比较(一)利用python绘制条形图
比较(一)利用python绘制条形图
条形图(Barplot)简介
条形图主要用来比较不同类别间的数据差异,一条轴表示类别,另一条则表示对应的数值度量。
快速绘制
-
基于seaborn
import seaborn as sns import matplotlib.pyplot as plt# 导入数据 tips = sns.load_dataset("tips")# 利用barplot函数快速绘制 sns.barplot(x="total_bill", y="day", data=tips, estimator=sum, errorbar=None, color='#69b3a2')plt.show()
-
基于matplotlib
import matplotlib.pyplot as plt# 导入数据 tips = sns.load_dataset("tips") grouped_tips = tips.groupby('day')['total_bill'].sum().reset_index()# 利用bar函数快速绘制 plt.bar(grouped_tips.day, grouped_tips.total_bill)plt.show()
-
基于pandas
import matplotlib.pyplot as plt import pandas as pd# 导入数据 tips = sns.load_dataset("tips") grouped_tips = tips.groupby('day')['total_bill'].sum().reset_index()# 利用plot.bar函数快速绘制 grouped_tips.plot.bar(x='day', y='total_bill', rot=0)plt.show()
定制多样化的条形图
自定义条形图一般是结合使用场景对相关参数进行修改,并辅以其他的绘图知识。参数信息可以通过官网进行查看,其他的绘图知识则更多来源于实战经验,大家不妨将接下来的绘图作为一种学习经验,以便于日后总结。
通过seaborn绘制多样化的条形图
seaborn主要利用barplot
绘制条形图,可以通过seaborn.barplot了解更多用法
-
修改参数
import seaborn as sns import matplotlib.pyplot as plt import numpy as npsns.set(font='SimHei', font_scale=0.8, style="darkgrid") # 解决Seaborn中文显示问题# 导入数据 tips = sns.load_dataset("tips")# 构造子图 fig, ax = plt.subplots(2,2,constrained_layout=True, figsize=(8, 8))# 修改方向-垂直 ax_sub = sns.barplot(y="total_bill", x="day", data=tips, estimator=sum, errorbar=None, color='#69b3a2',ax=ax[0][0]) ax_sub.set_title('垂直条形图')# 自定义排序 ax_sub = sns.barplot(y="total_bill", x="day", data=tips, estimator=sum, errorbar=None, color='#69b3a2',order=["Fri","Thur","Sat","Sun"],ax=ax[0][1]) ax_sub.set_title('自定义排序')# 数值排序 df = tips.groupby('day')['total_bill'].sum().sort_values(ascending=False).reset_index() ax_sub = sns.barplot(y="day", x="total_bill", data=df, errorbar=None, color='#69b3a2',order=df['day'],ax=ax[1][0]) ax_sub.set_title('数值排序')# 添加误差线 ax_sub = sns.barplot(x="day", y="total_bill", data=tips, estimator=np.mean, errorbar=('ci', 85), capsize=.2, color='lightblue',ax=ax[1][1]) ax_sub.set_title('添加误差线')plt.show()
-
分组条形图
import seaborn as sns import matplotlib.pyplot as plt import numpy as npsns.set(style="darkgrid")# 导入数据 tips = sns.load_dataset("tips")fig, ax = plt.subplots(figsize=(4, 4))# 分组条形图 colors = ["#69b3a2", "#4374B3"] sns.barplot(x="day", y="total_bill", hue="smoker", data=tips, errorbar=None, palette=colors)plt.show()# 分组/子分组条形图 sns.catplot(x="sex", y="total_bill", hue="smoker", col="day", data=tips, kind="bar", height=4, aspect=.7)plt.show()
-
引申-数量堆积条形图
import seaborn as sns import numpy as np import matplotlib.pyplot as plt import matplotlib.patches as mpatchessns.set(style="darkgrid")# 导入数据 tips = sns.load_dataset("tips") df = tips.groupby(['day', 'smoker'])['total_bill'].sum().reset_index() smoker_df = df[df['smoker']=='Yes'] non_smoker_df = df[df['smoker']=='No']# 布局 plt.figure(figsize=(6, 4))# 非吸烟者的条形图 bar1 = sns.barplot(x='day', y='total_bill', data=non_smoker_df, color='lightblue') # 吸烟者的条形图,底部开始位置设置为非吸烟者的total_bill值(即吸烟者条形图在上面) bar2 = sns.barplot(x='day', y='total_bill', bottom=non_smoker_df['total_bill'], data=smoker_df, color='darkblue')# 图例 top_bar = mpatches.Patch(color='darkblue', label='smoker = Yes') bottom_bar = mpatches.Patch(color='lightblue', label='smoker = No') plt.legend(handles=[top_bar, bottom_bar])plt.show()
-
引申-百分比堆积条形图
import seaborn as sns import matplotlib.pyplot as plt import pandas as pd# 导入数据 tips = sns.load_dataset("tips")# 计算百分比 day_total_bill = tips.groupby('day')['total_bill'].sum() # 每日数据 group_total_bill = tips.groupby(['day', 'smoker'])['total_bill'].sum().reset_index() # 每日每组数据 group_total_bill['percent'] = group_total_bill.apply(lambda row: row['total_bill'] / day_total_bill[row['day']] * 100, axis=1)# 将数据分成smoker和non-smoker两份,方便我们绘制两个条形图 smoker_df = group_total_bill[group_total_bill['smoker'] == 'Yes'] non_smoker_df = group_total_bill[group_total_bill['smoker'] == 'No']# 布局 plt.figure(figsize=(6, 4))# 非吸烟者的条形图 bar1 = sns.barplot(x='day', y='percent', data=non_smoker_df, color='lightblue') # 吸烟者的条形图,底部开始位置设置为非吸烟者的total_bill值(即吸烟者条形图在上面) bar2 = sns.barplot(x='day', y='percent', bottom=non_smoker_df['percent'], data=smoker_df, color='darkblue')# 图例 top_bar = mpatches.Patch(color='darkblue', label='smoker = Yes') bottom_bar = mpatches.Patch(color='lightblue', label='smoker = No') plt.legend(handles=[top_bar, bottom_bar])plt.show()
通过seaborn绘制多样化的条形图
seaborn主要利用barh
绘制条形图,可以通过matplotlib.pyplot.barh了解更多用法
-
修改参数
import matplotlib as mpl import matplotlib.pyplot as plt import numpy as np import pandas as pdmpl.rcParams.update(mpl.rcParamsDefault) # 恢复默认的matplotlib样式 plt.rcParams['font.sans-serif'] = ['SimHei'] # 用来正常显示中文标签# 自定义数据 height = [3, 12, 5, 18, 45] bars = ('A', 'B', 'C', 'D', 'E') y_pos = np.arange(len(bars)) x_pos = np.arange(len(bars))# 初始化布局 fig = plt.figure(figsize=(8,8))# 水平方向-水平条形图 plt.subplot(3, 3, 1) plt.barh(y_pos, height) plt.yticks(y_pos, bars) plt.title('水平条形图')# 指定顺序 height_order, bars_order = zip(*sorted(zip(height, bars), reverse=False)) # 自定义顺序plt.subplot(3, 3, 2) plt.barh(y_pos, height_order) plt.yticks(y_pos, bars_order) plt.title('指定顺序')# 自定义颜色 plt.subplot(3, 3, 3) plt.bar(x_pos, height, color=['black', 'red', 'green', 'blue', 'cyan']) plt.xticks(x_pos, bars) plt.title('自定义颜色')# 自定义颜色-边框颜色 plt.subplot(3, 3, 4) plt.bar(x_pos, height, color=(0.1, 0.1, 0.1, 0.1), edgecolor='blue') plt.xticks(x_pos, bars) plt.title('自定义边框颜色')# 控制距离 width = [0.1,0.2,3,1.5,0.3] x_pos_width = [0,0.3,2,4.5,5.5]plt.subplot(3, 3, 5) plt.bar(x_pos_width, height, width=width) plt.xticks(x_pos_width, bars) plt.title('控制距离')# 控制宽度 x_pos_space = [0,1,5,8,9]plt.subplot(3, 3, 6) plt.bar(x_pos_space, height) plt.xticks(x_pos_space, bars) plt.title('控制宽度')# 自定义布局 plt.subplot(3, 3, 7) plt.bar(x_pos, height) plt.xticks(x_pos, bars, color='orange', rotation=90) # 自定义x刻度名称颜色,自定义旋转 plt.xlabel('category', fontweight='bold', color = 'orange', fontsize='18') # 自定义x标签 plt.yticks(color='orange') # 自定义y刻度名称颜色plt.title('自定义布局')# 添加误差线 err = [val * 0.1 for val in height] # 计算误差(这里假设误差为height的10%)plt.subplot(3, 3, 8) plt.bar(x_pos, height, yerr=err, alpha=0.5, ecolor='black', capsize=10) plt.xticks(x_pos, bars) plt.title('添加误差线')# 增加数值文本信息 plt.subplot(3, 3, 9) ax = plt.bar(x_pos, height) for bar in ax:yval = bar.get_height()plt.text(bar.get_x() + bar.get_width()/2.0, yval, int(yval), va='bottom') # va参数代表垂直对齐方式 plt.xticks(x_pos, bars) plt.title('增加数值文本信息')fig.tight_layout() # 自动调整间距 plt.show()
-
分组条形图
import numpy as np import matplotlib.pyplot as plt# 宽度设置 barWidth = 0.25# 自定义数据 bars1 = [12, 30, 1, 8, 22] bars2 = [28, 6, 16, 5, 10] bars3 = [29, 3, 24, 25, 17]# x位置 r1 = np.arange(len(bars1)) r2 = [x + barWidth for x in r1] r3 = [x + barWidth for x in r2]# 绘制分组条形图 plt.bar(r1, bars1, color='#7f6d5f', width=barWidth, edgecolor='white', label='g1') plt.bar(r2, bars2, color='#557f2d', width=barWidth, edgecolor='white', label='g2') plt.bar(r3, bars3, color='#2d7f5e', width=barWidth, edgecolor='white', label='g3')# 轴标签、图例 plt.xlabel('group', fontweight='bold') plt.xticks([r + barWidth for r in range(len(bars1))], ['A', 'B', 'C', 'D', 'E']) plt.legend()plt.show()
-
数量堆积条形图
import numpy as np import matplotlib.pyplot as plt import pandas as pd# 自定义数据 bars1 = [12, 28, 1, 8, 22] bars2 = [28, 7, 16, 4, 10] bars3 = [25, 3, 23, 25, 17]# bars1 + bars2的高度 bars = np.add(bars1, bars2).tolist()# x位置 r = [0,1,2,3,4]# bar名称、宽度 names = ['A','B','C','D','E'] barWidth = 1# 底部bar plt.bar(r, bars1, color='#7f6d5f', edgecolor='white', width=barWidth, label="g1") # 中间bar plt.bar(r, bars2, bottom=bars1, color='#557f2d', edgecolor='white', width=barWidth, label="g2") # 顶部bar plt.bar(r, bars3, bottom=bars, color='#2d7f5e', edgecolor='white', width=barWidth, label="g3")# x轴设置、图例 plt.xticks(r, names, fontweight='bold') plt.xlabel("group") plt.legend()plt.show()
-
百分比堆积条形图
import numpy as np import matplotlib.pyplot as plt import pandas as pd# 自定义数据 r = [0,1,2,3,4] # x位置 raw_data = {'greenBars': [20, 1.5, 7, 10, 5], 'orangeBars': [5, 15, 5, 10, 15],'blueBars': [2, 15, 18, 5, 10]} df = pd.DataFrame(raw_data)# 转为百分比 totals = [i+j+k for i,j,k in zip(df['greenBars'], df['orangeBars'], df['blueBars'])] greenBars = [i / j * 100 for i,j in zip(df['greenBars'], totals)] orangeBars = [i / j * 100 for i,j in zip(df['orangeBars'], totals)] blueBars = [i / j * 100 for i,j in zip(df['blueBars'], totals)]# bar名称、宽度 barWidth = 0.85 names = ('A','B','C','D','E')# 底部bar plt.bar(r, greenBars, color='#b5ffb9', edgecolor='white', width=barWidth, label="g1") # 中间bar plt.bar(r, orangeBars, bottom=greenBars, color='#f9bc86', edgecolor='white', width=barWidth, label="g2") # 顶部bar plt.bar(r, blueBars, bottom=[i+j for i,j in zip(greenBars, orangeBars)], color='#a3acff', edgecolor='white', width=barWidth, label="g3")# x轴、图例 plt.xticks(r, names) plt.xlabel("group") plt.legend()plt.show()
通过pandas绘制多样化的条形图
pandas主要利用barh
绘制条形图,可以通过pandas.DataFrame.plot.barh了解更多用法
-
修改参数
import matplotlib as mpl import matplotlib.pyplot as plt import numpy as np import pandas as pdplt.rcParams['font.sans-serif'] = ['SimHei'] # 用来正常显示中文标签# 自定义数据 category = ['Group1']*30 + ['Group2']*50 + ['Group3']*20 df = pd.DataFrame({'category': category}) values = df['category'].value_counts()# 初始化布局 fig = plt.figure(figsize=(8,4))# 水平方向-水平条形图 plt.subplot(1, 2, 1) values.plot.barh(grid=True) plt.title('水平条形图')# 自定义顺序、颜色 # 指定顺序 desired_order = ['Group1', 'Group2', 'Group3'] values_order = values.reindex(desired_order) # 指定颜色 colors = ['#69b3a2', '#cb1dd1', 'palegreen']plt.subplot(1, 2, 2) values.plot.bar(color=colors,grid=True, ) plt.title('自定义顺序、颜色')fig.tight_layout() # 自动调整间距 plt.show()
-
分组条形图
import pandas as pd import matplotlib.pyplot as plt# 自定义数据 data = {"Product": ["Product A", "Product A", "Product A", "Product B", "Product B", "Product B"],"Segment": ["Segment 1", "Segment 2", "Segment 3", "Segment 1", "Segment 2", "Segment 3"],"Amount_sold": [100, 120, 120, 80, 160, 150] }df = pd.DataFrame(data) pivot_df = df.pivot(index='Segment',columns='Product',values='Amount_sold')# 分组条形图 pivot_df.plot.bar(grid=True)plt.show()
-
数量堆积条形图
import pandas as pd import matplotlib.pyplot as plt# 自定义数据 data = {"Product": ["Product A", "Product A", "Product A", "Product B", "Product B", "Product B"],"Segment": ["Segment 1", "Segment 2", "Segment 3", "Segment 1", "Segment 2", "Segment 3"],"Amount_sold": [100, 120, 120, 80, 160, 150] }df = pd.DataFrame(data) pivot_df = df.pivot(index='Segment',columns='Product',values='Amount_sold')# 堆积条形图 pivot_df.plot.bar(stacked=True,grid=True)plt.show()
-
百分比堆积条形图
import pandas as pd import matplotlib.pyplot as plt# 自定义数据 data = {"Product": ["Product A", "Product A", "Product A", "Product B", "Product B", "Product B"],"Segment": ["Segment 1", "Segment 2", "Segment 3", "Segment 1", "Segment 2", "Segment 3"],"Amount_sold": [100, 120, 120, 80, 160, 150] }df = pd.DataFrame(data) pivot_df = df.pivot(index='Segment',columns='Product',values='Amount_sold') pivot_df_percentage = pivot_df.div(pivot_df.sum(axis=1), axis=0) * 100# 百分比堆积条形图 pivot_df_percentage.plot.bar(stacked=True,grid=True)# 图例 plt.legend(bbox_to_anchor=(1.04, 1),loc='upper left') plt.show()
总结
以上通过seaborn的barplot
、matplotlib的bar
和pandas的bar
快速绘制条形图,并通过修改参数或者辅以其他绘图知识自定义各种各样的条形图来适应相关使用场景。
共勉~
相关文章:
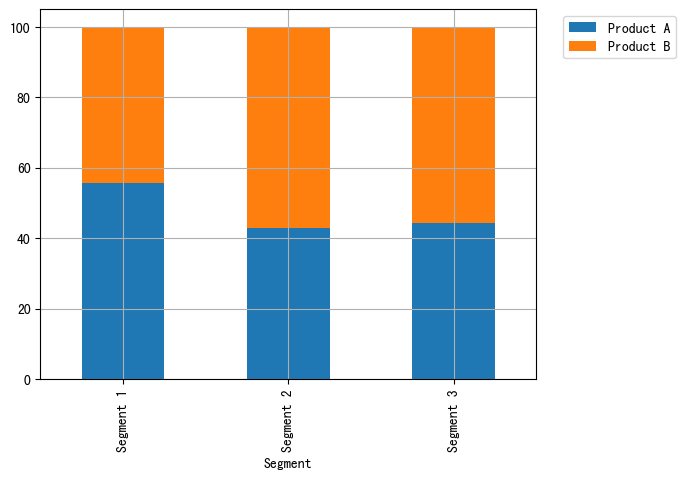
比较(一)利用python绘制条形图
比较(一)利用python绘制条形图 条形图(Barplot)简介 条形图主要用来比较不同类别间的数据差异,一条轴表示类别,另一条则表示对应的数值度量。 快速绘制 基于seaborn import seaborn as sns import matplo…...
【面试】Oracle JDK和Open JDK什么关系?
目录 1. 起源与发展2. 代码与许可3. 功能与组件4. 使用场景5. 版本更新与支持 1. 起源与发展 1.Oracle JDK是由Oracle公司基于Open JDK源代码开发的商业版本。2.Open JDK是java语言的一个开源实现。 2. 代码与许可 1.Oracle JDK包含了闭源组件,并根据二进制代码许…...
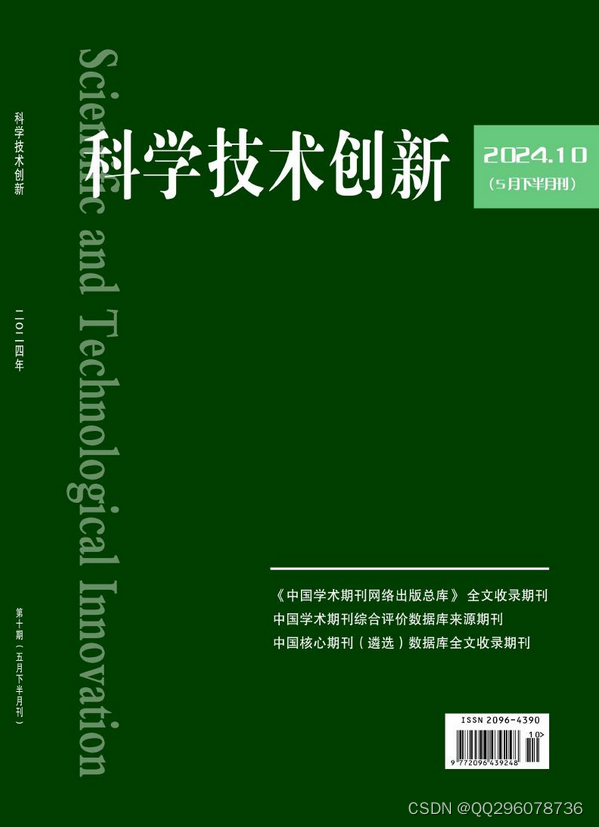
科学技术创新杂志科学技术创新杂志社科学技术创新编辑部2024年第10期目录
科技创新 单桩穿越岩溶发育地层力学特征与溶洞处置措施研究 刘飞; 1-7《科学技术创新》投稿:cnqikantg126.com 基于多目标优化的中低压配电网电力规划研究 向星山;杨承俊;张寒月; 8-11 激光雷达测绘技术在工程测绘中的应用研究 张军伟;闫宏昌; 12-15 …...
ES数据导出成csv文件
推荐使用es2csv 工具。 命令行实用程序,用Python编写,用于用Lucene查询语法或查询DSL语法查询Elasticsearch,并将结果作为文档导出到CSV文件中。该工具可以在多个索引中查询批量文档,并且只获取选定的字段,这减少了查…...
结构型设计模式之装饰模式
文章目录 概述装饰模式原理代码案例小结 概述 装饰模式(decorator pattern) 的原始定义是:动态的给一个对象添加一些额外的职责. 就扩展功能而言,装饰器模式提供了一种比使用子类更加灵活的替代方案。 装饰模式原理 装饰模式中的角色: 抽象构件角色 …...
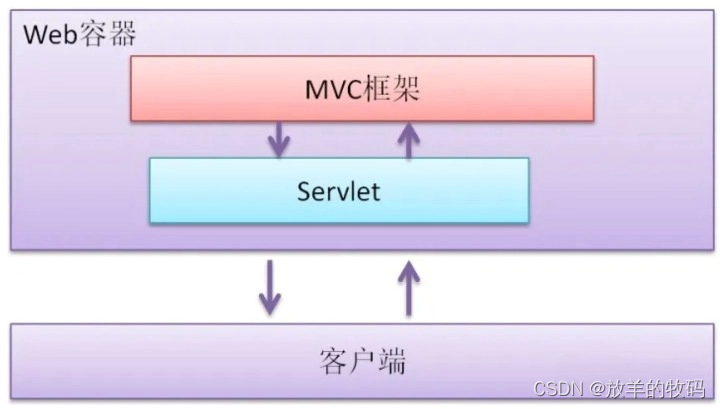
Java - 当年很流行,现在已经淘汰的 Java 技术,请不要在继续学了!!!
最近这段时间收到了一些读者的私信,问我某个技术要不要学,还有一些在国外的同学竟然对 Java 图形化很感兴趣,还想找这方面的工作。 比较忙,一直没抽出时间去回答这类问题,刚好看到我关注的一位大佬回答过,这…...
驻波比VSWR
最近看大家写的VSWR文章,发现有很多误解, 1)错误解释是入射波和反射波叠加的驻波的波峰/波谷。大家可以向下驻波也是正弦波,波峰和波谷的值不都是振幅吗?因此相当于VSWR恒等于1了。 2)VSWR越小越好; 正确…...
多线程-线程池
为什么要使用线程池 在Java中使用线程池的主要原因有以下几点: 提高性能:使用线程池可以减少线程的创建和销毁过程的开销。线程的创建和销毁是比较昂贵的操作,如果每次需要执行任务时都创建一个新线程,会造成系统资源的浪费。而线…...
护网期间遇到的几个上传bypass waf、edr
1. weblogic部署war的时候 http/1.1 改成http/2绕过waf 其实jar和ear部署应该也可以,但是我没成功。 weblogoic 部署war死活出错,用linux下的浏览器 linux下打包war马 zip -r zipjob4.zip job/ mv zipjob3.zip zipjob3.war 然后部署成功之后&am…...
简述MVC模式
这里为什么讲MVC模式,是因为在学习的过程中,很多人不知怎的,将观察者模式和MVC混为一谈。MVC模式最开始出现在WEB开发中,该模式能够很好的做到软件模块的高内聚,低耦合,所以其思想逐渐在各个软件开发领域都…...
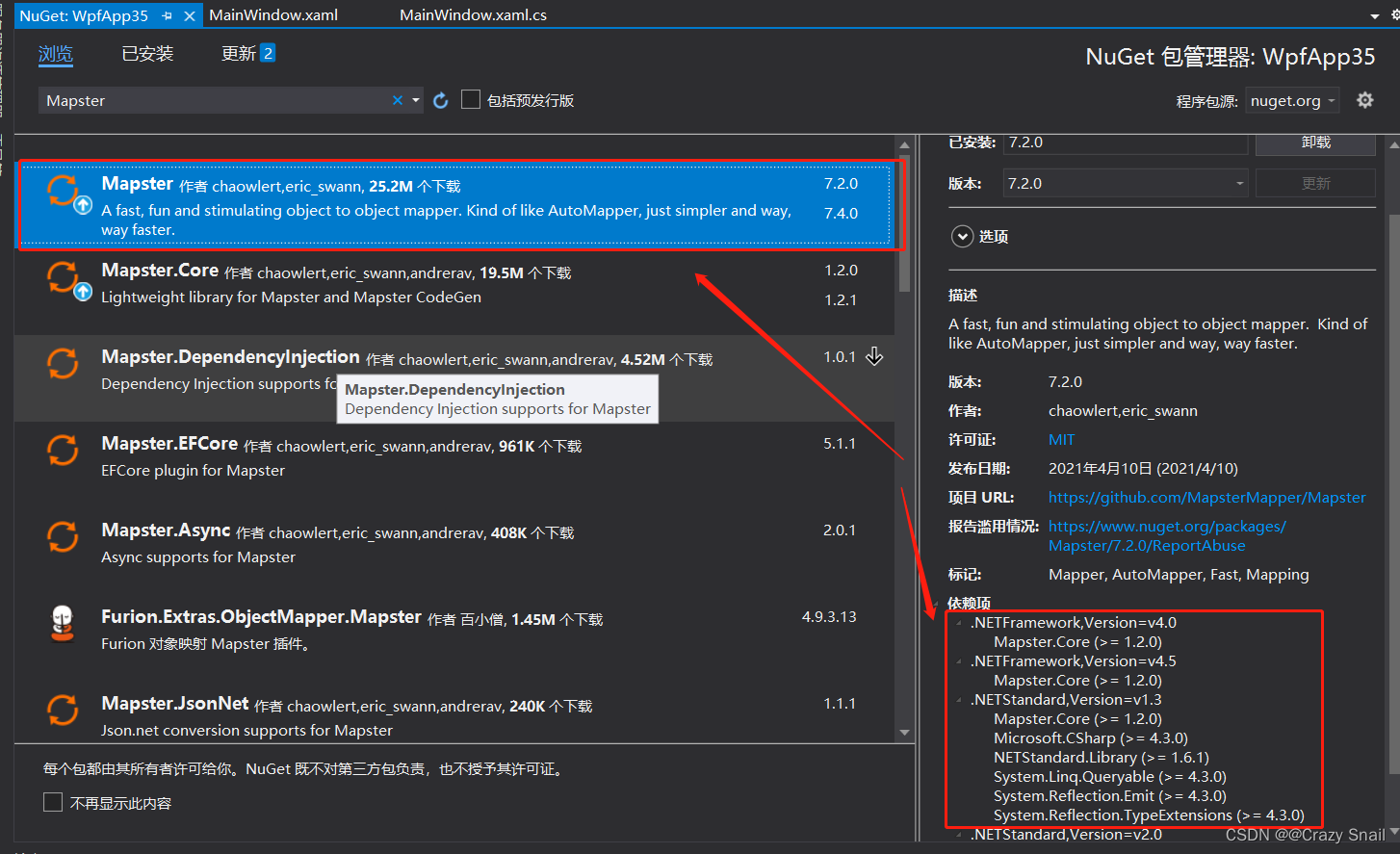
C#--Mapster(高性能映射)用法
1.Nuget安装Mapster包引用 2.界面XAML部分 <Window x:Class"WpfApp35.MainWindow"xmlns"http://schemas.microsoft.com/winfx/2006/xaml/presentation"xmlns:x"http://schemas.microsoft.com/winfx/2006/xaml"xmlns:d"http://schemas.m…...
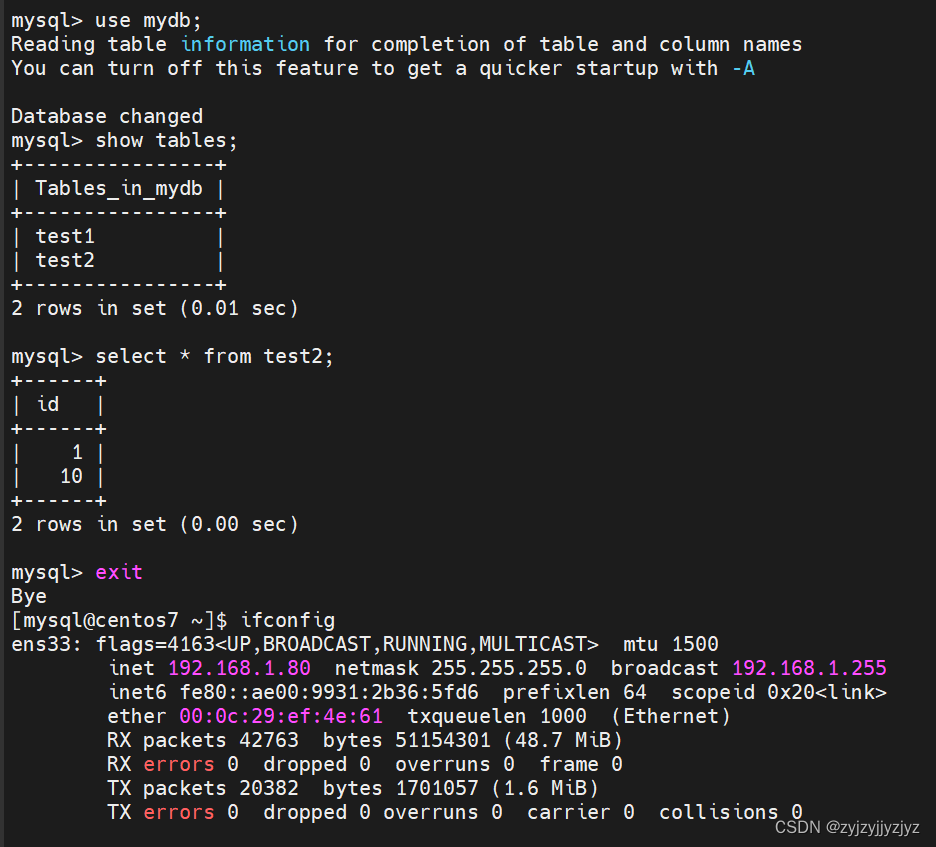
mysql实战——Mysql8.0高可用之双主+keepalived
一、介绍 利用keepalived实现Mysql数据库的高可用,KeepalivedMysql双主来实现MYSQL-HA,两台Mysql数据库的数据保持完全一致,实现方法是两台Mysql互为主从关系,通过keepalived配置VIP,实现当其中的一台Mysql数据库宕机…...
关于同一个地址用作两个不同页面时,列表操作栏按钮混淆状态
同一个地址用作两个不同页面时,列表页的操作栏中有好多个按钮,如果用了v-if,可能会导致按钮混淆状态如disabled等属性混乱 解决方法1: 将v-if换成v-show,用了v-show之后意味着所有按钮都在只是在页面上隐藏了 解决方…...
Oracle段延迟分配(Deferred Segment Creation)解析
目录 一、基本概念二、工作原理三、优势四、潜在风险与注意事项五、配置与管理 Oracle段延迟分配(Deferred Segment Creation)是Oracle 11g引入的一项重要特性,旨在优化资源使用和提高数据库管理效率。 一、基本概念 段延迟分配意味着当创建…...
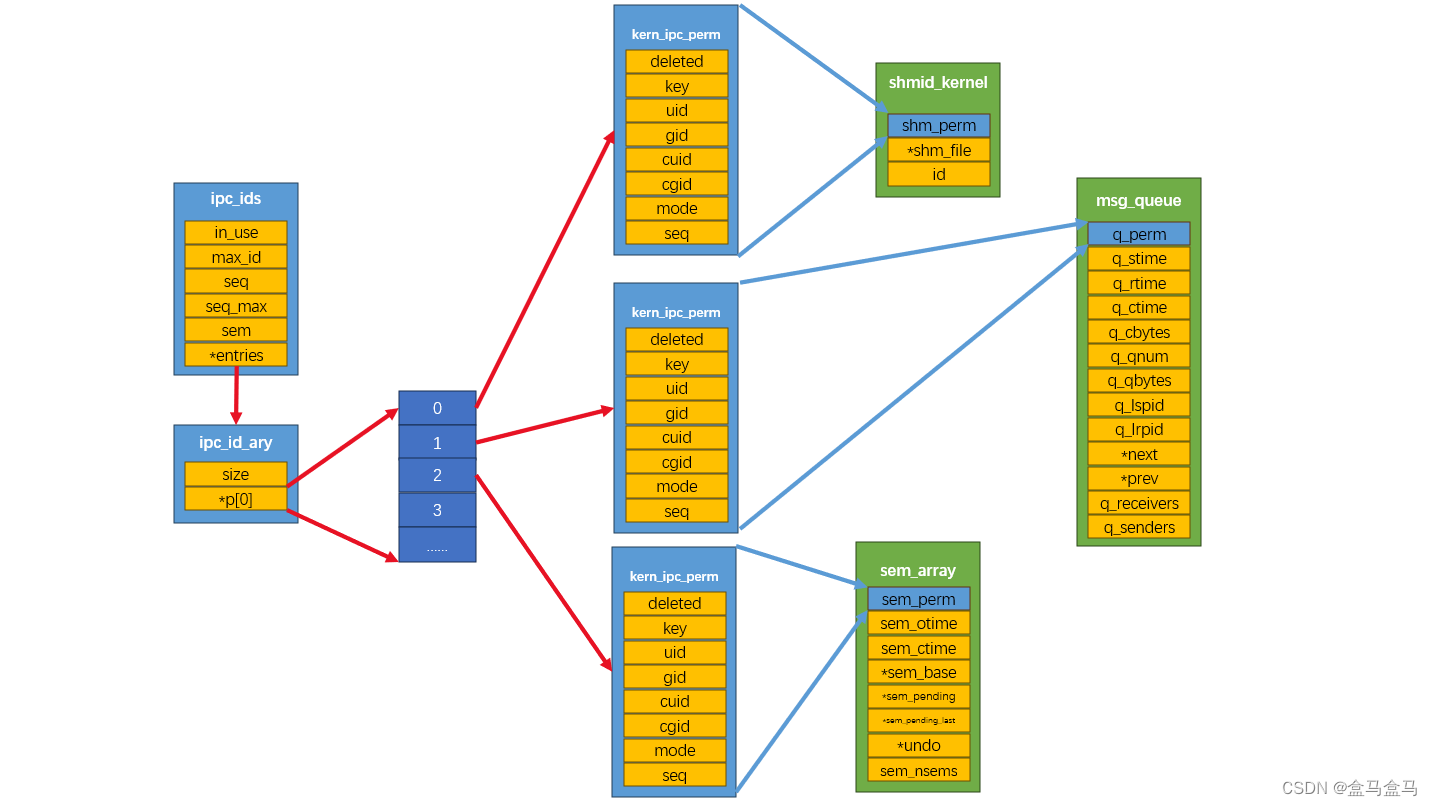
Linux:IPC - System V
Linux:IPC - System V 共享内存 shm创建共享内存shmgetshmctlftok 挂接共享内存shmatshmdt shm特性 消息队列 msgmsggetmsgctlmsgsndmsgrcv 信号量 semSystem V 管理机制 System V IPC 是Linux系统中一种重要的进程间通信机制,它主要包括共享内存 shm&am…...
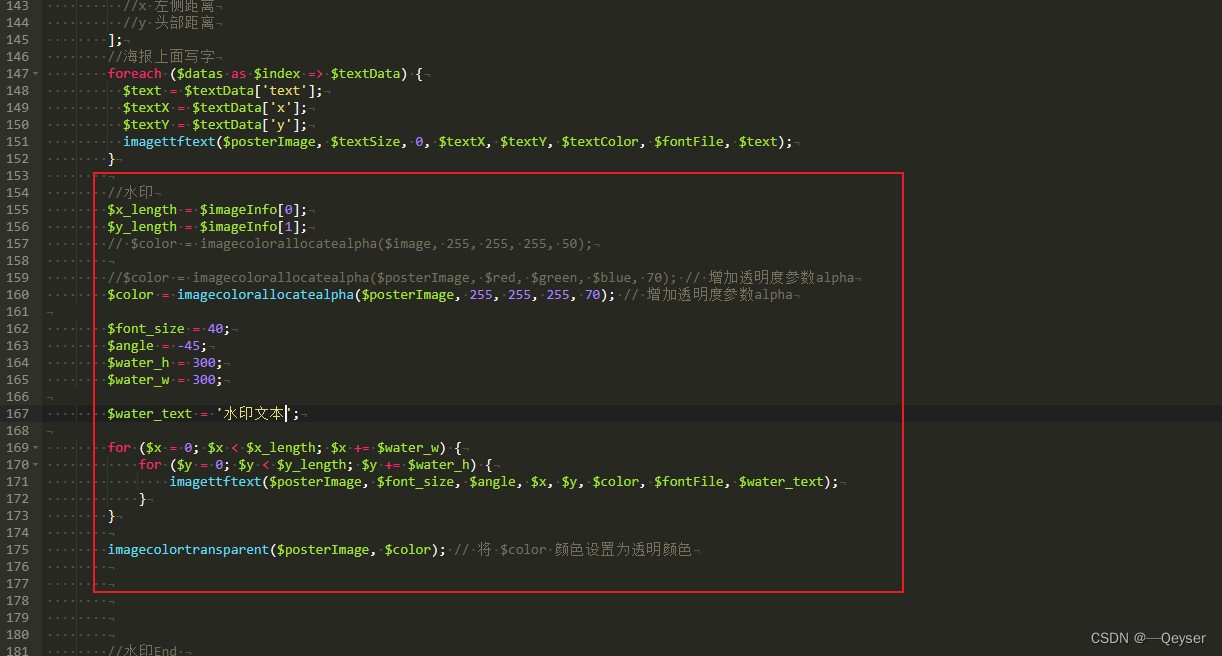
Laravel 图片添加水印
和这个配合使用 Laravel ThinkPhP 海报生成_laravel 制作海报-CSDN博客 代码 //水印 $x_length $imageInfo[0]; $y_length $imageInfo[1];$color imagecolorallocatealpha($posterImage, 255, 255, 255, 70); // 增加透明度参数alpha$font_size 40; //字体大小 $angle …...
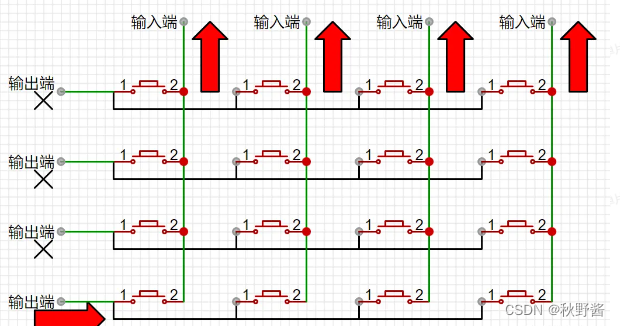
嵌入式进阶——矩阵键盘
🎬 秋野酱:《个人主页》 🔥 个人专栏:《Java专栏》《Python专栏》 ⛺️心若有所向往,何惧道阻且长 文章目录 矩阵按键原理图按键状态检测单行按键状态检测多行按键状态检测 状态记录状态优化循环优化 矩阵按键 矩阵键盘是一种常见的数字输入…...
请说出vue.cli项目中src目录每个文件夹和文件的用法
在Vue CLI项目中,src目录是存放项目源码及需要引用的资源文件的主要位置。以下是src目录下常见文件夹和文件的用法: components 用途:存放可重用的Vue组件。这些组件通常用于在多个页面或布局中共享UI和功能。特点:组件应该是模块…...
【MySQL精通之路】InnoDB磁盘I/O和文件空间管理(11)
主博客: 【MySQL精通之路】InnoDB存储引擎-CSDN博客 目录 1.InnoDB磁盘I/O 1.1 预读 1.2 双写缓冲区 2.文件空间管理 2.1 Pages, Extents, Segments, and Tablespaces(很重要) 2.2 配置保留文件段页面的百分比 2.3 页与表行的关系 …...
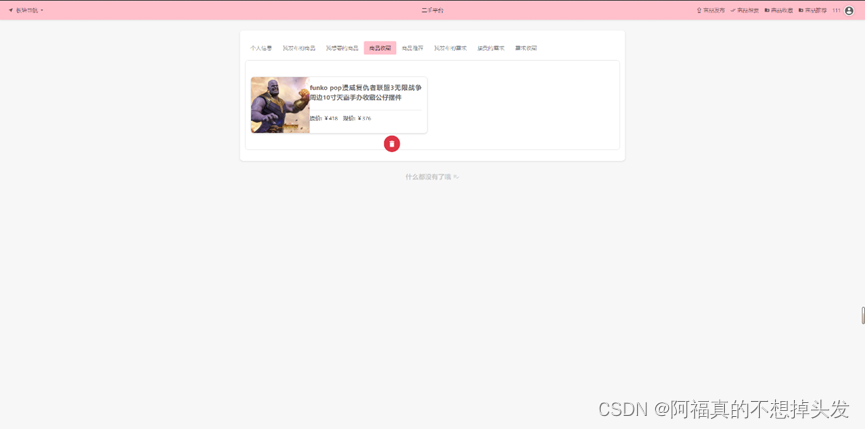
基于springboot+html的二手交易平台(附源码)
基于springboothtml的二手交易平台 介绍部分界面截图如下联系我 介绍 本系统是基于springboothtml的二手交易平台,数据库为mysql,可用于毕设或学习,附数据库 部分界面截图如下 联系我 VX:Zzllh_...
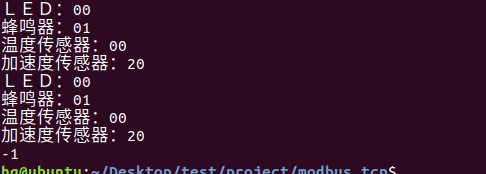
网络编程(Modbus进阶)
思维导图 Modbus RTU(先学一点理论) 概念 Modbus RTU 是工业自动化领域 最广泛应用的串行通信协议,由 Modicon 公司(现施耐德电气)于 1979 年推出。它以 高效率、强健性、易实现的特点成为工业控制系统的通信标准。 包…...
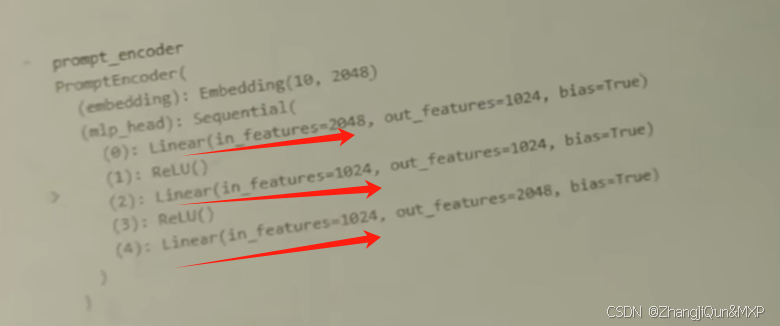
Prompt Tuning、P-Tuning、Prefix Tuning的区别
一、Prompt Tuning、P-Tuning、Prefix Tuning的区别 1. Prompt Tuning(提示调优) 核心思想:固定预训练模型参数,仅学习额外的连续提示向量(通常是嵌入层的一部分)。实现方式:在输入文本前添加可训练的连续向量(软提示),模型只更新这些提示参数。优势:参数量少(仅提…...
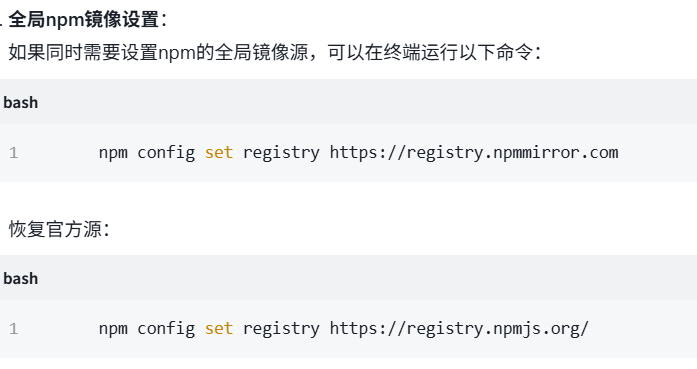
关于nvm与node.js
1 安装nvm 安装过程中手动修改 nvm的安装路径, 以及修改 通过nvm安装node后正在使用的node的存放目录【这句话可能难以理解,但接着往下看你就了然了】 2 修改nvm中settings.txt文件配置 nvm安装成功后,通常在该文件中会出现以下配置&…...
服务器硬防的应用场景都有哪些?
服务器硬防是指一种通过硬件设备层面的安全措施来防御服务器系统受到网络攻击的方式,避免服务器受到各种恶意攻击和网络威胁,那么,服务器硬防通常都会应用在哪些场景当中呢? 硬防服务器中一般会配备入侵检测系统和预防系统&#x…...
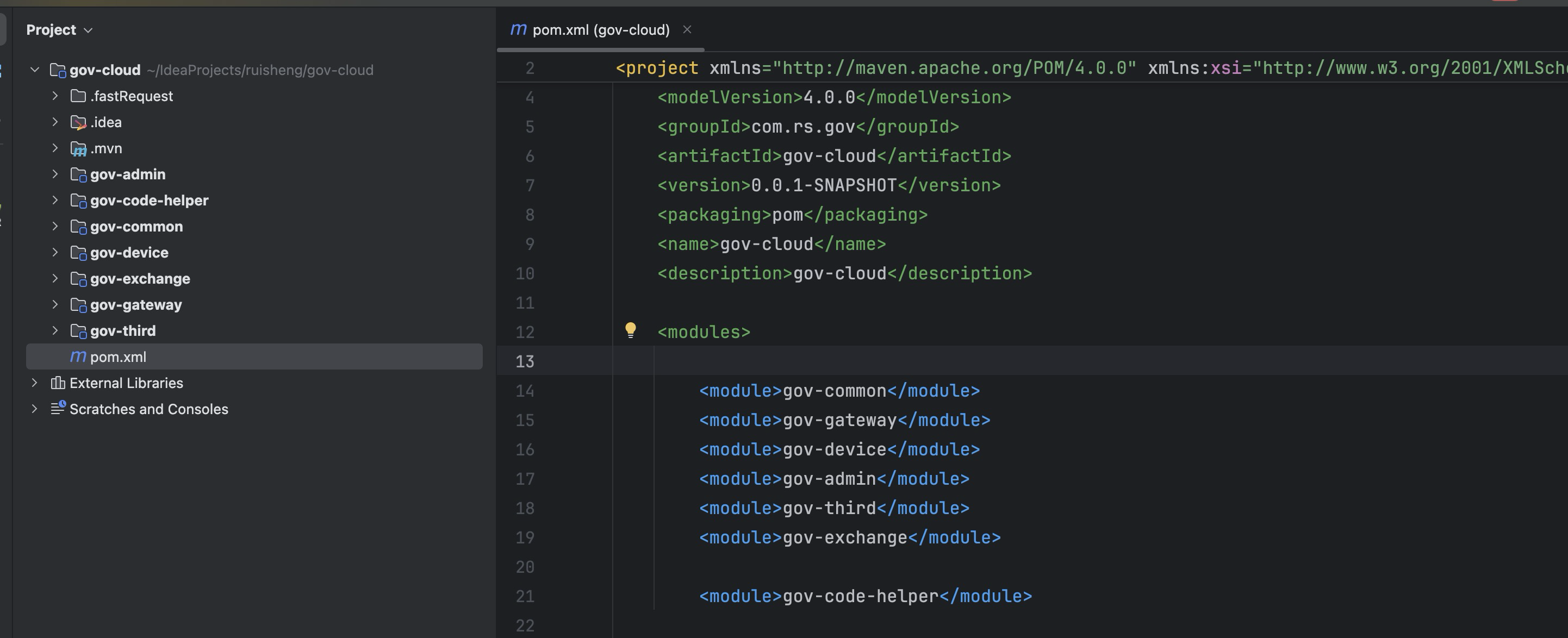
最新SpringBoot+SpringCloud+Nacos微服务框架分享
文章目录 前言一、服务规划二、架构核心1.cloud的pom2.gateway的异常handler3.gateway的filter4、admin的pom5、admin的登录核心 三、code-helper分享总结 前言 最近有个活蛮赶的,根据Excel列的需求预估的工时直接打骨折,不要问我为什么,主要…...
Qwen3-Embedding-0.6B深度解析:多语言语义检索的轻量级利器
第一章 引言:语义表示的新时代挑战与Qwen3的破局之路 1.1 文本嵌入的核心价值与技术演进 在人工智能领域,文本嵌入技术如同连接自然语言与机器理解的“神经突触”——它将人类语言转化为计算机可计算的语义向量,支撑着搜索引擎、推荐系统、…...
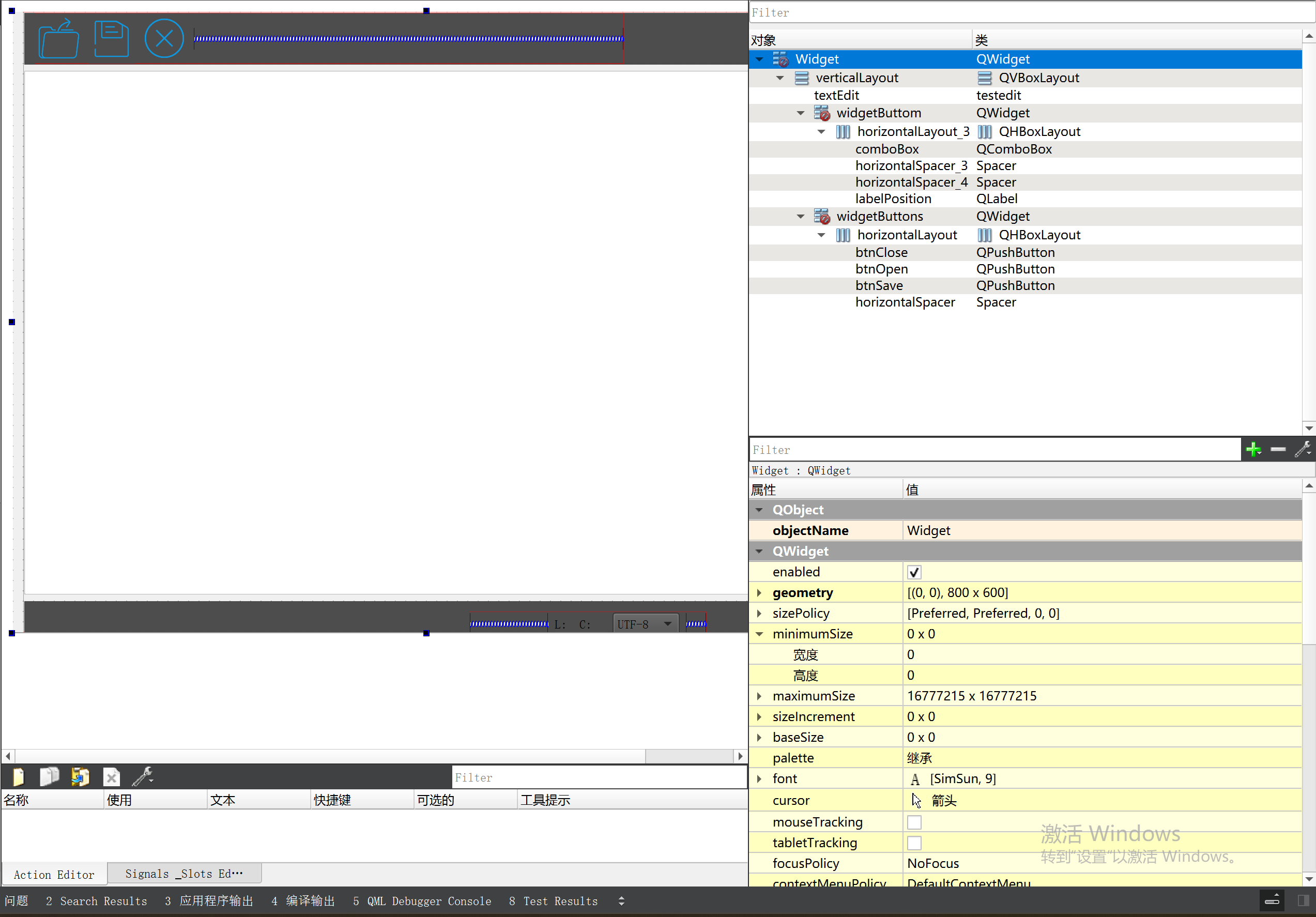
P3 QT项目----记事本(3.8)
3.8 记事本项目总结 项目源码 1.main.cpp #include "widget.h" #include <QApplication> int main(int argc, char *argv[]) {QApplication a(argc, argv);Widget w;w.show();return a.exec(); } 2.widget.cpp #include "widget.h" #include &q…...
稳定币的深度剖析与展望
一、引言 在当今数字化浪潮席卷全球的时代,加密货币作为一种新兴的金融现象,正以前所未有的速度改变着我们对传统货币和金融体系的认知。然而,加密货币市场的高度波动性却成为了其广泛应用和普及的一大障碍。在这样的背景下,稳定…...
ip子接口配置及删除
配置永久生效的子接口,2个IP 都可以登录你这一台服务器。重启不失效。 永久的 [应用] vi /etc/sysconfig/network-scripts/ifcfg-eth0修改文件内内容 TYPE"Ethernet" BOOTPROTO"none" NAME"eth0" DEVICE"eth0" ONBOOT&q…...
2023赣州旅游投资集团
单选题 1.“不登高山,不知天之高也;不临深溪,不知地之厚也。”这句话说明_____。 A、人的意识具有创造性 B、人的认识是独立于实践之外的 C、实践在认识过程中具有决定作用 D、人的一切知识都是从直接经验中获得的 参考答案: C 本题解…...