大模型训练框架DeepSpeed使用入门(1): 训练设置
文章目录
- 一、安装
- 二、训练设置
- Step1 第一步参数解析
- Step2 初始化后端
- Step3 训练初始化
- 三、训练代码展示
官方文档直接抄过来,留个笔记。
https://deepspeed.readthedocs.io/en/latest/initialize.html
使用案例来自:
https://github.com/OvJat/DeepSpeedTutorial
大模型训练的痛点是模型参数过大,动辄上百亿,如果单靠单个GPU来完成训练基本不可能。所以需要多卡或者分布式训练来完成这项工作。
DeepSpeed是由Microsoft提供的分布式训练工具,旨在支持更大规模的模型和提供更多的优化策略和工具。对于更大模型的训练来说,DeepSpeed提供了更多策略,例如:Zero、Offload等。
本文简单介绍下如何使用DeepSpeed。
一、安装
pip install deepspeed
二、训练设置
Step1 第一步参数解析
DeepSpeed 使用 argparse
来应用控制台的设置,使用
deepspeed.add_config_arguments()
可以将DeepSpeed内置的参数增加到我们自己的应用参数解析中。
parser = argparse.ArgumentParser(description='My training script.')
parser.add_argument('--local_rank', type=int, default=-1,help='local rank passed from distributed launcher')
# Include DeepSpeed configuration arguments
parser = deepspeed.add_config_arguments(parser)
cmd_args = parser.parse_args()
Step2 初始化后端
与Step3中的 deepspeed.initialize()
不同,
直接调用即可。
一般发生在以下场景
when using model parallelism, pipeline parallelism, or certain data loader scenarios.
在Step3的initialize前,进行调用
deepspeed.init_distributed()
Step3 训练初始化
首先调用 deepspeed.initialize()
进行初始化,是整个调用DeepSpeed训练的入口。
调用后,如果分布式后端没有被初始化后,此时会初始化分布式后端。
使用案例:
model_engine, optimizer, _, _ = deepspeed.initialize(args=cmd_args,model=net,model_parameters=net.parameters(),training_data=ds)
API如下:
def initialize(args=None,model: torch.nn.Module = None,optimizer: Optional[Union[Optimizer, DeepSpeedOptimizerCallable]] = None,model_parameters: Optional[torch.nn.Module] = None,training_data: Optional[torch.utils.data.Dataset] = None,lr_scheduler: Optional[Union[_LRScheduler, DeepSpeedSchedulerCallable]] = None,distributed_port: int = TORCH_DISTRIBUTED_DEFAULT_PORT,mpu=None,dist_init_required: Optional[bool] = None,collate_fn=None,config=None,config_params=None):"""Initialize the DeepSpeed Engine.Arguments:args: an object containing local_rank and deepspeed_config fields.This is optional if `config` is passed.model: Required: nn.module class before apply any wrappersoptimizer: Optional: a user defined Optimizer or Callable that returns an Optimizer object.This overrides any optimizer definition in the DeepSpeed json config.model_parameters: Optional: An iterable of torch.Tensors or dicts.Specifies what Tensors should be optimized.training_data: Optional: Dataset of type torch.utils.data.Datasetlr_scheduler: Optional: Learning Rate Scheduler Object or a Callable that takes an Optimizer and returns a Scheduler object.The scheduler object should define a get_lr(), step(), state_dict(), and load_state_dict() methodsdistributed_port: Optional: Master node (rank 0)'s free port that needs to be used for communication during distributed trainingmpu: Optional: A model parallelism unit object that implementsget_{model,data}_parallel_{rank,group,world_size}()dist_init_required: Optional: None will auto-initialize torch distributed if needed,otherwise the user can force it to be initialized or not via boolean.collate_fn: Optional: Merges a list of samples to form amini-batch of Tensor(s). Used when using batched loading from amap-style dataset.config: Optional: Instead of requiring args.deepspeed_config you can pass your deepspeed configas an argument instead, as a path or a dictionary.config_params: Optional: Same as `config`, kept for backwards compatibility.Returns:A tuple of ``engine``, ``optimizer``, ``training_dataloader``, ``lr_scheduler``* ``engine``: DeepSpeed runtime engine which wraps the client model for distributed training.* ``optimizer``: Wrapped optimizer if a user defined ``optimizer`` is supplied, or ifoptimizer is specified in json config else ``None``.* ``training_dataloader``: DeepSpeed dataloader if ``training_data`` was supplied,otherwise ``None``.* ``lr_scheduler``: Wrapped lr scheduler if user ``lr_scheduler`` is passed, orif ``lr_scheduler`` specified in JSON configuration. Otherwise ``None``."""
三、训练代码展示
def parse_arguments():import argparseparser = argparse.ArgumentParser(description='deepspeed training script.')parser.add_argument('--local_rank', type=int, default=-1,help='local rank passed from distributed launcher')# Include DeepSpeed configuration argumentsparser = deepspeed.add_config_arguments(parser)args = parser.parse_args()return argsdef train():args = parse_arguments()# init distributeddeepspeed.init_distributed()# init modelmodel = MyClassifier(3, 100, ch_multi=128)# init datasetds = MyDataset((3, 512, 512), 100, sample_count=int(1e6))# init engineengine, optimizer, training_dataloader, lr_scheduler = deepspeed.initialize(args=args,model=model,model_parameters=model.parameters(),training_data=ds,# config=deepspeed_config,)# load checkpointengine.load_checkpoint("./data/checkpoints/MyClassifier/")# trainlast_time = time.time()loss_list = []echo_interval = 10engine.train()for step, (xx, yy) in enumerate(training_dataloader):step += 1xx = xx.to(device=engine.device, dtype=torch.float16)yy = yy.to(device=engine.device, dtype=torch.long).reshape(-1)outputs = engine(xx)loss = tnf.cross_entropy(outputs, yy)engine.backward(loss)engine.step()loss_list.append(loss.detach().cpu().numpy())if step % echo_interval == 0:loss_avg = np.mean(loss_list[-echo_interval:])used_time = time.time() - last_timetime_p_step = used_time / echo_intervalif args.local_rank == 0:logging.info("[Train Step] Step:{:10d} Loss:{:8.4f} | Time/Batch: {:6.4f}s",step, loss_avg, time_p_step,)last_time = time.time()# save checkpointengine.save_checkpoint("./data/checkpoints/MyClassifier/")
最后~
码字不易~~
独乐不如众乐~~
如有帮助,欢迎点赞+收藏~~
相关文章:

大模型训练框架DeepSpeed使用入门(1): 训练设置
文章目录 一、安装二、训练设置Step1 第一步参数解析Step2 初始化后端Step3 训练初始化 三、训练代码展示 官方文档直接抄过来,留个笔记。 https://deepspeed.readthedocs.io/en/latest/initialize.html 使用案例来自: https://github.com/OvJat/DeepSp…...
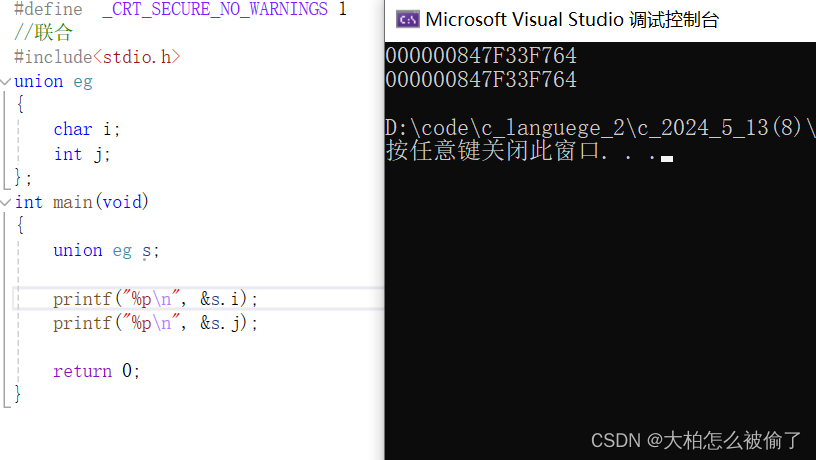
自定义类型——结构体、枚举和联合
自定义类型——结构体、枚举和联合 结构体结构体的声明匿名结构体结构体的自引用结构体的初始化结构体的内存对齐修改默认对齐数结构体传参 位段枚举联合 结构体 结构是一些值的集合,这些值被称为成员变量,结构的每个成员可以是不同类型的变量。 数组是…...
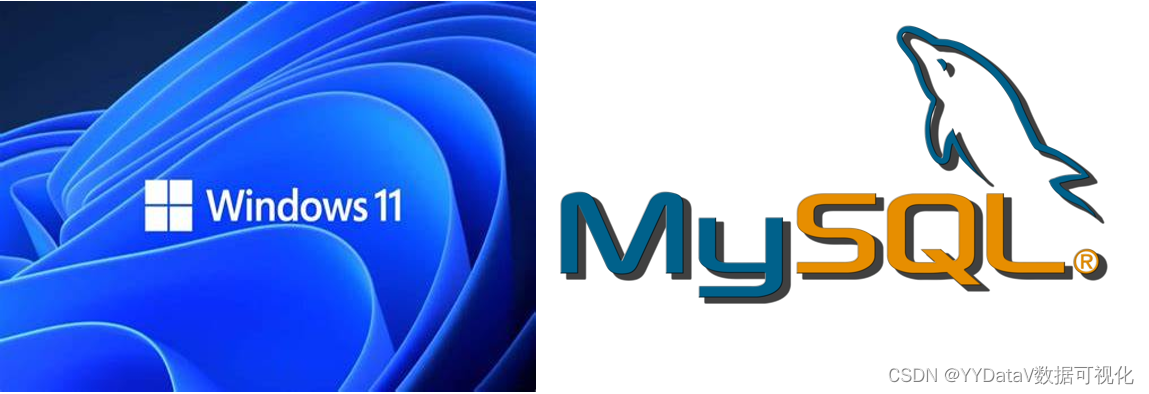
Windows11系统安装Mysql8之后,启动服务net start mysql报错“服务没有响应控制功能”的解决办法
问题 系统环境:Windows11 数据库版本:Mysql8 双击安装,一路下一步,完成,很顺利,但是开启服务后 net start mysql 报错: 服务没有响应控制功能。 请键入 NET HELPMSG 2186 以获得更多的帮助 不…...
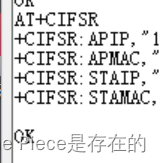
WIFI模块的AT指令联网数据交互--第十天
1.1.蓝牙,ESP-01s,Zigbee, NB-Iot等通信模块都是基于AT指令的设计 初始配置和验证 ESP-01s出厂波特率正常是115200, 注意:AT指令,控制类都要加回车,数据传输时不加回车 1.2.上电后,通过串口输出一串系统…...
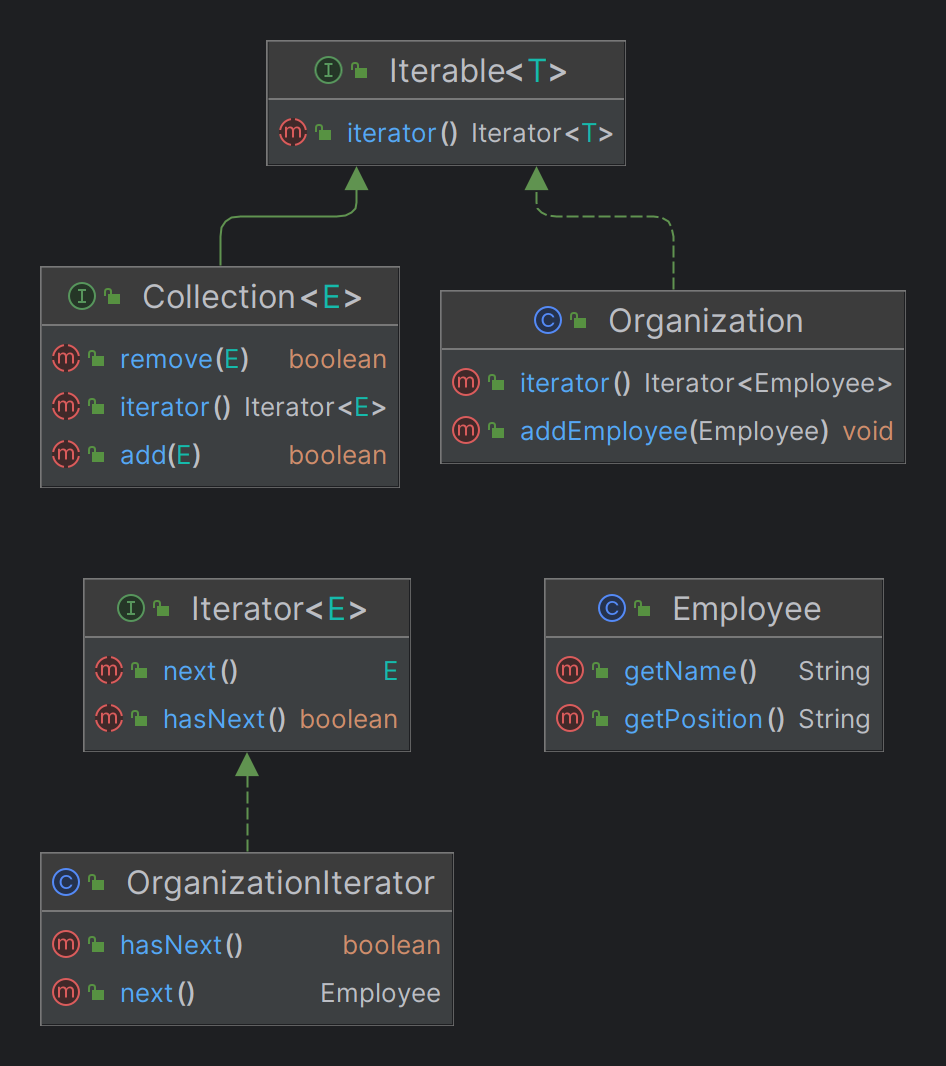
设计模式Java实现-迭代器模式
✨这里是第七人格的博客✨小七,欢迎您的到来~✨ 🍅系列专栏:设计模式🍅 ✈️本篇内容: 迭代器模式✈️ 🍱 本篇收录完整代码地址:https://gitee.com/diqirenge/design-pattern 🍱 楔子 很久…...
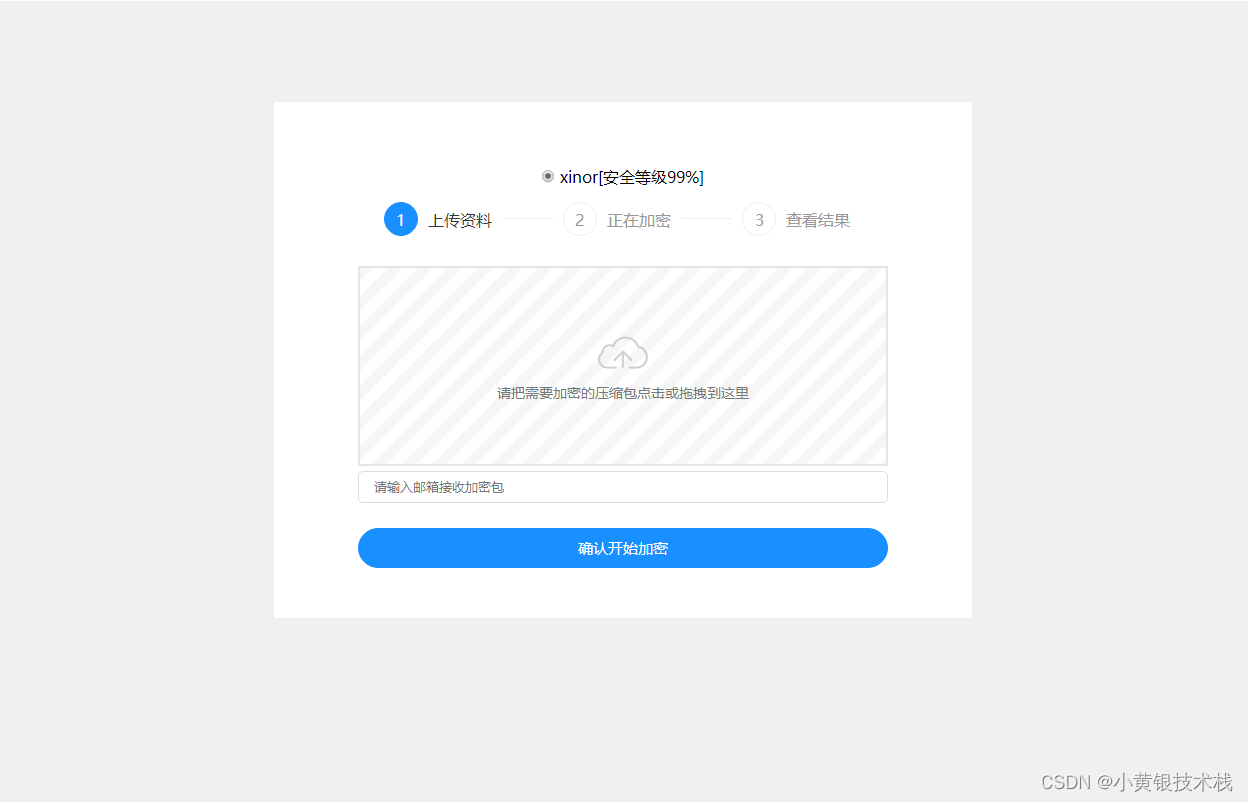
单页源码加密屋zip文件加密API源码
简介: 单页源码加密屋zip文件加密API源码 api源码里面的参数已改好,往服务器或主机一丢就行,出现不能加密了就是加密次数达到上限了,告诉我在到后台修改加密次数 点击下载...
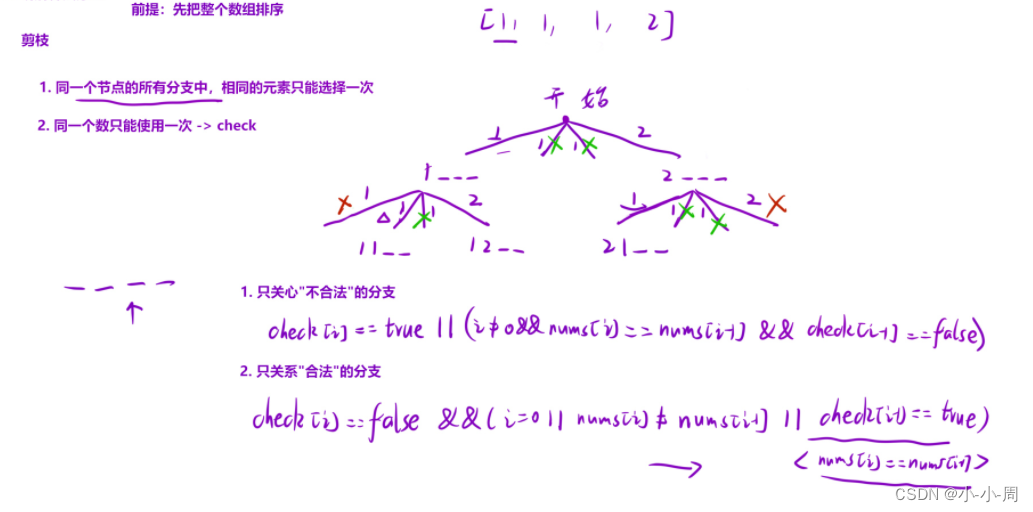
47.全排列
1.题目 47. 全排列 II - 力扣(LeetCode)https://leetcode.cn/problems/permutations-ii/description/ 2.思路 注意剪枝的条件 3.代码 class Solution {vector<int> path;vector<vector<int>> ret;bool check[9]; public:vector<…...
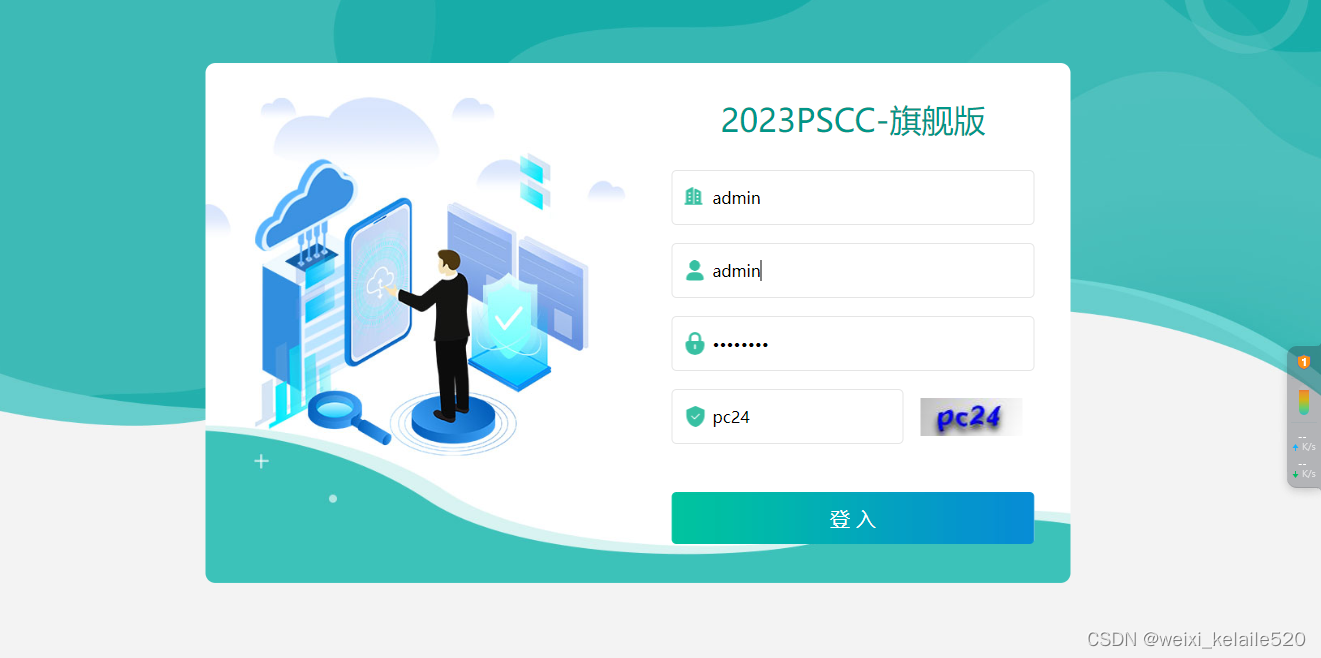
呼叫中心系统选pscc好还是okcc好
选择PSCC(商业软件呼叫中心)还是OKCC(开源呼叫中心),应基于以下几个关键因素来决定: 技术能力:如果企业拥有或愿意投入资源培养内部技术团队,开源解决方案可能更合适,因为…...

【SRC实战】前端脱敏信息泄露
挖个洞先 https://mp.weixin.qq.com/s/xnCQQCAneT21vYH8Q3OCpw “ 以下漏洞均为实验靶场,如有雷同,纯属巧合 ” 01 — 漏洞证明 一、前端脱敏,请求包泄露明文 “ 前端脱敏处理,请求包是否存在泄露? ” 1、获取验…...
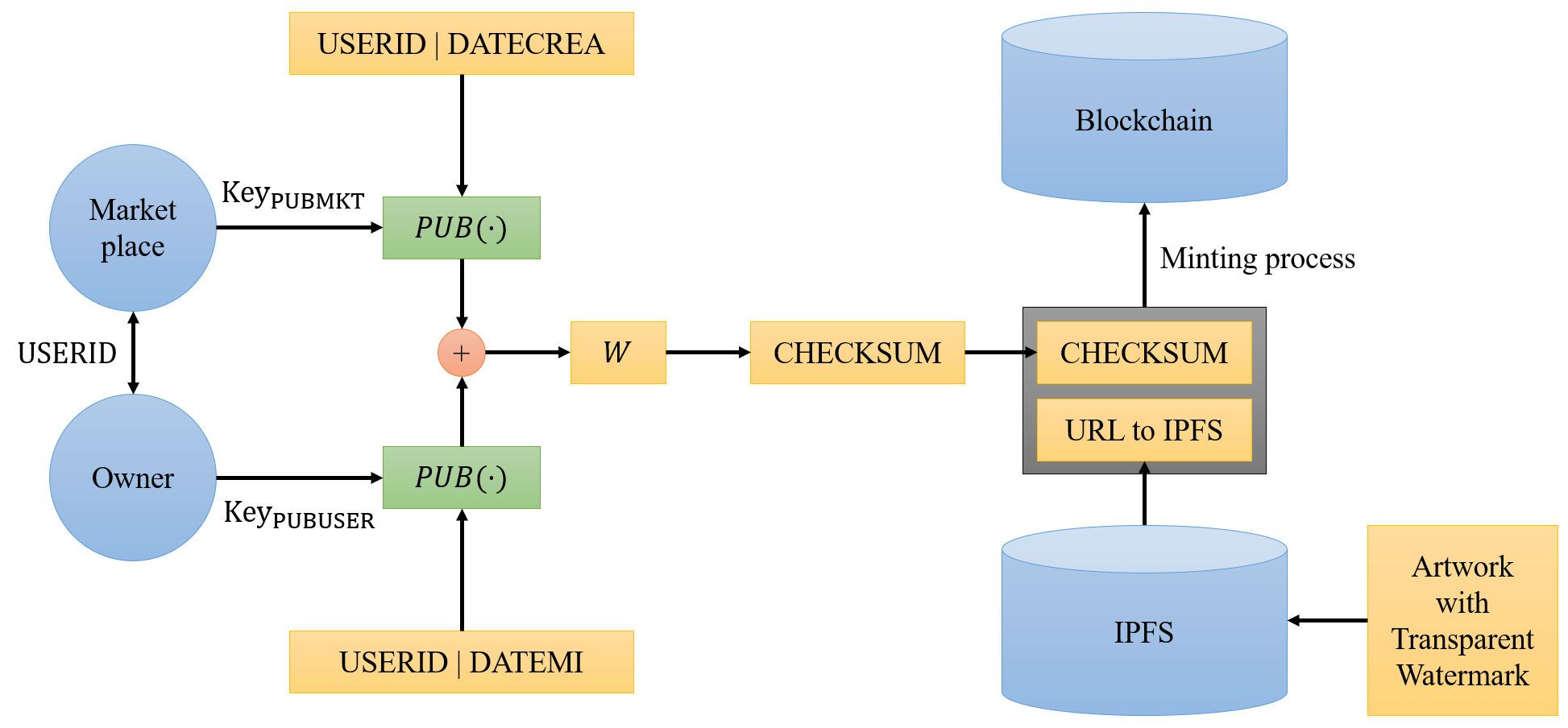
区块链 | NFT 水印:Review on Watermarking Techniques(三)
🍍原文:Review on Watermarking Techniques Aiming Authentication of Digital Image Artistic Works Minted as NFTs into Blockchains 一个 NFT 的水印认证协议 可以引入第三方实体来实现对交易的认证,即通过使用 R S A \mathsf{RSA} RSA…...
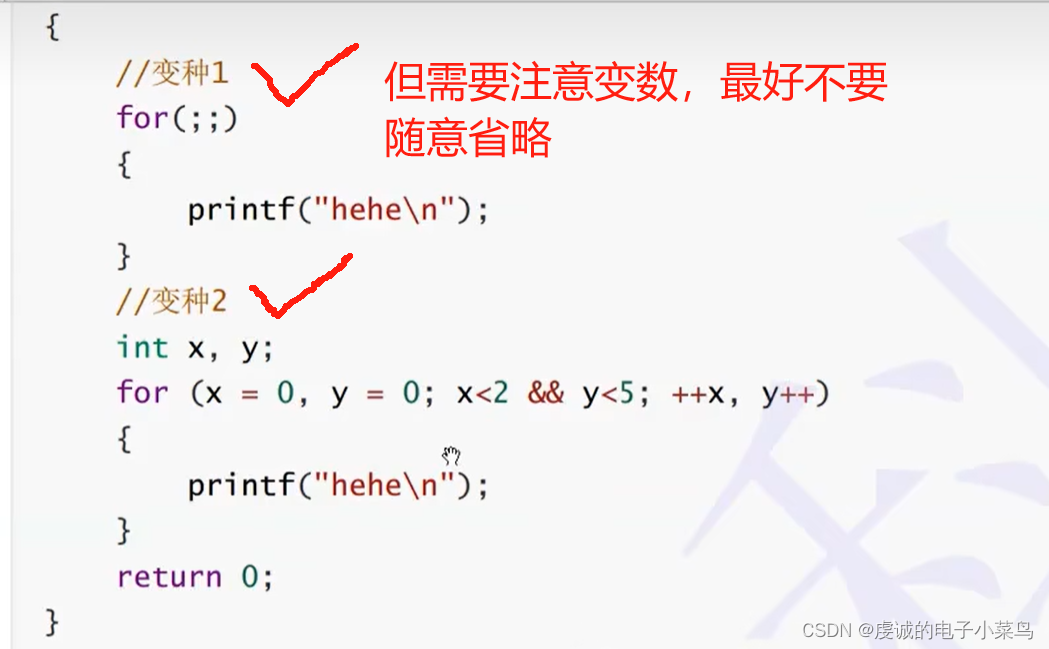
初识C语言——第十九天
for循环 1.简单概述 2.执行流程 3.建议事项:...
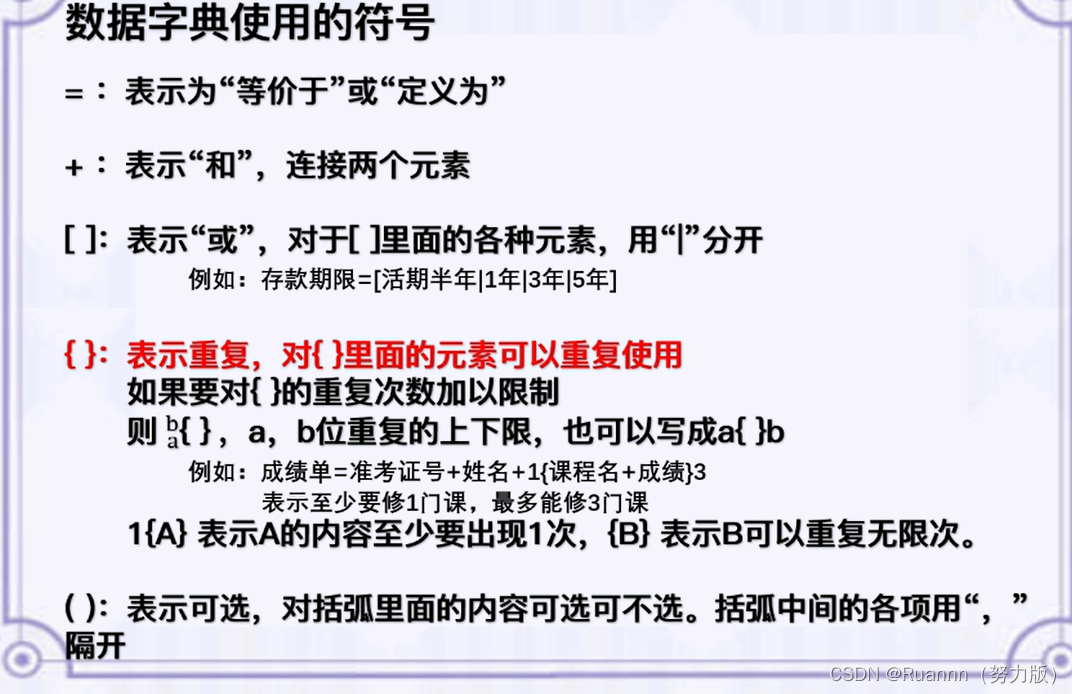
软件需求工程习题
1.(面谈)是需求获取活动中发生的需求工程师和用户间面对面的会见。 2.使用原型法进行需求获取,(演化式)原型必须具有健壮性,代码质量要从一开始就能达到最终系统的要求 3.利用面谈进行需求获取时…...

Win10弹出这个:https://logincdn.msauth.ne
问题描述: Win10脚本错误 Windows10家庭版操作系统开机后弹出这个 https://logincdn.msauth.net/shared/1.0/content/js/ConvergedLogin_PCore_vi321_9jVworKN8EONYo0A2.js 解决方法: 重启计算机后手动关闭第三方安全优化软件,然后在任务管理…...

Vue2 动态路由
VUE CLI 项目 router.js import Vue from "vue"; import Router from "vue-router"; import base from "/view/404/404.vue";const originalPush Router.prototype.push Router.prototype.push function push (location) {return originalPu…...
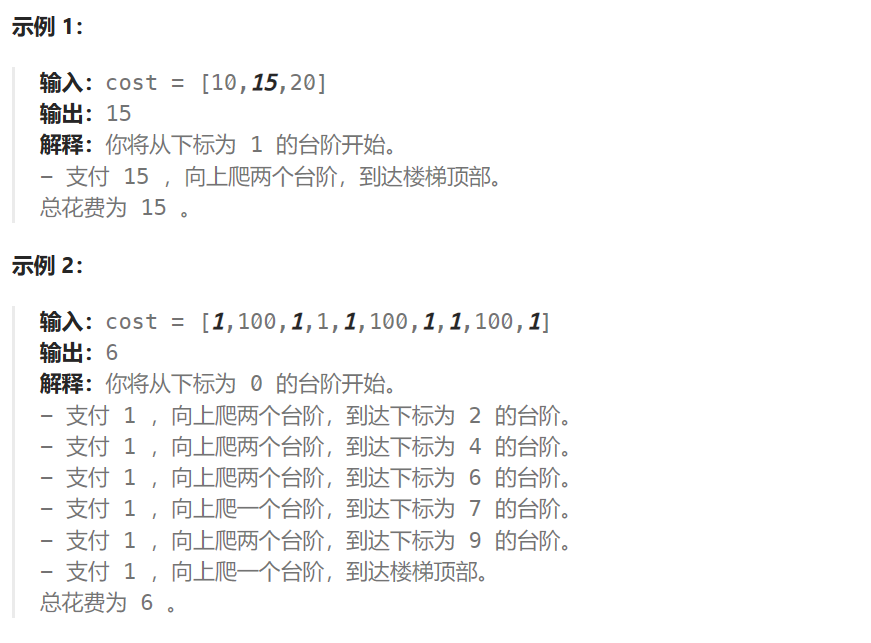
LeetCode746:使用最小花费爬楼梯
题目描述 给你一个整数数组 cost ,其中 cost[i] 是从楼梯第 i 个台阶向上爬需要支付的费用。一旦你支付此费用,即可选择向上爬一个或者两个台阶。 你可以选择从下标为 0 或下标为 1 的台阶开始爬楼梯。 请你计算并返回达到楼梯顶部的最低花费。 代码 …...
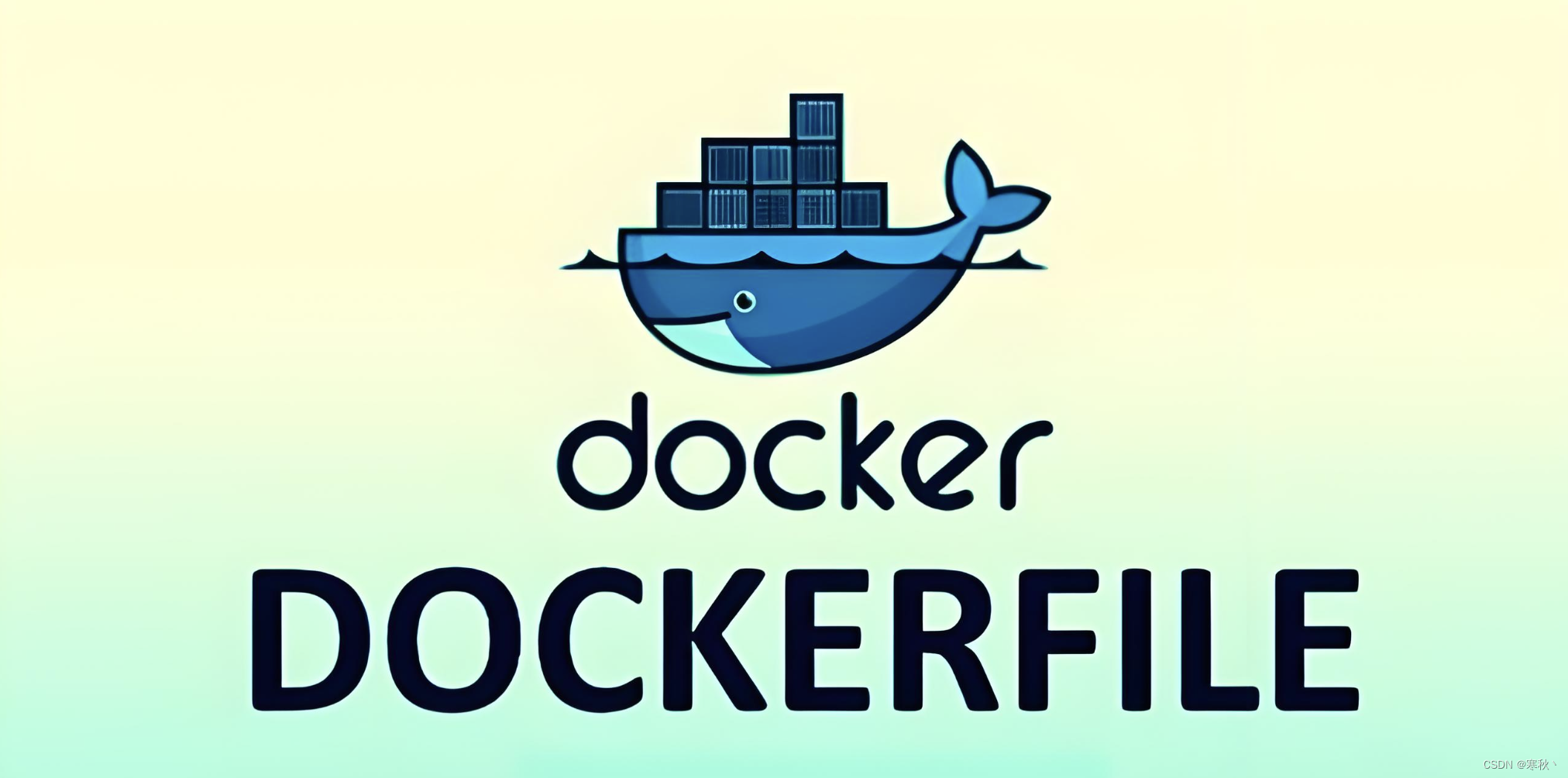
DockerFile介绍与使用
一、DockerFile介绍 大家好,今天给大家分享一下关于 DockerFile 的介绍与使用,DockerFile 是一个用于定义如何构建 Docker 镜像的文本文件,具体来说,具有以下重要作用: 标准化构建:提供了一种统一、可重复…...

Java基础知识(六) 字符串
六 字符串 6.1 String字符串 1、String类对象创建 定义String类对象格式:** 1)String 字符串变量名“字符串常量”; 2)String 字符串变量名new String(字符串常量); 3)String 字符串变量名; 字符串变量名“字符串常…...
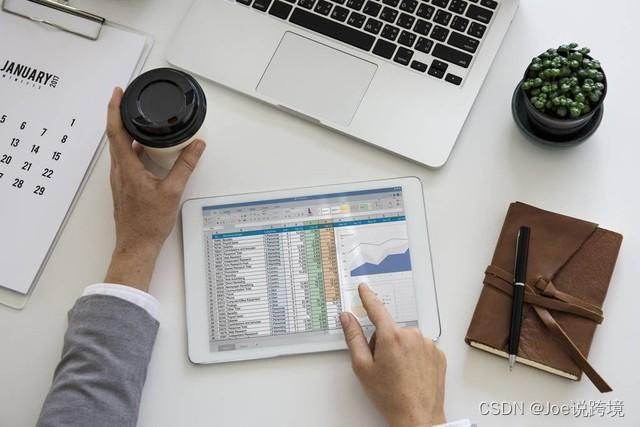
为什么跨境电商大佬都在自养号测评?看完你就懂了!
在跨境电商的激烈竞争中,各大平台如亚马逊、拼多多Temu、shopee、Lazada、wish、速卖通、煤炉、敦煌、独立站、雅虎、eBay、TikTok、Newegg、Allegro、乐天、美客多、阿里国际、沃尔玛、Nike、OZON、Target以及Joom等,纷纷成为商家们竞相角逐市场份额的焦…...

AtCoder Beginner Contest 353
A 题意:检查是否有比第一个数大的数 #include<bits/stdc.h>using namespace std;int main() {int n;cin>>n;int a;cin>>a;int f0;for(int i2;i<n;i){int k;cin>>k;if(k>a){cout<<i<<endl;f1;break;}}if(f0){cout<&l…...
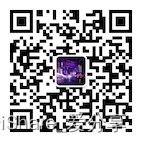
深度解读《深度探索C++对象模型》之虚继承的实现分析和效率评测(一)
目录 前言 具有虚基类的对象的构造过程 通过子类的对象存取虚基类成员的实现分析 接下来我将持续更新“深度解读《深度探索C对象模型》”系列,敬请期待,欢迎左下角点击关注!也可以关注公众号:iShare爱分享,或文章末…...
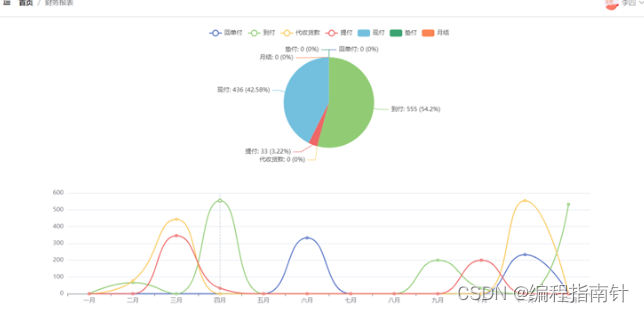
计算机Java项目|Springboot房产销售系统
作者主页:编程指南针 作者简介:Java领域优质创作者、CSDN博客专家 、CSDN内容合伙人、掘金特邀作者、阿里云博客专家、51CTO特邀作者、多年架构师设计经验、腾讯课堂常驻讲师 主要内容:Java项目、Python项目、前端项目、人工智能与大数据、简…...
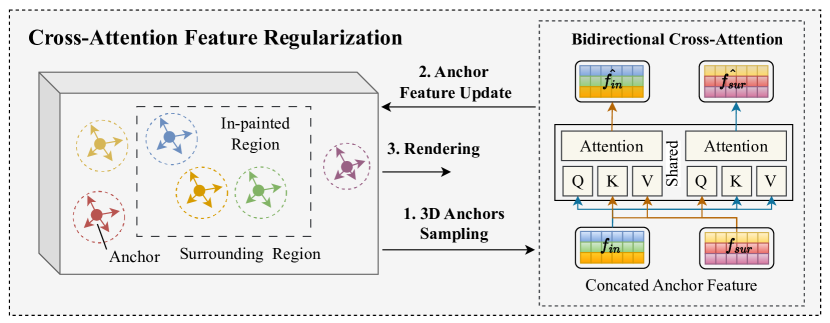
学习3D几何和特征一致的高斯溅射目标去除
earning 3D Geometry and Feature Consistent Gaussian Splatting for Object Removal 学习3D几何和特征一致的高斯溅射目标去除 Yuxin Wang 王玉欣 HKUST &Qianyi Wu Monash University &Guofeng Zhang Zhejiang University &Dan Xu HKUST 香港科技大学&吴倩…...

PHP 使用常量实现枚举类
PHP 使用常量实现枚举类 <?php abstract class Enum {private static $constCacheArray NULL;private static function getConstants() {if (self::$constCacheArray NULL) {self::$constCacheArray [];}$calledClass get_called_class();if (!array_key_exists($call…...

Linux操作系统基础题库
一. 单选题(共2题,40分) 1. (单选题)Linux操作系统自诞生至今,有数十万的程序开发人员参与到了它的开发与完善中,如今Linux已发展成为是一个成熟、稳定的操作系统。从以下选项中选出关于Linux特点描述完全正确的一项。…...
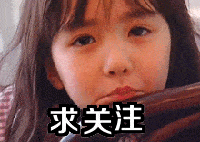
Java抽象类:为何它是你代码架构的基石?
目录 1、抽象类的概念 2、抽象类语法 3、抽象类特性 4、抽象类的作用 5、 完结散花 个人主页:秋风起,再归来~ 文章专栏:javaSE的修炼之路 个人格言:悟已往之不谏,知来者犹可追 克…...

Flutter 中的 ToggleButtons 小部件:全面指南
Flutter 中的 ToggleButtons 小部件:全面指南 在 Flutter 中,ToggleButtons 是一种允许用户在一组选项中进行切换选择的控件。它通常用于展示一组相关选项,让用户可以快速切换选择。ToggleButtons 是一种水平排列的按钮集合,其中…...
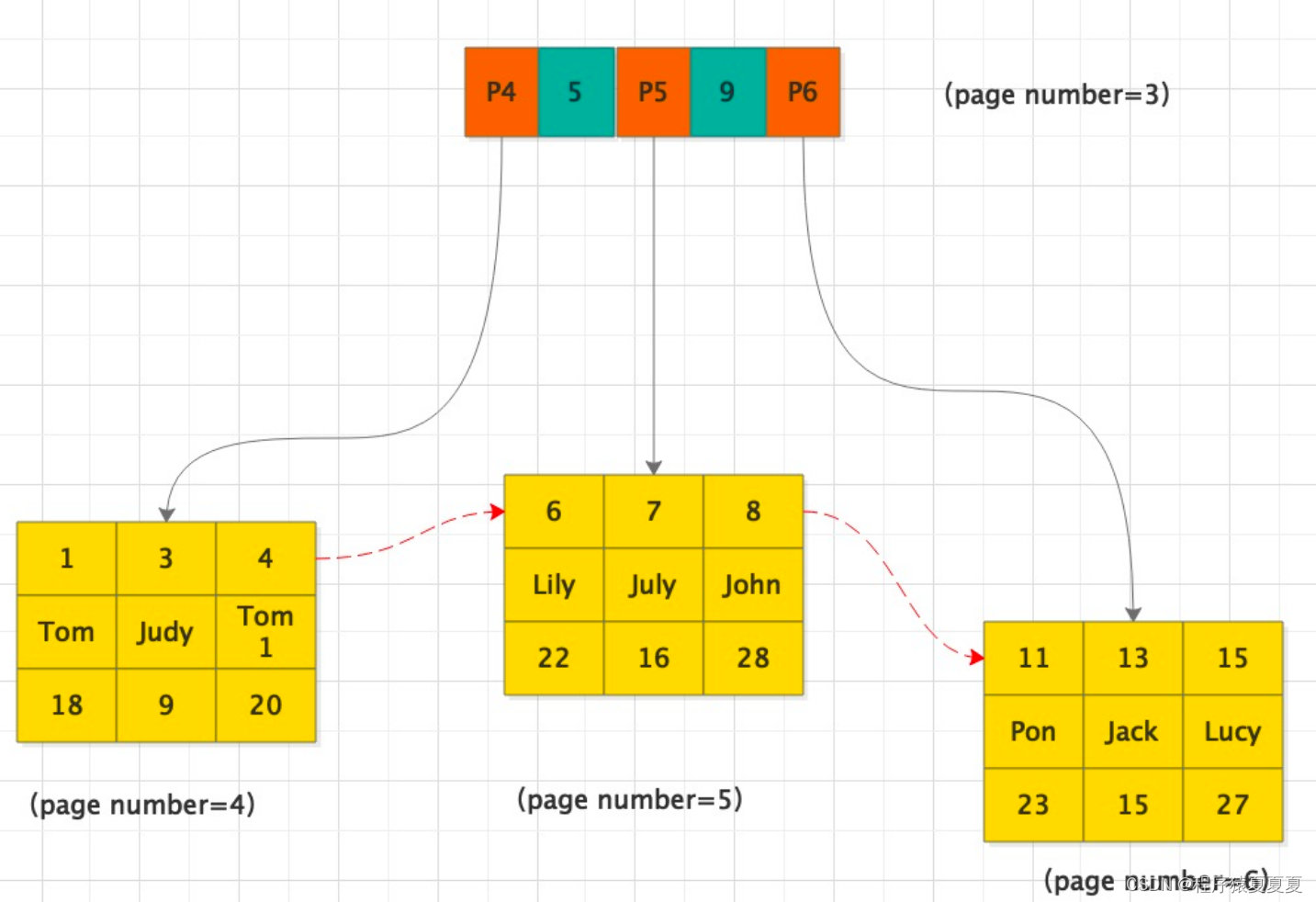
【MYSQL】一颗B+树可以保存多少条数据
引言 事万物都有自己的单元体系,若干个小单体组成一个个大的个体。就像拼乐高一样,可以自由组合。所以说,如果能熟悉最小单元,就意味着我们抓住了事物的本事,再复杂的问题也会迎刃而解。 存储单元 存储器范围比较大…...
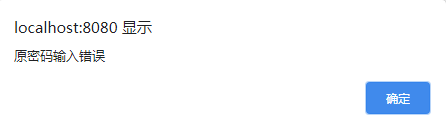
ssm125四六级报名与成绩查询系统+jsp
四六级报名与成绩查询系统的设计与实现 摘 要 互联网发展至今,无论是其理论还是技术都已经成熟,而且它广泛参与在社会中的方方面面。它让信息都可以通过网络传播,搭配信息管理工具可以很好地为人们提供服务。针对四六级报名信息管理混乱&am…...
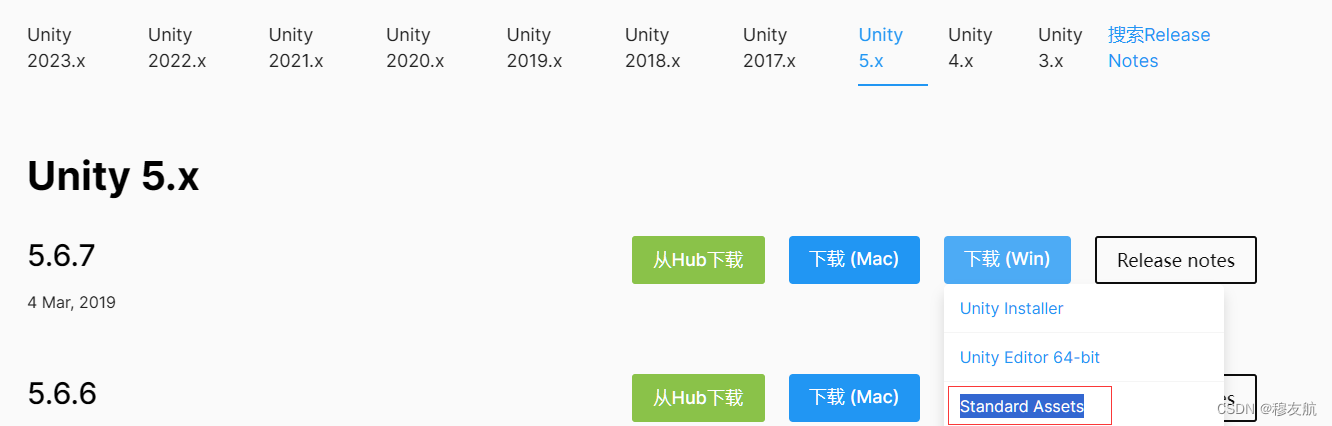
【Unity从零开始学习制作手机游戏】第01节:控制3D胶囊体运动
1. 新建Project L01 使用3D Mobile模板。 2. 建立一个平面,用来承载物体 3. 导入Unity库内的胶囊体 下载 StandardAssets https://download.unitychina.cn/download_unity/e80cc3114ac1/WindowsStandardAssetsInstaller/UnityStandardAssetsSetup-5.6.7f1.exe …...
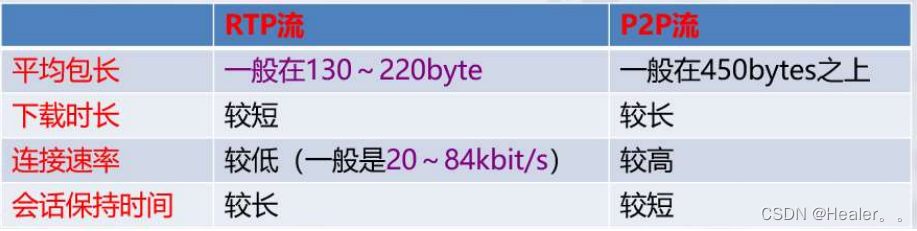
内容安全(DPI和DFI解析)
内容安全前言: 防火墙的本质其实就是包过滤,我们通常所说的安全设备(如:IPS、IDS、AV、WAF)的检测重心是应用层。下一代防火墙基于传统防火墙的拓展能力,就是可以将以上的安全设备模块集成在一起࿰…...