基于级联深度学习算法的前列腺病灶检测在双参数MRI中的评估| 文献速递-基于深度学习的乳房、前列腺疾病诊断系统
Title
题目
Evaluation of a Cascaded Deep Learning–based Algorithm for Prostate Lesion Detection at Biparametric MRI
基于级联深度学习算法的前列腺病灶检测在双参数MRI中的评估
Background
背景
Multiparametric MRI (mpMRI) improves prostate cancer (PCa) detection compared with systematic biopsy, but its interpretation is prone to interreader variation, which results in performance inconsistency. Artificial intelligence (AI) models can assist in mpMRI interpretation, but large training data sets and extensive model testing are required.
多参数MRI(mpMRI)相比系统性活检能提高前列腺癌(PCa)的检测率,但其解读易受不同阅片者之间的差异影响,导致性能不一致。人工智能(AI)模型可以辅助mpMRI的解读,但需要大量的训练数据集和广泛的模型测试。
Method
方法
This secondary analysis of a prospective registry included consecutive patients with suspected or known PCa who underwent mpMRI, US-guided systematic biopsy, or combined systematic and MRI/US fusion–guided biopsy between April 2019 and September 2022. All lesions were prospectively evaluated using Prostate Imaging Reporting and Data System version 2.1. The lesion- and participant-level performance of a previously developed cascaded deep learning algorithm was compared with histopathologic outcomes and radiologist readings using sensitivity, positive predictive value (PPV), and Dice similarity coefficient (DSC).
这项前瞻性登记的二次分析纳入了2019年4月至2022年9月期间连续接受多参数MRI(mpMRI)、超声引导的系统性活检或系统性活检与MRI/超声融合引导活检联合检查的疑似或已知前列腺癌(PCa)患者。所有病灶均按照前列腺影像报告与数据系统(Prostate Imaging Reporting and Data System,PI-RADS)2.1版本进行前瞻性评估。使用敏感性、阳性预测值(PPV)和Dice相似系数(DSC)比较了先前开发的级联深度学习算法与组织病理学结果及放射科医生解读之间的病灶级和参与者级表现。
Conclusion
结论
The AI algorithm detected cancer-suspicious lesions on biparametric MRI scans with a performance comparable to that of
该AI算法在双参数MRI扫描中检测癌症可疑病灶的表现与以下内容相当:
Results
结果
A total of 658 male participants (median age, 67 years [IQR, 61–71 years]) with 1029 MRI-visible lesions were included. At histopathologic analysis, 45% (294 of 658) of participants had lesions of International Society of Urological Pathology (ISUP) grade group (GG) 2 or higher. The algorithm identified 96% (282 of 294; 95% CI: 94%, 98%) of all participants with clinically significant PCa, whereas the radiologist identified 98% (287 of 294; 95% CI: 96%, 99%; P = .23). The algorithm identified 84% (103 of 122), 96% (152 of 159), 96% (47 of 49), 95% (38 of 40), and 98% (45 of 46) of participants with ISUP GG 1, 2, 3, 4, and 5 lesions, respectively. In the lesion-level analysis using radiologist ground truth, the detection sensitivity was 55% (569 of 1029; 95% CI: 52%, 58%), and the PPV was 57% (535 of 934; 95% CI: 54%, 61%). The mean number of false-positive lesions per participant was 0.61 (range, 0–3). The lesion segmentation DSC was 0.29.
共纳入了658名男性参与者(中位年龄67岁 [IQR, 61–71岁]),其中包括1029个MRI可见病灶。在组织病理学分析中,45%的参与者(658人中的294人)具有国际泌尿病理学会(ISUP)分级2级或更高的病灶。算法识别出了96%的临床意义显著的前列腺癌(PCa)参与者(294人中的282人;95% CI:94%,98%),而放射科医生识别出了98%(294人中的287人;95% CI:96%,99%;P = .23)。该算法分别识别出84%(122人中的103人)、96%(159人中的152人)、96%(49人中的47人)、95%(40人中的38人)和98%(46人中的45人)的ISUP分级1、2、3、4和5级病灶患者。在使用放射科医生作为标准的病灶级分析中,检测敏感性为55%(1029个病灶中的569个;95% CI:52%,58%),阳性预测值(PPV)为57%(934个检测出的病灶中的535个;95% CI:54%,61%)。每位参与者的平均假阳性病灶数为0.61(范围,0–3)。病灶分割的Dice相似系数(DSC)为0.29。
Figure
图
Figure 1: Participant flow diagram. mpMRI = multiparametric MRI, PI-RADS = Prostate Imaging Reporting and Data System.
图1: 参与者流程图。mpMRI = 多参数MRI,PI-RADS = 前列腺影像报告与数据系统。
Figure 2: Distribution of combined biopsy outcomes (percentage) based on highest International Society of Urological Pathology (ISUP) grade group per participant (PT). AI = artificial intelligence, PI-RADS = Prostate Imaging Reporting and Data System.
图2: 根据每位参与者的最高国际泌尿病理学会(ISUP)分级组,显示联合活检结果的分布情况(百分比)。AI = 人工智能,PI-RADS = 前列腺影像报告与数据系统。
Figure 3: Axial multiparametric MRI scans in a 72-year-old male participant with a serum prostate-specific antigen level of 9.1 ng/mL: (A) T2-weighted image, (B)apparent diffusion coefficient map, (C) high-b-value diffusion-weighted image (b = 1500 sec/mm2 ), (D) dynamic contrast-enhanced image (frame 17 of 54 acquired at 5.6-second intervals), (E) T2-weighted image with radiologist-segmented lesions (green contours) overlaid, (F) T2-weighted image with artificial intelligence (AI) prediction map overlaid (red contour is positive prediction; blue contour is AI prostate organ segmentation), and (G) T2-weighted image with AI probability map overlaid (red indicates higher probability). Two distinct lesions were detected by the radiologist and represented the ground truth. Lesion 1 (1.6 cm; arrow in A–D) was in the right midgland transition zone and was designated Prostate Imaging Reporting and Data System (PI-RADS) category 4. Lesion 2 (1.5 cm; arrowhead in A–D) was in the left midgland transition zone and was designated PI-RADS category 3. Lesion 1 was correctly detected (true positive), while lesion 2 was missed by the AI algorithm (false negative). Based on targeted biopsy samples, lesion 1 was positive for Gleason score 7 (3 + 4) prostate adenocarcinoma, and lesion 2 was benign.
图3: 一名72岁男性参与者的轴向多参数MRI扫描,血清前列腺特异性抗原(PSA)水平为9.1 ng/mL:(A) T2加权图像,(B) 表观扩散系数图,(C) 高b值扩散加权图像(b = 1500 sec/mm²),(D) 动态对比增强图像(在5.6秒间隔内获取的54帧中的第17帧),(E) 覆盖放射科医生分割病灶(绿色轮廓)的T2加权图像,(F) 覆盖人工智能(AI)预测图的T2加权图像(红色轮廓为阳性预测;蓝色轮廓为AI前列腺器官分割),以及(G) 覆盖AI概率图的T2加权图像(红色表示更高的概率)。放射科医生检测到两个不同的病灶,并作为标准。病灶1(1.6厘米;A–D中的箭头)位于右侧腺体中部过渡区,被指定为前列腺影像报告与数据系统(PI-RADS)类别4。病灶2(1.5厘米;A–D中的箭头头)位于左侧腺体中部过渡区,被指定为PI-RADS类别3。病灶1被正确检测到(真阳性),而病灶2被AI算法遗漏(假阴性)。根据靶向活检样本,病灶1被确诊为Gleason评分7(3 + 4)的前列腺腺癌,病灶2为良性。
Figure 4: Axial multiparametric MRI scans in a 64-year-old male participant with a serum prostate-specific antigen level of 8.1 ng/mL: (A)T2-weighted image, (B) apparent diffusion coefficient map, (C) high-b-value diffusion-weighted image (b = 1500 sec/mm2 ), (D) dynamic contrastenhanced image (frame 45 of 54 acquired at 5.6-second intervals), (E) T2-weighted image with artificial intelligence (AI) prediction map overlaid (red contour is positive prediction; blue contour is AI prostate organ segmentation), and (F) T2-weighted image with AI probability map overlaid (red indicates higher probability). No distinct lesion was detected by the radiologist (Prostate Imaging Reporting and Data System category 1). One lesion was called by the AI algorithm in the left midgland peripheral zone (arrow in E and F), representing a false positive based on the radiologist ground truth. Systematic biopsy obtained from this site (left midgland lateral) was positive for Gleason score 7 (3 + 4) prostate adenocarcinoma.
图4: 一名64岁男性参与者的轴向多参数MRI扫描,血清前列腺特异性抗原(PSA)水平为8.1 ng/mL:(A) T2加权图像,(B) 表观扩散系数图,(C) 高b值扩散加权图像(b = 1500 sec/mm²),(D) 动态对比增强图像(在5.6秒间隔内获取的54帧中的第45帧),(E) 覆盖人工智能(AI)预测图的T2加权图像(红色轮廓为阳性预测;蓝色轮廓为AI前列腺器官分割),以及(F) 覆盖AI概率图的T2加权图像(红色表示更高的概率)。放射科医生未检测到明显病灶(前列腺影像报告与数据系统类别1)。AI算法在左侧腺体中部外围区检测到一个病灶(E和F中的箭头),根据放射科医生的标准,该病灶被认为是假阳性。来自该部位(左侧腺体中部外侧)的系统性活检结果显示,确诊为Gleason评分7(3 + 4)的前列腺腺癌。
Figure 5: Axial multiparametric MRI scans in a 69-year-old male participant with a serum prostate-specific antigen level of 7.3 ng/mL: (A)T2-weighted image, (B) apparent diffusion coefficient map, (C) high-b-value diffusion-weighted image (b = 1500 sec/mm2 ), (D) dynamic contrastenhanced image (frame 25 of 54 acquired at 5.6-second intervals), (E) T2-weighted image with artificial intelligence (AI) prediction map overlaid (red contour is positive prediction; blue contour is AI prostate organ segmentation), and (F) T2-weighted image with AI probability map overlaid (red indicates higher probability). One lesion was called by the AI algorithm in the left midgland anterior transition zone (arrow in E and F), representing a false positive based on the radiologist ground truth. A systematic biopsy sample obtained from this site (left midgland medial) was benign.
图5: 一名69岁男性参与者的轴向多参数MRI扫描,血清前列腺特异性抗原(PSA)水平为7.3 ng/mL:(A) T2加权图像,(B) 表观扩散系数图,(C) 高b值扩散加权图像(b = 1500 sec/mm²),(D) 动态对比增强图像(在5.6秒间隔内获取的54帧中的第25帧),(E) 覆盖人工智能(AI)预测图的T2加权图像(红色轮廓为阳性预测;蓝色轮廓为AI前列腺器官分割),以及(F) 覆盖AI概率图的T2加权图像(红色表示更高的概率)。AI算法在左侧腺体中部前方过渡区检测到一个病灶(E和F中的箭头),根据放射科医生的标准,该病灶被认为是假阳性。从该部位(左侧腺体中部内侧)获取的系统性活检样本为良性。
Figure 6: Axial multiparametric MRI scans in a 74-year-old male participant with a serum prostate-specific antigen level of 12.9 ng/mL: (A) T2-weighted image, (B) apparent diffusion coefficient map, (C) high-b-value diffusion-weighted image (b = 1500 sec/mm2 ), (D) dynamic contrast-enhanced image (frame 16 of 54 acquired at 5.6-second intervals), (E) T2-weighted image with radiologist-segmented lesion (green contour) overlaid, and (F) T2-weighted image with artificial intelligence (AI) prediction map overlaid (no positive prediction; blue contour is AI prostate organ segmentation). One lesion was detected by the radiologist and represented the ground truth. The lesion (1.9 cm; arrow in A–D) was in the right apical midgland peripheral zone and was designated Prostate Imaging Reporting and Data System category 4. This lesion was missed by the AI algorithm, representing a false negative. A targeted biopsy sample obtained from the lesion was positive for Gleason score 7 (3 + 4) prostate adenocarcinoma.
图6: 一名74岁男性参与者的轴向多参数MRI扫描,血清前列腺特异性抗原(PSA)水平为12.9 ng/mL:(A) T2加权图像,(B) 表观扩散系数图,(C) 高b值扩散加权图像(b = 1500 sec/mm²),(D) 动态对比增强图像(在5.6秒间隔内获取的54帧中的第16帧),(E) 覆盖放射科医生分割病灶(绿色轮廓)的T2加权图像,(F) 覆盖人工智能(AI)预测图的T2加权图像(无阳性预测;蓝色轮廓为AI前列腺器官分割)。放射科医生检测到一个病灶,并作为标准。病灶(1.9厘米;A–D中的箭头)位于右侧顶端腺体中部外围区,被指定为前列腺影像报告与数据系统(PI-RADS)类别4。该病灶被AI算法遗漏,属于假阴性。从该病灶获取的靶向活检样本显示为Gleason评分7(3 + 4)的前列腺腺癌。
Table
表
Table 1: Participant and Lesion Characteristics (n = 658 Participants)
表1: 参与者及病灶特征(n = 658名参与者)
Table 2: Lesion-Level Comparisons of Radiologist and AI Cancer Detection Rates Based on Histopathologic Ground Truth
表2: 基于组织病理学标准的放射科医生与AI癌症检测率的病灶级比较
Table 3: Participant-Level Sensitivity and PPV of AI versus Radiologist Detection of Prostate Cancer Based on Combined Biopsy Results
表3: 基于联合活检结果的AI与放射科医生检测前列腺癌的参与者级敏感性和阳性预测值(PPV)比较
Table 4: Participant-Level Sensitivity and PPV of AI versus Radiologist Detection of Clinically Significant Prostate Cancer Based on Combined Biopsy Results
表4: 基于联合活检结果的AI与放射科医生检测临床意义显著的前列腺癌的参与者级敏感性和阳性预测值(PPV)比较
Table 5: Lesion- and Participant-Level Detection Performance Metrics Based on Radiologist Ground Truth
表5: 基于放射科医生标准的病灶级和参与者级检测性能指标
相关文章:
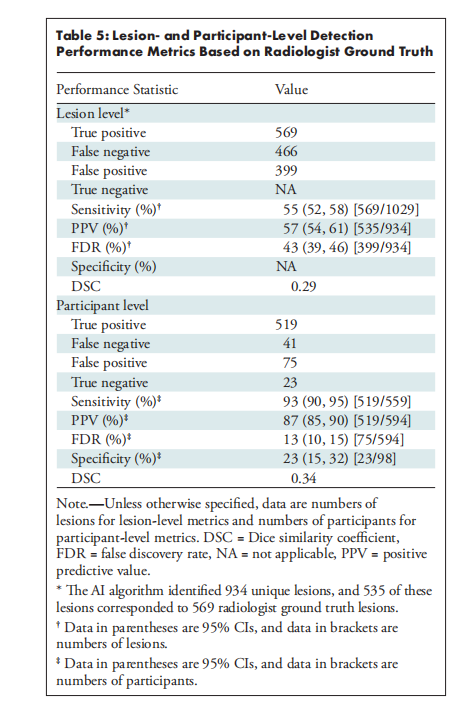
基于级联深度学习算法的前列腺病灶检测在双参数MRI中的评估| 文献速递-基于深度学习的乳房、前列腺疾病诊断系统
Title 题目 Evaluation of a Cascaded Deep Learning–based Algorithm for Prostate Lesion Detection at Biparametric MRI 基于级联深度学习算法的前列腺病灶检测在双参数MRI中的评估 Background 背景 Multiparametric MRI (mpMRI) improves prostate cancer (PCa) de…...
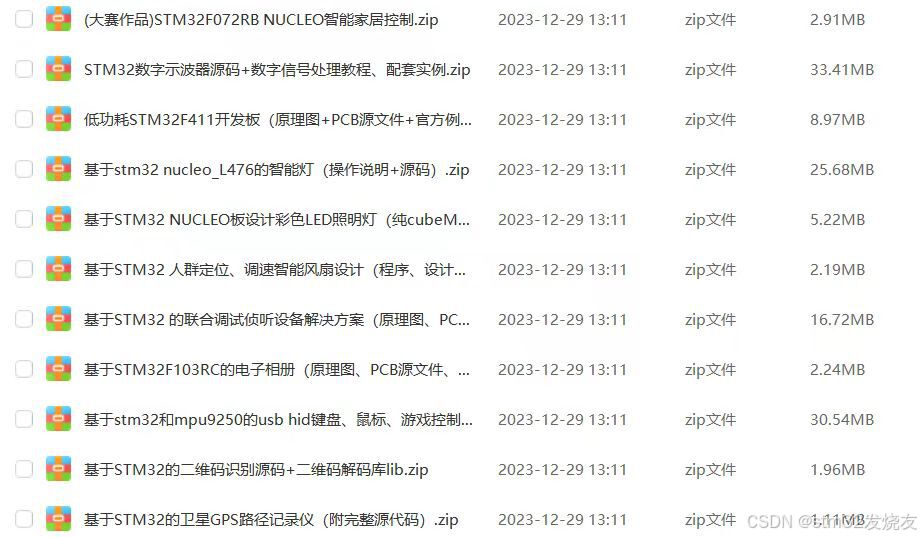
基于STM32开发的智能门铃系统
目录 引言环境准备工作 硬件准备软件安装与配置系统设计 系统架构硬件连接代码实现 初始化代码控制代码应用场景 家庭门铃系统智能社区门禁管理常见问题及解决方案 常见问题解决方案结论 1. 引言 智能门铃系统结合了传统门铃功能与现代技术,通过摄像头、麦克风、…...
【WebRTC指南】远程视频流
远程视频流使用入门 RTCPeerConnection 连接到远程对等设备后,就可以在它们之间流式传输音频和视频。此时,我们会将从 getUserMedia() 收到的数据流连接到 RTCPeerConnection。媒体流包含至少一个媒体轨道,当我们想将媒体传输到远程对等设备时,它们会分别添加到 RTCPeerCo…...
前端构建URL的几种方法比对,以及函数实现
当我们在前端开发中处理 URL 时,可能会用到字符串拼接、ES6 模板语法 (template literals) 或者使用 new URL() 构造函数。这三者各有优劣,适用于不同的场景。 1. 字符串拼接与 ES6 模板语法 字符串拼接 和 ES6 模板语法 都是将不同的字符串片段组合在…...
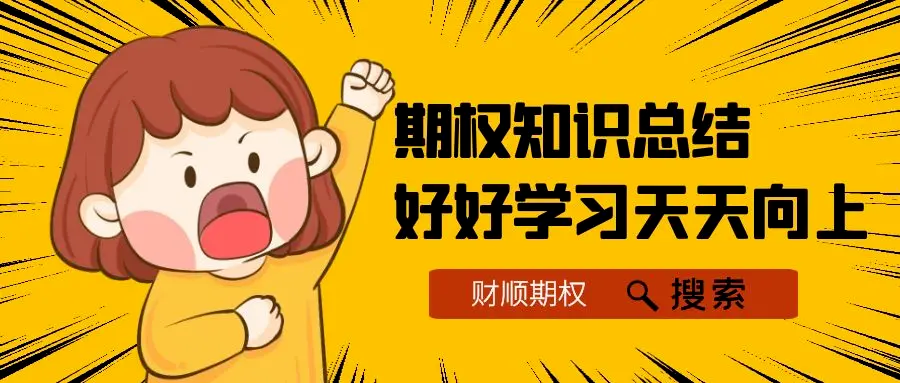
场外个股期权如何发出行权指令?
场外期权行权指令也就是平仓指令的意思,一般场外个股期权交易有三种方式开仓和行权平仓指令,分别是市价,限价和半小时询价,跟普通股票的买卖和交易方式类似,唯一区别是手动发出场外个股期权的行权指令,下文…...
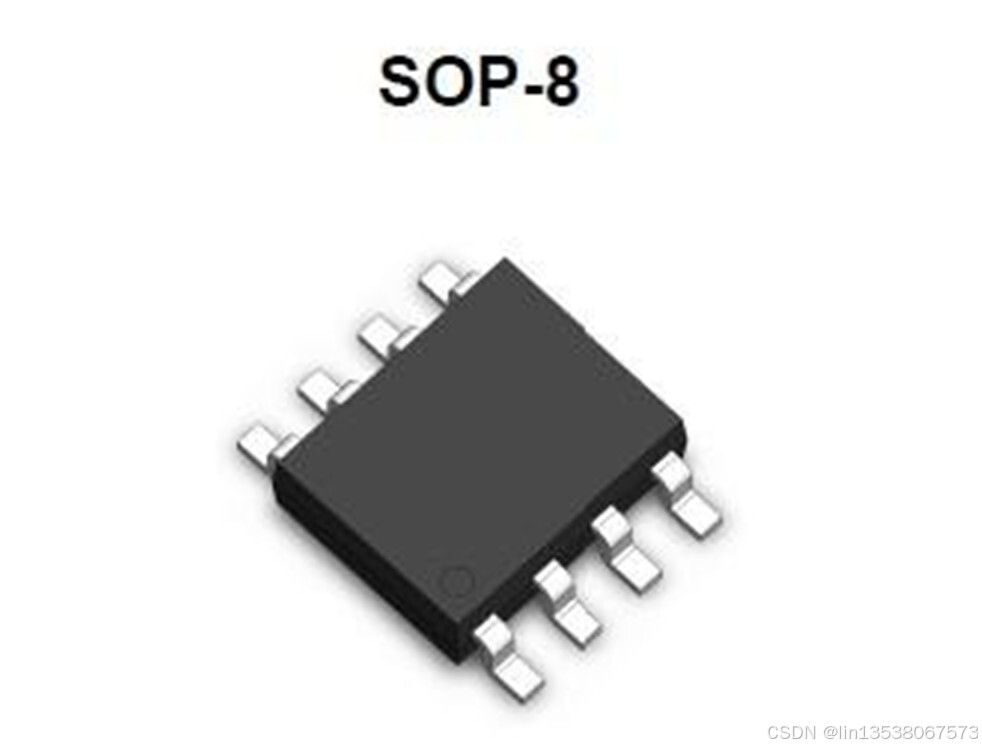
AH8681锂电升压3.7升5V升12V 2A可支持QC2.0 3.0
135.3806.7573在探讨AH8681这款专为3.7V升压5V至12V,并具备2A输出能力,同时兼容QC2.0与QC3.0快充协议的升压芯片时,我们不得不深入其技术细节、应用场景、设计优势以及市场定位等多个维度,以全面理解其在现代电子设备中的重要作用…...
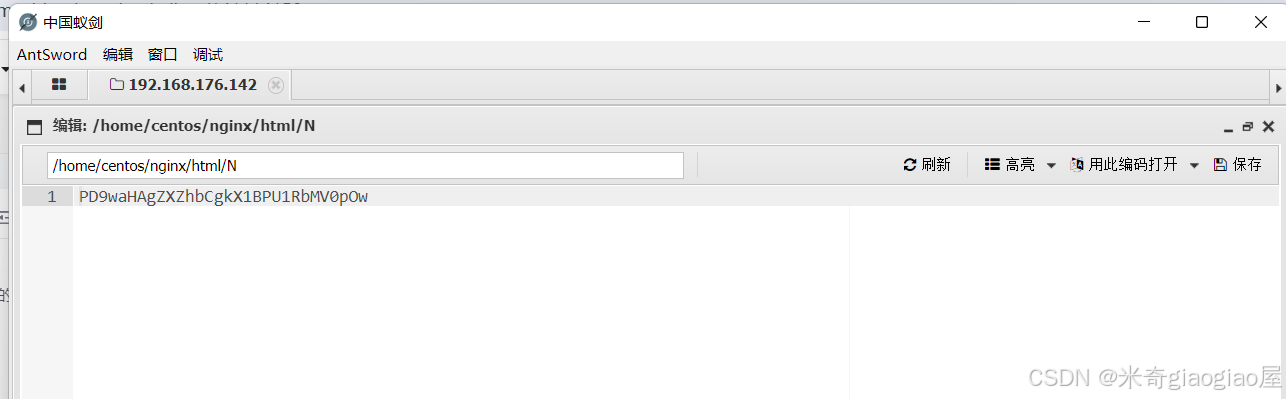
那些年我们一起遇到过的奇技淫巧
EVAL长度限制突破技巧 PHP Eval函数参数限制在16个字符的情况下 ,如何拿到Webshell? 写一段限制长度在小于17位的字符,拿下webshell <?php highlight_file(__FILE__); $param $_REQUEST[param]; if (strlen($param) < 17 &&am…...
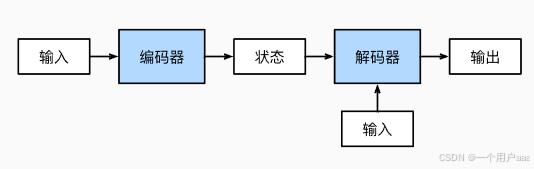
机器学习笔记:编码器与解码器
目录 介绍 组成结构 代码实现 编码器 解码器 合并编码器-解码器 思考 介绍 在机器翻译中,输入的序列与输出的序列经常是长度不相等的序列,此时,像自然语言处理这种直接使用循环神经网络或是门控循环单元的方法就行不通了。因此&#x…...
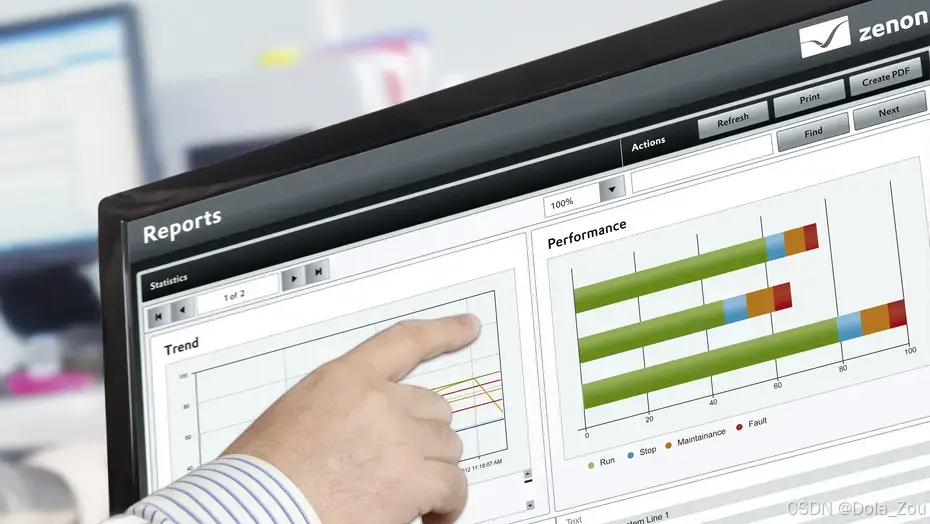
加密狗创新解决方案助力工业自动化
面临的挑战 早在1991年,COPA-DATA就认识到需要一个既能提供长期保护又能灵活应对的解决方案,以防止软件盗版并确保客户在各种复杂的工业环境下能够顺利使用其产品。这一解决方案不仅要兼容Windows系统,还必须在网络连接受限的情况下ÿ…...
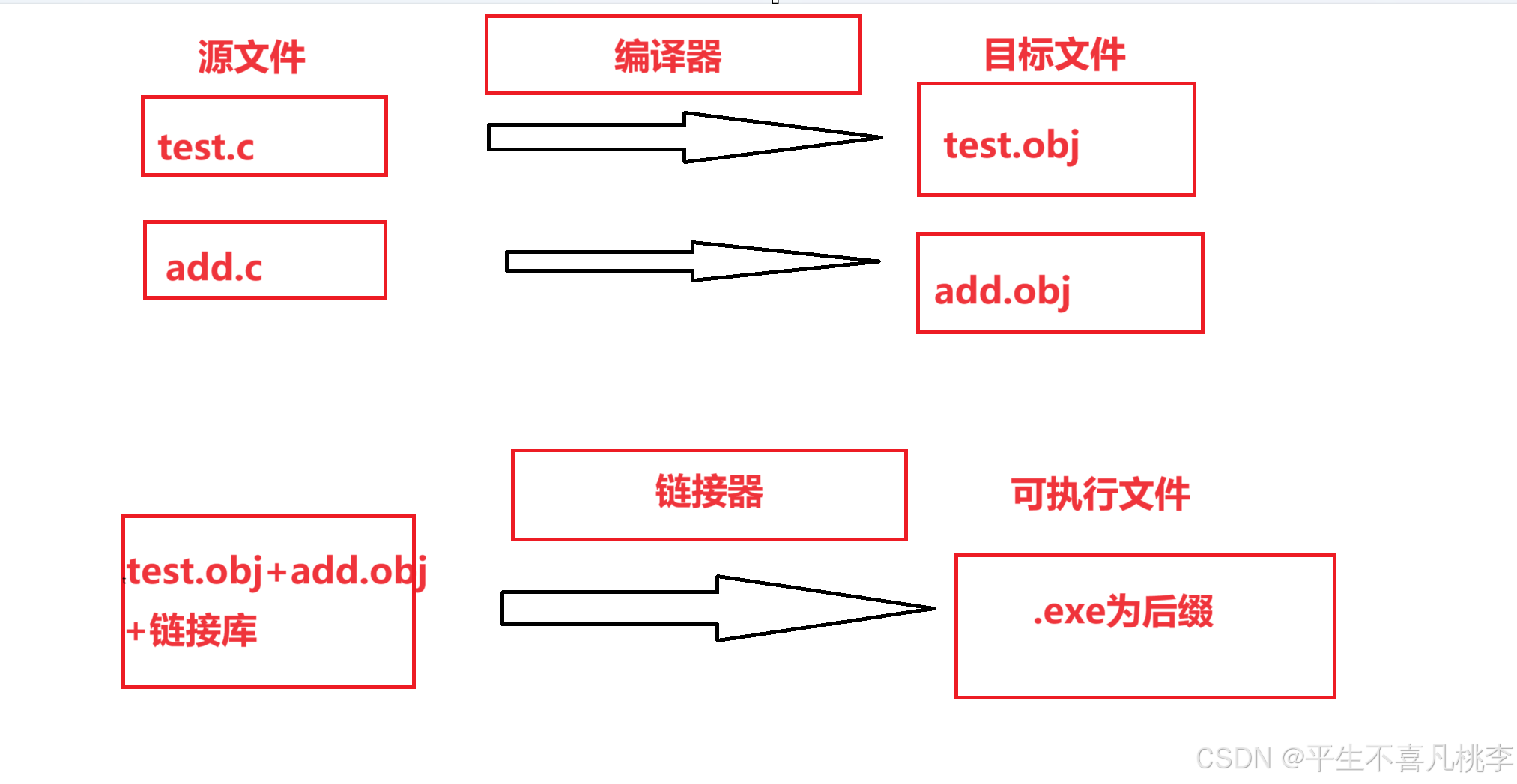
浅谈文件缓冲区和翻译环境
文章目录 1、文件缓冲区2、程序环境A、翻译过程概述B、详解编译和链接a、编译b、链接 1、文件缓冲区 ANSIC 标准采用”文件缓冲系统“处理数据文件,即在文件的读写过程中会使用到文件缓冲区,而文件缓冲区分为输入缓冲区和输出缓冲区。 读写文件 写文件…...
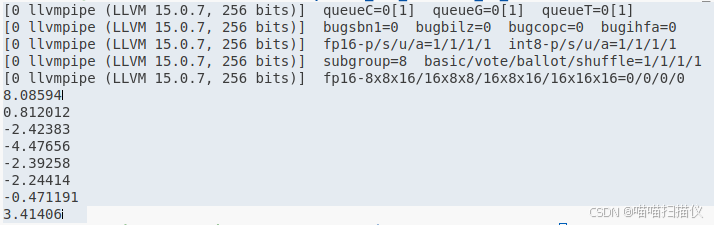
《腾讯NCNN框架的模型转换x86/mips交叉编译推理》详细教程
NCNN的编译运行交叉编译 1.在Ubuntu上编译运行ncnn1)编译ncnn x86 linux2)测试ncnn x86 linux 2. 模型转换1)onnx2)pnnx 3.在x86上加载推理模型1)准备工作2)编写C推理代码3)编写Cmakelist编译 4.在MIPS上进行交叉编译推理1&#x…...
关于近期安卓开发书籍阅读观后感
概述 由于笔者是Java转Android,对于安卓相关知识欠缺,故找一些入门和进阶书籍观看。笔者搜到的相关的安卓推荐博客:【Android – 学习】学习资料汇总_android书籍强烈推荐-CSDN博客相对来说比较全面。 阅读历程 笔者先阅读的是郭霖老师的…...
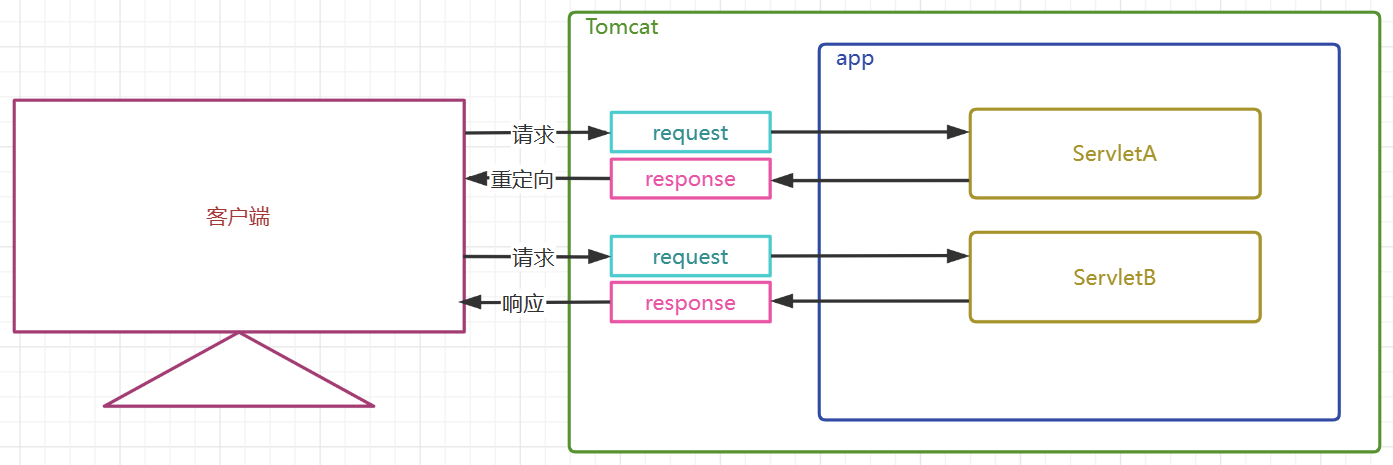
Servlet——个人笔记
Servlet——个人笔记 文章目录 [toc]Servlet简介Servlet命名Servlet由来实现过程 Servlet 相对 CGI 的优势简要说说什么是CGI Servlet 在IDEA中开发流程Servlet注解方式配置WebServlet注解源码WebServlet注解使用 Servlet常见容器Servlet 生命周期简介测试 Servlet 方法init()…...
富格林:戳穿虚假交易保证安全
富格林指出,虚假交易亏损骗局一直以来都是投资者的诟病。不少投资者来到这个赛道的目的铁定是为了安全盈利增值财富,因此如何去杜绝虚假交易便成了当务之急。实际上,有不少投资技巧可以为保障我们的交易安全带来一些庇护。下面富格林就给大家…...
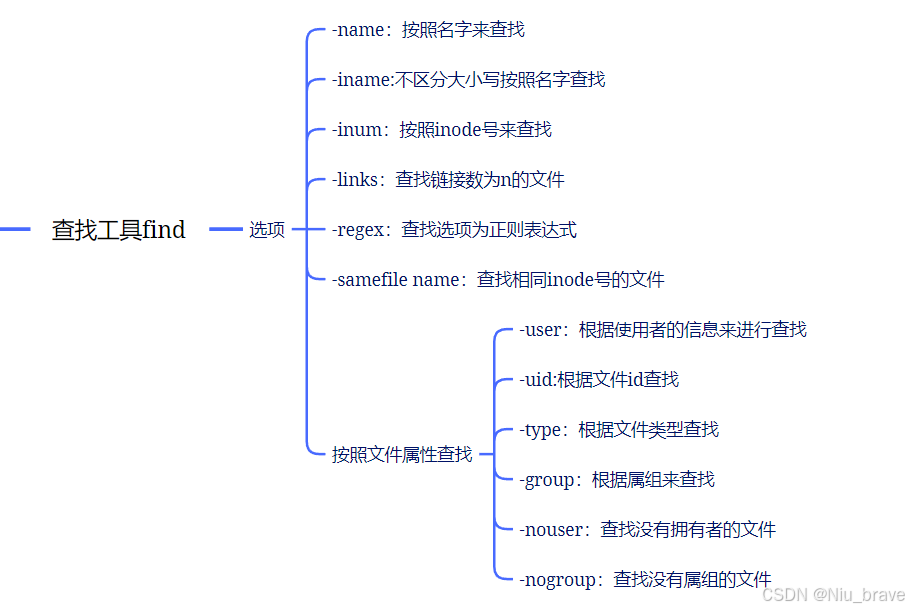
Linux学习——文本处理工具与正则表达式
目录 一,grep 1,grep介绍 2,grep的常用选项 3,grep使用演示 1,基本使用 直接查找字符串: 使用选项 2,使用正则表达式进行匹配 1,正则表达式介绍 2,使用范例 二&…...
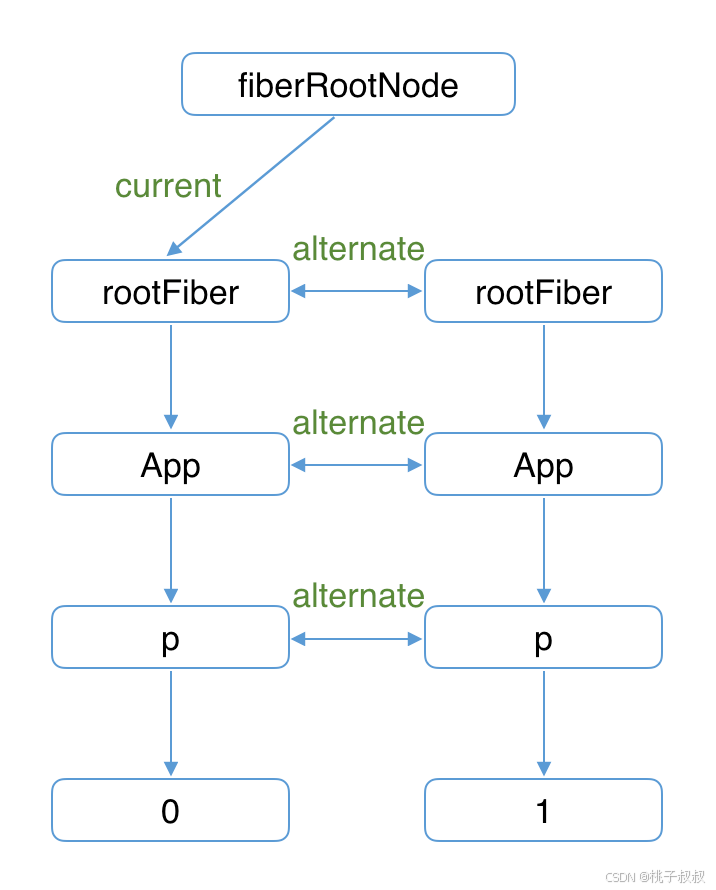
大厂进阶四:React源码Fiber架构解析
本文主要内容: 1、React Concurrent 2、React15架构 3、React16架构 4、Fiber架构 5、任务调度循环和fiber构造循环区别 一、React Concurrent React在解决CPU卡顿是会用到React Concurrent的概念,它是React中的一个重要特性和模块,主要的…...
MongoDB的WiredTiger存储引擎
作者:太阳 从MongoDB 3.2 开始,MongoDB实例默认的存储引擎为WiredTiger,WiredTiger存储引擎具体以下几大优点: 文档级并发 将数据持久化到磁盘 快照和checkpoint 数据压缩 本地数据加密 一、文档级别并发 1、WiredTiger使…...
windows 版本Jenkins的Jenkinsfile中共享变量
场景 jenkins部署在windows服务器上的,需要在Jenkinsfile中获取命令执行的结果存入一个变量,然后在后续的执行中使用此变量 一开始想的是定义一个环境变量,如下所示, pipeline {agent anystages {stage(test) {steps {bat for /…...
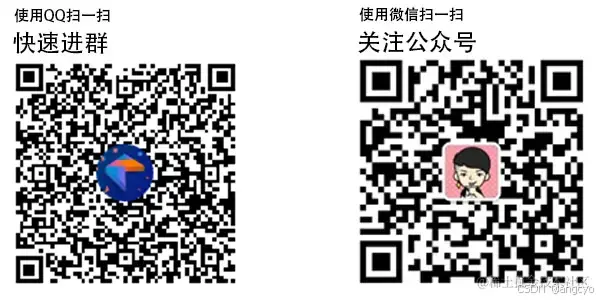
Android-->产物收集(含apk文件重命名, aab文件重命名)
以前写过修改apk生成路径和文件名的文章, 如下: AS–›Gradle 7.0.0/4.1.0/4.0/3.3/3.0 修改APK生成路径和文件名(附AAR修改方式以及分析过程)_com.android.build.gradle.internal.api.libraryvaria-CSDN博客 这种方法入侵了gradle,破坏了原有的gradle环境, 经常会导致如下问…...
matlab实现迷宫最佳路径规划
在MATLAB中实现迷宫路径的最佳路径规划,我们可以使用多种算法,其中最常见和高效的是A搜索算法(A Search Algorithm)。A*算法结合了最佳优先搜索和Dijkstra算法的优点,通过启发式函数来评估每个节点的优先级,…...
React 第五十五节 Router 中 useAsyncError的使用详解
前言 useAsyncError 是 React Router v6.4 引入的一个钩子,用于处理异步操作(如数据加载)中的错误。下面我将详细解释其用途并提供代码示例。 一、useAsyncError 用途 处理异步错误:捕获在 loader 或 action 中发生的异步错误替…...
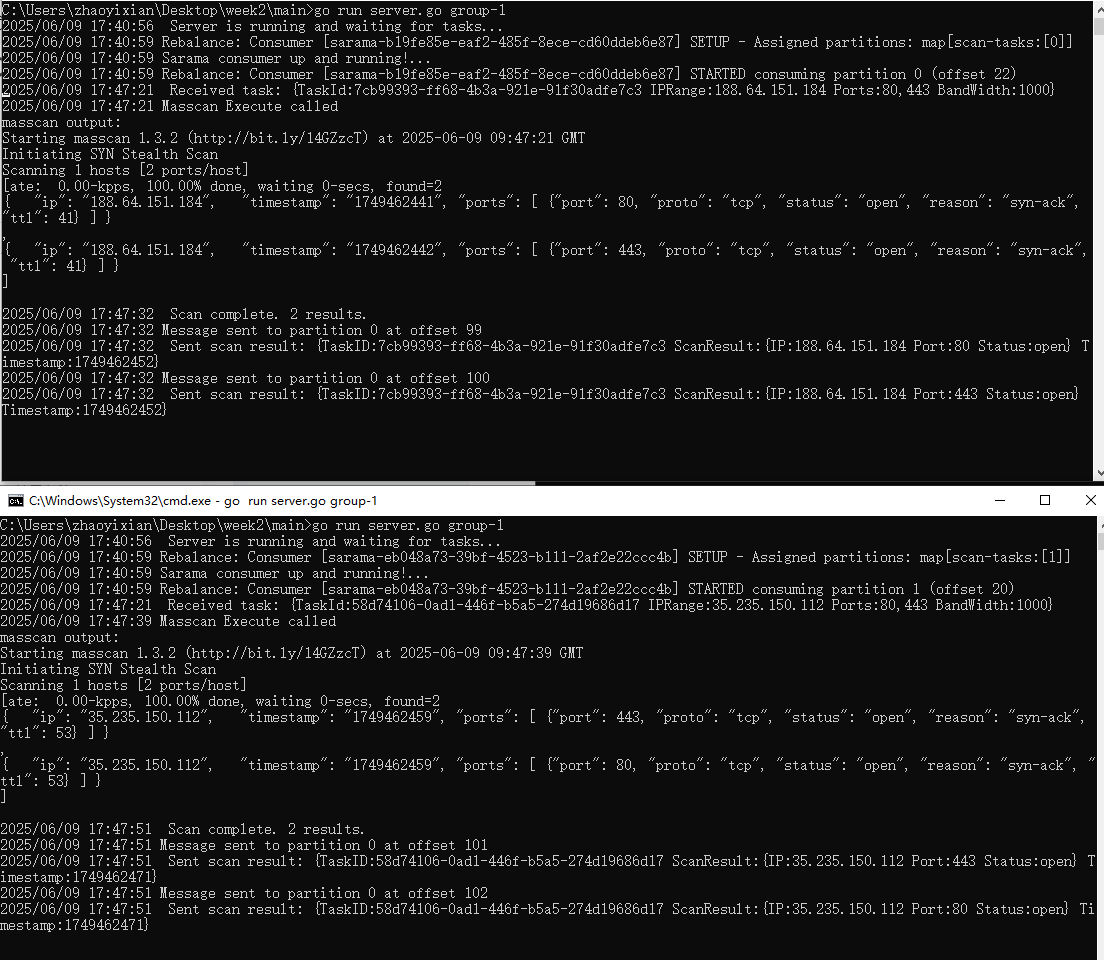
【kafka】Golang实现分布式Masscan任务调度系统
要求: 输出两个程序,一个命令行程序(命令行参数用flag)和一个服务端程序。 命令行程序支持通过命令行参数配置下发IP或IP段、端口、扫描带宽,然后将消息推送到kafka里面。 服务端程序: 从kafka消费者接收…...
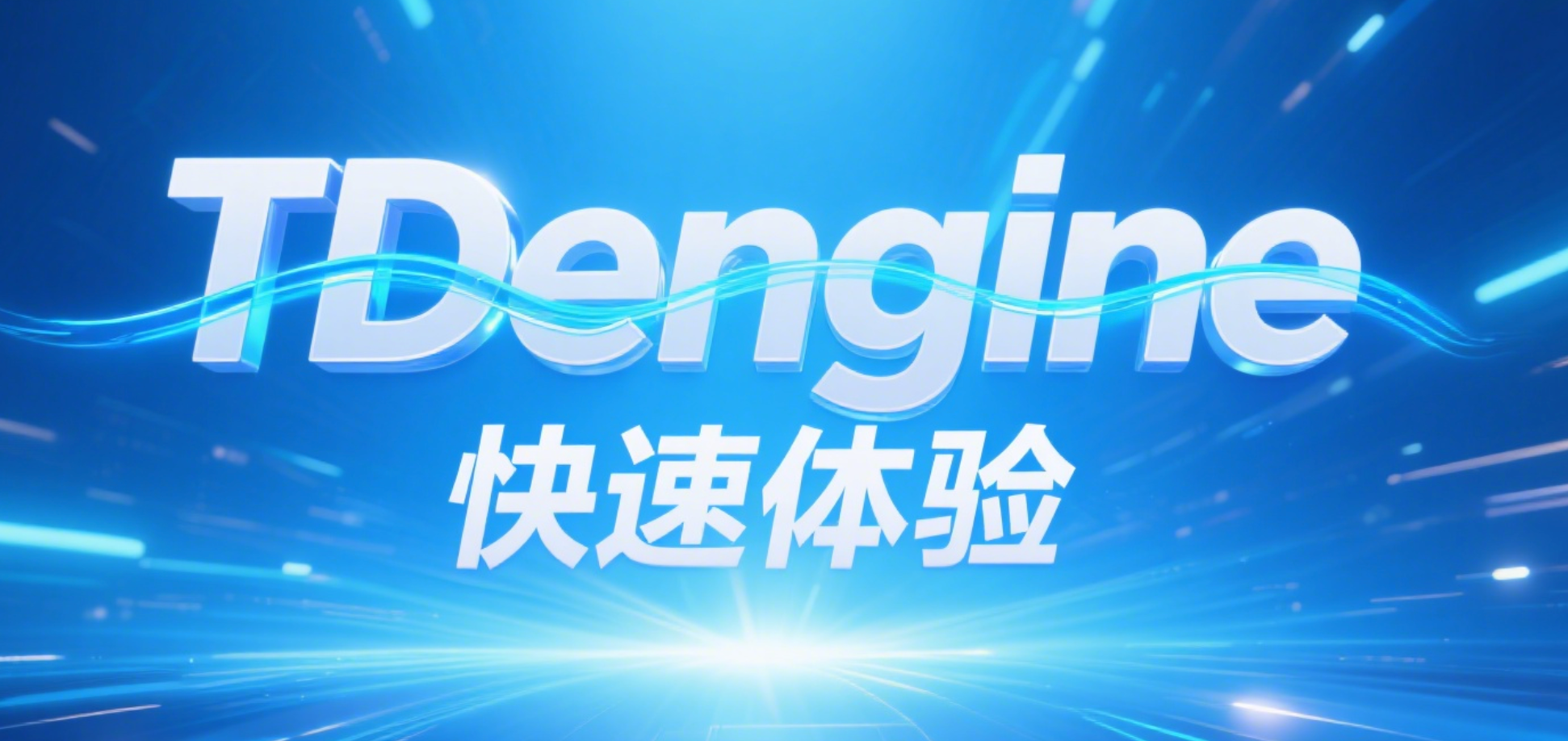
TDengine 快速体验(Docker 镜像方式)
简介 TDengine 可以通过安装包、Docker 镜像 及云服务快速体验 TDengine 的功能,本节首先介绍如何通过 Docker 快速体验 TDengine,然后介绍如何在 Docker 环境下体验 TDengine 的写入和查询功能。如果你不熟悉 Docker,请使用 安装包的方式快…...
在 Nginx Stream 层“改写”MQTT ngx_stream_mqtt_filter_module
1、为什么要修改 CONNECT 报文? 多租户隔离:自动为接入设备追加租户前缀,后端按 ClientID 拆分队列。零代码鉴权:将入站用户名替换为 OAuth Access-Token,后端 Broker 统一校验。灰度发布:根据 IP/地理位写…...
鸿蒙中用HarmonyOS SDK应用服务 HarmonyOS5开发一个生活电费的缴纳和查询小程序
一、项目初始化与配置 1. 创建项目 ohpm init harmony/utility-payment-app 2. 配置权限 // module.json5 {"requestPermissions": [{"name": "ohos.permission.INTERNET"},{"name": "ohos.permission.GET_NETWORK_INFO"…...
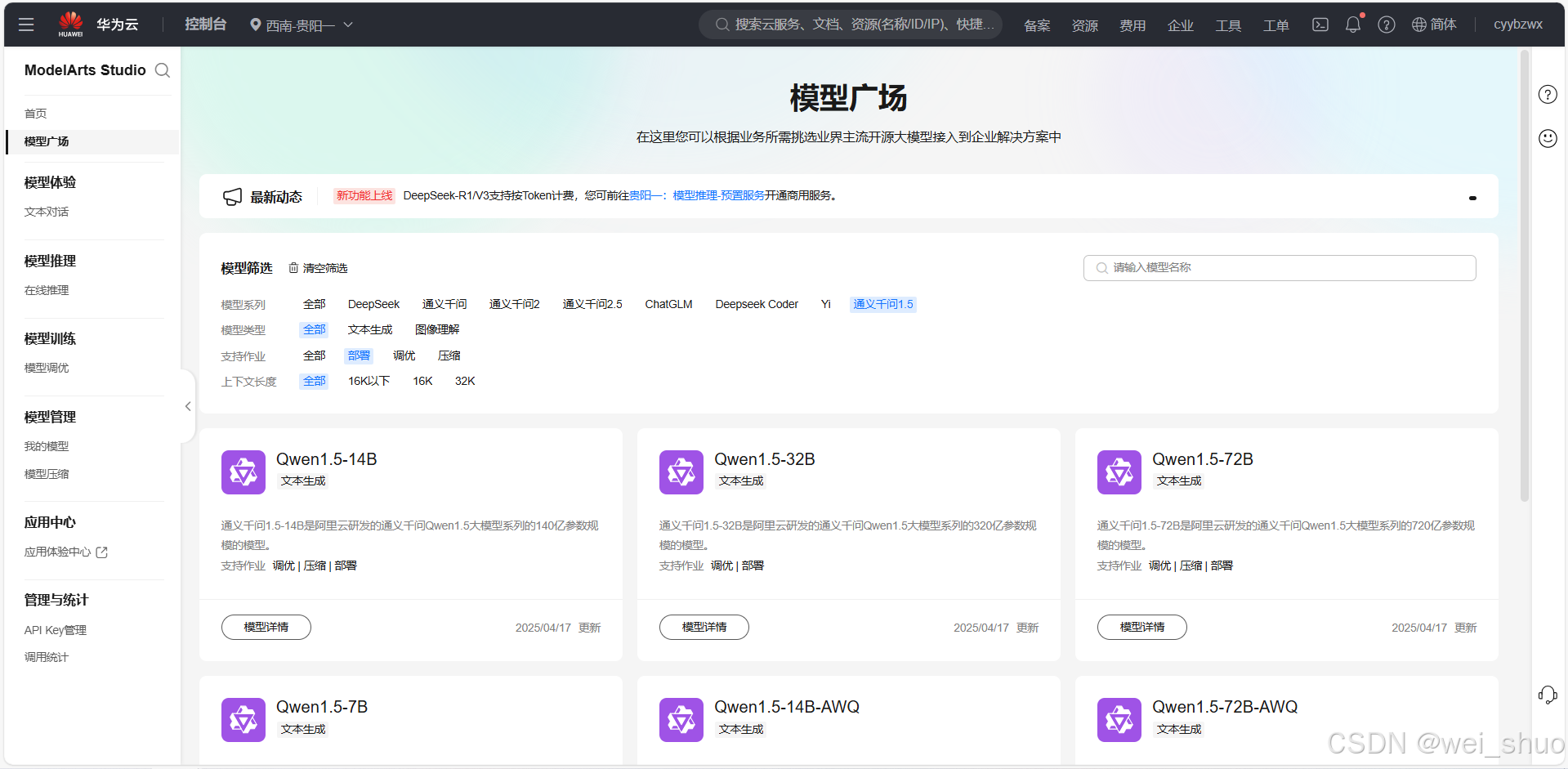
华为云Flexus+DeepSeek征文|DeepSeek-V3/R1 商用服务开通全流程与本地部署搭建
华为云FlexusDeepSeek征文|DeepSeek-V3/R1 商用服务开通全流程与本地部署搭建 前言 如今大模型其性能出色,华为云 ModelArts Studio_MaaS大模型即服务平台华为云内置了大模型,能助力我们轻松驾驭 DeepSeek-V3/R1,本文中将分享如何…...
Spring AI与Spring Modulith核心技术解析
Spring AI核心架构解析 Spring AI(https://spring.io/projects/spring-ai)作为Spring生态中的AI集成框架,其核心设计理念是通过模块化架构降低AI应用的开发复杂度。与Python生态中的LangChain/LlamaIndex等工具类似,但特别为多语…...
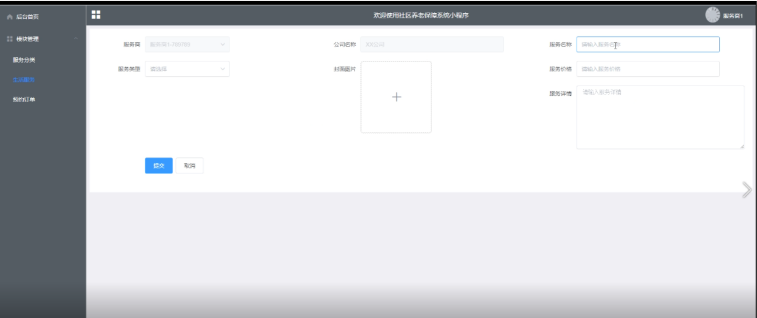
Springboot社区养老保险系统小程序
一、前言 随着我国经济迅速发展,人们对手机的需求越来越大,各种手机软件也都在被广泛应用,但是对于手机进行数据信息管理,对于手机的各种软件也是备受用户的喜爱,社区养老保险系统小程序被用户普遍使用,为方…...
【生成模型】视频生成论文调研
工作清单 上游应用方向:控制、速度、时长、高动态、多主体驱动 类型工作基础模型WAN / WAN-VACE / HunyuanVideo控制条件轨迹控制ATI~镜头控制ReCamMaster~多主体驱动Phantom~音频驱动Let Them Talk: Audio-Driven Multi-Person Conversational Video Generation速…...
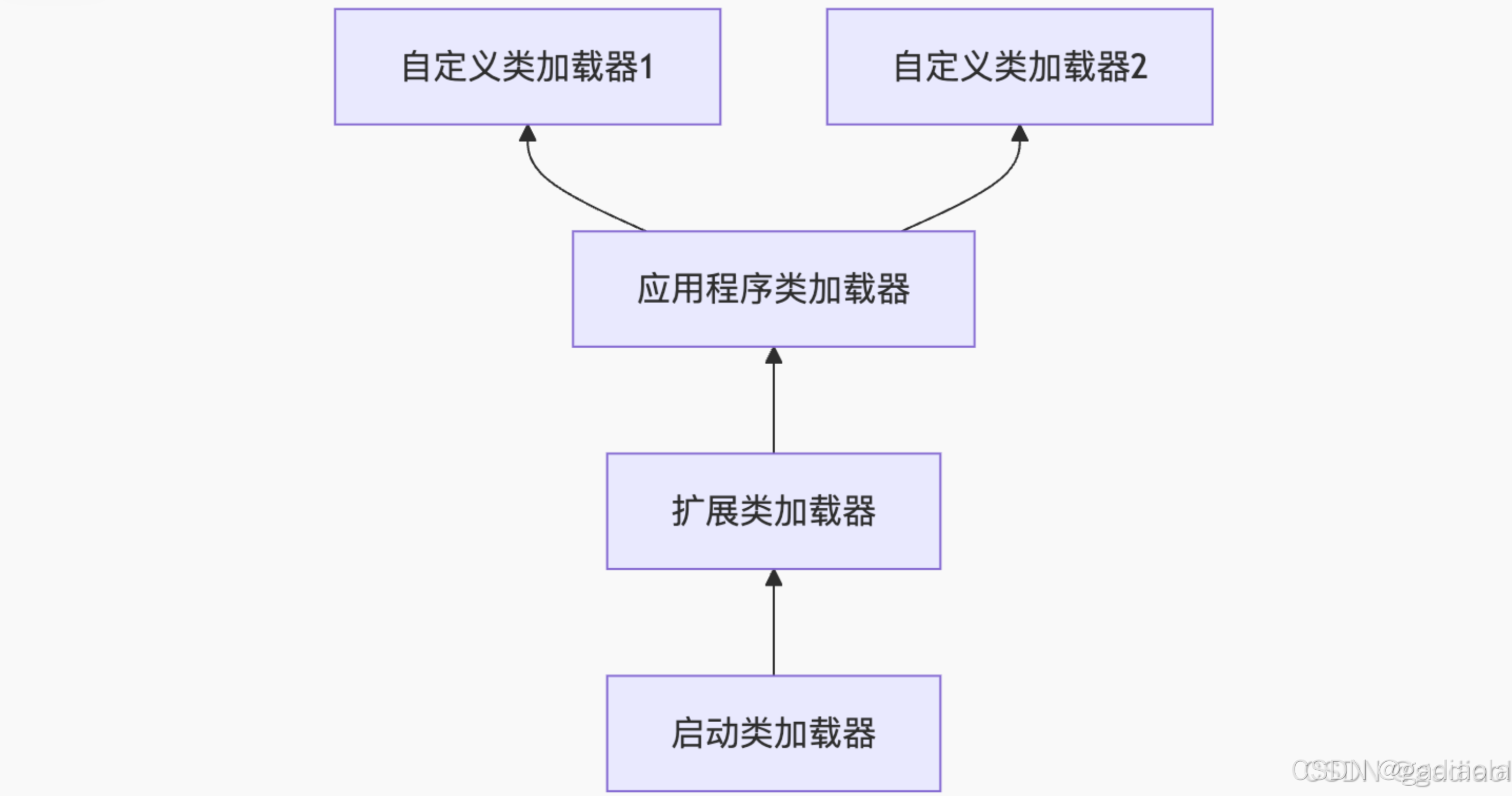
【JVM面试篇】高频八股汇总——类加载和类加载器
目录 1. 讲一下类加载过程? 2. Java创建对象的过程? 3. 对象的生命周期? 4. 类加载器有哪些? 5. 双亲委派模型的作用(好处)? 6. 讲一下类的加载和双亲委派原则? 7. 双亲委派模…...