") # Draw the summary table of Sepal.Length #:::::::::::::::::::::::::::::::::::::: # Compute descriptive statistics by groups stable <- desc_statby(iris, measure.var = "Sepal.Length",grps = "Species") stable <- stable[, c("Species", "length", "mean", "sd")] # Summary table plot, medium orange theme stable.p <- ggtexttable(stable, rows = NULL, theme = ttheme("mOrange")) # Draw text #:::::::::::::::::::::::::::::::::::::: text <- paste("iris data set gives the measurements in cm","of the variables sepal length and width","and petal length and width, respectively,","for 50 flowers from each of 3 species of iris.","The species are Iris setosa, versicolor, and virginica.", sep = " ") text.p <- ggparagraph(text = text, face = "italic", size = 11, color = "black") # Arrange the plots on the same page ggarrange(density.p, stable.p, text.p, ncol = 1, nrow = 3,heights = c(1, 0.5, 0.3))
-
注释table在图上
density.p <- ggdensity(iris, x = "Sepal.Length", fill = "Species", palette = "jco") stable <- desc_statby(iris, measure.var = "Sepal.Length",grps = "Species") stable <- stable[, c("Species", "length", "mean", "sd")] stable.p <- ggtexttable(stable, rows = NULL, theme = ttheme("mOrange")) density.p + annotation_custom(ggplotGrob(stable.p),xmin = 5.5, ymin = 0.7,xmax = 8)
systemic information
sessionInfo()
R version 3.6.1 (2019-07-05) Platform: x86_64-w64-mingw32/x64 (64-bit) Running under: Windows 10 x64 (build 19042) Matrix products: default locale: [1] LC_COLLATE=Chinese (Simplified)_China.936 LC_CTYPE=Chinese (Simplified)_China.936 [3] LC_MONETARY=Chinese (Simplified)_China.936 LC_NUMERIC=C [5] LC_TIME=Chinese (Simplified)_China.936 attached base packages: [1] stats graphics grDevices utils datasets methods base other attached packages: [1] ggpubr_0.4.0 ggplot2_3.3.2 loaded via a namespace (and not attached):[1] zip_2.0.4 Rcpp_1.0.3 cellranger_1.1.0 pillar_1.4.6 compiler_3.6.1 forcats_0.5.0 [7] tools_3.6.1 digest_0.6.27 lifecycle_0.2.0 tibble_3.0.4 gtable_0.3.0 pkgconfig_2.0.3 [13] rlang_0.4.8 openxlsx_4.2.3 ggsci_2.9 rstudioapi_0.10 curl_4.3 haven_2.3.1 [19] rio_0.5.16 withr_2.1.2 dplyr_1.0.2 generics_0.0.2 vctrs_0.3.4 hms_0.5.3 [25] grid_3.6.1 tidyselect_1.1.0 glue_1.4.2 data.table_1.13.2 R6_2.4.1 rstatix_0.6.0 [31] readxl_1.3.1 foreign_0.8-73 carData_3.0-4 farver_2.0.3 tidyr_1.0.0 purrr_0.3.3 [37] car_3.0-10 magrittr_1.5 scales_1.1.0 backports_1.1.10 ellipsis_0.3.1 abind_1.4-5 [43] colorspace_1.4-1 ggsignif_0.6.0 labeling_0.4.2 stringi_1.4.3 munsell_0.5.0 broom_0.7.2 [49] crayon_1.3.4
相关文章:
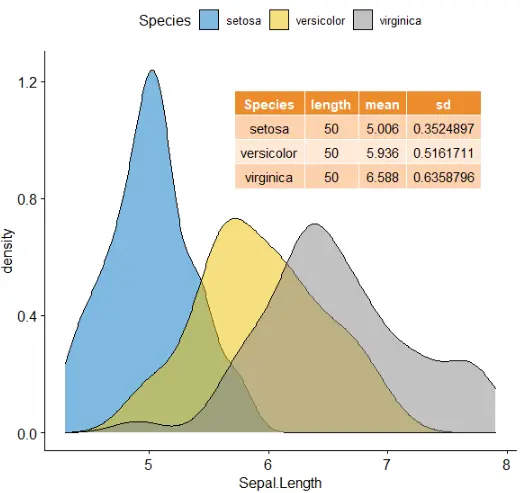
R可视化:ggpubr包学习
欢迎大家关注全网生信学习者系列: WX公zhong号:生信学习者 Xiao hong书:生信学习者 知hu:生信学习者 CDSN:生信学习者2 介绍 ggpubr是我经常会用到的R包,它傻瓜式的画图方式对很多初次接触R绘图的人来…...
优化Spring Boot项目启动时间:详解与实践
目录 引言了解Spring Boot框架启动机制常见启动瓶颈分析优化策略 禁用不必要的自动配置使用Profile进行开发和生产环境区分精简依赖延迟加载Bean并行初始化Bean缓存数据源连接优化Spring Data JPA使用Spring Boot DevTools 通过性能测试工具分析和优化实战示例:一个…...
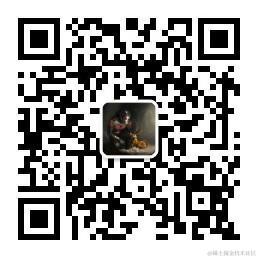
Android如何简单快速实现RecycleView的拖动重排序功能
本文首发于公众号“AntDream”,欢迎微信搜索“AntDream”或扫描文章底部二维码关注,和我一起每天进步一点点 要实现这个拖动重排序功能,主要是用到了RecycleView的ItemTouchHelper类 首先是定义一个接口 interface ItemTouchHelperAdapter …...
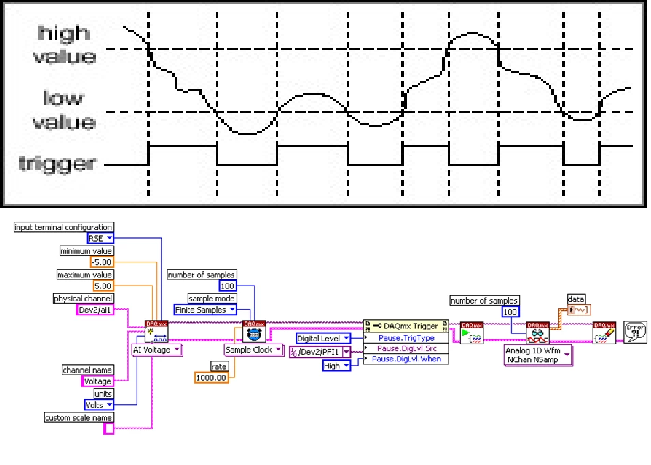
LabVIEW利用旋转编码器脉冲触发数据采集
利用旋转编码器发出的脉冲控制数据采集,可以采用硬件触发方式,以确保每个脉冲都能触发一次数据采集。本文提供了详细的解决方案,包括硬件连接、LabVIEW编程和触发设置,确保数据采集的准确性和实时性。 一、硬件连接 1. 旋转编码…...
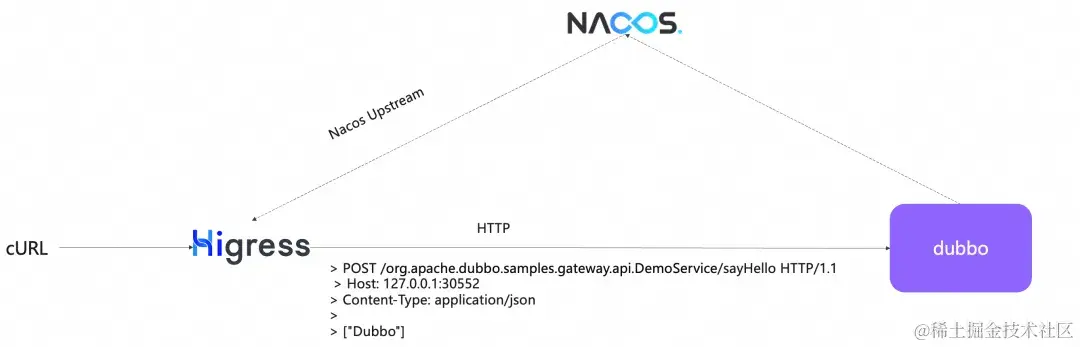
Dubbo3 服务原生支持 http 访问,兼具高性能与易用性
作者:刘军 作为一款 rpc 框架,Dubbo 的优势是后端服务的高性能的通信、面向接口的易用性,而它带来的弊端则是 rpc 接口的测试与前端流量接入成本较高,我们需要专门的工具或协议转换才能实现后端服务调用。这个现状在 Dubbo3 中得…...
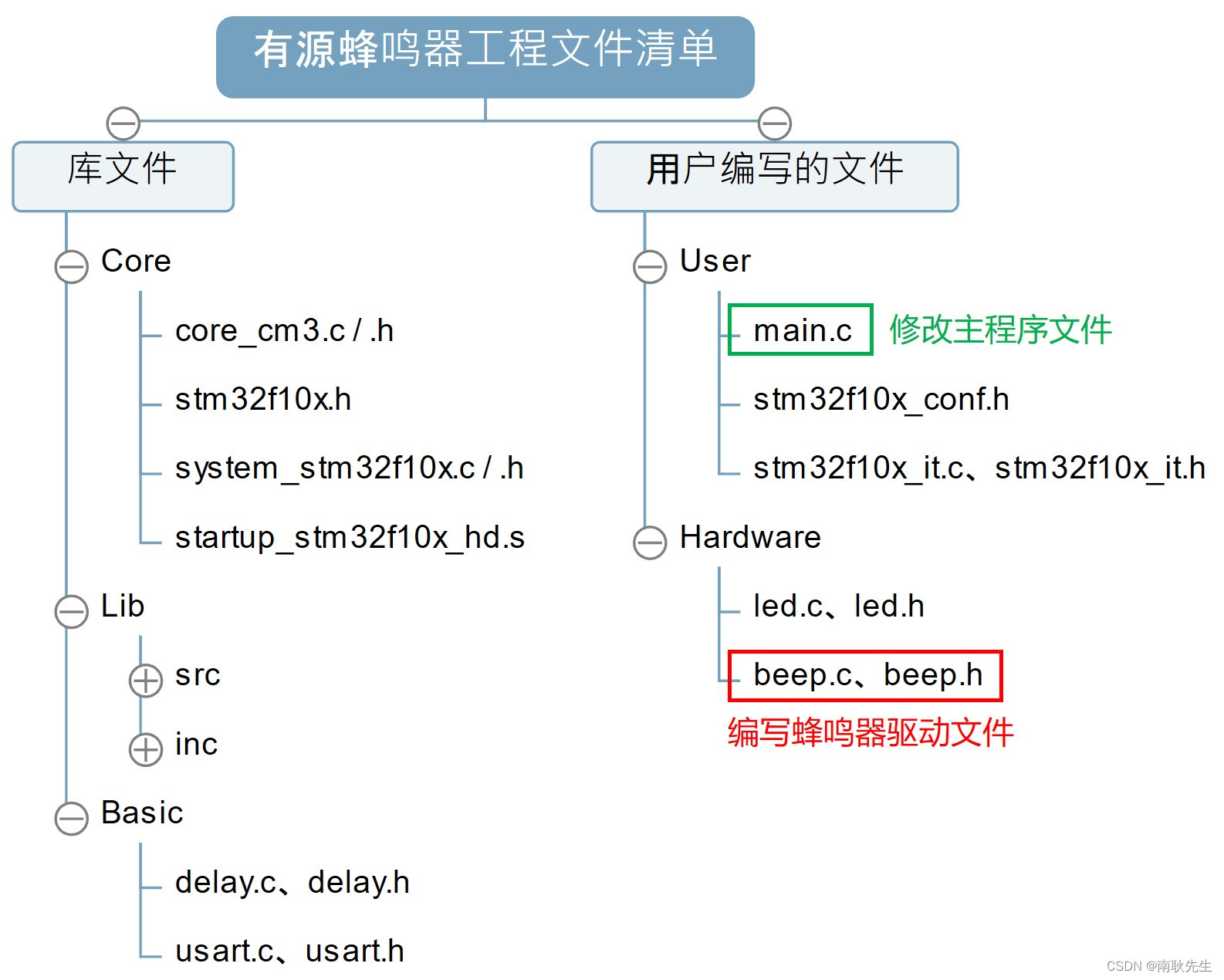
我在高职教STM32——GPIO入门之蜂鸣器
大家好,我是老耿,高职青椒一枚,一直从事单片机、嵌入式、物联网等课程的教学。对于高职的学生层次,同行应该都懂的,老师在课堂上教学几乎是没什么成就感的。正因如此,才有了借助 CSDN 平台寻求认同感和成就…...
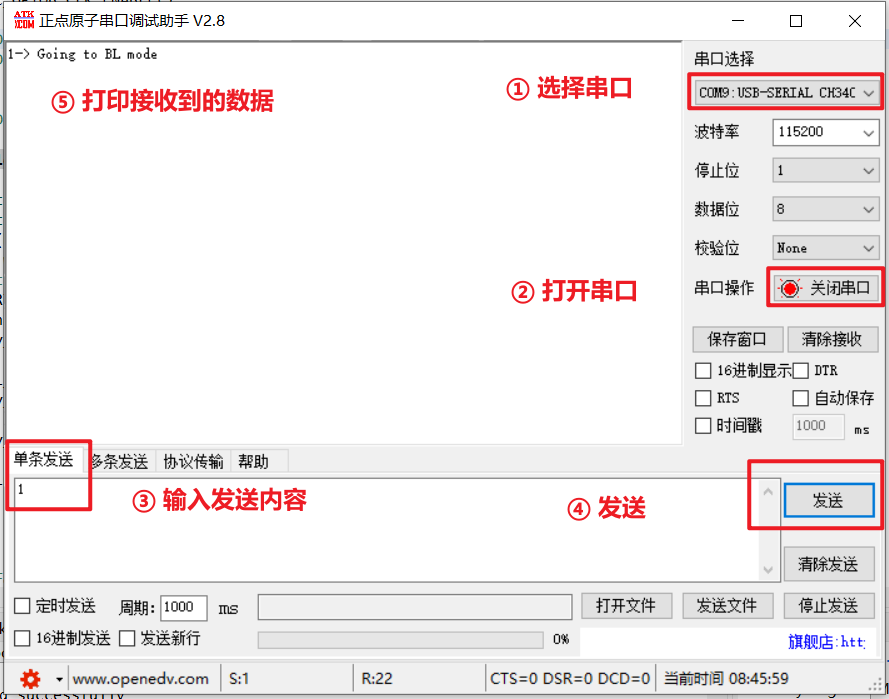
STM32 Customer BootLoader 刷新项目 (一) STM32CubeMX UART串口通信工程搭建
STM32 Customer BootLoader 刷新项目 (一) STM32CubeMX UART串口通信工程搭建 文章目录 STM32 Customer BootLoader 刷新项目 (一) STM32CubeMX UART串口通信工程搭建功能与作用典型工作流程 1. 硬件原理图介绍2. STM32 CubeMX工程搭建2.1 创建工程2.2 系统配置2.3 USART串口配…...
如果搜索一定超时,如何用dp来以空间换时间
E - Alphabet Tiles (atcoder.jp) 题目大意:1到k长度的字符串时,在A-Z给定数量下,搭配出多少种不同的字符串 思路 排列组合,会死人的 暴搜:可以解决,但是时间太长 dp:考虑前 i 个字母&…...

MySQL常见的命令
MySQL常见的命令 查看数据库(注意添加分号) show databases;进入到某个库 use 库; 例如:进入test use test;显示表格 show tables;直接展示某个库里面的表 show tables from 库; 例如:展示mysql中的表格 show tabl…...
11 类型泛化
11 类型泛化 1、函数模版1.1 前言1.2 函数模版1.3 隐式推断类型实参1.4 函数模板重载1.5 函数模板类型形参的默认类型(C11标准) 2、类模版2.1 类模板的成员函数延迟实例化2.2 类模板的静态成员2.3 类模板的递归实例化2.4 类模板类型形参缺省值 3、类模板…...
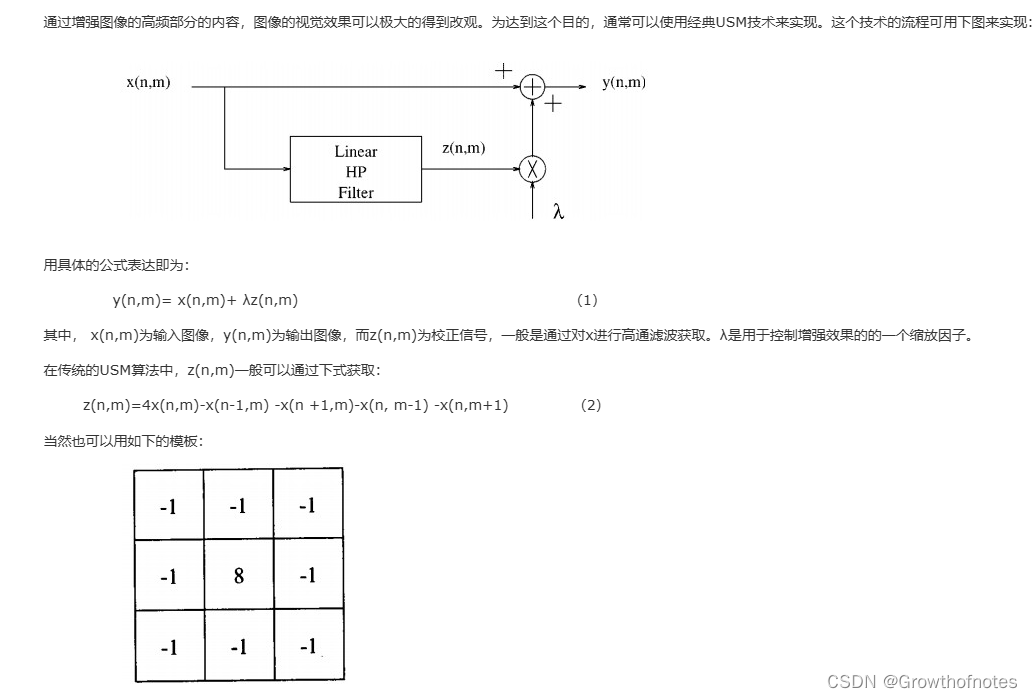
UE4_后期_ben_模糊和锐化滤镜
学习笔记,不喜勿喷,侵权立删,祝愿生活越来越好! 本篇教程主要介绍后期处理的简单模糊和锐化滤镜效果,学习之前首先要回顾下上节课介绍的屏幕扭曲效果: 这是全屏效果,然后又介绍了几种蒙版&#…...
Spring Boot中Excel的导入导出的实现之Apache POI框架使用教程
文章目录 前言一、Apache POI 是什么?二、使用 Apache POI 实现 Excel 的导入和导出① 导入 Excel1. 添加依赖2. 编写导入逻辑3. 在 Controller 中处理上传请求 ② 导出 Excel1. 添加依赖2. 编写导出逻辑3. 在 Controller 中处理导出请求 总结 前言 在 Spring Boot …...

CentOS搭建kubernetes集群详细过程(yum安装方式)
kubernetes集群搭建详细过程(yum安装方式) Kubernetes,也被称为K8s,是一个多功能的容器管理工具,它不仅能够协调和调度容器的部署,而且还能监控容器的健康状况并自动修复常见问题。这个平台是在谷歌十多年…...
Java 面试题:Java 的 Exception 和 Error 有什么区别?
在Java编程中,异常处理是确保程序稳健性和可靠性的重要机制。Java提供了一套完善的异常处理框架,通过捕获和处理异常,开发者可以有效地应对程序运行时可能出现的各种问题。在这一框架中,Exception和Error是两个核心概念࿰…...
在Vue 3中,el-select循环el-option的常见踩坑点,value值绑定对象类型?选中效果不准确?
在Vue 3中,el-select 组件是来自 Element Plus UI 库的一部分。 如果你想要设置默认选中的选项,你可以使用 v-model 来绑定选中的值。如果你想要在某个时刻让某个选项显示为已选中,可以设置对应的值到 v-model 绑定的数据。 <template>…...

Qt实现单例模式:Q_GLOBAL_STATIC和Q_GLOBAL_STATIC_WITH_ARGS
目录 1.引言 2.了解Q_GLOBAL_STATIC 3.了解Q_GLOBAL_STATIC_WITH_ARGS 4.实现原理 4.1.对象的创建 4.2.QGlobalStatic 4.3.宏定义实现 4.4.注意事项 5.总结 1.引言 设计模式之单例模式-CSDN博客 所谓的全局静态对象,大多是在单例类中所见,在之前…...
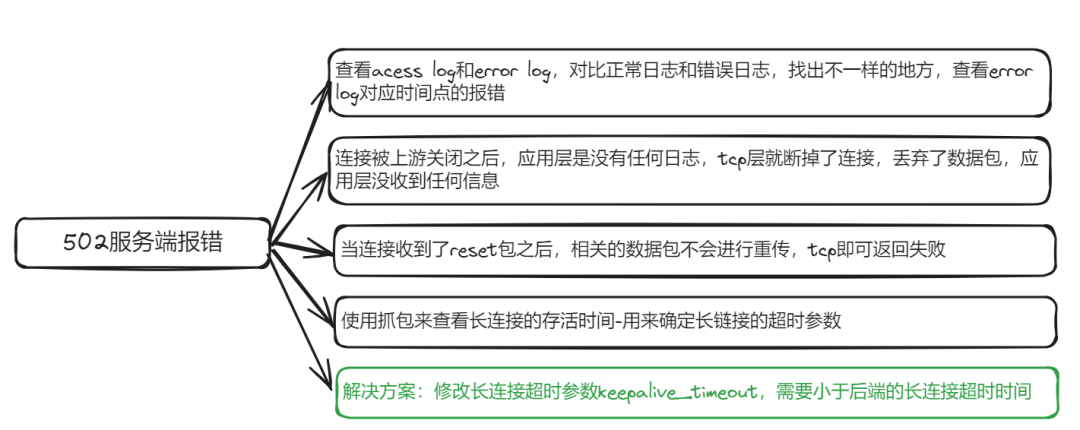
通过nginx转发后应用偶发502bad gateway
序言 学习了一些东西,如何才是真正自己能用的呢?好像就是看自己的潜意识的反应,例如解决了一个问题,那么下次再碰到类似的问题,能直接下意识的去找到对应的信息,从而解决,而不是和第一次碰到一样…...
linux中如何进行yum源的挂载
linux中如何进行yum源的挂载 1.首先创建目录[rootserver /]# mkdir /rhel92.使用mount命令进行、dev/cdrom/的镜像文件进行挂载[rootserver /]# mount /dev/cdrom /rhel9/ 注意:此时设立的是临时命令。重启后则失效,若想在下次开启后仍然挂载&a…...
ffmpeg的部署踩坑及简单使用方式
ffmpeg的使用方式有以下几种: 使用原生安装包 直接在ffmpeg官网上下载安装该软件,加入到环境变量中就可以使用了 优点:简单,灵活,代码中也不用添加其他第三方的包 缺点:需要手动安装ffmpeg,这点比较麻烦 部署-windows 在windows环境下,有时就算加入到了环境变量,…...
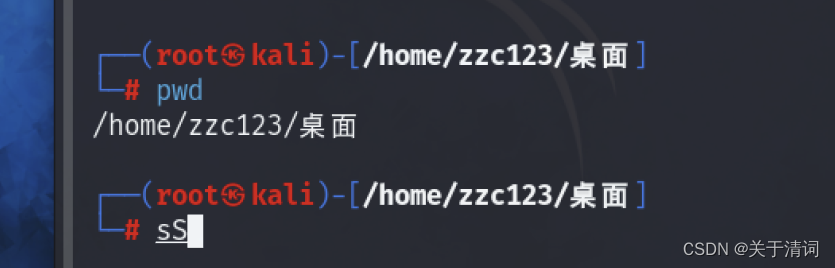
misc刷题记录2[陇剑杯 2021]
[陇剑杯 2021]webshell (1)单位网站被黑客挂马,请您从流量中分析出webshell,进行回答: 黑客登录系统使用的密码是_____________。得到的flag请使用NSSCTF{}格式提交。 这里我的思路是,既然要选择的时间段是黑客登录网站以后&…...
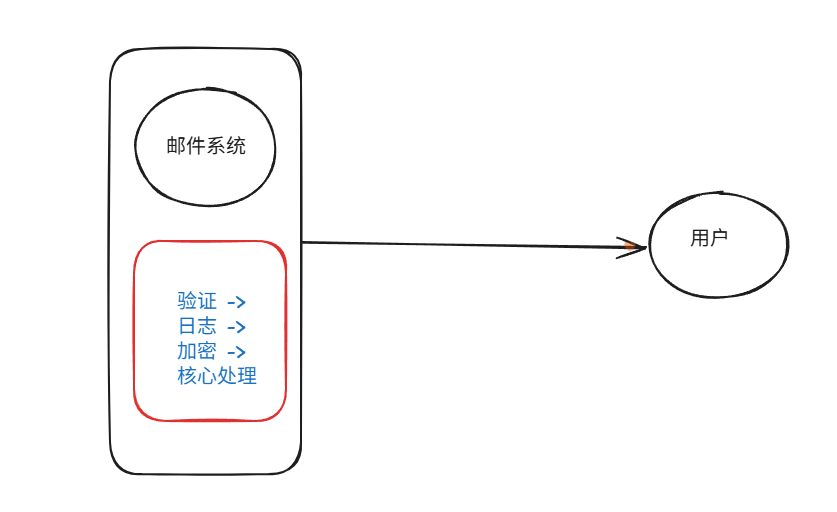
装饰模式(Decorator Pattern)重构java邮件发奖系统实战
前言 现在我们有个如下的需求,设计一个邮件发奖的小系统, 需求 1.数据验证 → 2. 敏感信息加密 → 3. 日志记录 → 4. 实际发送邮件 装饰器模式(Decorator Pattern)允许向一个现有的对象添加新的功能,同时又不改变其…...
Axios请求超时重发机制
Axios 超时重新请求实现方案 在 Axios 中实现超时重新请求可以通过以下几种方式: 1. 使用拦截器实现自动重试 import axios from axios;// 创建axios实例 const instance axios.create();// 设置超时时间 instance.defaults.timeout 5000;// 最大重试次数 cons…...
【python异步多线程】异步多线程爬虫代码示例
claude生成的python多线程、异步代码示例,模拟20个网页的爬取,每个网页假设要0.5-2秒完成。 代码 Python多线程爬虫教程 核心概念 多线程:允许程序同时执行多个任务,提高IO密集型任务(如网络请求)的效率…...
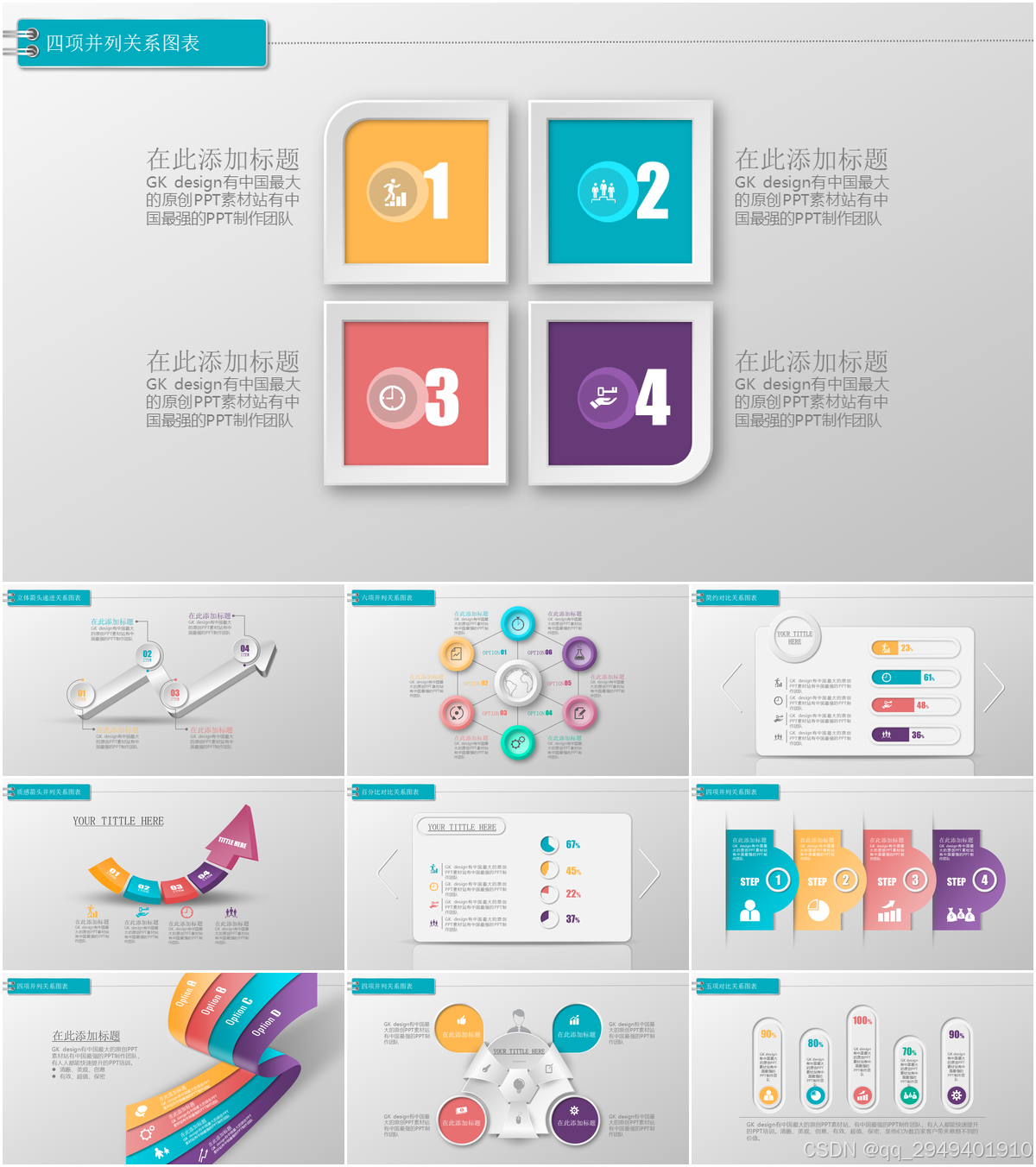
图表类系列各种样式PPT模版分享
图标图表系列PPT模版,柱状图PPT模版,线状图PPT模版,折线图PPT模版,饼状图PPT模版,雷达图PPT模版,树状图PPT模版 图表类系列各种样式PPT模版分享:图表系列PPT模板https://pan.quark.cn/s/20d40aa…...
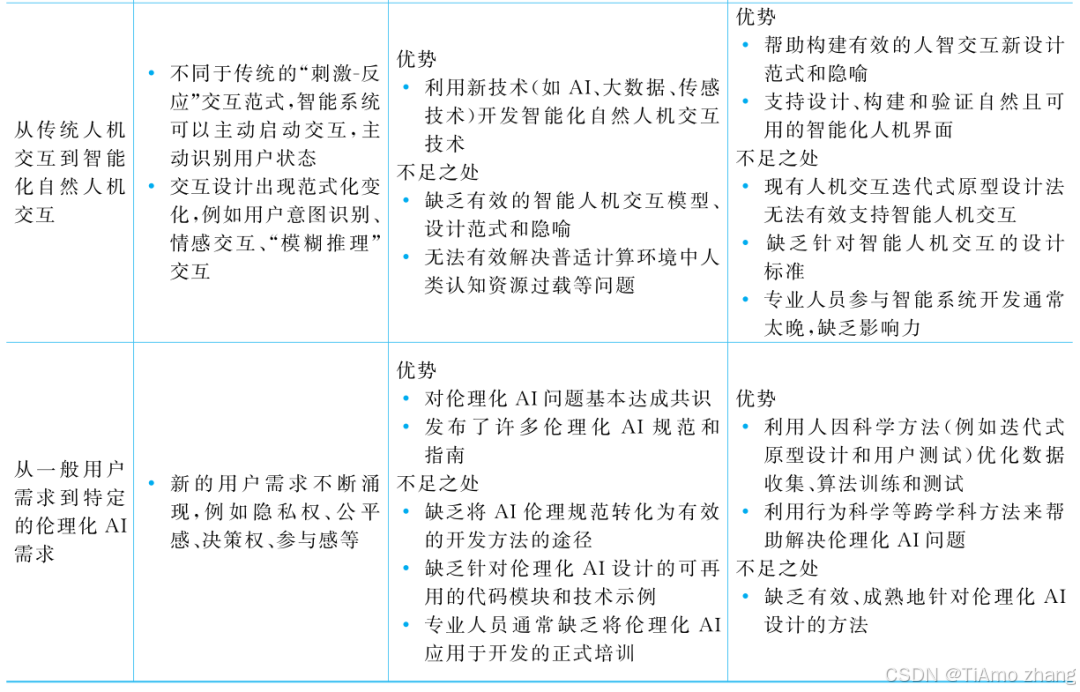
人机融合智能 | “人智交互”跨学科新领域
本文系统地提出基于“以人为中心AI(HCAI)”理念的人-人工智能交互(人智交互)这一跨学科新领域及框架,定义人智交互领域的理念、基本理论和关键问题、方法、开发流程和参与团队等,阐述提出人智交互新领域的意义。然后,提出人智交互研究的三种新范式取向以及它们的意义。最后,总结…...
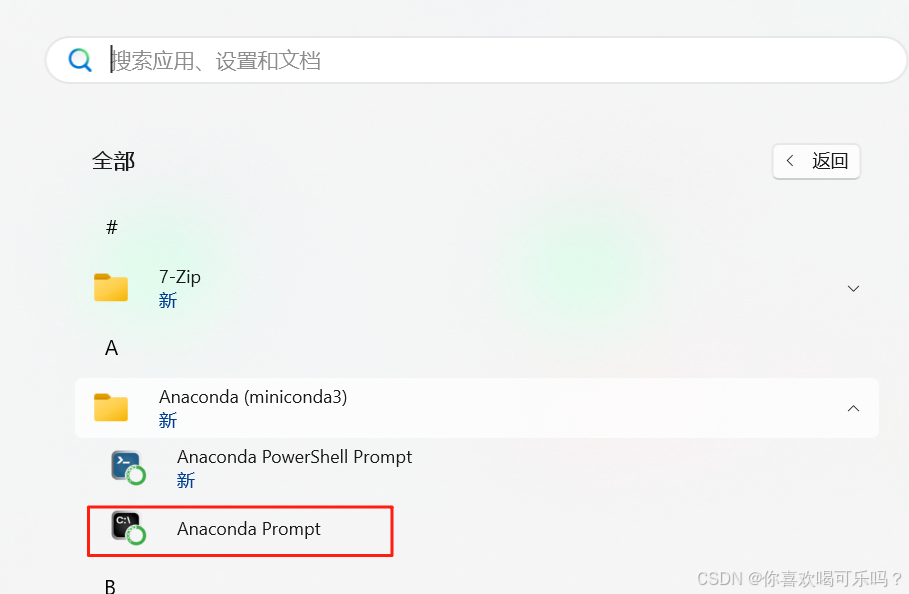
Windows安装Miniconda
一、下载 https://www.anaconda.com/download/success 二、安装 三、配置镜像源 Anaconda/Miniconda pip 配置清华镜像源_anaconda配置清华源-CSDN博客 四、常用操作命令 Anaconda/Miniconda 基本操作命令_miniconda创建环境命令-CSDN博客...
MinIO Docker 部署:仅开放一个端口
MinIO Docker 部署:仅开放一个端口 在实际的服务器部署中,出于安全和管理的考虑,我们可能只能开放一个端口。MinIO 是一个高性能的对象存储服务,支持 Docker 部署,但默认情况下它需要两个端口:一个是 API 端口(用于存储和访问数据),另一个是控制台端口(用于管理界面…...
探索Selenium:自动化测试的神奇钥匙
目录 一、Selenium 是什么1.1 定义与概念1.2 发展历程1.3 功能概述 二、Selenium 工作原理剖析2.1 架构组成2.2 工作流程2.3 通信机制 三、Selenium 的优势3.1 跨浏览器与平台支持3.2 丰富的语言支持3.3 强大的社区支持 四、Selenium 的应用场景4.1 Web 应用自动化测试4.2 数据…...
libfmt: 现代C++的格式化工具库介绍与酷炫功能
libfmt: 现代C的格式化工具库介绍与酷炫功能 libfmt 是一个开源的C格式化库,提供了高效、安全的文本格式化功能,是C20中引入的std::format的基础实现。它比传统的printf和iostream更安全、更灵活、性能更好。 基本介绍 主要特点 类型安全:…...
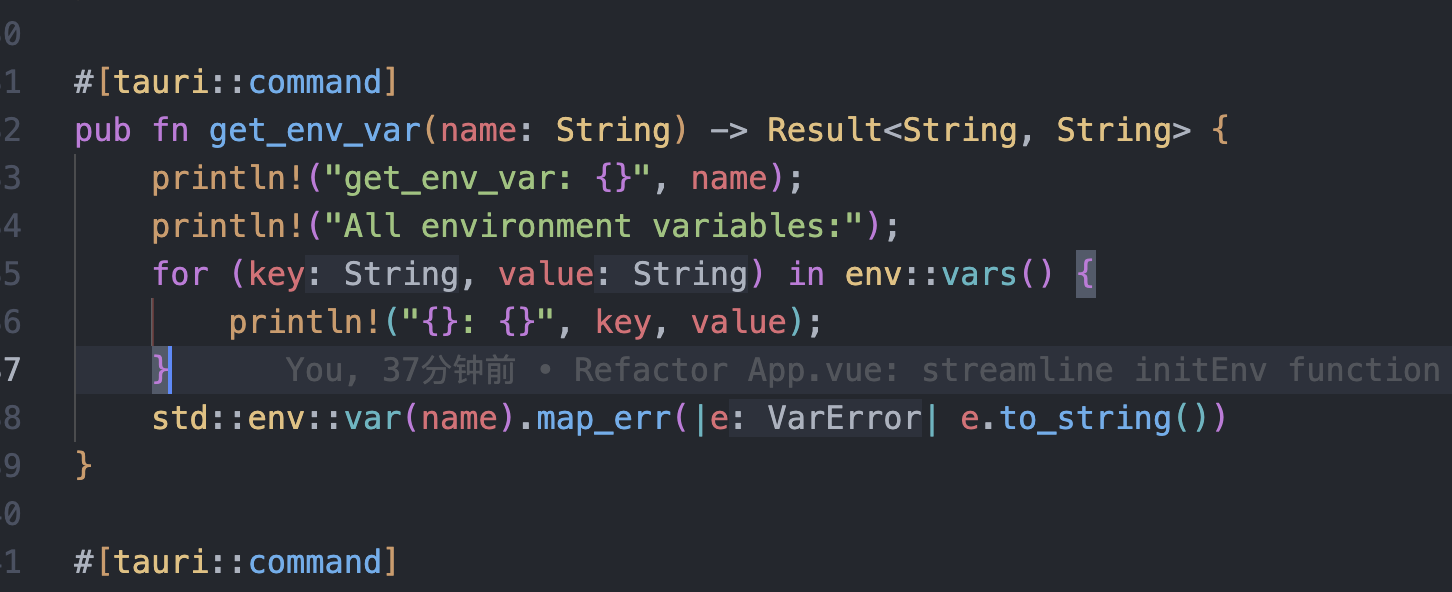
tauri项目,如何在rust端读取电脑环境变量
如果想在前端通过调用来获取环境变量的值,可以通过标准的依赖: std::env::var(name).ok() 想在前端通过调用来获取,可以写一个command函数: #[tauri::command] pub fn get_env_var(name: String) -> Result<String, Stri…...