Hyper-SD: diffusion实时出图,一步搞定,字节出品
Hyper-SD: diffusion实时出图,一步搞定,字节出品
先看效果
Real-Time Generation Demo of Hyper-SD.
Abstract
近来,一系列面向扩散模型(Diffusion Models,DM)的迭代紧凑式传播推断算法陆续出现,以解决其中的复杂性问题。目前,这些算法常常将方法分为两类:一是保持 ODE 流形连续性;二是重写 ODE 流形。然而,这两种方法在压缩后的执行效果中存在显著问题。因此,我们提出了 Hyper-SD 框架,通过有机结合以上两类算法的优点,并将其应用于压缩后模型的学习,从而实现高质量执行。此外,我们引入了人工反馈学习,以提高在低步长情况下的表现和改进该过程中可能发生的损失。同时还使用了分数学习来进一步改善模型在低步长情况下的输出效果。最后我们采用统一 LoRA 框架,将其应用于所有执行过程中的所有步骤。实际上,在不同步长下测试时,Hyper-SDXL 模型都超越了 SDXL-Lightning,并且其在 CLIP Score 和 Aes Score 方面分别提高了 +0.68 以及 +0.51。
Pipeline
超声速降维算法以两阶段进行连续性极化过程,首先在时间段[0,T/2]和[T/2,T]上分别对其进行二阶段连续性极化过程,得到两个连续性方程,之后将这些连续性方程作为训练全局连续性模型的输入
Experiment
对于基于LoRA的Hyper-SD和其他SDXL架构优化方法进行量化比较。
对于 Hyper-SD和其他基于LoRA的加速器架构,包括SD15架构进行了性能比较。
Hyper-SD 比现有优先考虑加速器的方法拥有显著的优势,并在 SD1.5 和 SDXL 架构上得到了更多用户的青睐。
不同尺度的超透明LoRa在应用于不同基础模型时可以产生高质量图像,其步长也是相应变化的。
超宽频LoRA通信模式在超高速数字化(Hyper-SD)中的统一应用,与控制网络相容。例子是基于批荡或画笔图像进行的条件性分叉。
权重文件
Hyper-SDXL-Nstep-lora.safetensors: Lora checkpoint, for SDXL-related models.
Hyper-SD15-Nstep-lora.safetensors: Lora checkpoint, for SD1.5-related models.
Hyper-SDXL-1step-unet.safetensors: Unet checkpoint distilled from SDXL-Base.
文生图模式
SDXL-related models
2-Steps, 4-Steps, 8-steps LoRA
使用 2-steps LoRA, 可自行设置其他LoRA.
import torch
from diffusers import DiffusionPipeline, DDIMScheduler
from huggingface_hub import hf_hub_download
base_model_id = "stabilityai/stable-diffusion-xl-base-1.0"
repo_name = "ByteDance/Hyper-SD"
# Take 2-steps lora as an example
ckpt_name = "Hyper-SDXL-2steps-lora.safetensors"
# Load model.
pipe = DiffusionPipeline.from_pretrained(base_model_id, torch_dtype=torch.float16, variant="fp16").to("cuda")
pipe.load_lora_weights(hf_hub_download(repo_name, ckpt_name))
pipe.fuse_lora()
# Ensure ddim scheduler timestep spacing set as trailing !!!
pipe.scheduler = DDIMScheduler.from_config(pipe.scheduler.config, timestep_spacing="trailing")
# lower eta results in more detail
prompt="a photo of a cat"
image=pipe(prompt=prompt, num_inference_steps=2, guidance_scale=0).images[0]
Unified LoRA (support 1 to 8 steps inference)
可以灵活调整推理步数 以及 eta value 达到最佳效果.
import torch
from diffusers import DiffusionPipeline, TCDScheduler
from huggingface_hub import hf_hub_download
base_model_id = "stabilityai/stable-diffusion-xl-base-1.0"
repo_name = "ByteDance/Hyper-SD"
ckpt_name = "Hyper-SDXL-1step-lora.safetensors"
# Load model.
pipe = DiffusionPipeline.from_pretrained(base_model_id, torch_dtype=torch.float16, variant="fp16").to("cuda")
pipe.load_lora_weights(hf_hub_download(repo_name, ckpt_name))
pipe.fuse_lora()
# Use TCD scheduler to achieve better image quality
pipe.scheduler = TCDScheduler.from_config(pipe.scheduler.config)
# Lower eta results in more detail for multi-steps inference
eta=1.0
prompt="a photo of a cat"
image=pipe(prompt=prompt, num_inference_steps=1, guidance_scale=0, eta=eta).images[0]
1-step SDXL Unet
单步推理.
import torch
from diffusers import DiffusionPipeline, UNet2DConditionModel, LCMScheduler
from huggingface_hub import hf_hub_download
from safetensors.torch import load_file
base_model_id = "stabilityai/stable-diffusion-xl-base-1.0"
repo_name = "ByteDance/Hyper-SD"
ckpt_name = "Hyper-SDXL-1step-Unet.safetensors"
# Load model.
unet = UNet2DConditionModel.from_config(base_model_id, subfolder="unet").to("cuda", torch.float16)
unet.load_state_dict(load_file(hf_hub_download(repo_name, ckpt_name), device="cuda"))
pipe = DiffusionPipeline.from_pretrained(base_model_id, unet=unet, torch_dtype=torch.float16, variant="fp16").to("cuda")
# Use LCM scheduler instead of ddim scheduler to support specific timestep number inputs
pipe.scheduler = LCMScheduler.from_config(pipe.scheduler.config)
# Set start timesteps to 800 in the one-step inference to get better results
prompt="a photo of a cat"
image=pipe(prompt=prompt, num_inference_steps=1, guidance_scale=0, timesteps=[800]).images[0]
SD1.5-related models
2-Steps, 4-Steps, 8-steps LoRA
使用 2-steps LoRA
import torch
from diffusers import DiffusionPipeline, DDIMScheduler
from huggingface_hub import hf_hub_download
base_model_id = "runwayml/stable-diffusion-v1-5"
repo_name = "ByteDance/Hyper-SD"
# Take 2-steps lora as an example
ckpt_name = "Hyper-SD15-2steps-lora.safetensors"
# Load model.
pipe = DiffusionPipeline.from_pretrained(base_model_id, torch_dtype=torch.float16, variant="fp16").to("cuda")
pipe.load_lora_weights(hf_hub_download(repo_name, ckpt_name))
pipe.fuse_lora()
# Ensure ddim scheduler timestep spacing set as trailing !!!
pipe.scheduler = DDIMScheduler.from_config(pipe.scheduler.config, timestep_spacing="trailing")
prompt="a photo of a cat"
image=pipe(prompt=prompt, num_inference_steps=2, guidance_scale=0).images[0]
Unified LoRA (support 1 to 8 steps inference)
可以灵活调整推理步数 以及 eta value 达到最佳效果.
import torch
from diffusers import DiffusionPipeline, TCDScheduler
from huggingface_hub import hf_hub_download
base_model_id = "runwayml/stable-diffusion-v1-5"
repo_name = "ByteDance/Hyper-SD"
ckpt_name = "Hyper-SD15-1step-lora.safetensors"
# Load model.
pipe = DiffusionPipeline.from_pretrained(base_model_id, torch_dtype=torch.float16, variant="fp16").to("cuda")
pipe.load_lora_weights(hf_hub_download(repo_name, ckpt_name))
pipe.fuse_lora()
# Use TCD scheduler to achieve better image quality
pipe.scheduler = TCDScheduler.from_config(pipe.scheduler.config)
# Lower eta results in more detail for multi-steps inference
eta=1.0
prompt="a photo of a cat"
image=pipe(prompt=prompt, num_inference_steps=1, guidance_scale=0, eta=eta).images[0]
ControlNet 模式
SDXL-related models
2-Steps, 4-Steps, 8-steps LoRA
使用 Canny Controlnet 以及 2-steps 推理:
import torch
from diffusers.utils import load_image
import numpy as np
import cv2
from PIL import Image
from diffusers import ControlNetModel, StableDiffusionXLControlNetPipeline, AutoencoderKL, DDIMScheduler
from huggingface_hub import hf_hub_download# Load original image
image = load_image("https://huggingface.co/datasets/hf-internal-testing/diffusers-images/resolve/main/sd_controlnet/hf-logo.png")
image = np.array(image)
# Prepare Canny Control Image
low_threshold = 100
high_threshold = 200
image = cv2.Canny(image, low_threshold, high_threshold)
image = image[:, :, None]
image = np.concatenate([image, image, image], axis=2)
control_image = Image.fromarray(image)
control_image.save("control.png")
control_weight = 0.5 # recommended for good generalization# Initialize pipeline
controlnet = ControlNetModel.from_pretrained("diffusers/controlnet-canny-sdxl-1.0",torch_dtype=torch.float16
)
vae = AutoencoderKL.from_pretrained("madebyollin/sdxl-vae-fp16-fix", torch_dtype=torch.float16)
pipe = StableDiffusionXLControlNetPipeline.from_pretrained("stabilityai/stable-diffusion-xl-base-1.0", controlnet=controlnet, vae=vae, torch_dtype=torch.float16).to("cuda")pipe.load_lora_weights(hf_hub_download("ByteDance/Hyper-SD", "Hyper-SDXL-2steps-lora.safetensors"))
# Ensure ddim scheduler timestep spacing set as trailing !!!
pipe.scheduler = DDIMScheduler.from_config(pipe.scheduler.config, timestep_spacing="trailing")
pipe.fuse_lora()
image = pipe("A chocolate cookie", num_inference_steps=2, image=control_image, guidance_scale=0, controlnet_conditioning_scale=control_weight).images[0]
image.save('image_out.png')
Unified LoRA (support 1 to 8 steps inference)
使用 Canny Controlnet:
import torch
from diffusers.utils import load_image
import numpy as np
import cv2
from PIL import Image
from diffusers import ControlNetModel, StableDiffusionXLControlNetPipeline, AutoencoderKL, TCDScheduler
from huggingface_hub import hf_hub_download# Load original image
image = load_image("https://huggingface.co/datasets/hf-internal-testing/diffusers-images/resolve/main/sd_controlnet/hf-logo.png")
image = np.array(image)
# Prepare Canny Control Image
low_threshold = 100
high_threshold = 200
image = cv2.Canny(image, low_threshold, high_threshold)
image = image[:, :, None]
image = np.concatenate([image, image, image], axis=2)
control_image = Image.fromarray(image)
control_image.save("control.png")
control_weight = 0.5 # recommended for good generalization# Initialize pipeline
controlnet = ControlNetModel.from_pretrained("diffusers/controlnet-canny-sdxl-1.0",torch_dtype=torch.float16
)
vae = AutoencoderKL.from_pretrained("madebyollin/sdxl-vae-fp16-fix", torch_dtype=torch.float16)
pipe = StableDiffusionXLControlNetPipeline.from_pretrained("stabilityai/stable-diffusion-xl-base-1.0",controlnet=controlnet, vae=vae, torch_dtype=torch.float16).to("cuda")# Load Hyper-SD15-1step lora
pipe.load_lora_weights(hf_hub_download("ByteDance/Hyper-SD", "Hyper-SDXL-1step-lora.safetensors"))
pipe.fuse_lora()
# Use TCD scheduler to achieve better image quality
pipe.scheduler = TCDScheduler.from_config(pipe.scheduler.config)
# Lower eta results in more detail for multi-steps inference
eta=1.0
image = pipe("A chocolate cookie", num_inference_steps=4, image=control_image, guidance_scale=0, controlnet_conditioning_scale=control_weight, eta=eta).images[0]
image.save('image_out.png')
SD1.5-related models
2-Steps, 4-Steps, 8-steps LoRA
使用 Canny Controlnet 以及 2-steps 推理:
import torch
from diffusers.utils import load_image
import numpy as np
import cv2
from PIL import Image
from diffusers import ControlNetModel, StableDiffusionControlNetPipeline, DDIMSchedulerfrom huggingface_hub import hf_hub_downloadcontrolnet_checkpoint = "lllyasviel/control_v11p_sd15_canny"# Load original image
image = load_image("https://huggingface.co/lllyasviel/control_v11p_sd15_canny/resolve/main/images/input.png")
image = np.array(image)
# Prepare Canny Control Image
low_threshold = 100
high_threshold = 200
image = cv2.Canny(image, low_threshold, high_threshold)
image = image[:, :, None]
image = np.concatenate([image, image, image], axis=2)
control_image = Image.fromarray(image)
control_image.save("control.png")# Initialize pipeline
controlnet = ControlNetModel.from_pretrained(controlnet_checkpoint, torch_dtype=torch.float16)
pipe = StableDiffusionControlNetPipeline.from_pretrained("runwayml/stable-diffusion-v1-5", controlnet=controlnet, torch_dtype=torch.float16).to("cuda")
pipe.load_lora_weights(hf_hub_download("ByteDance/Hyper-SD", "Hyper-SD15-2steps-lora.safetensors"))
pipe.fuse_lora()
# Ensure ddim scheduler timestep spacing set as trailing !!!
pipe.scheduler = DDIMScheduler.from_config(pipe.scheduler.config, timestep_spacing="trailing")
image = pipe("a blue paradise bird in the jungle", num_inference_steps=2, image=control_image, guidance_scale=0).images[0]
image.save('image_out.png')
Unified LoRA (support 1 to 8 steps inference)
使用 Canny Controlnet :
import torch
from diffusers.utils import load_image
import numpy as np
import cv2
from PIL import Image
from diffusers import ControlNetModel, StableDiffusionControlNetPipeline, TCDScheduler
from huggingface_hub import hf_hub_downloadcontrolnet_checkpoint = "lllyasviel/control_v11p_sd15_canny"# Load original image
image = load_image("https://huggingface.co/lllyasviel/control_v11p_sd15_canny/resolve/main/images/input.png")
image = np.array(image)
# Prepare Canny Control Image
low_threshold = 100
high_threshold = 200
image = cv2.Canny(image, low_threshold, high_threshold)
image = image[:, :, None]
image = np.concatenate([image, image, image], axis=2)
control_image = Image.fromarray(image)
control_image.save("control.png")# Initialize pipeline
controlnet = ControlNetModel.from_pretrained(controlnet_checkpoint, torch_dtype=torch.float16)
pipe = StableDiffusionControlNetPipeline.from_pretrained("runwayml/stable-diffusion-v1-5", controlnet=controlnet, torch_dtype=torch.float16).to("cuda")
# Load Hyper-SD15-1step lora
pipe.load_lora_weights(hf_hub_download("ByteDance/Hyper-SD", "Hyper-SD15-1step-lora.safetensors"))
pipe.fuse_lora()
# Use TCD scheduler to achieve better image quality
pipe.scheduler = TCDScheduler.from_config(pipe.scheduler.config)
# Lower eta results in more detail for multi-steps inference
eta=1.0
image = pipe("a blue paradise bird in the jungle", num_inference_steps=1, image=control_image, guidance_scale=0, eta=eta).images[0]
image.save('image_out.png')
相关文章:
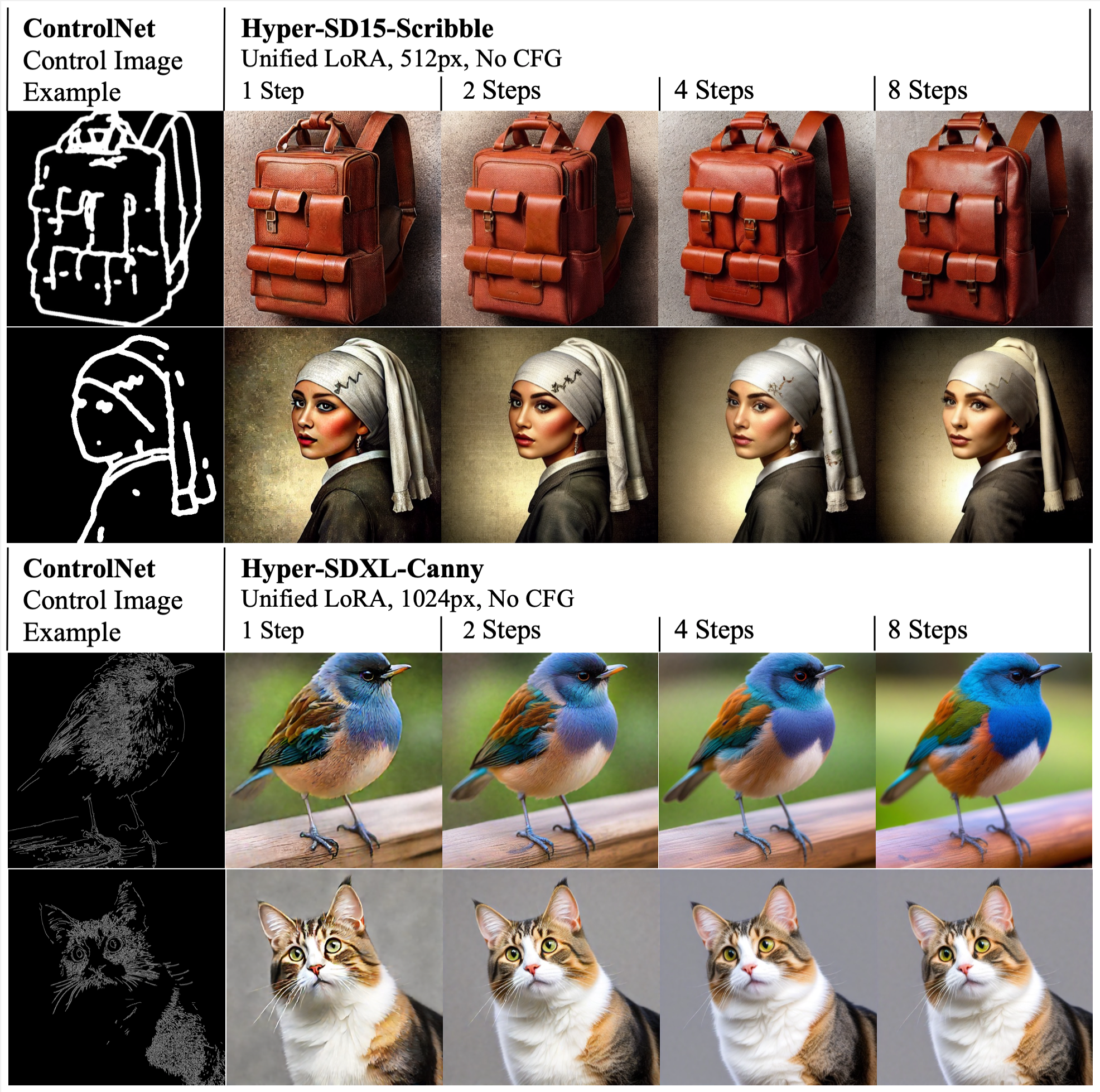
Hyper-SD: diffusion实时出图,一步搞定,字节出品
Hyper-SD: diffusion实时出图,一步搞定,字节出品 先看效果 Real-Time Generation Demo of Hyper-SD. Abstract 近来,一系列面向扩散模型(Diffusion Models,DM)的迭代紧凑式传播推断算法陆续出现…...
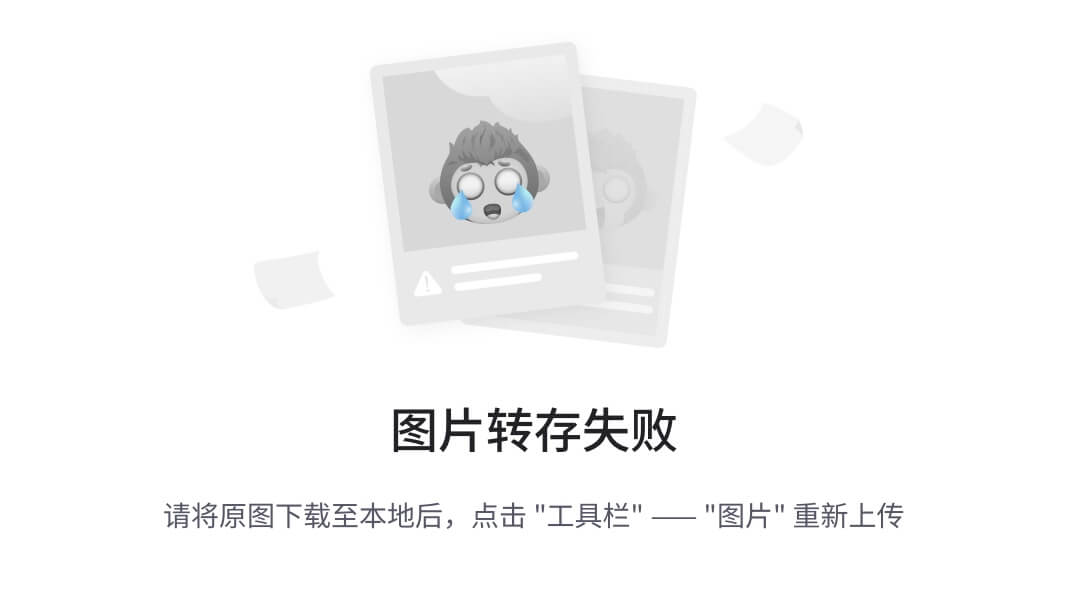
:长亭雷池社区版动态防护体验测评
序 长亭雷池在最近发布了动态防护功能,据说可以动态加密保护网页前端代码和阻止爬虫行为、阻止漏洞扫描行为等。今天就来体验测试一下 WAF 是什么 WAF 是 Web Application Firewall 的缩写,也被称为 Web 应用防火墙。区别于传统防火墙,WAF …...
数据结构复习
基本概念和术语: 数据:是描述客观事物的符号,是计算机中可以操作的对象,是能被计算机识别,并输入给计算机处理的符号集合。 数据元素:是组成数据的,具有一定意义的基本单位,在计算机…...
小世界网络生成及其分析
研究背景: 小世界网络是一种介于规则网络和随机网络之间的网络模型,具有短平均路径和高聚集性的特点。这种网络模型被广泛应用于社交网络、互联网、生物网络等领域的研究中。研究小世界网络的生成和分析可以帮助我们理解和揭示复杂网络的结构和特性,以及网络中信息传播、动力…...
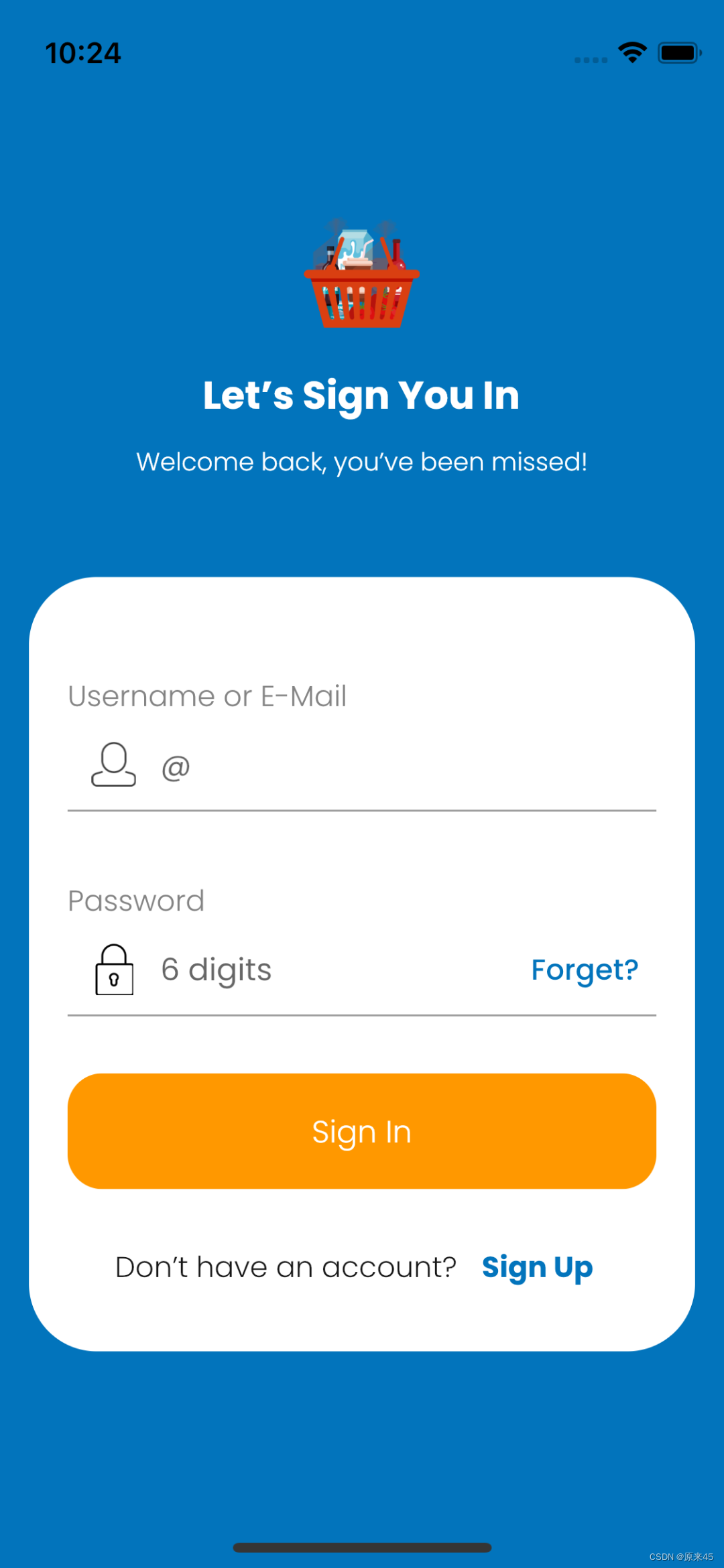
Flutter基础 -- Flutter布局练习(小项目)
目录 1. Splash 布局(第一页) 1.1 目标 1.2 当前效果图 1.3 创建 Splash 界面 1.4 设置 MaterialApp 1.5 设置 Splash 背景色 1.6 布局 Splash 界面 1.7 总结 2. Splash 圆角图片 2.1 目标 2.2 当前效果图 2.3 蓝湖下载图片 2.4 图片导入项…...
详解布隆过滤器,实现分布式布隆过滤器
什么是布隆过滤器? 原理 布隆过滤器是一种基于位数组(bit array)和多个哈希函数的数据结构。其核心原理是: 初始化一个长度为m的位数组,所有位初始化为0。使用k个不同的哈希函数将元素映射到位数组中的k个位置。当插…...
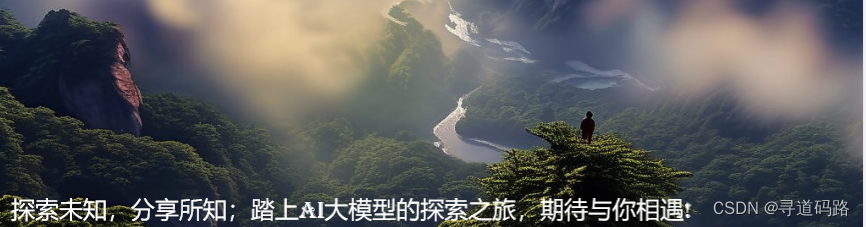
程序员职业素养:AI新时代下的机遇与挑战
目录 一、引言二、程序员职业素养的五大要点1. 技术能力2. 沟通能力3. 团队合作4. 责任心5. 敬业精神 三、实际案例解析四、程序员职业素养在实际工作中的应用五、AI新时代的程序员的职业发展建议六、总结七、结语 一、引言 在当今这个科技飞速发展的时代,程序员这…...

智能管理,无忧报修——高校校园报事报修系统小程序全解析
随着数字化、智能化的发展,高校生活也迎来了前所未有的变革。你是否还在为宿舍的水龙头漏水、图书馆的灯光闪烁而烦恼?你是否还在为报修流程繁琐、等待时间长而焦虑?今天,这一切都将成为过去式!因为一款震撼高校圈的新…...
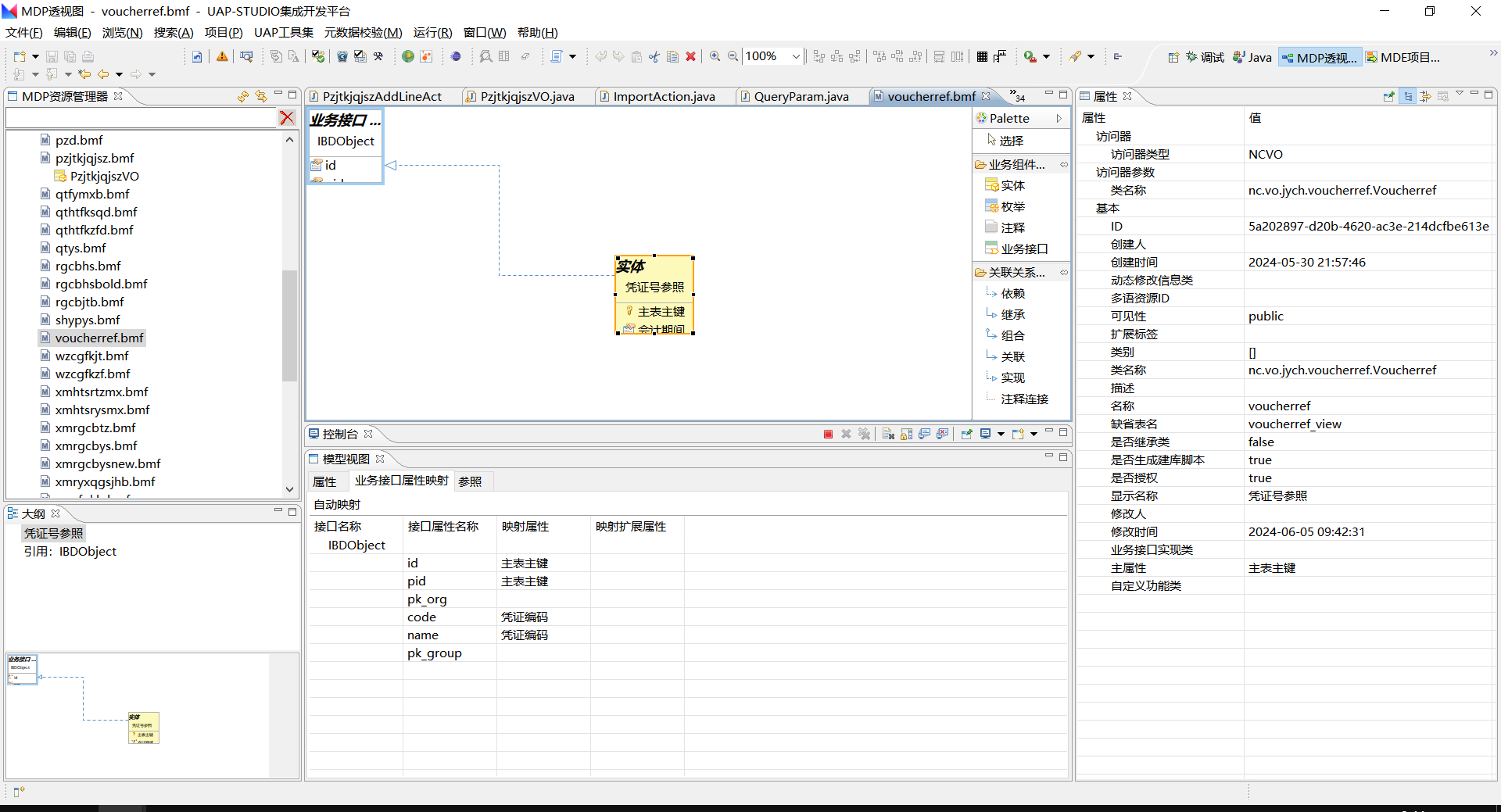
nc解决自定义参照字段前台保存后只显示主键的问题
nc解决自定义参照字段前台保存后只显示主键的问题 自定义参照类VoucherRefModel.java package nc.ui.jych.ref;import nc.ui.bd.ref.AbstractRefModel;/*** desc 凭证号参照* author hanh**/ public class VoucherRefModel extends AbstractRefModel {Overridepublic String[…...
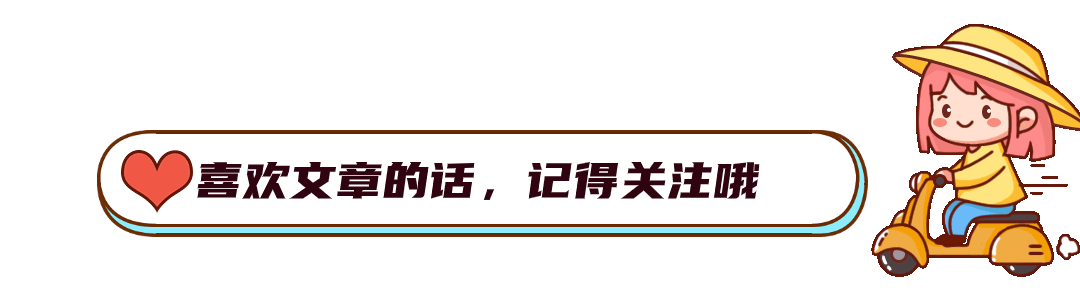
鸿蒙全栈开发-一文读懂鸿蒙同模块不同模块下的UIAbility跳转详解
前言 根据第三方机构Counterpoint数据,截至2023年三季度末,HarmonyOS在中国智能手机操作系统的市场份额已经提升至13%。短短四年的时间,HarmonyOS就成长为仅次于安卓、苹果iOS的全球第三大操作系统。 因此,对于鸿蒙生态建设而言&a…...
【Python】使用 SQLObject orm 库快速将接口数据存入数据库
使用 SQLObject orm 库快速将接口数据存入数据库 文章目录 使用 SQLObject orm 库快速将接口数据存入数据库背景orm python 版本都有哪些? SQLObject 简单的使用 背景 因为测试需要,要将百万条数据接口查询数据存入数据库中,为了减少 mysql …...
@EnableResourceServer资源服务注解源码分析
文章目录 学习参考EnableResourceServer概要ResourceServerConfiguration属性定义configure(HttpSecurity)ResourceServerSecurityConfigurerinit(HttpSecurit)configure(HttpSecurity) 学习参考 Spring Security框架配置运行流程完整分析 - 【必看】 Security OAuth2 授权 &…...
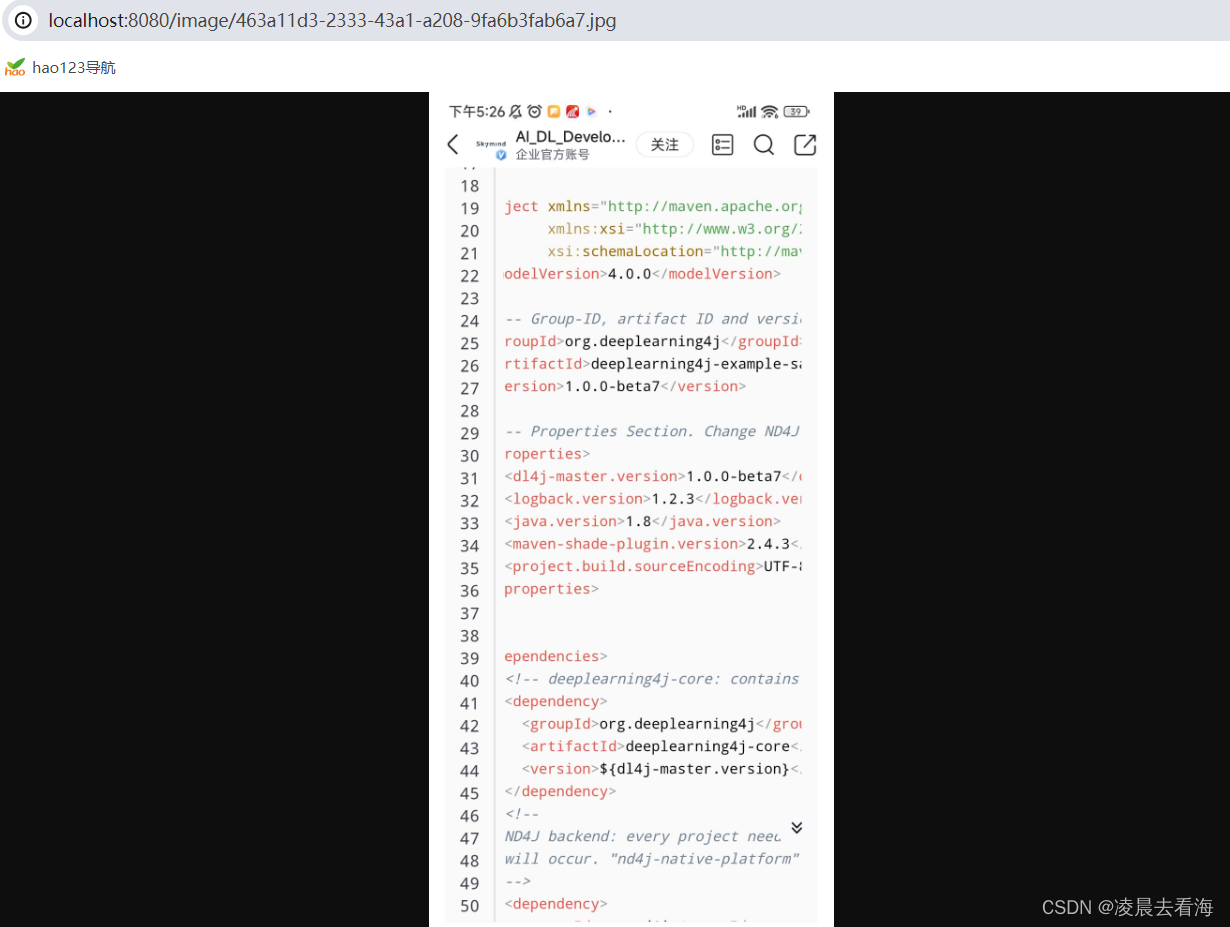
SpringBoot实现图片文件上传和回显的两种方式
目录 一 功能需求 二 上传本地 2.1 实现文件上传的controller层 2.2 图片访问资源映射 二 上传OSS 一 功能需求 实现图片的上传和回显功能其实在业务中是非常常见的,比如需要上传头像,或者交易平台需要上传物品的图片等等,都需要上传和回…...
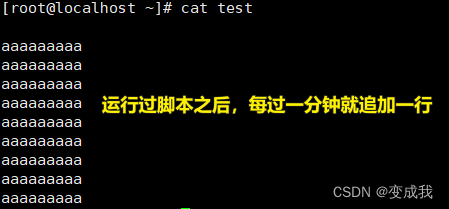
进程和计划任务以及步骤
进程 进程和程序有关,把该文件放到内存里,进程是动态的,不同时刻的状态不一样 内存:放置正在运行的程序和所需数据的位置 程序启动 ——》将相关文件和数据放到内存里 ——》进程(processes) 进程相关命令 …...
使用Python实现深度学习模型:序列到序列模型(Seq2Seq)
序列到序列(Seq2Seq)模型是一种深度学习模型,广泛应用于机器翻译、文本生成和对话系统等自然语言处理任务。它的核心思想是将一个序列(如一句话)映射到另一个序列。本文将详细介绍 Seq2Seq 模型的原理,并使…...
力扣283. 移动零
给定一个数组 nums,编写一个函数将所有 0 移动到数组的末尾,同时保持非零元素的相对顺序。 请注意 ,必须在不复制数组的情况下原地对数组进行操作。 示例 1: 输入: nums [0,1,0,3,12] 输出:[1,3,12,0,0] 示例 2: 输入: nums [0] …...
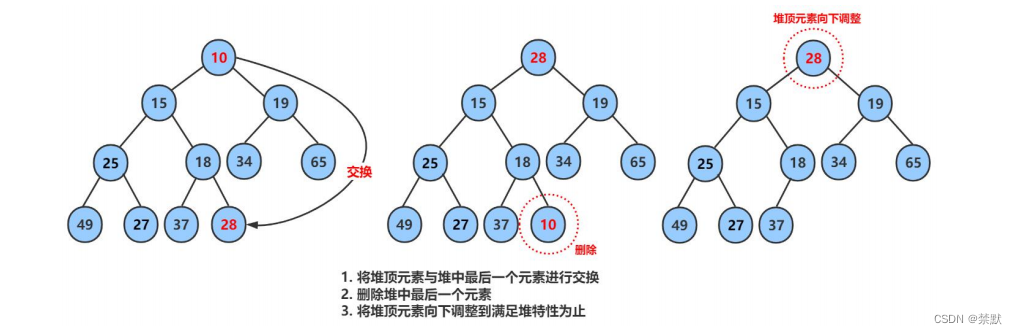
二叉树的顺序结构(堆的实现)
前言 普通的二叉树是不适合用数组来存储的,因为可能会存在大量的空间浪费。而完全二叉树更适合使用顺序结 构存储。 现实中我们通常把堆 ( 一种二叉树 ) 使用顺序结构的数组来存储,需要注意的是这里的堆和操作系统 虚拟进程地址空间中的堆是两回事&…...
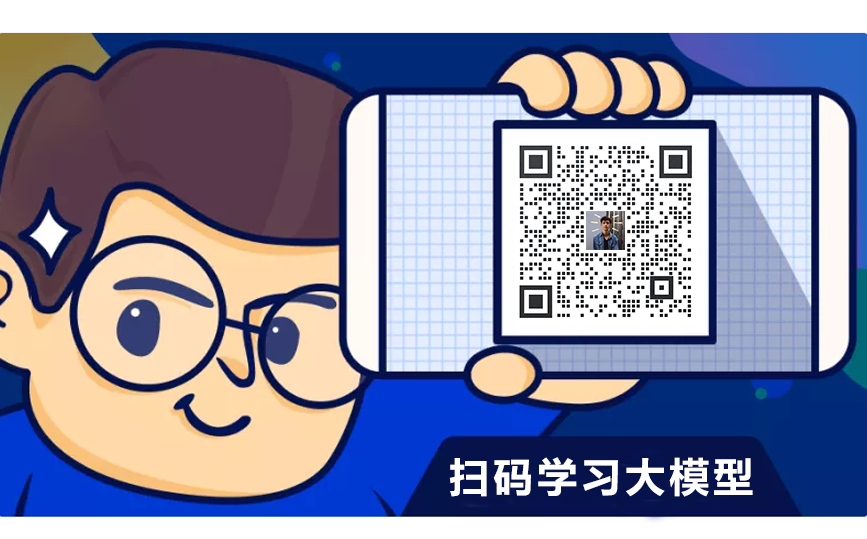
2024大模型如何学习【附学习资料】
摘要: 通过深入了解本文中的这些细节,并在实际项目中应用相关知识,将能够更好地理解和利用大模型的潜力,不仅在学术研究中,也在工程实践中。通过不断探索新方法、参与项目和保持热情,并将其应用于各种领域&…...
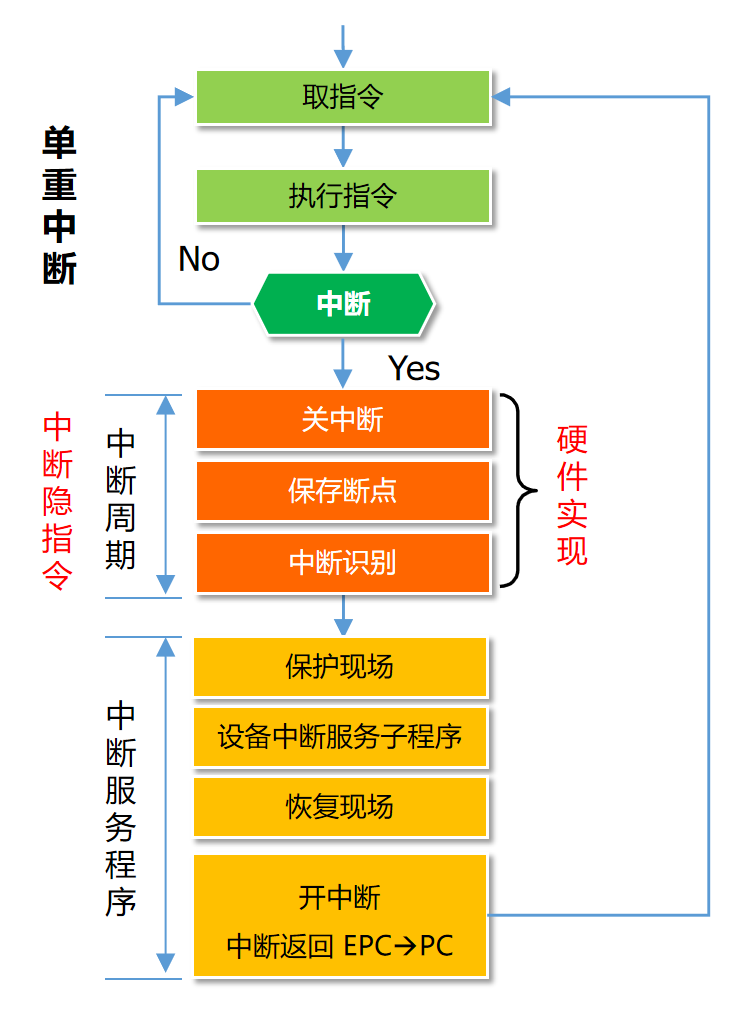
计算机组成原理·考点知识点整理
根据往年考试题,对考点和知识点的一个整理。 校验编码 码距 一种编码的最小码距,其实就是指这种编码的码距。码距有两种定义: 码距所描述的对象含义 2 2 2 个特定的码其二进制表示中不同位的个数一种编码这种编码中任意 2 2 2 个合法编码的…...
python-datetime模块时间戳常用方法汇总
文章目录 datetime模块常用方法1、导入模块2、获取当前日期和时间3、获取当前日期4、创建特定日期或时间5、日期和时间的运算6、使用timedelta运算日期时间创建 timedelta 对象timedelta 的加减运算timedelta 的属性timedelta 的比较示例代码格式化日期和时间获取日期和时间的各…...
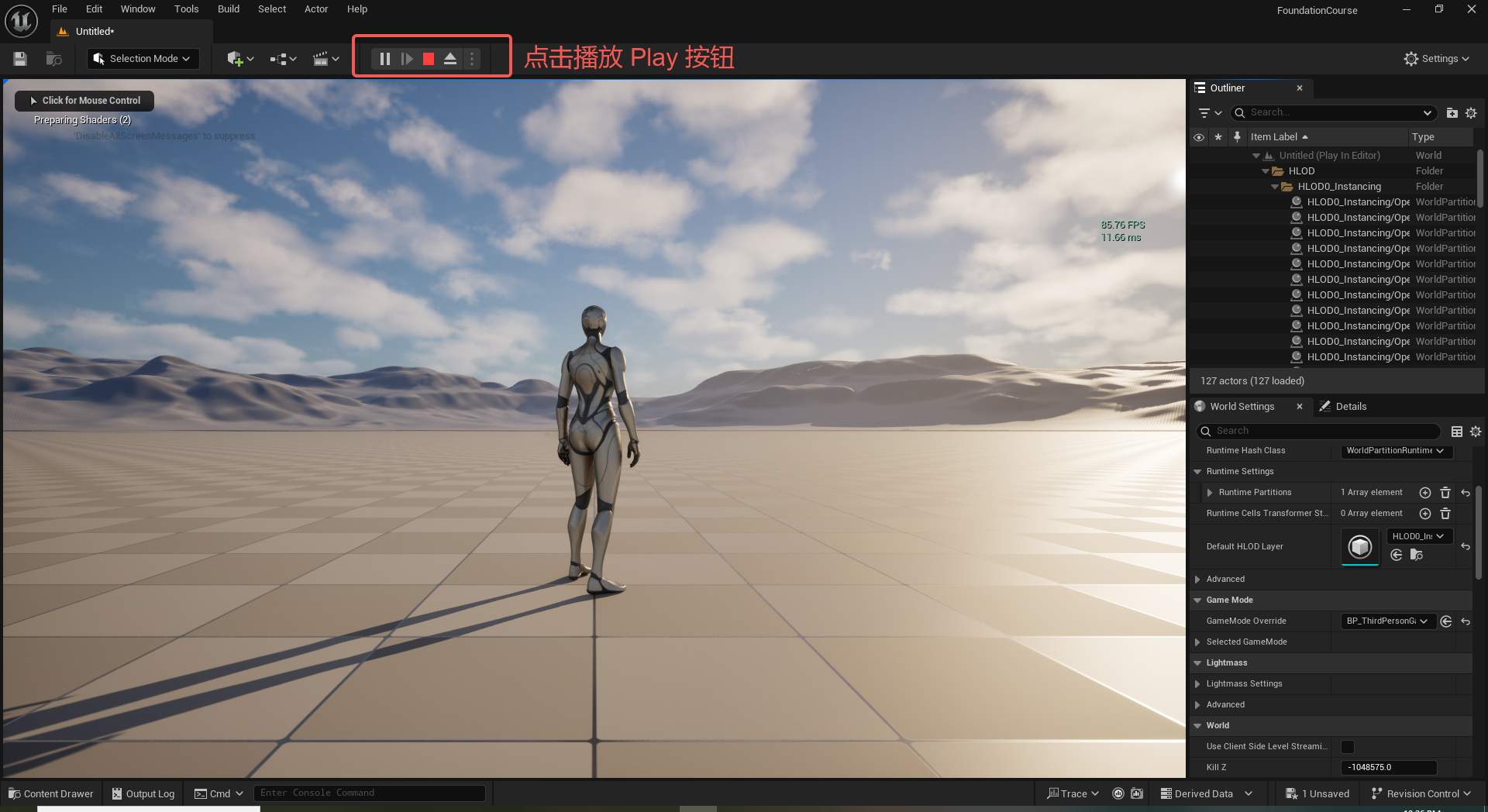
UE5 学习系列(二)用户操作界面及介绍
这篇博客是 UE5 学习系列博客的第二篇,在第一篇的基础上展开这篇内容。博客参考的 B 站视频资料和第一篇的链接如下: 【Note】:如果你已经完成安装等操作,可以只执行第一篇博客中 2. 新建一个空白游戏项目 章节操作,重…...
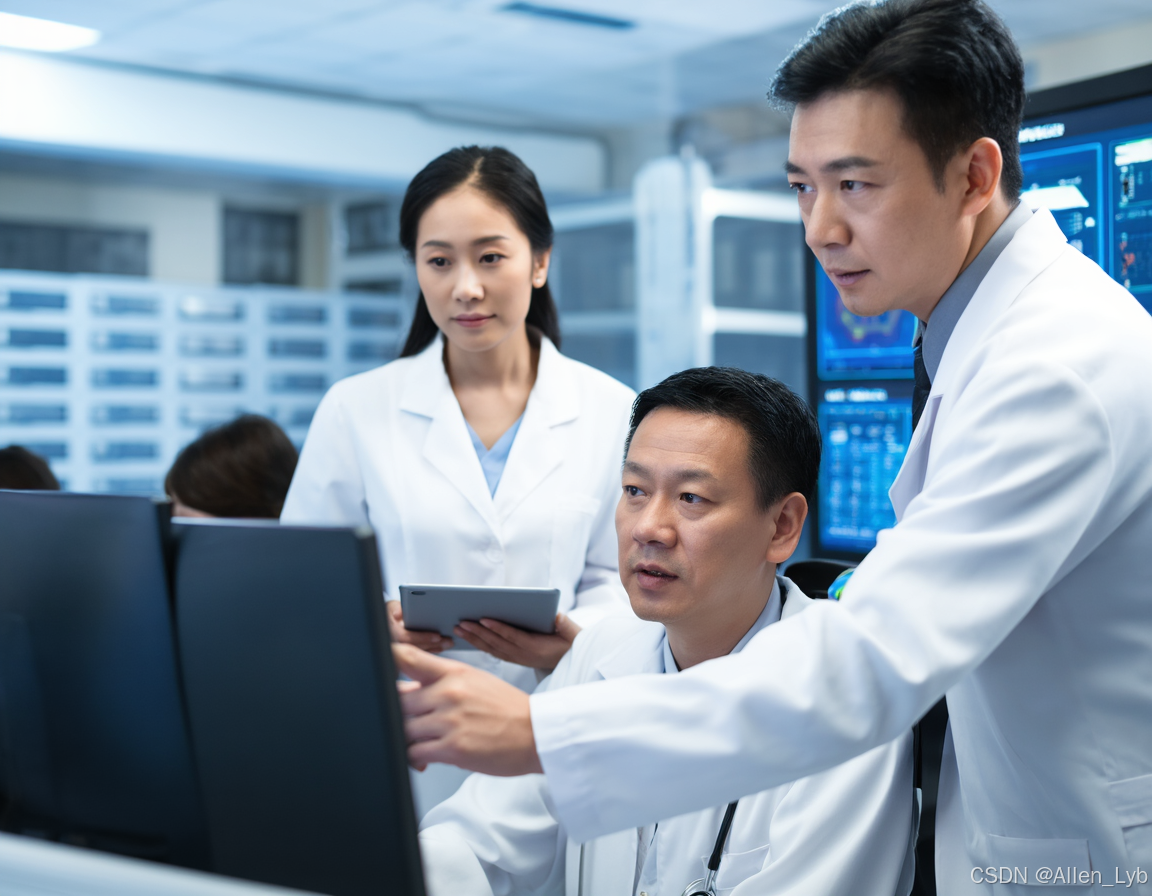
智慧医疗能源事业线深度画像分析(上)
引言 医疗行业作为现代社会的关键基础设施,其能源消耗与环境影响正日益受到关注。随着全球"双碳"目标的推进和可持续发展理念的深入,智慧医疗能源事业线应运而生,致力于通过创新技术与管理方案,重构医疗领域的能源使用模式。这一事业线融合了能源管理、可持续发…...
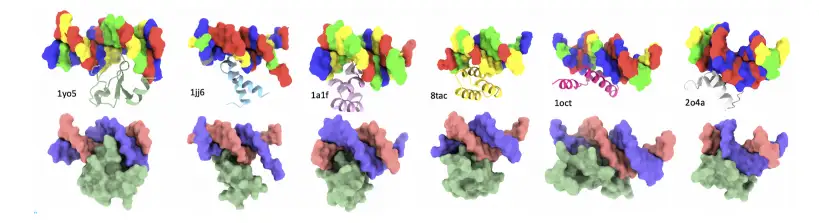
8k长序列建模,蛋白质语言模型Prot42仅利用目标蛋白序列即可生成高亲和力结合剂
蛋白质结合剂(如抗体、抑制肽)在疾病诊断、成像分析及靶向药物递送等关键场景中发挥着不可替代的作用。传统上,高特异性蛋白质结合剂的开发高度依赖噬菌体展示、定向进化等实验技术,但这类方法普遍面临资源消耗巨大、研发周期冗长…...
服务器硬防的应用场景都有哪些?
服务器硬防是指一种通过硬件设备层面的安全措施来防御服务器系统受到网络攻击的方式,避免服务器受到各种恶意攻击和网络威胁,那么,服务器硬防通常都会应用在哪些场景当中呢? 硬防服务器中一般会配备入侵检测系统和预防系统&#x…...
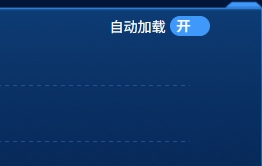
el-switch文字内置
el-switch文字内置 效果 vue <div style"color:#ffffff;font-size:14px;float:left;margin-bottom:5px;margin-right:5px;">自动加载</div> <el-switch v-model"value" active-color"#3E99FB" inactive-color"#DCDFE6"…...
Nginx server_name 配置说明
Nginx 是一个高性能的反向代理和负载均衡服务器,其核心配置之一是 server 块中的 server_name 指令。server_name 决定了 Nginx 如何根据客户端请求的 Host 头匹配对应的虚拟主机(Virtual Host)。 1. 简介 Nginx 使用 server_name 指令来确定…...
三体问题详解
从物理学角度,三体问题之所以不稳定,是因为三个天体在万有引力作用下相互作用,形成一个非线性耦合系统。我们可以从牛顿经典力学出发,列出具体的运动方程,并说明为何这个系统本质上是混沌的,无法得到一般解…...
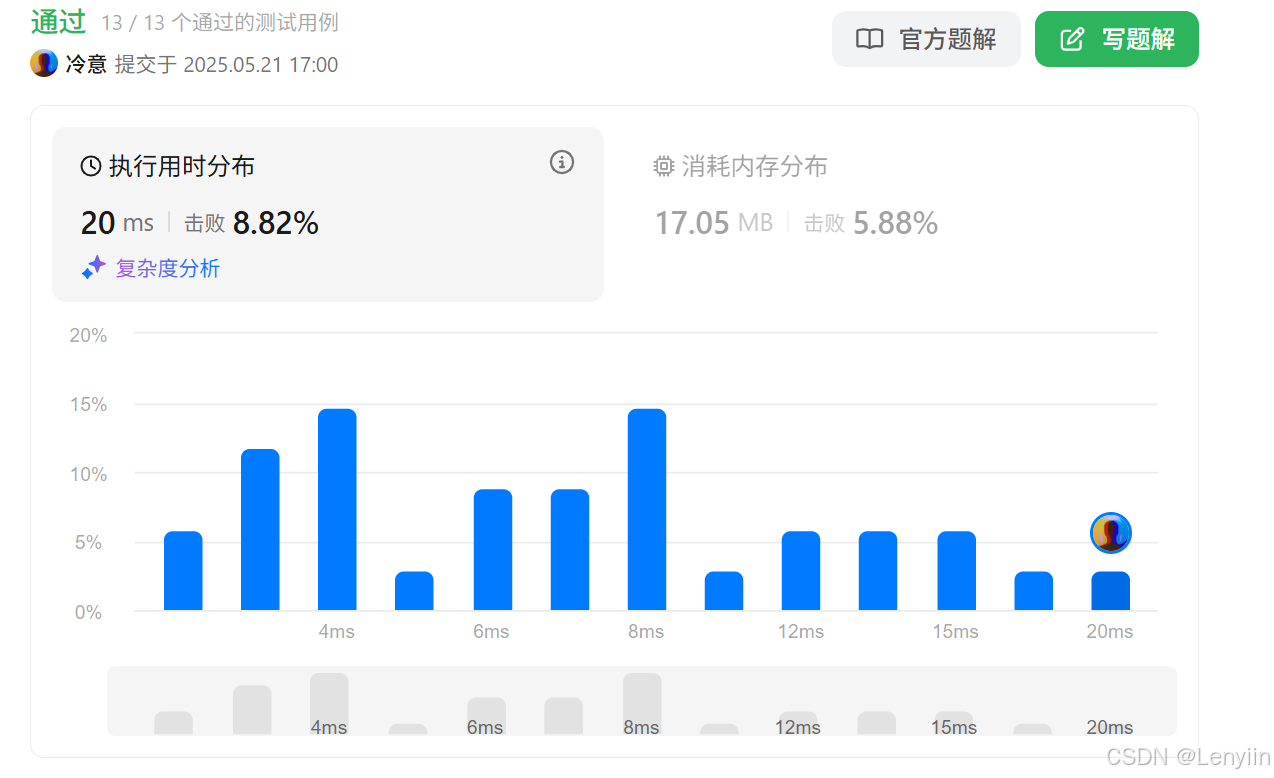
第 86 场周赛:矩阵中的幻方、钥匙和房间、将数组拆分成斐波那契序列、猜猜这个单词
Q1、[中等] 矩阵中的幻方 1、题目描述 3 x 3 的幻方是一个填充有 从 1 到 9 的不同数字的 3 x 3 矩阵,其中每行,每列以及两条对角线上的各数之和都相等。 给定一个由整数组成的row x col 的 grid,其中有多少个 3 3 的 “幻方” 子矩阵&am…...
安卓基础(aar)
重新设置java21的环境,临时设置 $env:JAVA_HOME "D:\Android Studio\jbr" 查看当前环境变量 JAVA_HOME 的值 echo $env:JAVA_HOME 构建ARR文件 ./gradlew :private-lib:assembleRelease 目录是这样的: MyApp/ ├── app/ …...
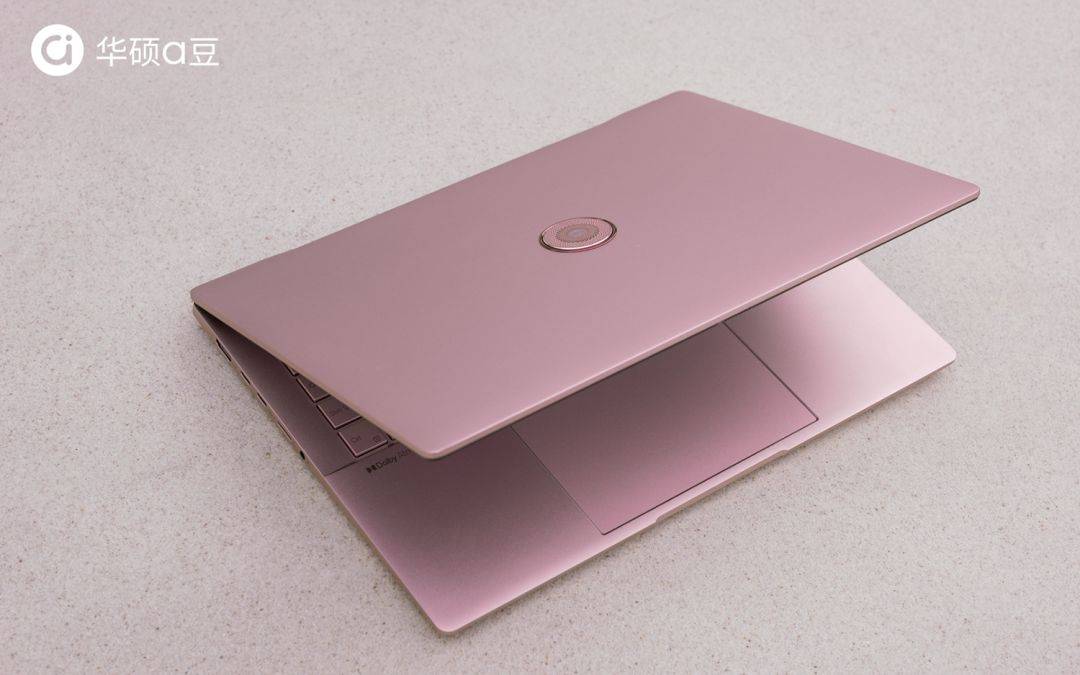
华硕a豆14 Air香氛版,美学与科技的馨香融合
在快节奏的现代生活中,我们渴望一个能激发创想、愉悦感官的工作与生活伙伴,它不仅是冰冷的科技工具,更能触动我们内心深处的细腻情感。正是在这样的期许下,华硕a豆14 Air香氛版翩然而至,它以一种前所未有的方式&#x…...