在亚马逊云科技上部署开源大模型并利用RAG和LangChain开发生成式AI应用
项目简介:
小李哥将继续每天介绍一个基于亚马逊云科技AWS云计算平台的全球前沿AI技术解决方案,帮助大家快速了解国际上最热门的云计算平台亚马逊云科技AWS AI最佳实践,并应用到自己的日常工作里。
本次介绍的是如何在亚马逊云科技上利用SageMaker机器学习服务部署开源大模型,使用LangChain框架调用大模型生成回复,并利用RAG技术增强内容生成,再通过Streamlit框架开发用户界面回答用于关于用户关于特定文档的问题。本架构设计全部采用了云原生Serverless架构,提供可扩展和安全的AI解决方案。本方案的解决方案架构图如下:
方案所需基础知识
什么是 Amazon SageMaker?
Amazon SageMaker 是亚马逊云科技提供的一站式机器学习服务,旨在帮助开发者和数据科学家轻松构建、训练和部署机器学习模型。SageMaker 提供了从数据准备、模型训练到模型部署的全流程工具,使用户能够高效地在云端实现机器学习项目。
为什么利用 LangChain 框架调用大模型 API 生成回复?
LangChain 是一个强大的框架,旨在简化调用大型语言模型(如 GPT-3、Amazon Bedrock 上的模型等)API 的过程。通过 LangChain,开发者可以轻松地构建和管理复杂的对话逻辑,将多个 API 调用和自然语言处理(NLP)任务无缝集成到一起,从而实现智能、上下文丰富的对话体验。
利用 RAG 开发AI问答服务的好处
RAG(检索增强生成,Retrieval-Augmented Generation)是一种结合文档检索与生成式 AI 模型的技术,用于构建强大的问答系统。通过将文档检索与生成模型结合,RAG 可以在提供实时、准确的回答时,确保回复的内容基于最新的文档信息。
提高回答准确性:
RAG 结合检索和生成模型,使系统能够从大量文档中提取相关信息,并生成符合上下文的准确答案,确保回答内容的可靠性和权威性。
支持实时更新:
RAG 系统可以动态检索和生成答案,确保用户获得基于最新信息的回复,特别适用于内容频繁更新的领域。
增强用户体验:
通过使用 LangChain 调用大模型 API 和 RAG 技术,问答服务不仅能提供流畅的自然语言交互,还能回答复杂、多样的问题,显著提升用户体验。
降低开发复杂性:
LangChain 框架简化了与大模型的集成,RAG 技术则优化了信息检索和答案生成过程,二者结合有效降低了开发智能问答系统的复杂性。
本方案包括的内容
1. 利用Amazon SageMaker部署一个Flan-T5大语言模型
2. 通过LangChain框架调用大语言模型
3. 在SageMaker Studio上利用RAG技术开发一个基于文档内容回答问题的GenAI应用
4. 开发和部署Streamlit框架开发的网页应用
项目搭建具体步骤:
1. 登录亚马逊云科技控制台,进入Studio功能,点击Open Studio进入Studio,用于在Jupyter Notebook中运行大模型代码。
2.点击Studio Classic,再点击Open进入Jupyter Notebook
3. 点击主页中的JumpStart功能快速创建大语言模型
4. 搜索并选择”Falcon 7B Instruct BF16“大模型
5. 选择部署大模型的计算资源类型为”ml.g5.4xlarge“,再点击开始部署
6. 我们创建一个requirements.txt文件,复制以下内容用于安装必要依赖。
boto3==1.34.37
langchain==0.0.276
numpy==1.23.5
pandas==1.5.3
scikit_learn==1.2.1
seaborn==0.12.2
sentence-transformers
faiss-gpu-cu11
7. 创建一个Jupyter Notebook ipynb文件,首先运行以下代码指定模型id,并安装必要依赖。
endpoint_name = "jumpstart-dft-hf-llm-falcon-7b-instruct-bf16"
!pip install --upgrade pip
!pip install --upgrade --no-cache --force-reinstall -r requirements.txt
8. 运行以下代码,导入必要依赖
import boto3, json
import pandas as pd
import langchain
from langchain.embeddings import SagemakerEndpointEmbeddings
from langchain.llms.sagemaker_endpoint import ContentHandlerBase
from langchain.chains import RetrievalQA
from langchain.document_loaders import TextLoader
from langchain.indexes import VectorstoreIndexCreator
from langchain.vectorstores import Chroma, AtlasDB, FAISS
from langchain.text_splitter import CharacterTextSplitter
from langchain import PromptTemplate
from langchain.chains.question_answering import load_qa_chain
from langchain.document_loaders.csv_loader import CSVLoader
from langchain.text_splitter import RecursiveCharacterTextSplitter
from langchain.embeddings import HuggingFaceEmbeddings
9. 接下来我们定义两个函数”query_endpoint_with_json_payload“和”parse_response_model“,用于调用大模型和提取生成回复中的内容,并配置模型区域、节点名、回复提取函数、提示词。
def query_endpoint_with_json_payload(encoded_json, endpoint_name, content_type="application/json"):client = boto3.client("runtime.sagemaker")response = client.invoke_endpoint(EndpointName=endpoint_name, ContentType=content_type, Body=encoded_json)return response# parse the Sagemaker Endpoint response to the user query
def parse_response_model(query_response):model_predictions = json.loads(query_response["Body"].read())return [gen["generated_text"] for gen in model_predictions]_MODEL_CONFIG_ = {"jumpstart-dft-hf-llm-falcon-7b-instruct-bf16" : {"aws_region": "us-east-1","endpoint_name": endpoint_name,"parse_function": parse_response_model,"prompt": """{context}\n\nGiven the above context, answer the following question:\n{question}\nAnswer: """,},}
10. 定义模型参数,定义模型输入、输出请求格式,并按该格式调用SageMaker上部署的Falcon模型。
import json
from langchain.llms.sagemaker_endpoint import LLMContentHandler, SagemakerEndpointparameters ={"max_new_tokens": 100,"num_return_sequences": 1,"top_k": 50,"top_p": 0.95,"do_sample": False,"return_full_text": False,"temperature": 0.2}class ContentHandler(LLMContentHandler):content_type = "application/json"accepts = "application/json"def transform_input(self, prompt: str, model_kwargs={}) -> bytes:input_str = json.dumps({"inputs": prompt, "parameters": model_kwargs})return input_str.encode("utf-8")def transform_output(self, output: bytes) -> str:response_json = json.loads(output.read().decode("utf-8"))return response_json[0]["generated_text"]content_handler = ContentHandler()sm_llm_falcon_instruct = SagemakerEndpoint(endpoint_name=_MODEL_CONFIG_["jumpstart-dft-hf-llm-falcon-7b-instruct-bf16"]["endpoint_name"],region_name=_MODEL_CONFIG_["jumpstart-dft-hf-llm-falcon-7b-instruct-bf16"]["aws_region"],model_kwargs=parameters,content_handler=content_handler,
)
11. 向大模型提问示例问题,可以得到常规回复。
sm_llm_falcon_instruct("Which day comes after Friday?")
12. 初始化一个HuggingFace向量模型
sm_llm_embeddings = HuggingFaceEmbeddings()
13. 创建一个关于Amazon SageMaker产品问题与回复的csv文件,再将该csv文件导入Pandas DataFrame,只提取答案后再存入到processd.csv文件中。
s3_path = "s3://jumpstart-cache-prod-us-east-2/training-datasets/Amazon_SageMaker_FAQs/Amazon_SageMaker_FAQs.csv"!mkdir -p rag_data
!aws s3 cp $s3_path rag_data/Amazon_SageMaker_FAQs.csvdf_knowledge = pd.read_csv("rag_data/Amazon_SageMaker_FAQs.csv", header=None, usecols=[1], names=["Answer"])
df_knowledge.to_csv("rag_data/processed.csv", header=False, index=False)
14. 利用LangChain读取csv文件
loader = CSVLoader(file_path="rag_data/processed.csv")
documents = loader.load()
15. 设置llm大模型模型回复参数
sm_llm_falcon_instruct.model_kwargs = {"max_new_tokens": 50,"num_return_sequences": 1,"top_k": 50,"top_p": 0.95,"do_sample": False,"return_full_text": False,"temperature": 0.1
}
16. 利用LangChain将文档进行分割,再使用FAISS
(Facebook AI Similarity Search)通过HuggingFace向量模型将文档转为向量并创建索引。
text_splitter = RecursiveCharacterTextSplitter(chunk_size=100, chunk_overlap=5)
texts = text_splitter.split_documents(documents)
sm_llm_embeddings
docsearch = FAISS.from_documents(texts, sm_llm_embeddings)
17. 通过问题对文档内容进行语义搜索得到回复,问题为:”如何为Amazon SageMaker上的托管临时训练选择实例类型“。
question = "Which instances can I use with managed spot training in Amazon SageMaker?"
docs = docsearch.similarity_search(question, k=3)
docs
得到相关回复和所在文档元数据信息
18. 下面我们通过提示词模板构建问答链,再基于问题调用知识库进行语义搜索得到回复
prompt_template = """{context}\n\nGiven the above context, answer the following question:\n{question}\n\nAnswer:"""PROMPT = PromptTemplate(template=prompt_template, input_variables=["context", "question"])sm_llm_falcon_instruct.model_kwargs = {"max_new_tokens": 50,"num_return_sequences": 1,"top_k": 50,"top_p": 0.95,"do_sample": False,"return_full_text": True,"temperature": 0.1,
}
chain = load_qa_chain(llm=sm_llm_falcon_instruct, prompt=PROMPT)result = chain({"input_documents": docs, "question": question}, return_only_outputs=True)["output_text"]print(result)
我们可以看到LangChain问答链根据我们定义的提示词中的格式得到了正确回复。
19. 我们再创建一个新的Python函数”main.py“,复制以下代码。这个文件包括了我们刚刚通过LangChain文本向量化、利用RAG与大模型与知识库API交互全部完整代码。同时创建了一个streamlit服务器与用户在UI进行交互。
# From Cell 2 with small modifications
import os
import streamlit as st
import json
import boto3
import loggingfrom langchain.chains import RetrievalQA
from langchain.indexes import VectorstoreIndexCreator
from langchain_community.vectorstores import Chroma, FAISS
from langchain.prompts import PromptTemplate
from langchain_community.embeddings import SagemakerEndpointEmbeddings
from langchain_community.embeddings.sagemaker_endpoint import EmbeddingsContentHandler
from langchain_community.llms.sagemaker_endpoint import LLMContentHandler, SagemakerEndpoint
from langchain.text_splitter import RecursiveCharacterTextSplitter, CharacterTextSplitter
from langchain_community.embeddings import HuggingFaceEmbeddings# Set up logging
logger = logging.getLogger()
logger.setLevel(logging.INFO)# From Cell 5
sm_llm_embeddings = HuggingFaceEmbeddings()# From Cell 4 and 6
class ContentHandler(LLMContentHandler):content_type = "application/json"accepts = "application/json"def transform_input(self, prompt: str, model_kwargs={}) -> bytes:input_str = json.dumps({"inputs": prompt, "parameters": model_kwargs})return input_str.encode("utf-8")def transform_output(self, output: bytes) -> str:response_json = json.loads(output.read().decode("utf-8"))return response_json[0]["generated_text"]def query_endpoint_with_json_payload(encoded_json, endpoint_name, content_type="application/json"):client = boto3.client("runtime.sagemaker")response = client.invoke_endpoint(EndpointName=endpoint_name, ContentType=content_type, Body=encoded_json)return responsedef parse_response_model(query_response):model_predictions = json.loads(query_response["Body"].read())return [gen["generated_text"] for gen in model_predictions]# The following replaces cells 8 and 9
# loading PDF, DOCX and TXT files as LangChain Documents
def load_document(file):import osname, extension = os.path.splitext(file)if extension == '.pdf':from langchain.document_loaders import PyPDFLoaderprint(f'Loading {file}')loader = PyPDFLoader(file)elif extension == '.docx':from langchain.document_loaders import Docx2txtLoaderprint(f'Loading {file}')loader = Docx2txtLoader(file)elif extension == '.txt':from langchain.document_loaders import TextLoaderloader = TextLoader(file)elif extension == '.csv':from langchain_community.document_loaders.csv_loader import CSVLoaderloader = CSVLoader(file)else:print('Document format is not supported!')return Nonedocument = loader.load()return document# From Cell 11
def split_text(document):text_splitter = RecursiveCharacterTextSplitter(chunk_size=100, chunk_overlap=5)texts = text_splitter.split_documents(document)return texts# Cell 11
def create_embeddings(texts):docsearch = FAISS.from_documents(texts, sm_llm_embeddings)return docsearch# Not in notebook but needed for streamlite application
def clear_history():if 'history' in st.session_state:del st.session_state['history']# Application build from notebook - see individual parts
def ask_and_get_answer(question, documents):from langchain_community.llms.sagemaker_endpoint import LLMContentHandler, SagemakerEndpointfrom langchain.chains.question_answering import load_qa_chain# From Cell 13docs = documents.similarity_search(question, k=3)# From Cell 14prompt_template = """You are an AI assistant for answering questions.Refrane from providing any information that is not in the provide context.If there is not an answer in the provided context respond with "I don't know."{context}Question: {question}Answer:"""parameters ={"max_new_tokens": 100,"num_return_sequences": 1,"top_k": 50,"top_p": 0.95,"do_sample": False,"return_full_text": False,"temperature": 0.2}# From Cell 4_MODEL_CONFIG_ = {"jumpstart-dft-hf-llm-falcon-7b-instruct-bf16" : {"aws_region": "us-east-1","endpoint_name": "jumpstart-dft-hf-llm-falcon-7b-instruct-bf16","parse_function": parse_response_model,"prompt": prompt_template,},}# From Cell 6content_handler = ContentHandler()sm_llm_falcon_instruct = SagemakerEndpoint(endpoint_name=_MODEL_CONFIG_["jumpstart-dft-hf-llm-falcon-7b-instruct-bf16"]["endpoint_name"],region_name=_MODEL_CONFIG_["jumpstart-dft-hf-llm-falcon-7b-instruct-bf16"]["aws_region"],model_kwargs=parameters,content_handler=content_handler,)# From Cell 10sm_llm_falcon_instruct.model_kwargs = {"max_new_tokens": 50,"num_return_sequences": 1,"top_k": 50,"top_p": 0.95,"do_sample": False,"return_full_text": True,"temperature": 0.1,}# From Cell 14 and 15PROMPT = PromptTemplate(template=prompt_template, input_variables=["context", "question"])chain = load_qa_chain(llm=sm_llm_falcon_instruct, prompt=PROMPT)answer = chain({"input_documents": docs, "question": question}, return_only_outputs=True)["output_text"]return answer# Code required for the Streamlite app
if __name__ == "__main__":import osst.subheader('Retrieval Augmented Generation (RAG)')with st.sidebar:# file uploader widgetuploaded_file = st.file_uploader('Upload context file:', type=['pdf', 'docx', 'txt', 'csv'])# add data button widgetadd_data = st.button('Process Context File', on_click=clear_history)if uploaded_file and add_data: # if the user browsed a filewith st.spinner('Reading, chunking and embedding file ... Please Wait'):# writing the file from RAM to the current directory on diskbytes_data = uploaded_file.read()file_name = os.path.join('./', uploaded_file.name)with open(file_name, 'wb') as f:f.write(bytes_data)document = load_document(file_name)texts = split_text(document)# creating the embeddings and returning FAISS vector store.vector_store = create_embeddings(texts)# saving the vector store in the streamlit session state (to be persistent between reruns)st.session_state.vs = vector_storest.success('File processing completed successfully! You can now ask questions.')# user's question text input widgetquestion = st.text_input('Ask a question about the content of your file:')if question: # if the user entered a question and hit enterquestion = f"{question}"if 'vs' in st.session_state: # if there's the vector store (user uploaded, split and embedded a file)vector_store = st.session_state.vs# st.write(f'k: {k}')response = ask_and_get_answer(question, vector_store)answer = response.partition("Answer:")[2]# text area widget for the LLM answerst.text_area('LLM Answer: ', value=answer, height=400)st.divider()# if there's no chat history in the session state, create itif 'history' not in st.session_state:st.session_state.history = ''# the current question and answervalue = f'Q: {question} \nA: {answer}'st.session_state.history = f'{value} \n {"-" * 100} \n {st.session_state.history}'h = st.session_state.history# text area widget for the chat historyst.text_area(label='Chat History', value=h, key='history')
20. 我们通过以下命令启动streamlit服务器。启动后会返回服务器的URL地址,复制该地址在浏览器中打开。
streamlit run main.py --server.enableXsrfProtection false
21. 浏览器中打开后,我们进入了UI界面,点击”Browse files“上传文档,上传刚刚我们从Amazon Sagemaker产品常见问题csv文件中提取的问题csv文件,"processed.csv"。
22. 上传成功后,我们再问题界面提问”什么是SageMaker“,就可以得到利用RAG基于知识库中文档得到的相关回答。
以上就是在亚马逊云科技上利用亚马逊云科技上利用部署开源大模型,并利用RAG技术和Streamlit开发GenAI文档问答服务的全部步骤。欢迎大家未来与我一起,未来获取更多国际前沿的生成式AI开发方案。
相关文章:
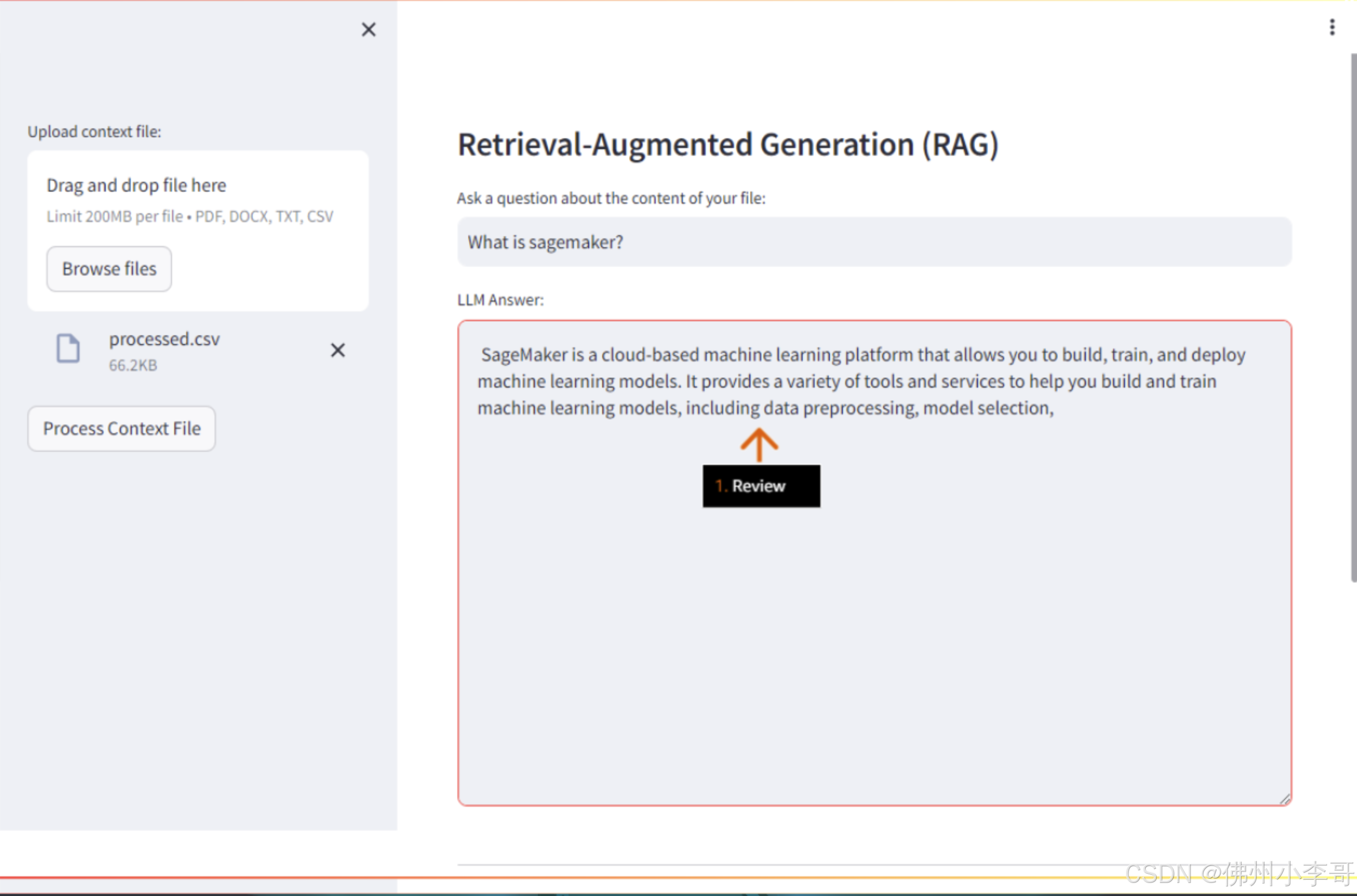
在亚马逊云科技上部署开源大模型并利用RAG和LangChain开发生成式AI应用
项目简介: 小李哥将继续每天介绍一个基于亚马逊云科技AWS云计算平台的全球前沿AI技术解决方案,帮助大家快速了解国际上最热门的云计算平台亚马逊云科技AWS AI最佳实践,并应用到自己的日常工作里。 本次介绍的是如何在亚马逊云科技上利用Sag…...
Spring——Bean的生命周期
Bean的生命周期牵扯到Bean的实例化、属性赋值、初始化、销毁 其中Bean的实例化有四种方法、构造器实例化、静态工厂、实例工厂、实现FactoryBean接口 对于Bean的生命周期我们可以在Bean初始化之后、销毁之前对Bean进行控制 两种方法: 一、配置 1、在Bean的对象…...
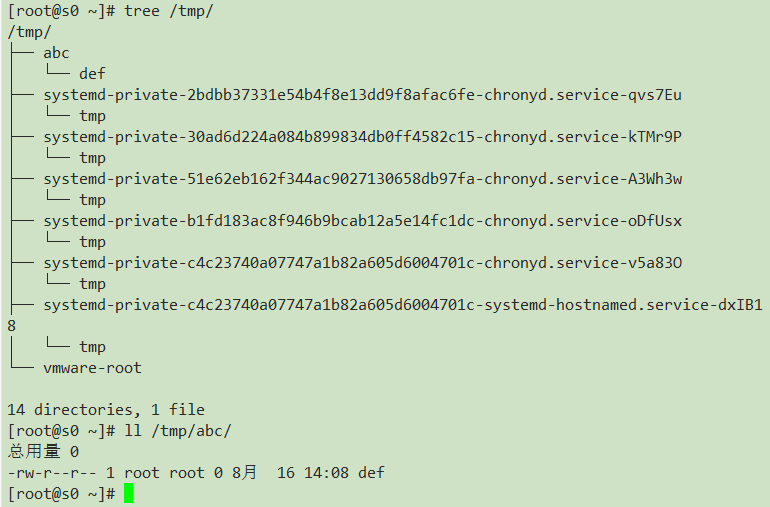
云计算实训30——自动化运维(ansible)
自动化运维 ansible----自动化运维工具 特点: 部署简单,使用ssh管理 管理端与被管理端不需要启动服务 配置简单、功能强大,扩展性强 一、ansible环境搭建 准备四台机器 安装步骤 mo服务器: #下载epel [rootmo ~]# yum -y i…...
网络性能优化:从问题诊断到解决方案
网络性能优化是确保网络高效、稳定运行的关键过程,它通过改进网络设备、协议和配置,以提高网络吞吐量、降低延迟并提升用户体验。在网络性能优化的全过程中,从问题诊断到解决方案的实施,需要经过一系列详细的步骤和策略。本文将从…...
深度学习10--强化学习
强化学习(增强学习、再励学习、评价学习简称RL)是近年来机器学习领域最热门的方向之一,是实现通用人工智能的重要方法之一。本章将通俗易懂地讲一下强化学习中的两个重要的模型DQN 和DDPG。 马尔可夫决策过程(Markov Decison Process,MDP)包括两个对象ÿ…...
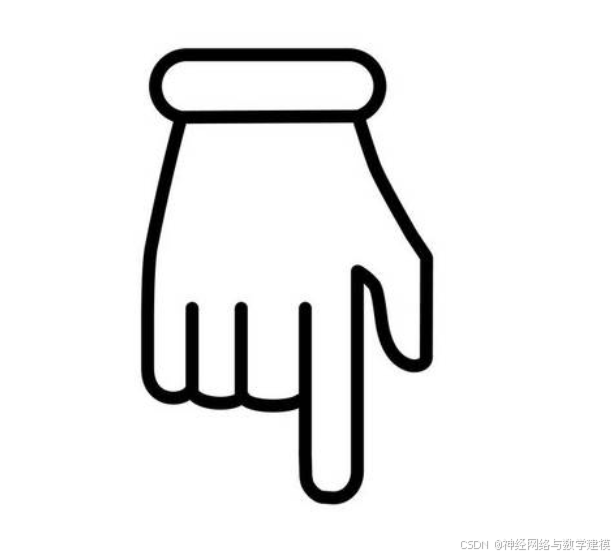
SSA-SVM多变量回归预测|樽海鞘群优化算法-支持向量机|Matalb
目录 一、程序及算法内容介绍: 基本内容: 亮点与优势: 二、实际运行效果: 三、算法介绍: 四、完整程序下载: 一、程序及算法内容介绍: 基本内容: 本代码基于Matlab平台编译&a…...
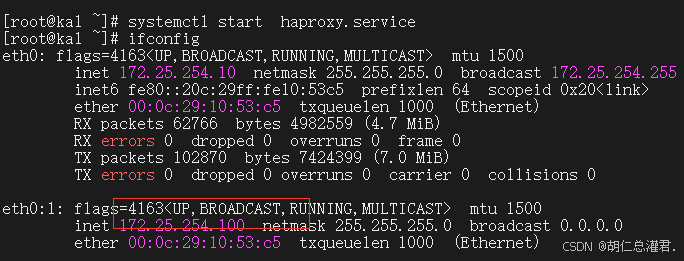
KEEPALIVED高可用集群知识大全
目录 一、KEEPALIVED高可用集群简介 1、Keepalived 高可用集群的工作原理 2、Keepalived 高可用集群的作用 二、KEEPALIVED部署 1、网络配置 2、软件安装与启动 3、配置虚拟路由器 4、效果实现 三、启用keepalived日志功能 四、KEEPALIVED的几种工作模式 1、KEEPALI…...
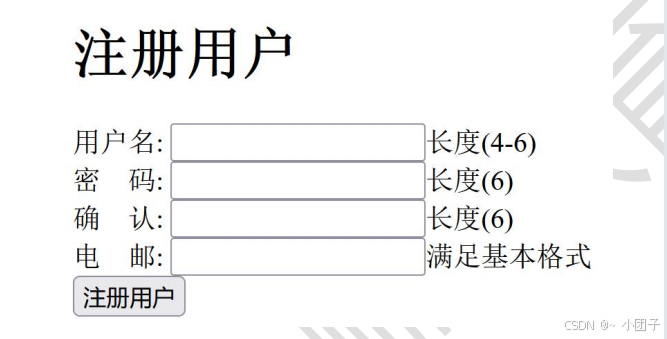
JavaWeb系列三: JavaScript学习 下
JavaScript学习 数组学习数组定义数组使用和遍历 js函数快速入门函数定义方式方式1: function关键字定义函数方式2: 将函数赋给变量 js函数注意事项和细节js函数练习 js自定义对象方式1: Object形式方式2: {}形式 事件基本介绍事件分类onload加载完成事件onclick单击事件onblur…...
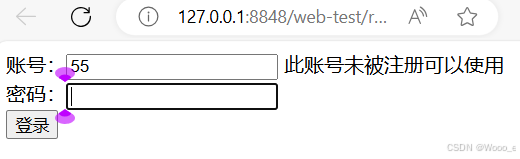
web开发,过滤器,前后端交互
目录 web开发概述 web开发环境搭建 Servlet概述 Servlet的作用: Servlet创建和使用 Servlet生命周期 http请求 过滤器 过滤器的使用场景: 通过Filter接口来实现: 前后端项目之间的交互: 1、同步请求 2、异步请求 优化…...
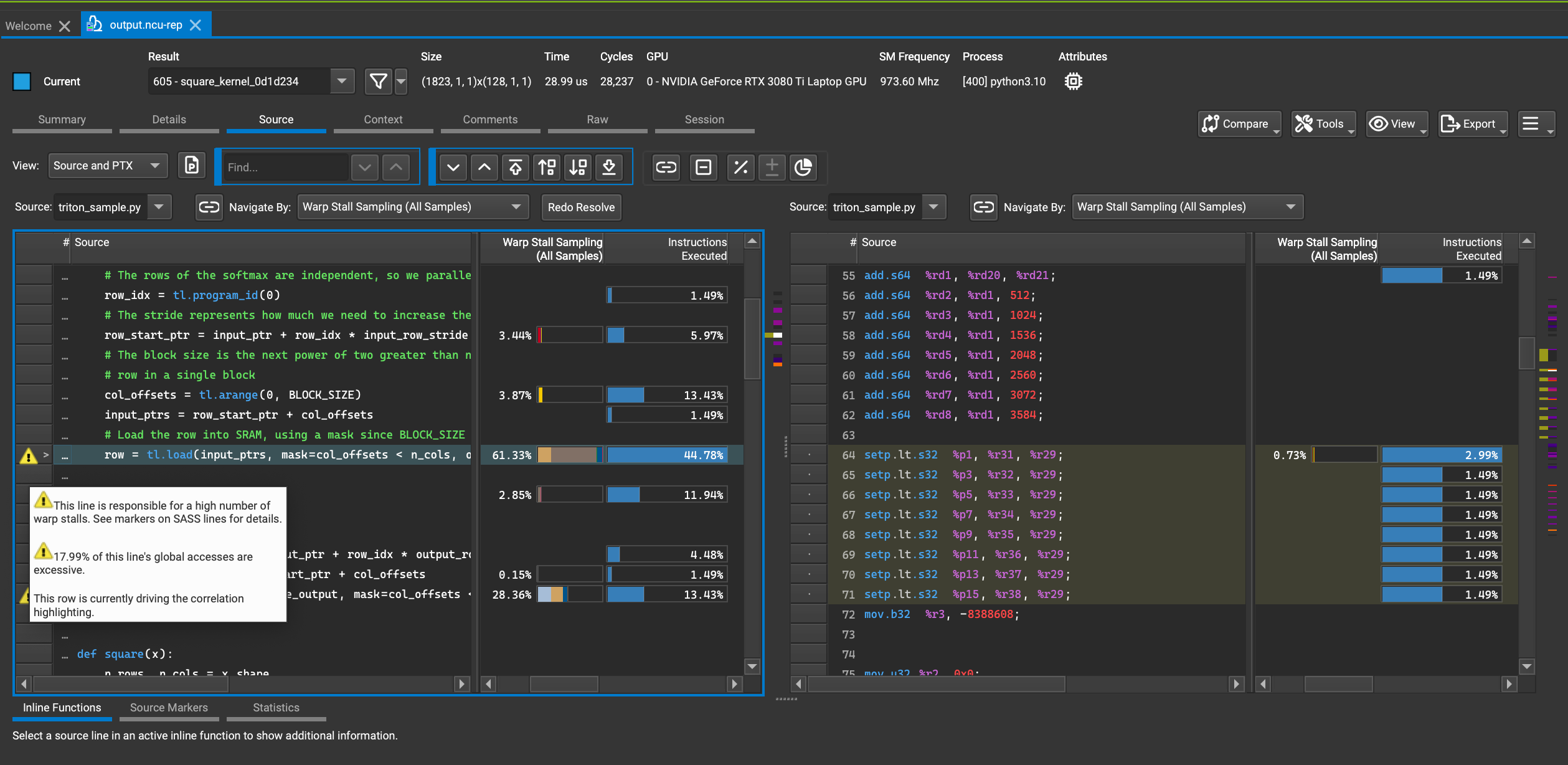
CUDA-MODE 第一课课后实战(下)
我的课程笔记,欢迎关注:https://github.com/BBuf/how-to-optim-algorithm-in-cuda/tree/master/cuda-mode CUDA-MODE 第一课课后实战(下) Nsight Compute Profile结果分析 继续对Nsight Compute的Profile结果进行分析࿰…...
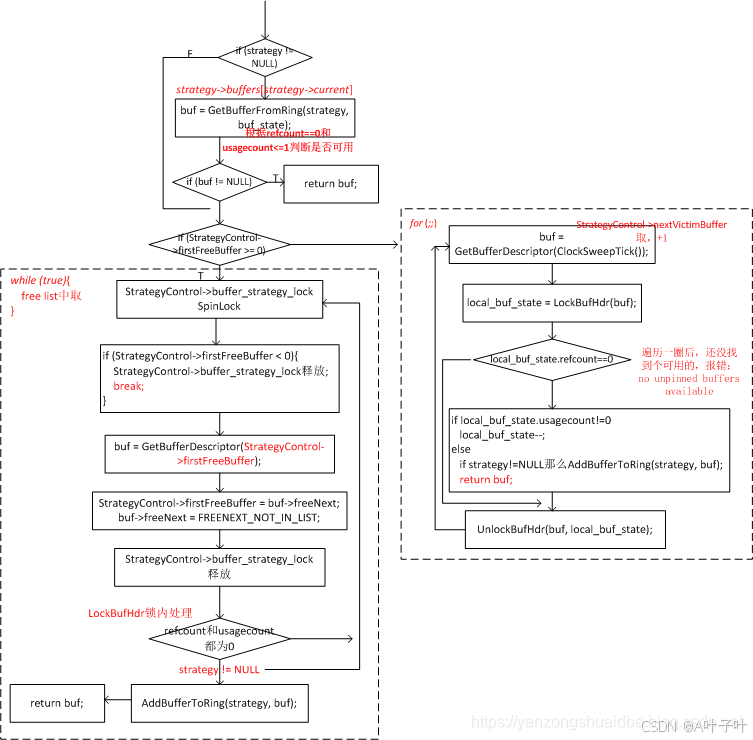
PostgreSQL数据库内核(三):缓冲区管理器
文章目录 共享缓冲区基础知识逻辑读和物理读LRU算法和CLOCK时钟算法 共享缓冲区管理器结构共享缓冲表层共享缓冲区描述符层共享缓冲页层 共享缓冲区管理器工作流程初始化缓冲区读缓冲区淘汰策略共享缓冲区锁 共享缓冲区基础知识 通常数据库系统都会在内存中预留buffer缓冲空间…...
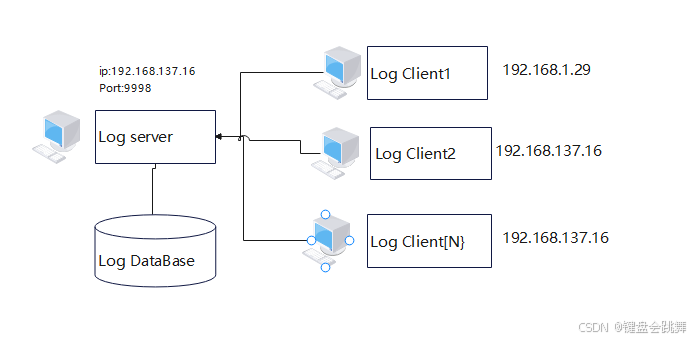
[log4cplus]: 快速搭建分布式日志系统
关键词: 日志系统 、日志分类、自动分文件夹、按时间(月/周/日/小时/分)轮替 一、引言 这里我默认看此文的我的朋友们都已经具备一定的基础,所以,我们本篇不打算讲关于log4cplus的基础内容,文中如果涉及到没有吃透的点,需要朋友们动动自己聪明的脑袋和发财的手指,进一…...
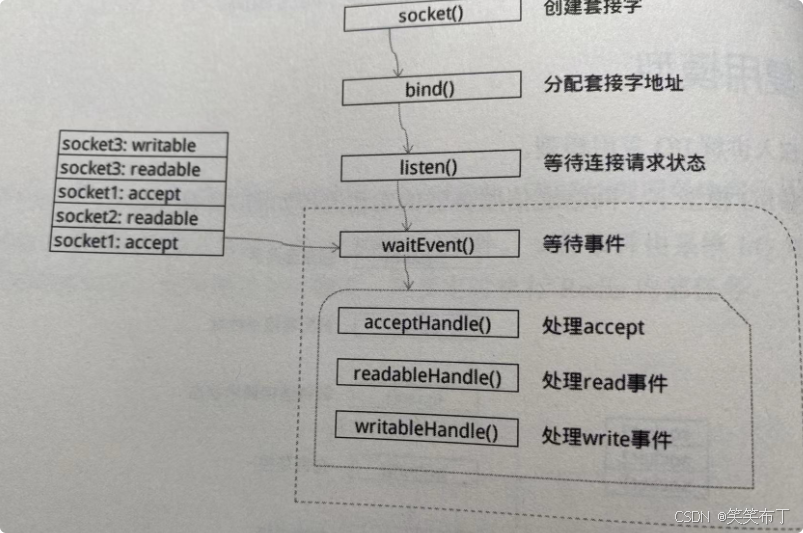
redis I/O复用机制
I/O复用模型 传统阻塞I/O模型 串行化处理,就是要等,假如进行到accept操作,cpu需要等待客户端发送的数据到tcp接收缓冲区才能进行read操作,而在此期间cpu不能执行任何操作。 I/O复用 用一个进程监听大量连接,当某个连…...

Adobe PhotoShop - 制图操作
1. 排布照片 菜单 - 视图 - 对齐:打开后图层将会根据鼠标的移动智能对齐 菜单 - 视图 - 标尺:打开后在页面出现横纵标尺,方便图层的对齐与排列 2. 自动生成全景照 在日常处理中,我们常常想要将几张图片进行拼接获得一张全景图&…...
Mysql 中的Undo日志
在 MySQL 的 InnoDB 存储引擎中,Undo Log 是用于实现数据库事务的回滚功能的一种日志。Undo Log 记录了对数据的修改,以便在事务出现问题时可以恢复到之前的状态。下面将介绍 Undo Log 的结构和样本数据。 Undo Log 的基本概念 目的: Undo Log 的主要目…...
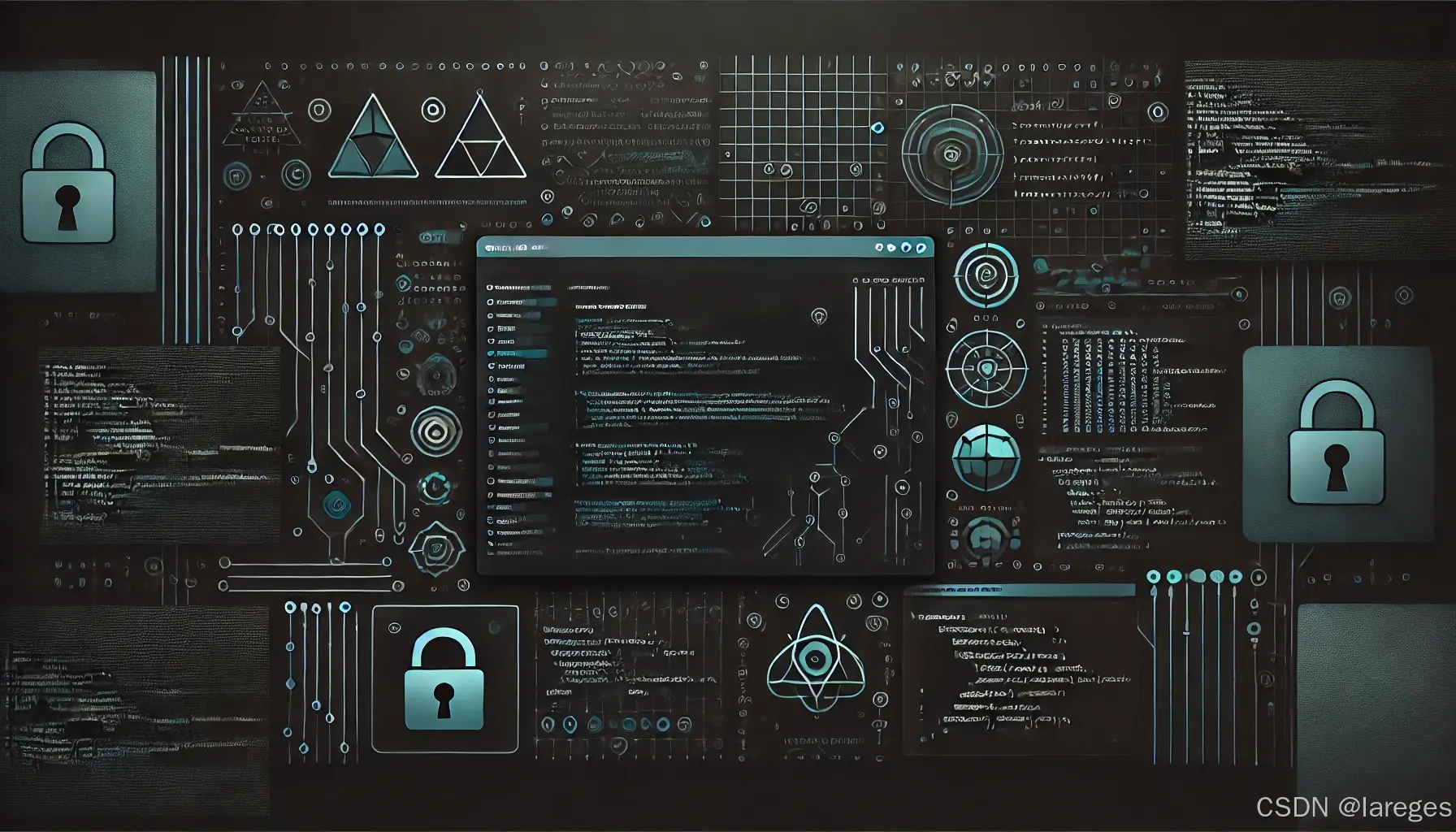
虹软科技25届校招笔试算法 A卷
目录 1. 第一题2. 第二题3. 论述题 ⏰ 时间:2024/08/18 🔄 输入输出:ACM格式 ⏳ 时长:2h 本试卷分为不定项选择,编程题,必做论述题和选做论述题,这里只展示编程题和必做论述题,一共三…...
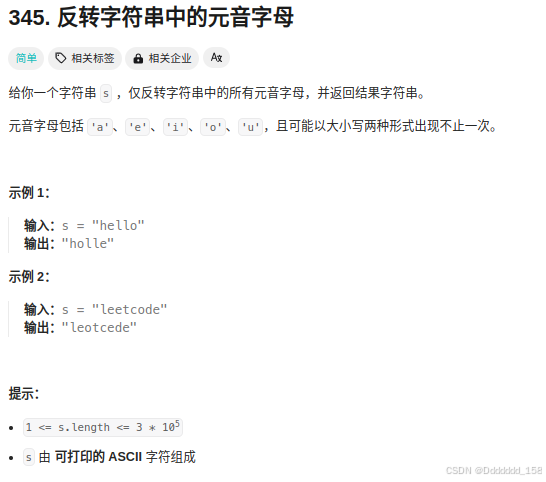
C++ | Leetcode C++题解之第345题反转字符串中的元音字母
题目: 题解: class Solution { public:string reverseVowels(string s) {auto isVowel [vowels "aeiouAEIOU"s](char ch) {return vowels.find(ch) ! string::npos;};int n s.size();int i 0, j n - 1;while (i < j) {while (i < …...
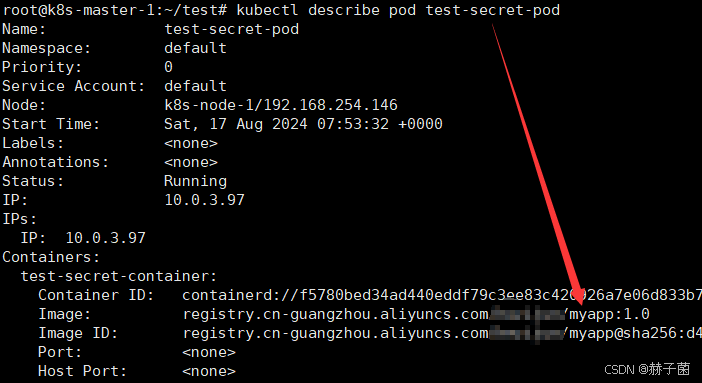
Kubernetes拉取阿里云的私人镜像
前提条件 登录到阿里云控制台 拥有阿里云的ACR服务 创建一个命名空间 获取仓库的访问凭证(可以设置固定密码) 例如 sudo docker login --usernameyourAliyunAccount registry.cn-guangzhou.aliyuncs.com 在K8s集群中创建一个secret 使用kubectl命令行…...
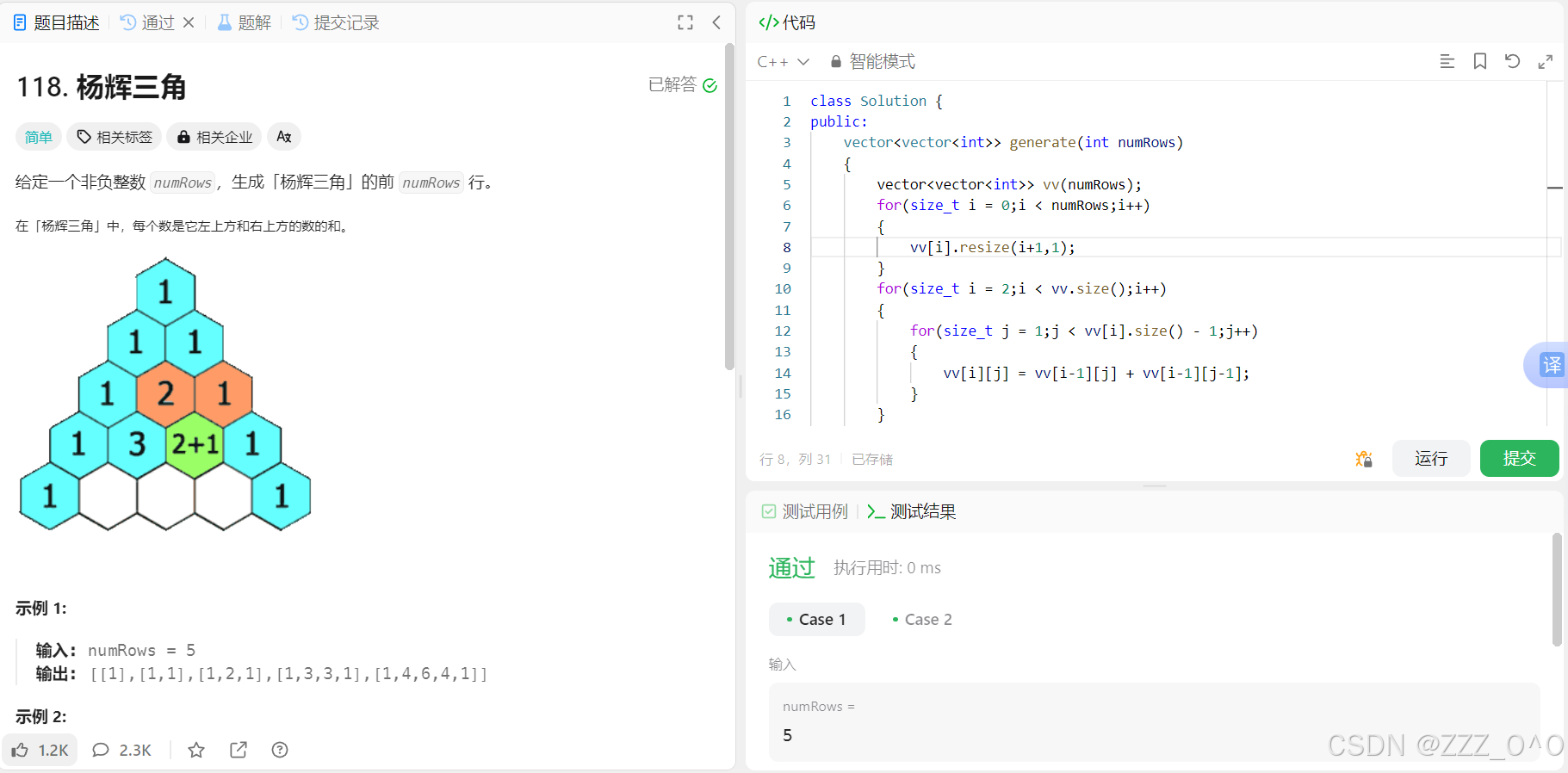
Leetcode每日刷题之118.杨辉三角
1.题目解析 杨辉三角作为一个经典的数学模型,其基本原理相信大家已经耳熟能详,这里主要是在学习了vector之后,对于本题有了新的解法,更加简便。关于vector的基本使用详见 面向对象程序设计(C)之 vector(初阶࿰…...
【ARM 芯片 安全与攻击 5.2 -- 芯片中侧信道攻击与防御方法介绍】
文章目录 什么是 Speculation Barriers?如何使用 Speculation Barriers?什么是 PAN?如何启用 PAN?使用 PAN 保护操作系统Spectre 攻击防御示例Meltdown 攻击防御示例Summary什么是 Speculation Barriers? Speculation Barriers,是一种防止处理器在投机执行中泄漏敏感信息…...
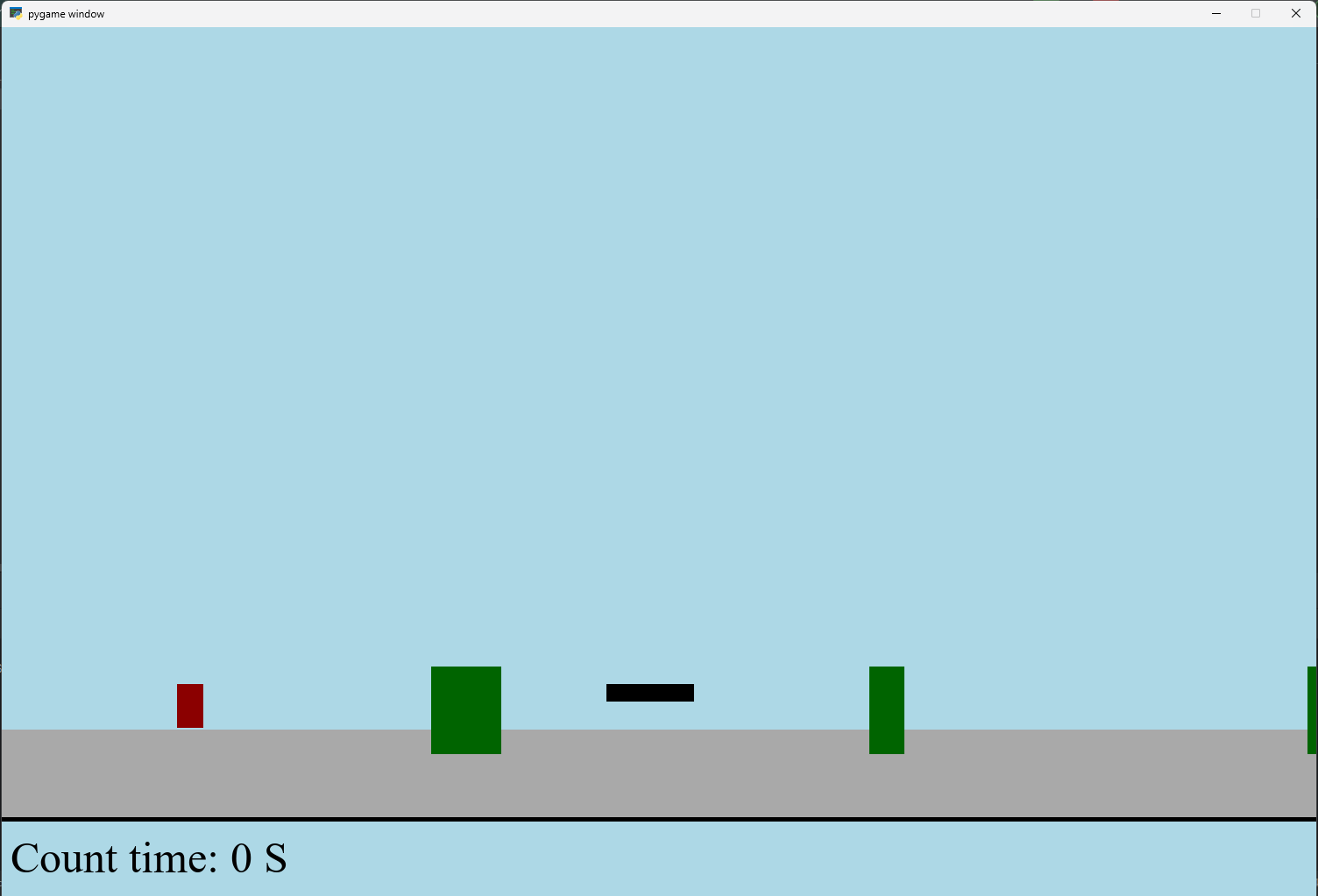
【Python】 -- 趣味代码 - 小恐龙游戏
文章目录 文章目录 00 小恐龙游戏程序设计框架代码结构和功能游戏流程总结01 小恐龙游戏程序设计02 百度网盘地址00 小恐龙游戏程序设计框架 这段代码是一个基于 Pygame 的简易跑酷游戏的完整实现,玩家控制一个角色(龙)躲避障碍物(仙人掌和乌鸦)。以下是代码的详细介绍:…...
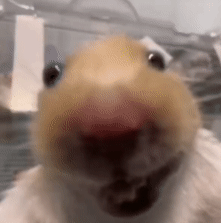
C++初阶-list的底层
目录 1.std::list实现的所有代码 2.list的简单介绍 2.1实现list的类 2.2_list_iterator的实现 2.2.1_list_iterator实现的原因和好处 2.2.2_list_iterator实现 2.3_list_node的实现 2.3.1. 避免递归的模板依赖 2.3.2. 内存布局一致性 2.3.3. 类型安全的替代方案 2.3.…...
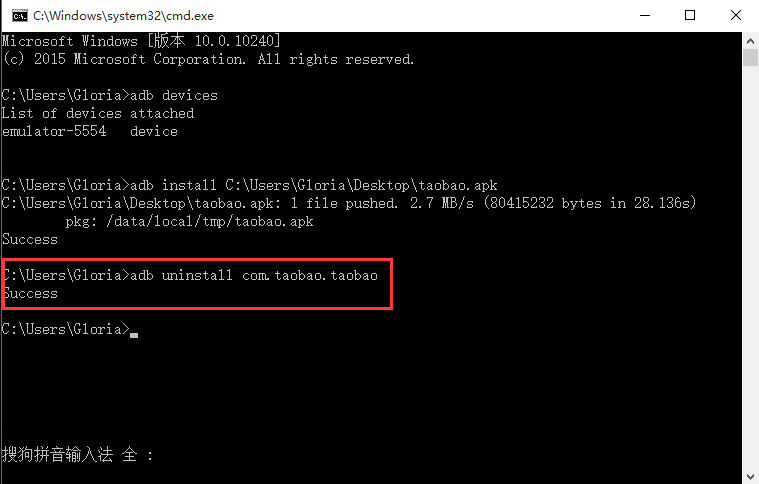
Appium+python自动化(十六)- ADB命令
简介 Android 调试桥(adb)是多种用途的工具,该工具可以帮助你你管理设备或模拟器 的状态。 adb ( Android Debug Bridge)是一个通用命令行工具,其允许您与模拟器实例或连接的 Android 设备进行通信。它可为各种设备操作提供便利,如安装和调试…...
Java如何权衡是使用无序的数组还是有序的数组
在 Java 中,选择有序数组还是无序数组取决于具体场景的性能需求与操作特点。以下是关键权衡因素及决策指南: ⚖️ 核心权衡维度 维度有序数组无序数组查询性能二分查找 O(log n) ✅线性扫描 O(n) ❌插入/删除需移位维护顺序 O(n) ❌直接操作尾部 O(1) ✅内存开销与无序数组相…...
【解密LSTM、GRU如何解决传统RNN梯度消失问题】
解密LSTM与GRU:如何让RNN变得更聪明? 在深度学习的世界里,循环神经网络(RNN)以其卓越的序列数据处理能力广泛应用于自然语言处理、时间序列预测等领域。然而,传统RNN存在的一个严重问题——梯度消失&#…...
Java多线程实现之Callable接口深度解析
Java多线程实现之Callable接口深度解析 一、Callable接口概述1.1 接口定义1.2 与Runnable接口的对比1.3 Future接口与FutureTask类 二、Callable接口的基本使用方法2.1 传统方式实现Callable接口2.2 使用Lambda表达式简化Callable实现2.3 使用FutureTask类执行Callable任务 三、…...
第25节 Node.js 断言测试
Node.js的assert模块主要用于编写程序的单元测试时使用,通过断言可以提早发现和排查出错误。 稳定性: 5 - 锁定 这个模块可用于应用的单元测试,通过 require(assert) 可以使用这个模块。 assert.fail(actual, expected, message, operator) 使用参数…...
【HTTP三个基础问题】
面试官您好!HTTP是超文本传输协议,是互联网上客户端和服务器之间传输超文本数据(比如文字、图片、音频、视频等)的核心协议,当前互联网应用最广泛的版本是HTTP1.1,它基于经典的C/S模型,也就是客…...
管理学院权限管理系统开发总结
文章目录 🎓 管理学院权限管理系统开发总结 - 现代化Web应用实践之路📝 项目概述🏗️ 技术架构设计后端技术栈前端技术栈 💡 核心功能特性1. 用户管理模块2. 权限管理系统3. 统计报表功能4. 用户体验优化 🗄️ 数据库设…...
AGain DB和倍数增益的关系
我在设置一款索尼CMOS芯片时,Again增益0db变化为6DB,画面的变化只有2倍DN的增益,比如10变为20。 这与dB和线性增益的关系以及传感器处理流程有关。以下是具体原因分析: 1. dB与线性增益的换算关系 6dB对应的理论线性增益应为&…...