OpenAI API推出结构化输出功能
每周跟踪AI热点新闻动向和震撼发展 想要探索生成式人工智能的前沿进展吗?订阅我们的简报,深入解析最新的技术突破、实际应用案例和未来的趋势。与全球数同行一同,从行业内部的深度分析和实用指南中受益。不要错过这个机会,成为AI领域的领跑者。点击订阅,与未来同行! 订阅:https://rengongzhineng.io/
去年在DevDay大会上,OpenAI介绍了JSON模式,这个工具对于开发者来说是构建可靠应用程序的有用模块。虽然JSON模式提高了模型生成有效JSON输出的可靠性,但并不能保证模型的响应会符合特定的架构。今天,OpenAI推出了一项新功能——API中的结构化输出,旨在确保模型生成的输出完全符合开发者提供的JSON架构。
从非结构化输入生成结构化数据是现代应用程序中人工智能的核心用例之一。开发者使用OpenAI API构建功能强大的助手,这些助手可以通过函数调用获取数据并回答问题,提取结构化数据进行数据输入,并构建多步代理工作流,使大型语言模型能够采取行动。开发者长期以来一直通过开源工具、提示和反复重试请求来解决大型语言模型在这方面的局限性,确保模型输出符合其系统所需的格式。结构化输出通过约束OpenAI模型以符合开发者提供的架构,并训练模型更好地理解复杂的架构,解决了这一问题。
在对复杂JSON架构遵循性的评估中,OpenAI的新模型gpt-4o-2024-08-06在结构化输出方面得分为100%,而gpt-4-0613得分不到40%。
有了结构化输出,gpt-4o-2024-08-06在评估中实现了100%的可靠性,完美匹配输出架构。
如何使用结构化输出
OpenAI在API中以两种形式引入结构化输出:
- 函数调用:通过工具启用的结构化输出可以在函数定义中设置strict: true来实现。这一功能适用于所有支持工具的模型,包括gpt-4-0613和gpt-3.5-turbo-0613及其后续版本。当启用结构化输出时,模型输出将匹配提供的工具定义。
请求:
POST /v1/chat/completions
{"model": "gpt-4o-2024-08-06","messages": [{"role": "system","content": "You are a helpful assistant. The current date is August 6, 2024. You help users query for the data they are looking for by calling the query function."},{"role": "user","content": "look up all my orders in may of last year that were fulfilled but not delivered on time"}],"tools": [{"type": "function","function": {"name": "query","description": "Execute a query.","strict": true,"parameters": {"type": "object","properties": {"table_name": {"type": "string","enum": ["orders"]},"columns": {"type": "array","items": {"type": "string","enum": ["id","status","expected_delivery_date","delivered_at","shipped_at","ordered_at","canceled_at"]}},"conditions": {"type": "array","items": {"type": "object","properties": {"column": {"type": "string"},"operator": {"type": "string","enum": ["=", ">", "<", ">=", "<=", "!="]},"value": {"anyOf": [{"type": "string"},{"type": "number"},{"type": "object","properties": {"column_name": {"type": "string"}},"required": ["column_name"],"additionalProperties": false}]}},"required": ["column", "operator", "value"],"additionalProperties": false}},"order_by": {"type": "string","enum": ["asc", "desc"]}},"required": ["table_name", "columns", "conditions", "order_by"],"additionalProperties": false}}}]
}
输出:
{"table_name": "orders","columns": ["id", "status", "expected_delivery_date", "delivered_at"],"conditions": [{"column": "status","operator": "=","value": "fulfilled"},{"column": "ordered_at","operator": ">=","value": "2023-05-01"},{"column": "ordered_at","operator": "<","value": "2023-06-01"},{"column": "delivered_at","operator": ">","value": {"column_name": "expected_delivery_date"}}],"order_by": "asc"
}
- 响应格式的新选项:开发者现在可以通过json_schema提供JSON Schema,这是response_format参数的新选项。当模型不调用工具而是以结构化方式响应用户时,这一功能非常有用。这一功能适用于OpenAI最新的GPT-4o模型:gpt-4o-2024-08-06和gpt-4o-mini-2024-07-18。当提供response_format并设置strict: true时,模型输出将匹配提供的架构。
请求:
POST /v1/chat/completions
{"model": "gpt-4o-2024-08-06","messages": [{"role": "system","content": "You are a helpful math tutor."},{"role": "user","content": "solve 8x + 31 = 2"}],"response_format": {"type": "json_schema","json_schema": {"name": "math_response","strict": true,"schema": {"type": "object","properties": {"steps": {"type": "array","items": {"type": "object","properties": {"explanation": {"type": "string"},"output": {"type": "string"}},"required": ["explanation", "output"],"additionalProperties": false}},"final_answer": {"type": "string"}},"required": ["steps", "final_answer"],"additionalProperties": false}}}
}
输出:
{"steps": [{"explanation": "Subtract 31 from both sides to isolate the term with x.","output": "8x + 31 - 31 = 2 - 31"},{"explanation": "This simplifies to 8x = -29.","output": "8x = -29"},{"explanation": "Divide both sides by 8 to solve for x.","output": "x = -29 / 8"}],"final_answer": "x = -29 / 8"
}
安全的结构化输出
安全性是OpenAI的首要任务——新的结构化输出功能将遵循现有的安全政策,并且仍然允许模型拒绝不安全的请求。为了简化开发,API响应中增加了一个新的refusal字符串值,允许开发者以编程方式检测模型是否生成了拒绝,而不是匹配架构的输出。当响应不包含拒绝且模型的响应未被提前中断时(如由finish_reason指示),则模型的响应将可靠地产生匹配提供架构的有效JSON。
原生SDK支持
OpenAI的Python和Node SDK已更新,提供对结构化输出的原生支持。提供工具或响应格式的架构与提供Pydantic或Zod对象一样简单,SDK将处理将数据类型转换为支持的JSON架构,自动将JSON响应反序列化为类型化的数据结构,并在出现拒绝时解析它们。
以下示例显示了使用函数调用的结构化输出的原生支持。
Python:
from enum import Enum
from typing import Union
from pydantic import BaseModel
import openai
from openai import OpenAIclass Table(str, Enum):orders = "orders"customers = "customers"products = "products"class Column(str, Enum):id = "id"status = "status"expected_delivery_date = "expected_delivery_date"delivered_at = "delivered_at"shipped_at = "shipped_at"ordered_at = "ordered_at"canceled_at = "canceled_at"class Operator(str, Enum):eq = "="gt = ">"lt = "<"le = "<="ge = ">="ne = "!="class OrderBy(str, Enum):asc = "asc"desc = "desc"class DynamicValue(BaseModel):column_name: strclass Condition(BaseModel):column: stroperator: Operatorvalue: Union[str, int, DynamicValue]class Query(BaseModel):table_name: Tablecolumns: list[Column]conditions: list[Condition]order_by: OrderByclient = OpenAI()completion = client.beta.chat.completions.parse(model="gpt-4o-2024-08-06",messages=[{"role": "system","content": "You are a helpful assistant. The current date is August 6, 2024. You help users query for the data they are looking for by calling the query function.",},{"role": "user","content": "look up all my orders in may of last year that were fulfilled but not delivered on time",},],tools=[openai.pydantic_function_tool(Query),],
)print(completion.choices[0].message.tool_calls[0].function.parsed_arguments)
Node:
import OpenAI from 'openai';
import z from 'zod';
import { zodFunction } from 'openai/helpers/zod';const Table = z.enum(['orders', 'customers', 'products']);
const Column = z.enum(['id','status','expected_delivery_date','delivered_at','shipped_at','ordered_at','canceled_at',
]);
const Operator = z.enum(['=', '>', '<', '<=', '>=', '!=']);
const OrderBy = z.enum(['asc', 'desc']);const DynamicValue = z.object({column_name: z.string(),
});const Condition = z.object({column: z.string(),operator: Operator,value: z.union([z.string(), z.number(), DynamicValue]),
});const QueryArgs = z.object({table_name: Table,columns: z.array(Column),conditions: z.array(Condition),order_by: OrderBy,
});const client = new OpenAI();const completion = await client.beta.chat.completions.parse({model: 'gpt-4o-2024-08-06',messages: [{ role: 'system', content: 'You are a helpful assistant. The current date is August 6, 2024. You help users query for the data they are looking for by calling the query function.' },{ role: 'user', content: 'look up all my orders in may of last year that were fulfilled but not delivered on time' }],tools: [zodFunction({ name: 'query', parameters: QueryArgs })],
});
console.log(completion.choices[0].message.tool_calls[0].function.parsed_arguments);
响应格式的原生支持也可用。
Python:
from pydantic import BaseModel
from openai import OpenAIclass Step(BaseModel):explanation: stroutput: strclass MathResponse(BaseModel):steps: list[Step]final_answer: strclient = OpenAI()completion = client.beta.chat.completions.parse(model="gpt-4o-2024-08-06",messages=[{"role": "system", "content": "You are a helpful math tutor."},{"role": "user", "content": "solve 8x + 31 = 2"},],response_format=MathResponse,
)message = completion.choices[0].message
if message.parsed:print(message.parsed.steps)print(message.parsed.final_answer)
else:print(message.refusal)
Node:
import OpenAI from 'openai';
import { zodResponseFormat } from 'openai/helpers/zod';
import { z } from 'zod';const Step = z.object({explanation: z.string(),output: z.string(),
});const MathResponse = z.object({steps: z.array(Step),final_answer: z.string(),
});const client = new OpenAI();const completion = await client.beta.chat.completions.parse({model: 'gpt-4o-2024-08-06',messages: [{"role": "system","content": "You are a helpful math tutor. Only use the schema for math responses.",},{ "role": "user", "content": "solve 8x + 3 = 21" },],response_format: zodResponseFormat(MathResponse, 'mathResponse'),
});const message = completion.choices[0]?.message;
if (message?.parsed) {console.log(message.parsed.steps);console.log(message.parsed.final_answer);
} else {console.log(message.refusal);
}
其他使用案例
开发者经常使用OpenAI的模型生成结构化数据以满足各种使用需求。以下是一些额外的示例:
根据用户意图动态生成用户界面
例如,开发者可以使用结构化输出创建代码或用户界面生成应用程序。以下所有示例都使用相同的response_format,可以根据用户输入生成不同的用户界面。
系统:
{"type": "div","label": "","children": [{"type": "header","label": "","children": [{"type": "div","label": "Green Thumb Gardening","children": [],"attributes": [{ "name": "className", "value": "site-title" }]},{"type": "div","label": "Bringing Life to Your Garden","children": [],"attributes": [{ "name": "className", "value": "site-tagline" }]}],"attributes": [{ "name": "className", "value": "header" }]},{"type": "section","label": "","children": [{"type": "div","label": "","children": [{"type": "div","label": "About Us","children": [{"type": "div","label": "At Green Thumb Gardening, we specialize in transforming your outdoor spaces into beautiful, thriving gardens. Our team has decades of experience in horticulture and landscape design.","children": [],"attributes": [{ "name": "className", "value": "about-description" }]}],"attributes": [{ "name": "className", "value": "about-section" }]}],"attributes": [{ "name": "className", "value": "content" }]}],"attributes": [{ "name": "className", "value": "about-container" }]},{"type": "section","label": "","children": [{"type": "div","label": "","children": [{"type": "div","label": "Our Services","children": [{"type": "div","label": "Garden Design","children": [],"attributes": [{ "name": "className", "value": "service-item" }]},{"type": "div","label": "Plant Care & Maintenance","children": [],"attributes": [{ "name": "className", "value": "service-item" }]},{"type": "div","label": "Seasonal Cleanup","children": [],"attributes": [{ "name": "className", "value": "service-item" }]},{"type": "div","label": "Custom Landscaping","children": [],"attributes": [{ "name": "className", "value": "service-item" }]}],"attributes": [{ "name": "className", "value": "services-list" }]}],"attributes": [{ "name": "className", "value": "content" }]}],"attributes": [{ "name": "className", "value": "services-container" }]}],"attributes": [{ "name": "className", "value": "landing-page" }]
}
将最终答案与支持推理或额外评论分开
给模型一个单独的思维链字段可以提高最终响应的质量。
请求:
{"model": "gpt-4o-2024-08-06","messages": [{"role": "system","content": "You are a helpful assistant"},{"role": "user","content": "9.11和9.9哪个更大?"}],"response_format": {"type": "json_schema","json_schema": {"name": "reasoning_schema","strict":true,"schema": {"type": "object","properties": {"reasoning_steps": {"type": "array","items": {"type": "string"},"description": "推理步骤,得出最终结论。"},"answer": {"type": "string","description": "最终答案,考虑推理步骤。"}},"required": ["reasoning_steps", "answer"],"additionalProperties": false}}}
}
输出:
{"reasoning_steps": ["第一步是比较数字9.11和9.9。","这两个数字都有相同的整数部分,即9。","要比较小数部分,请将它们转换为相同的小数位数。","9.11有两位小数:它是9.11。","9.9有一位小数:可以写成9.90。","现在,通过查看小数部分来比较9.11和9.90。","比较11和90。","90大于11,所以9.90大于9.11。"],"answer": "9.9大于9.11。"
}
从非结构化数据中提取结构化数据
例如,指示模型从会议记录中提取待办事项、截止日期和负责人。
请求:
POST /v1/chat/completions
{"model": "gpt-4o-2024-08-06","messages": [{"role": "system","content": "从会议记录中提取行动项、截止日期和负责人。"},{"role": "user","content": "...会议记录在此..."}],"response_format": {"type": "json_schema","json_schema": {"name": "action_items","strict": true,"schema": {"type": "object","properties": {"action_items": {"type": "array","items": {"type": "object","properties": {"description": {"type": "string","description": "行动项描述。"},"due_date": {"type": ["string", "null"],"description": "行动项的截止日期,如果没有指定可以为null。"},"owner": {"type": ["string", "null"],"description": "负责行动项的负责人,如果没有指定可以为null。"}},"required": ["description", "due_date", "owner"],"additionalProperties": false},"description": "会议中的行动项列表。"}},"required": ["action_items"],"additionalProperties": false}}}
}
输出:
{"action_items": [{"description": "协作优化路径规划算法","due_date": "2024-06-30","owner": "Jason Li"},{"description": "联系行业合作伙伴获取更多数据集","due_date": "2024-06-25","owner": "Aisha Patel"},{"description": "探索替代的LIDAR传感器配置并报告结果","due_date": "2024-06-27","owner": "Kevin Nguyen"},{"description": "安排集成导航系统的扩展压力测试","due_date": "2024-06-28","owner": "Emily Chen"},{"description": "修复错误后重新测试系统并更新团队","due_date": "2024-07-01","owner": "David Park"}]
}
工作原理
OpenAI采用了两步方法来提高与JSON Schema匹配的模型输出的可靠性。首先,训练了最新的模型gpt-4o-2024-08-06,以理解复杂的架构并最佳地生成匹配它们的输出。然而,模型行为本质上是非确定性的——尽管这一模型的性能有所提高(在基准测试中得分为93%),但它仍未达到开发者构建强大应用程序所需的可靠性。因此,OpenAI还采用了一种确定性、工程性的方法来约束模型的输出,以实现100%的可靠性。
限制与注意事项
使用结构化输出时需要注意以下几点:
- 结构化输出仅允许JSON Schema的一个子集,详细信息请参见文档。这有助于确保最佳性能。
- 第一次API响应使用新架构时会有额外的延迟,但随后的响应将快速且无延迟惩罚。因为在第一次请求期间,OpenAI需要处理架构并生成可高效重用的缓存结构。
- 如果模型选择拒绝不安全的请求,它可能会无法遵循架构。如果选择拒绝,返回消息将包含refusal布尔值以指示这一点。
- 如果生成达到max_tokens或其他停止条件之前,模型可能无法遵循架构。
- 结构化输出并不能防止所有类型的模型错误。例如,模型仍可能在JSON对象的值中犯错(例如,在数学方程中出错)。如果开发者发现错误,建议在系统指令中提供示例或将任务拆分为更简单的子任务。
- 结构化输出与并行函数调用不兼容。生成并行函数调用时,可能不会匹配提供的架构。设置parallel_tool_calls: false以禁用并行函数调用。
- 提供的JSON架构不符合零数据保留(ZDR)资格。
可用性
结构化输出现已在API中普遍可用。
通过函数调用实现的结构化输出适用于所有支持函数调用的API模型。这包括OpenAI最新的模型(gpt-4o,gpt-4o-mini),所有gpt-4-0613及以后和gpt-3.5-turbo-0613及其后续版本,以及任何支持函数调用的微调模型。此功能适用于聊天补全API、助手API和批量API。通过函数调用实现的结构化输出也兼容视觉输入。
通过响应格式实现的结构化输出适用于gpt-4o-mini和gpt-4o-2024-08-06及基于这些模型的任何微调模型。此功能适用于聊天补全API、助手API和批量API。通过响应格式实现的结构化输出也兼容视觉输入。
通过切换到新的gpt-4o-2024-08-06,开发者在输入方面节省50%($2.50/百万输入tokens),在输出方面节省33%($10.00/百万输出tokens),相较于gpt-4o-2024-05-13。
要开始使用结构化输出,请查看OpenAI的文档。(https://platform.openai.com/docs/guides/structured-outputs)
相关文章:
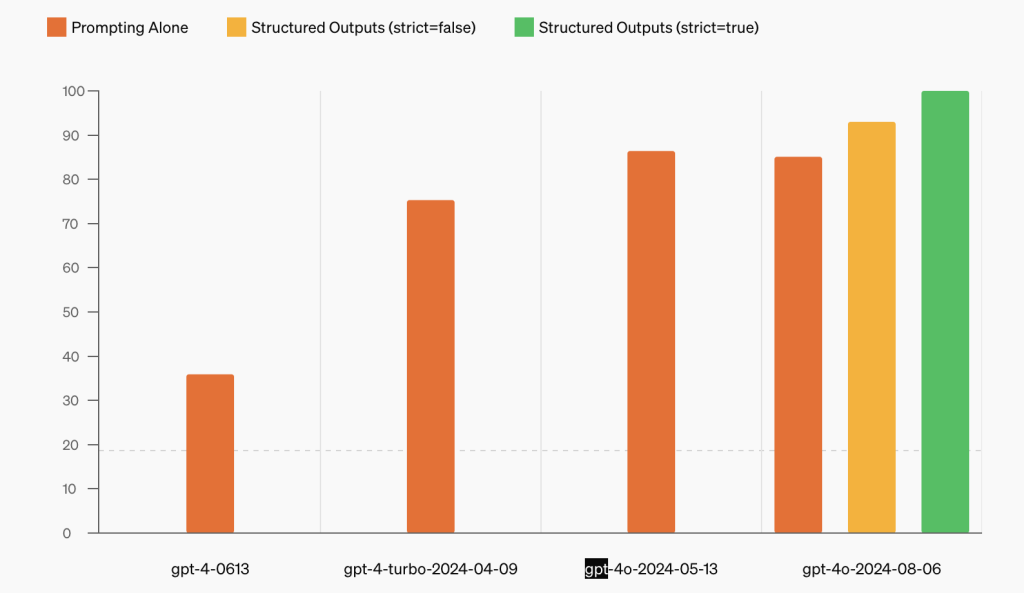
OpenAI API推出结构化输出功能
每周跟踪AI热点新闻动向和震撼发展 想要探索生成式人工智能的前沿进展吗?订阅我们的简报,深入解析最新的技术突破、实际应用案例和未来的趋势。与全球数同行一同,从行业内部的深度分析和实用指南中受益。不要错过这个机会,成为AI领…...
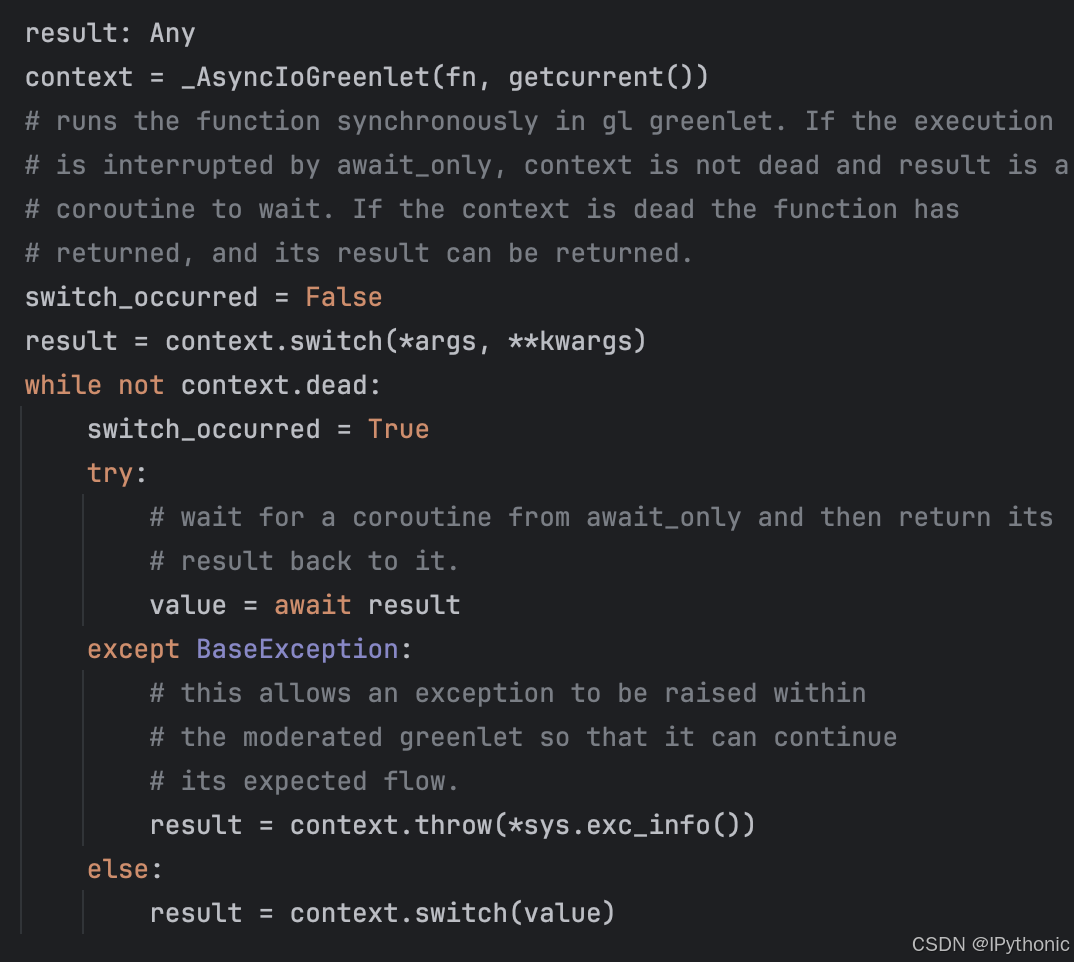
Python 异步编程:Sqlalchemy 异步实现方式
SQLAlchemy 是 Python 中最流行的数据库工具之一,在新版本中引入了对异步操作的支持。这为使用异步框架(如 FastAPI)开发应用程序带来了极大的便利。在这篇文章中,简单介绍下 SQLAlchemy 是如何利用 Greenlet 实现异步操作的。 什…...
父类引用指向子类对象
在 Java 中,父类引用可以指向子类对象,这是多态的一种表现。这种特性允许你使用父类的引用来操作子类对象,从而实现更灵活和可扩展的代码设计。 基本概念 多态:父类引用可以指向子类对象。这使得你可以用统一的接口处理不同的对象…...
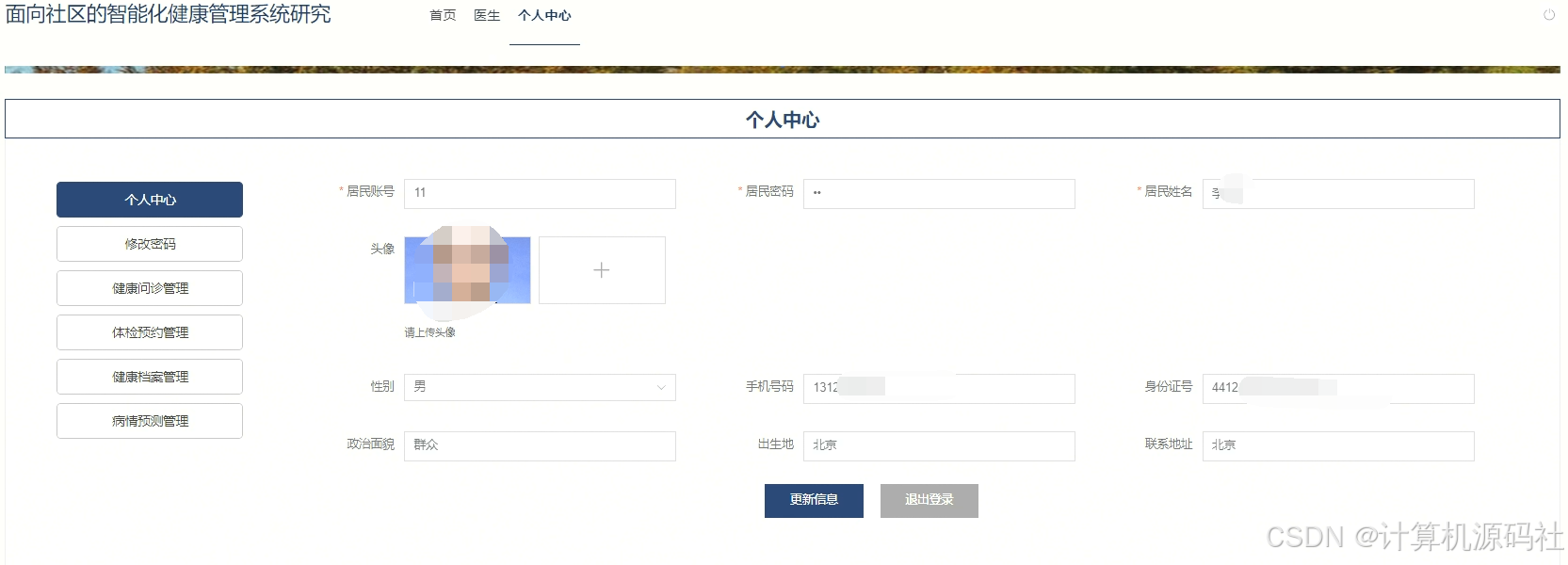
分享一个基于Spring Boot的面向社区的智能化健康管理系统的设计与实现(源码、调试、LW、开题、PPT)
💕💕作者:计算机源码社 💕💕个人简介:本人 八年开发经验,擅长Java、Python、PHP、.NET、Node.js、Android、微信小程序、爬虫、大数据、机器学习等,大家有这一块的问题可以一起交流&…...
【扒代码】reduction参数是什么
model DensityMapRegressor(in_channels256, reduction8)reduction 参数在 DensityMapRegressor 类中用于决定模型在上采样过程中的层级配置。具体来说,它决定了上采样过程中使用多少个 UpsamplingLayer,从而影响输出的分辨率。 reduction 参数的作用 …...
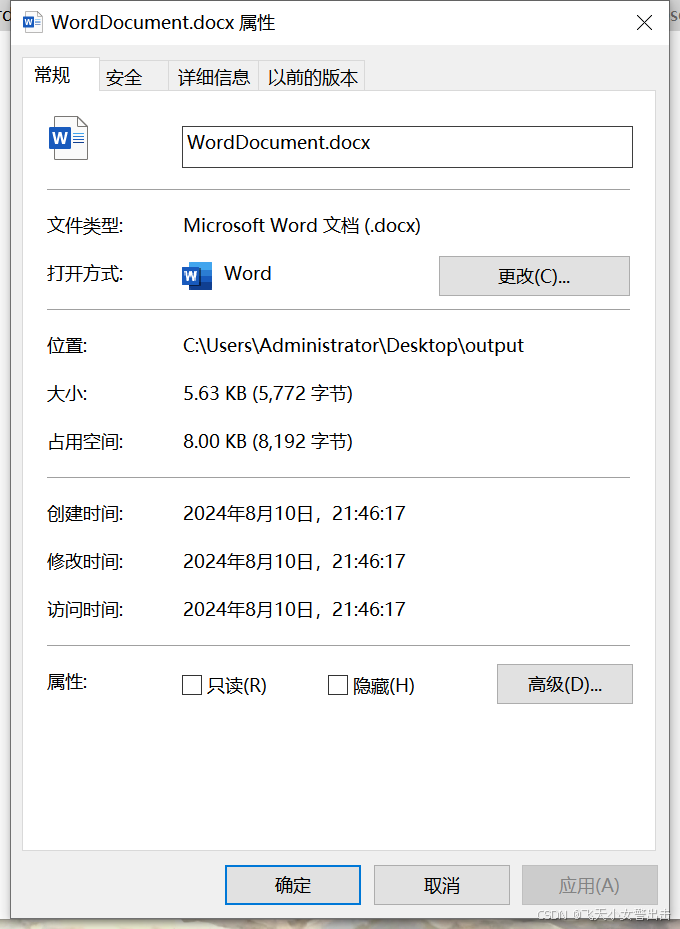
Python,Spire.Doc模块,处理word、docx文件,极致丝滑
Python处理word文件,一般都是推荐的Python-docx,但是只写出一个,一句话的文件,也没有什么样式,就是36K。 再打开word在另存一下,就可以到7-8k,我想一定是python-docx的问题,但一直没…...
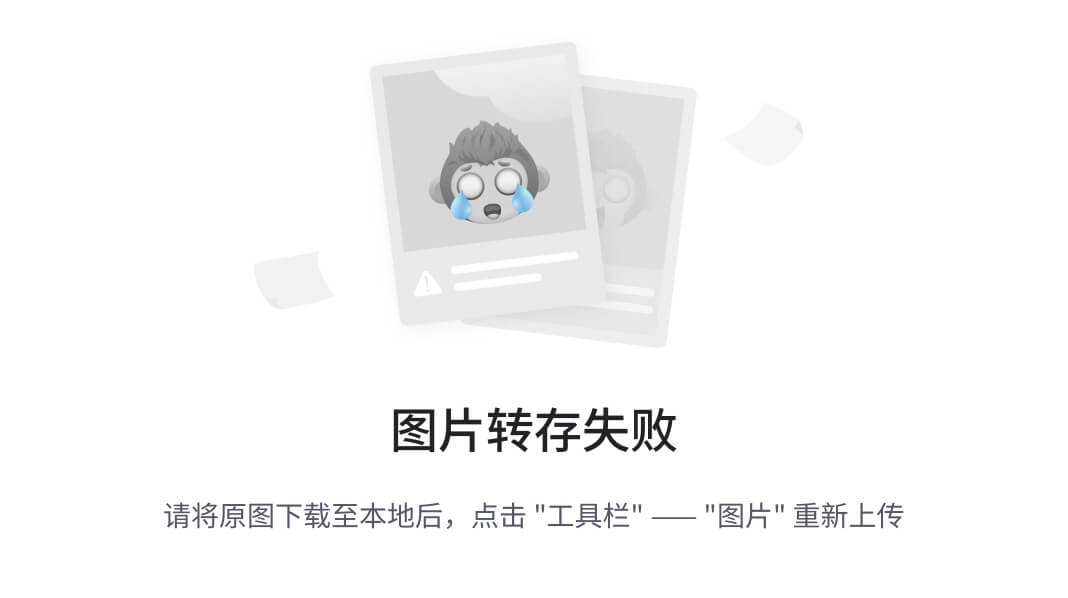
redis的安装与命令
一、redis与memcache总体对比 1.性能 Redis:只使用单核,平均每一个核上Redis在存储小数据时比Memcached性能更高。 Memcached:可以使用多核,而在100k以上的数据中,Memcached性能要高于Redis。 2.内存使用效率 Mem…...
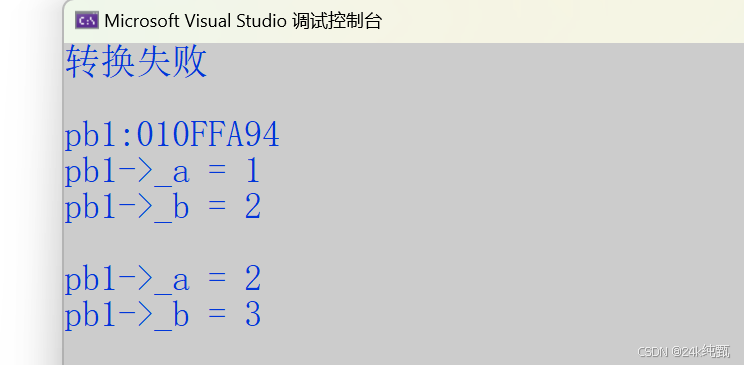
【C++】特殊类设计类型转换
目录 💡前言一,特殊类设计1. 请设计一个类,不能被拷贝2. 请设计一个类,只能在堆上创建对象3. 请设计一个类,只能在栈上创建对象4. 请设计一个类,不能被继承5. 请设计一个类,只能创建一个对象(单…...
为git 命令行 设置代理环境变量
http://t.csdnimg.cn/cAxkg 国内需要修改pinoko根目录下gitconfig文件,添加 [http]proxy http://127.0.0.1:1080 [https]proxy https://127.0.0.1:1080或者通过命令行配置: git config --global http.proxy http://127.0.0.1:1080 git config --glo…...
自定义linux某些常见配置
1.当前路径 echo "PS1\u\h:\w\$ " >> /etc/profile source /etc/profile 2.ssh使能 1.开启openssh 2.权限赋予chown root.root /var/empty/ 3.开发板作为server echo "PermitRootLogin yes" >> /etc/ssh/sshd_config 3开机自启动脚本 1.init…...
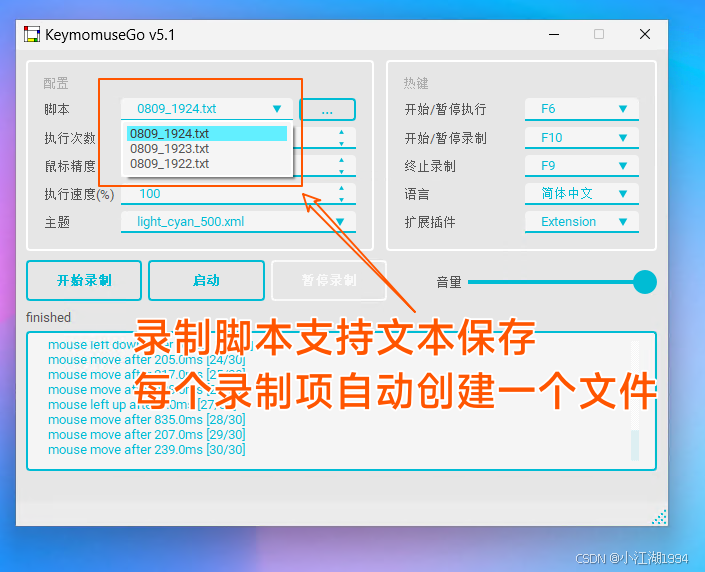
告别手动操作!KeyMouseGo实现自动化工作流
前言 在这个快节奏的时代,我们每天都在与电脑打交道,重复着那些繁琐而单调的操作;你是否曾想过,能让电脑自己完成这些任务,而你则悠闲地喝着咖啡,享受着生活?今天,就让我们一起揭开一…...
大型语言模型微调 新进展-4篇 论文
1. Brevity is the soul of wit: Pruning long files for code generation 发布时间:2024-06-29链接:https://arxiv.org/abs/2407.00434机构:伦敦大学学院 (UCL) 本研究针对大型语言模型的代码生成任务中的数据清理问题进行了探索。研究发现…...
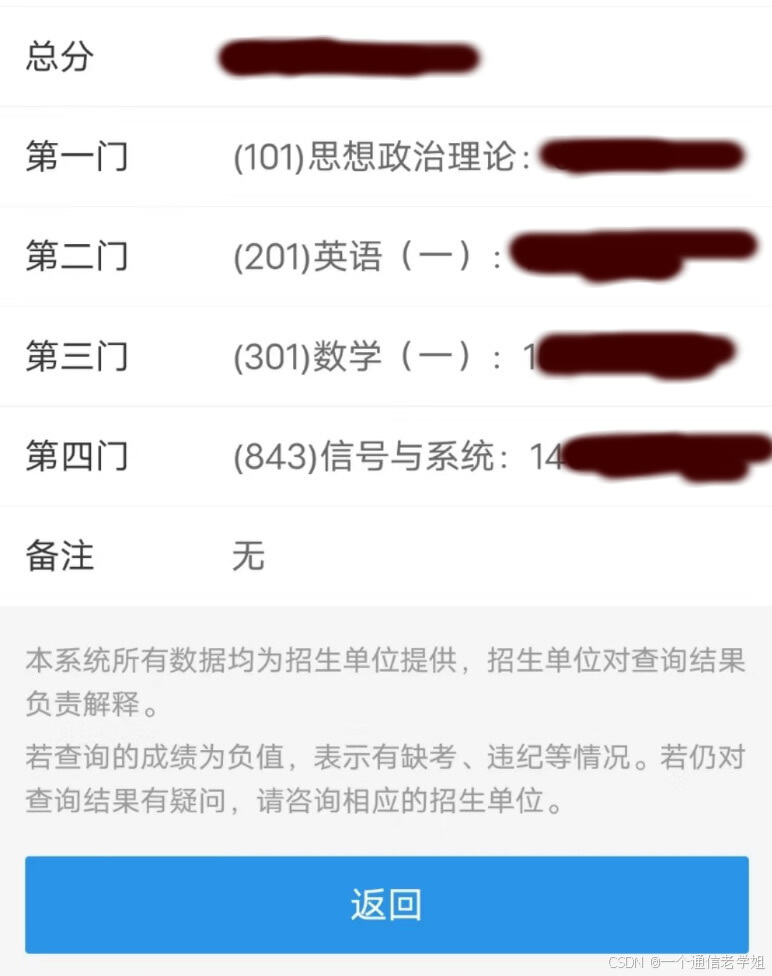
专业课140+杭电杭州电子科技大学843信号与系统考研经验电子信息与通信工程真题,大纲,参考书。
顺利上岸杭电,由于专业课考的不错140,群里不少同学希望分享一点经验,回头看看这一年考研复习,确实有得有失,总结一下自己的专业课复习经验,希望对大家有帮助,基础课考的没有专业好,而…...
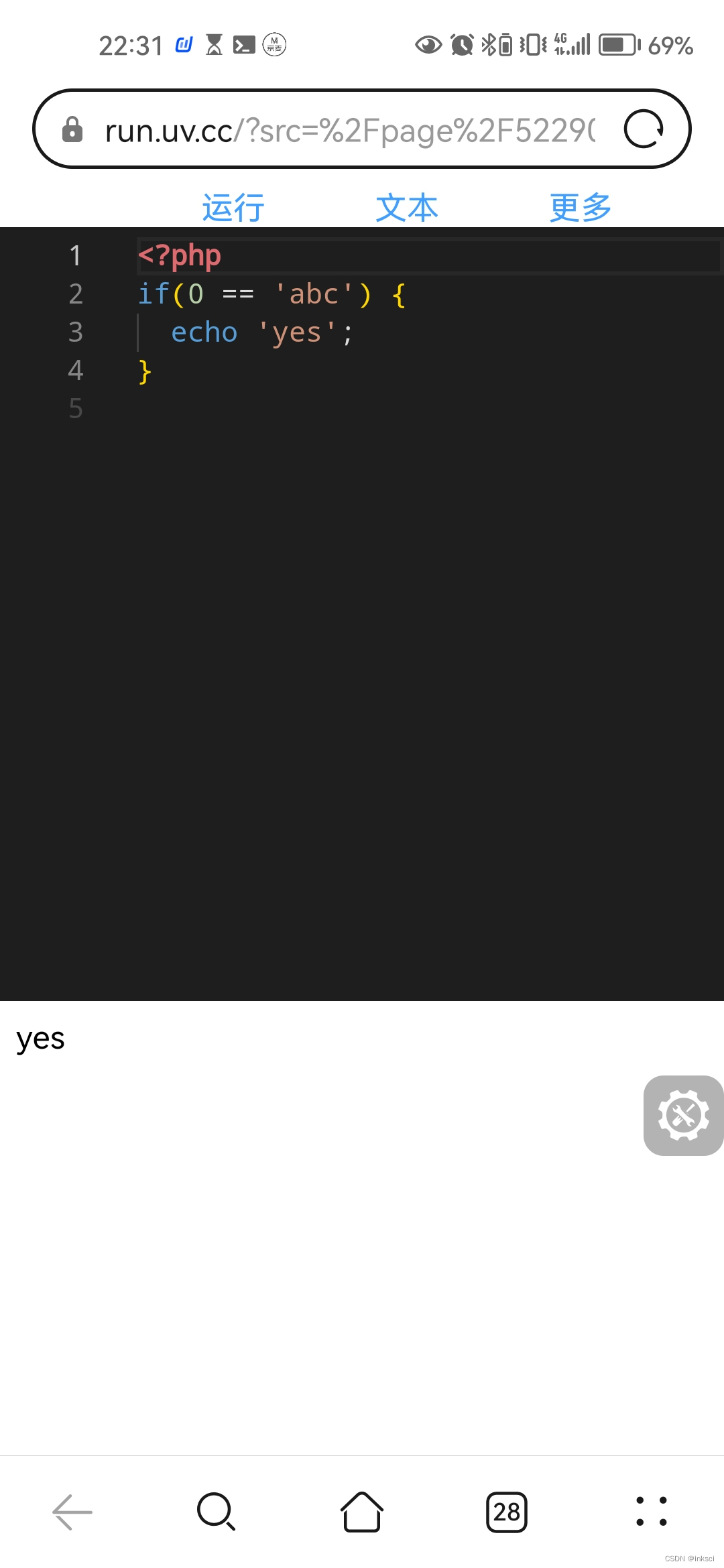
php 中 (0 == ‘abc‘) 为真
https://andi.cn/page/621653.html...
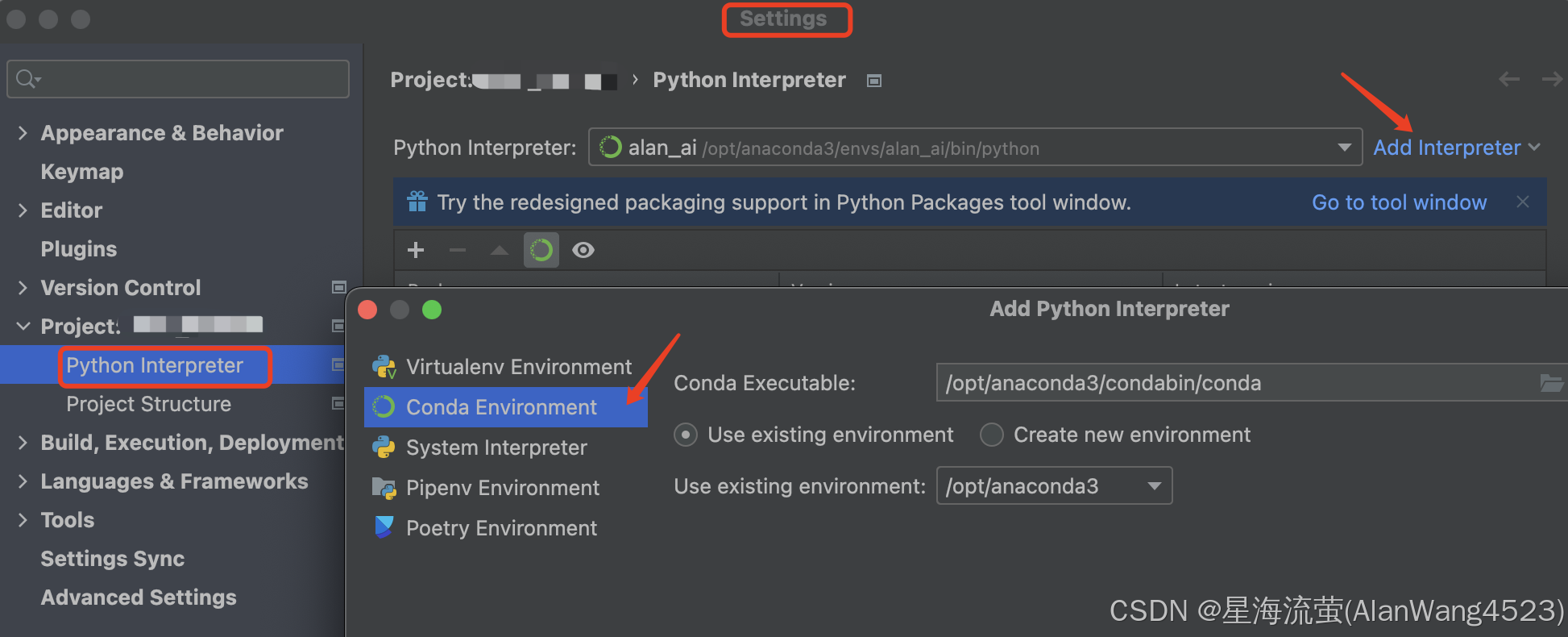
MacOS Anaconda 安装教程及虚拟环境创建
一、下载 Anaconda 1、Anaconda 官网 2、清华大学开源软件镜像站 点 Date 按时间排序,根据自己 Mac 芯片类型下载对应最新版本的。 Intel 芯片的下载 x86_64 版本的Apple m1 芯片的下载 arm64 版本的 二、安装 Anaconda 将安装包下载到本地后,双击安…...
Mac快速配置ADB环境变量
ADB是进行 Androd 开发时很常用的调试工具,Android SDK 中就包含了该工具,所以如果安装了SDK那只需要在环境变量中配置 Android SDK 的路径即可,本文的环境配置也基于这种场景。 如果需要独立下载 ADB 工具,请参考下面网址&#x…...
Kylin的工作原理及使用分享
前言 在当今信息爆炸的时代,企业和研究机构每天都在生成和收集大量的数据。这些数据中蕴藏着巨大的商业价值和研究潜力,但要从中提取出有用的信息却并非易事。传统的数据处理和分析技术在面对如此庞大的数据量时,往往难以提供快速和有效的响…...
python 使用seleniumwire获取响应数据
seleniumwire 是一个在 Selenium WebDriver 基础上扩展的库,它允许你在使用 Selenium 进行网页自动化测试或爬虫时捕获和修改 HTTP 请求和响应。这对于需要分析网页数据或进行更复杂的网络交互的自动化任务特别有用。 以下是如何使用 seleniumwire 来获取响应数据的…...
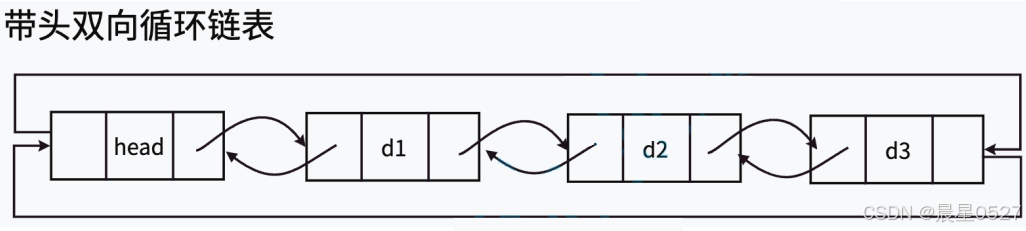
用C语言实现双向链表
目录 一.双向链表的结构 二. 双向链表的实现 1. 在List.h中结构体的定义和各函数的声明 1.1 结构体(节点)的定义 1.2 各函数的声明 2. 在List.c中各函数的实现 2.1 初始化 LTInit 2.2 尾插 LTPushBack 2.3 打印 LTPrint 2.4 头插 LTPushFron…...
Github 2024-08-10 Rust开源项目日报Top10
根据Github Trendings的统计,今日(2024-08-10统计)共有10个项目上榜。根据开发语言中项目的数量,汇总情况如下: 开发语言项目数量Rust项目10Python项目1Turbo:下一代前端开发工具链 创建周期:977 天开发语言:Rust协议类型:MIT LicenseStar数量:25308 个Fork数量:1713 …...
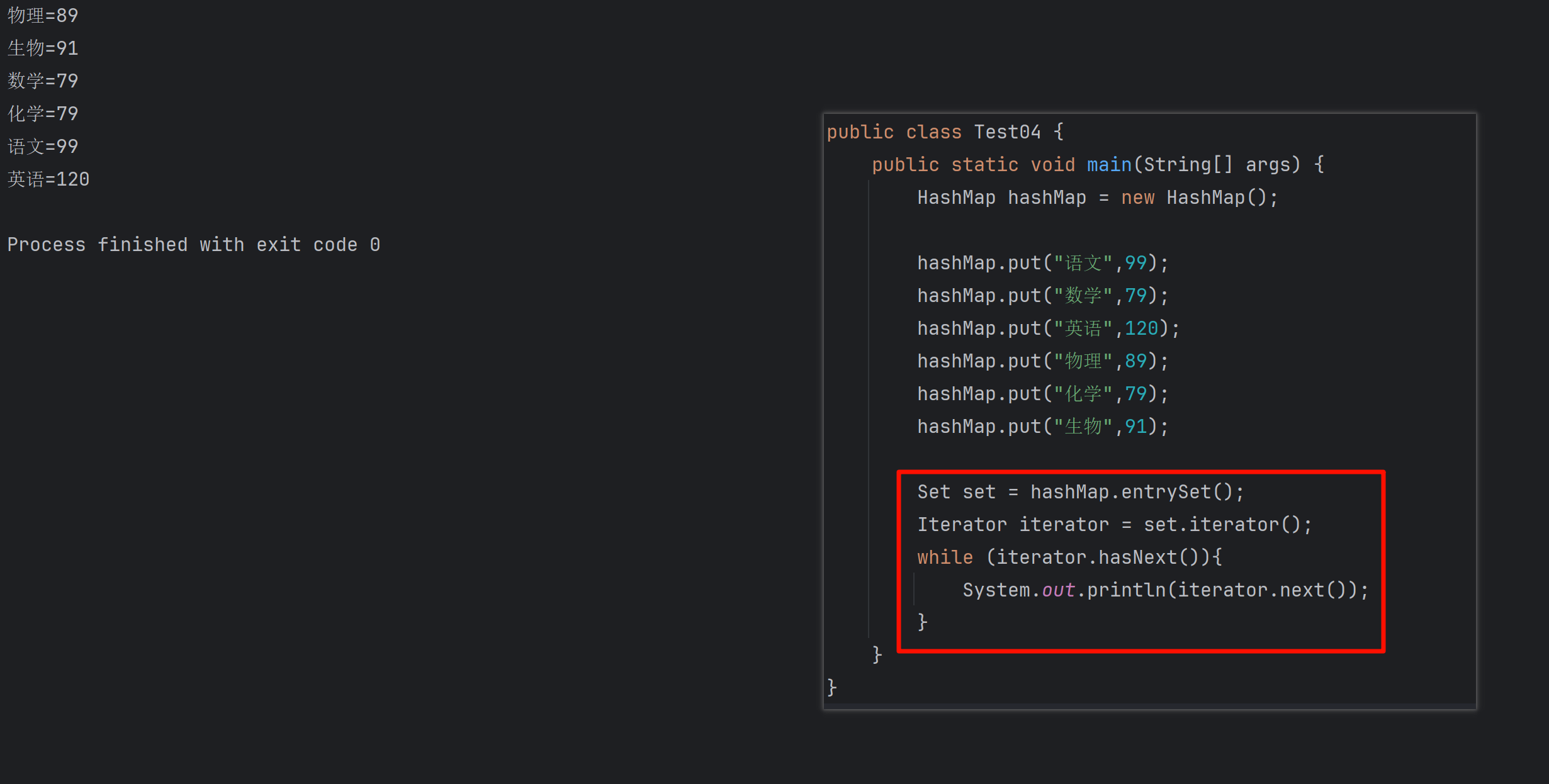
遍历 Map 类型集合的方法汇总
1 方法一 先用方法 keySet() 获取集合中的所有键。再通过 gey(key) 方法用对应键获取值 import java.util.HashMap; import java.util.Set;public class Test {public static void main(String[] args) {HashMap hashMap new HashMap();hashMap.put("语文",99);has…...
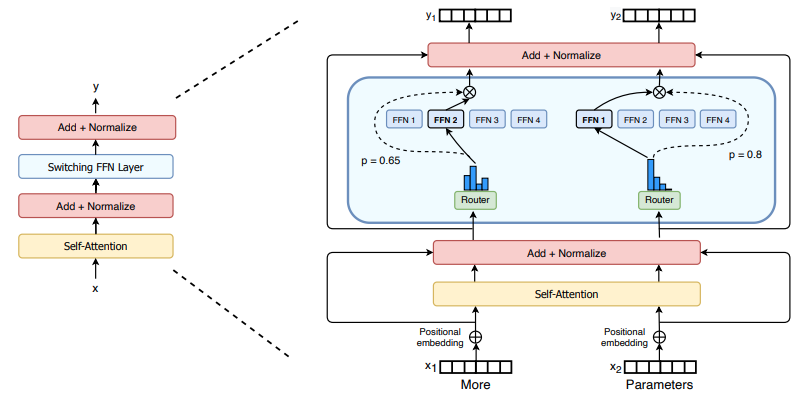
(二)TensorRT-LLM | 模型导出(v0.20.0rc3)
0. 概述 上一节 对安装和使用有个基本介绍。根据这个 issue 的描述,后续 TensorRT-LLM 团队可能更专注于更新和维护 pytorch backend。但 tensorrt backend 作为先前一直开发的工作,其中包含了大量可以学习的地方。本文主要看看它导出模型的部分&#x…...
电脑插入多块移动硬盘后经常出现卡顿和蓝屏
当电脑在插入多块移动硬盘后频繁出现卡顿和蓝屏问题时,可能涉及硬件资源冲突、驱动兼容性、供电不足或系统设置等多方面原因。以下是逐步排查和解决方案: 1. 检查电源供电问题 问题原因:多块移动硬盘同时运行可能导致USB接口供电不足&#x…...
vue3 字体颜色设置的多种方式
在Vue 3中设置字体颜色可以通过多种方式实现,这取决于你是想在组件内部直接设置,还是在CSS/SCSS/LESS等样式文件中定义。以下是几种常见的方法: 1. 内联样式 你可以直接在模板中使用style绑定来设置字体颜色。 <template><div :s…...
Axios请求超时重发机制
Axios 超时重新请求实现方案 在 Axios 中实现超时重新请求可以通过以下几种方式: 1. 使用拦截器实现自动重试 import axios from axios;// 创建axios实例 const instance axios.create();// 设置超时时间 instance.defaults.timeout 5000;// 最大重试次数 cons…...
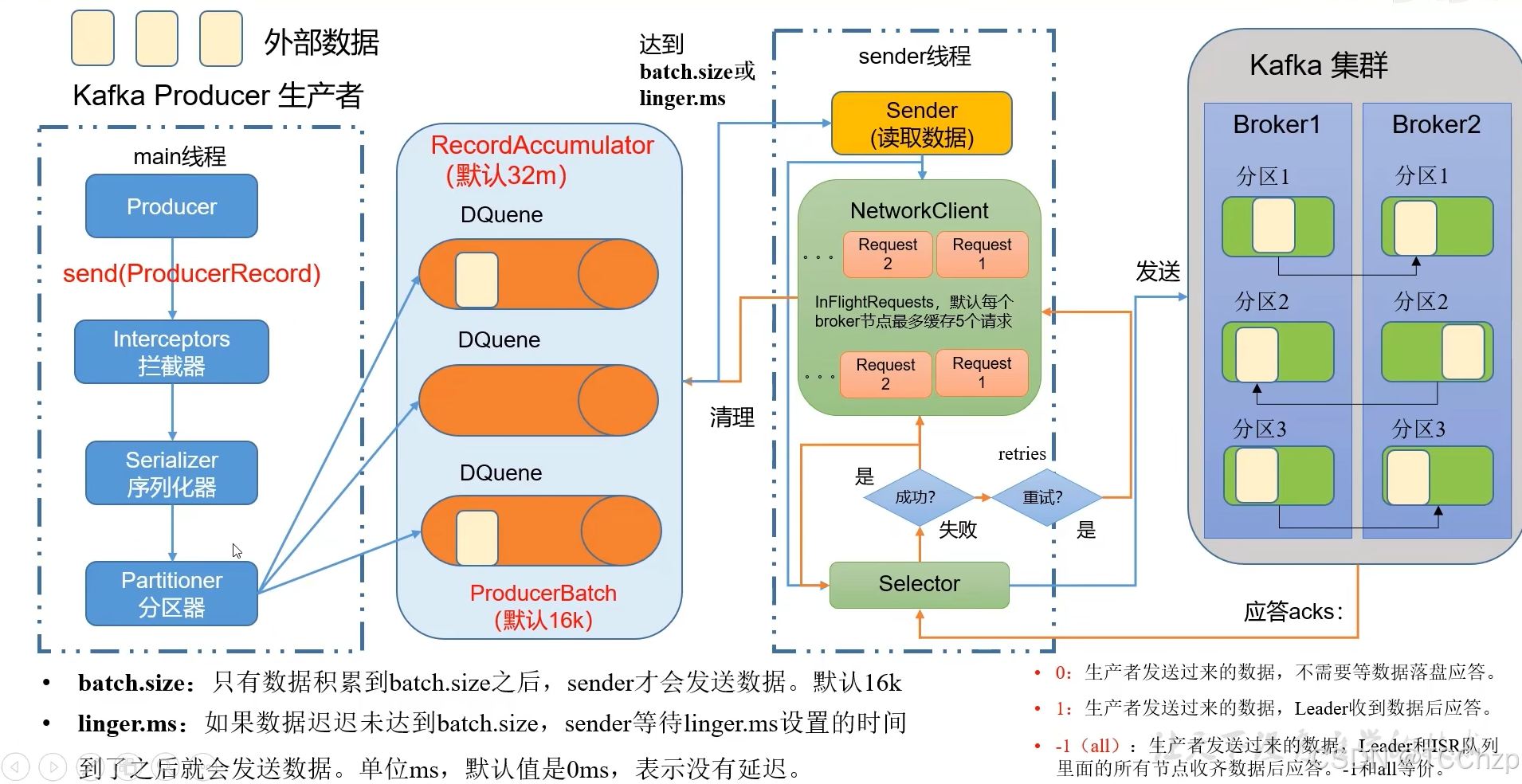
Kafka入门-生产者
生产者 生产者发送流程: 延迟时间为0ms时,也就意味着每当有数据就会直接发送 异步发送API 异步发送和同步发送的不同在于:异步发送不需要等待结果,同步发送必须等待结果才能进行下一步发送。 普通异步发送 首先导入所需的k…...
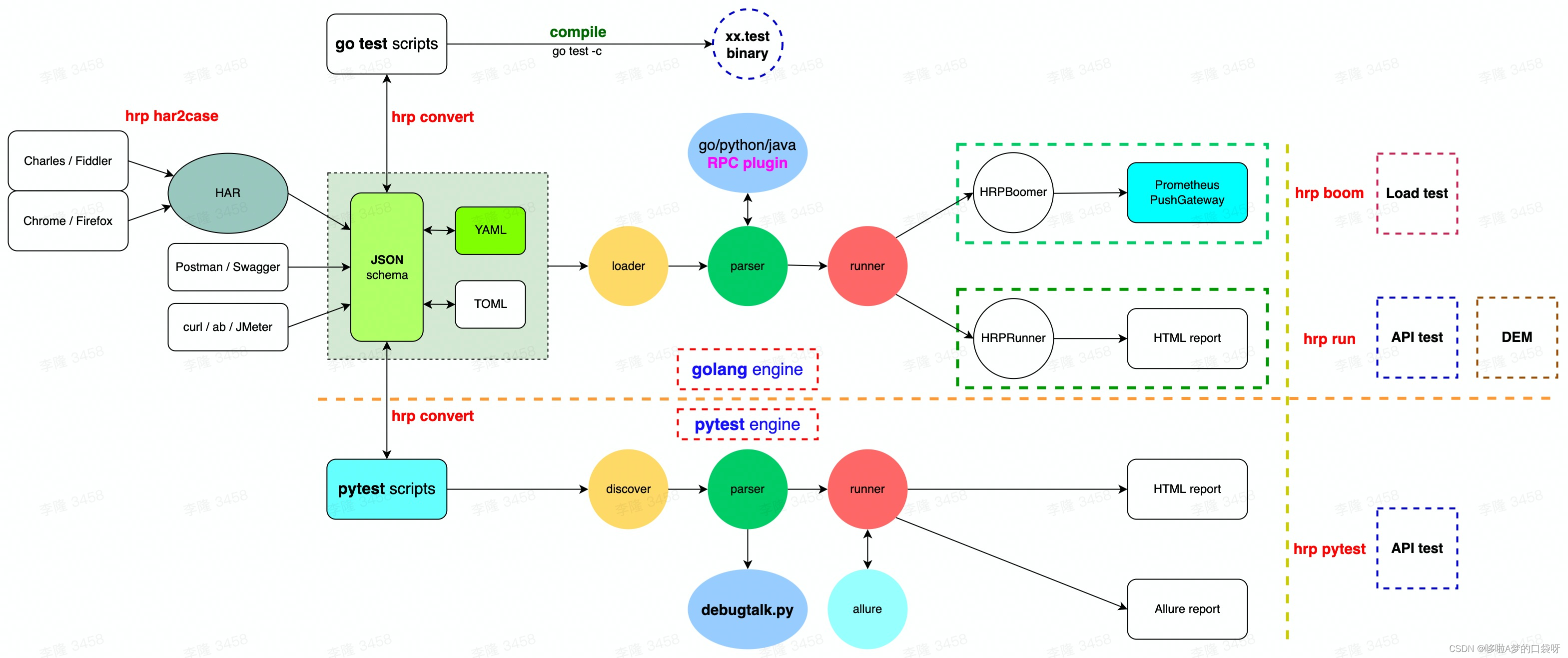
接口自动化测试:HttpRunner基础
相关文档 HttpRunner V3.x中文文档 HttpRunner 用户指南 使用HttpRunner 3.x实现接口自动化测试 HttpRunner介绍 HttpRunner 是一个开源的 API 测试工具,支持 HTTP(S)/HTTP2/WebSocket/RPC 等网络协议,涵盖接口测试、性能测试、数字体验监测等测试类型…...
提升移动端网页调试效率:WebDebugX 与常见工具组合实践
在日常移动端开发中,网页调试始终是一个高频但又极具挑战的环节。尤其在面对 iOS 与 Android 的混合技术栈、各种设备差异化行为时,开发者迫切需要一套高效、可靠且跨平台的调试方案。过去,我们或多或少使用过 Chrome DevTools、Remote Debug…...
离线语音识别方案分析
随着人工智能技术的不断发展,语音识别技术也得到了广泛的应用,从智能家居到车载系统,语音识别正在改变我们与设备的交互方式。尤其是离线语音识别,由于其在没有网络连接的情况下仍然能提供稳定、准确的语音处理能力,广…...
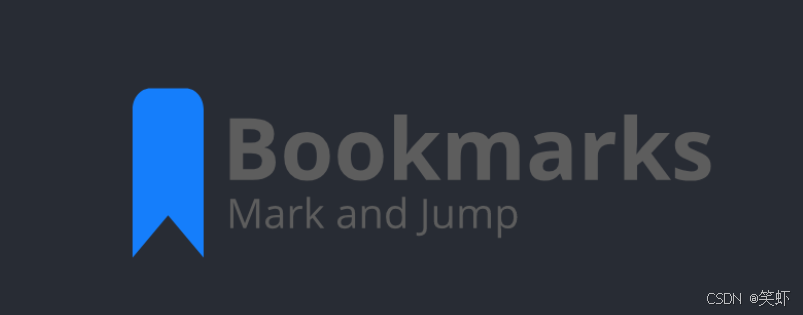
Visual Studio Code 扩展
Visual Studio Code 扩展 change-case 大小写转换EmmyLua for VSCode 调试插件Bookmarks 书签 change-case 大小写转换 https://marketplace.visualstudio.com/items?itemNamewmaurer.change-case 选中单词后,命令 changeCase.commands 可预览转换效果 EmmyLua…...