MetaGPT源码 (ContextMixin 类)
目录
- 理解 ContextMixin
- 什么是 ContextMixin?
- 主要组件
- 实现细节
- 测试 ContextMixin
- 示例:ModelX
- 1. 配置优先级
- 2. 多继承
- 3. 多继承重写
- 4. 配置优先级
在本文中,我们将探索 ContextMixin 类,它在多重继承场景中的集成及其在 Python 配置和上下文管理中的应用。此外,我们将通过测试验证其功能,以了解它如何简化模型配置的处理。让我们深入了解代码片段的详细解释。
理解 ContextMixin
什么是 ContextMixin?
ContextMixin
是一个用于高效管理上下文和配置的 Python 类。继承该类的模型或对象能够:
- 通过灵活的优先级规则处理上下文(
private_context
)和配置(private_config
)。 - 管理与 LLM(
private_llm
)实例的交互。 - 支持动态设置属性的覆盖机制。
主要组件
-
私有上下文和配置:
private_context
和private_config
被设计为内部属性,为每个实例提供灵活的作用域。- 这些属性默认值为
None
,但可以显式覆盖。
-
LLM 管理:
- 通过
private_llm
集成 LLM,支持从配置动态初始化。
- 通过
实现细节
以下是核心 ContextMixin
类:
from typing import Optionalfrom pydantic import BaseModel, ConfigDict, Field, model_validatorfrom metagpt.config2 import Config
from metagpt.context import Context
from metagpt.provider.base_llm import BaseLLMclass ContextMixin(BaseModel):"""Mixin class for context and config"""model_config = ConfigDict(arbitrary_types_allowed=True, extra="allow")# Pydantic has bug on _private_attr when using inheritance, so we use private_* instead# - https://github.com/pydantic/pydantic/issues/7142# - https://github.com/pydantic/pydantic/issues/7083# - https://github.com/pydantic/pydantic/issues/7091# Env/Role/Action will use this context as private context, or use self.context as public contextprivate_context: Optional[Context] = Field(default=None, exclude=True)# Env/Role/Action will use this config as private config, or use self.context.config as public configprivate_config: Optional[Config] = Field(default=None, exclude=True)# Env/Role/Action will use this llm as private llm, or use self.context._llm instanceprivate_llm: Optional[BaseLLM] = Field(default=None, exclude=True)@model_validator(mode="after")def validate_context_mixin_extra(self):self._process_context_mixin_extra()return selfdef _process_context_mixin_extra(self):"""Process the extra field"""kwargs = self.model_extra or {}self.set_context(kwargs.pop("context", None))self.set_config(kwargs.pop("config", None))self.set_llm(kwargs.pop("llm", None))def set(self, k, v, override=False):"""Set attribute"""if override or not self.__dict__.get(k):self.__dict__[k] = vdef set_context(self, context: Context, override=True):"""Set context"""self.set("private_context", context, override)def set_config(self, config: Config, override=False):"""Set config"""self.set("private_config", config, override)if config is not None:_ = self.llm # init llmdef set_llm(self, llm: BaseLLM, override=False):"""Set llm"""self.set("private_llm", llm, override)@propertydef config(self) -> Config:"""Role config: role config > context config"""if self.private_config:return self.private_configreturn self.context.config@config.setterdef config(self, config: Config) -> None:"""Set config"""self.set_config(config)@propertydef context(self) -> Context:"""Role context: role context > context"""if self.private_context:return self.private_contextreturn Context()@context.setterdef context(self, context: Context) -> None:"""Set context"""self.set_context(context)@propertydef llm(self) -> BaseLLM:"""Role llm: if not existed, init from role.config"""# print(f"class:{self.__class__.__name__}({self.name}), llm: {self._llm}, llm_config: {self._llm_config}")if not self.private_llm:self.private_llm = self.context.llm_with_cost_manager_from_llm_config(self.config.llm)return self.private_llm@llm.setterdef llm(self, llm: BaseLLM) -> None:"""Set llm"""self.private_llm = llm
ContextMixin
通过 Pydantic 进行模型验证和数据管理,在处理任意字段时提供了灵活性。
测试 ContextMixin
示例:ModelX
为了演示 ContextMixin
的工作原理,我们创建了一个简单的模型 ModelX
,继承自 ContextMixin
, 验证 ModelX
能正确继承默认属性,同时保留 ContextMixin
的功能。
ContextMixin
可以无缝集成到多重继承的层次结构中,
ModelY
结合了 ContextMixin
和 WTFMixin
,继承了两者的字段和功能。
class ModelX(ContextMixin, BaseModel):a: str = "a"b: str = "b"class WTFMixin(BaseModel):c: str = "c"d: str = "d"class ModelY(WTFMixin, ModelX):passdef test_config_mixin_1():new_model = ModelX()assert new_model.a == "a"assert new_model.b == "b"
test_config_mixin_1()
1. 配置优先级
from metagpt.configs.llm_config import LLMConfigmock_llm_config = LLMConfig(llm_type="mock",api_key="mock_api_key",base_url="mock_base_url",app_id="mock_app_id",api_secret="mock_api_secret",domain="mock_domain",
)
mock_llm_config_proxy = LLMConfig(llm_type="mock",api_key="mock_api_key",base_url="mock_base_url",proxy="http://localhost:8080",
)def test_config_mixin_2():i = Config(llm=mock_llm_config)j = Config(llm=mock_llm_config_proxy)obj = ModelX(config=i)assert obj.config == iassert obj.config.llm == mock_llm_configobj.set_config(j)# obj already has a config, so it will not be setassert obj.config == i
test_config_mixin_2()
2. 多继承
def test_config_mixin_3_multi_inheritance_not_override_config():"""Test config mixin with multiple inheritance"""i = Config(llm=mock_llm_config)j = Config(llm=mock_llm_config_proxy)obj = ModelY(config=i)assert obj.config == iassert obj.config.llm == mock_llm_configobj.set_config(j)# obj already has a config, so it will not be setassert obj.config == iassert obj.config.llm == mock_llm_configassert obj.a == "a"assert obj.b == "b"assert obj.c == "c"assert obj.d == "d"print(obj.__dict__.keys())print(obj.__dict__)assert "private_config" in obj.__dict__.keys()test_config_mixin_3_multi_inheritance_not_override_config()
dict_keys(['private_context', 'private_config', 'private_llm', 'a', 'b', 'c', 'd'])
{'private_context': None, 'private_config': Config(extra_fields=None, project_path='', project_name='', inc=False, reqa_file='', max_auto_summarize_code=0, git_reinit=False, llm=LLMConfig(extra_fields=None, api_key='mock_api_key', api_type=<LLMType.OPENAI: 'openai'>, base_url='mock_base_url', api_version=None, model=None, pricing_plan=None, access_key=None, secret_key=None, session_token=None, endpoint=None, app_id='mock_app_id', api_secret='mock_api_secret', domain='mock_domain', max_token=4096, temperature=0.0, top_p=1.0, top_k=0, repetition_penalty=1.0, stop=None, presence_penalty=0.0, frequency_penalty=0.0, best_of=None, n=None, stream=True, seed=None, logprobs=None, top_logprobs=None, timeout=600, context_length=None, region_name=None, proxy=None, calc_usage=True, use_system_prompt=True), embedding=EmbeddingConfig(extra_fields=None, api_type=None, api_key=None, base_url=None, api_version=None, model=None, embed_batch_size=None, dimensions=None), omniparse=OmniParseConfig(extra_fields=None, api_key='', base_url=''), proxy='', search=SearchConfig(extra_fields=None, api_type=<SearchEngineType.DUCK_DUCK_GO: 'ddg'>, api_key='', cse_id='', search_func=None, params={'engine': 'google', 'google_domain': 'google.com', 'gl': 'us', 'hl': 'en'}), browser=BrowserConfig(extra_fields=None, engine=<WebBrowserEngineType.PLAYWRIGHT: 'playwright'>, browser_type='chromium'), mermaid=MermaidConfig(extra_fields=None, engine='nodejs', path='mmdc', puppeteer_config='', pyppeteer_path='/usr/bin/google-chrome-stable'), s3=None, redis=None, repair_llm_output=False, prompt_schema='json', workspace=WorkspaceConfig(extra_fields=None, path=WindowsPath('d:/llm/metagpt/workspace'), use_uid=False, uid=''), enable_longterm_memory=False, code_review_k_times=2, agentops_api_key='', metagpt_tti_url='', language='English', redis_key='placeholder', iflytek_app_id='', iflytek_api_secret='', iflytek_api_key='', azure_tts_subscription_key='', azure_tts_region=''), 'private_llm': <metagpt.provider.openai_api.OpenAILLM object at 0x00000128F0753910>, 'a': 'a', 'b': 'b', 'c': 'c', 'd': 'd'}
3. 多继承重写
mock_llm_config_zhipu = LLMConfig(llm_type="zhipu",api_key="mock_api_key.zhipu",base_url="mock_base_url",model="mock_zhipu_model",proxy="http://localhost:8080",
)def test_config_mixin_4_multi_inheritance_override_config():"""Test config mixin with multiple inheritance"""i = Config(llm=mock_llm_config)j = Config(llm=mock_llm_config_zhipu)obj = ModelY(config=i)assert obj.config == iassert obj.config.llm == mock_llm_configobj.set_config(j, override=True)# override obj.configassert obj.config == jassert obj.config.llm == mock_llm_config_zhipuassert obj.a == "a"assert obj.b == "b"assert obj.c == "c"assert obj.d == "d"print(obj.__dict__.keys())assert "private_config" in obj.__dict__.keys()assert obj.config.llm.model == "mock_zhipu_model"
test_config_mixin_4_multi_inheritance_override_config()
dict_keys(['private_context', 'private_config', 'private_llm', 'a', 'b', 'c', 'd'])
4. 配置优先级
from pathlib import Path
import pytest
from metagpt.actions import Action
from metagpt.config2 import Config
from metagpt.const import CONFIG_ROOT
from metagpt.environment import Environment
from metagpt.roles import Role
from metagpt.team import Team@pytest.mark.asyncio
async def test_config_priority():"""If action's config is set, then its llm will be set, otherwise, it will use the role's llm"""home_dir = Path.home() / CONFIG_ROOTgpt4t = Config.from_home("gpt-4-turbo.yaml")if not home_dir.exists():assert gpt4t is Nonegpt35 = Config.default()gpt35.llm.model = "gpt35"gpt4 = Config.default()gpt4.llm.model = "gpt-4-0613"a1 = Action(name="Say", instruction="Say your opinion with emotion and don't repeat it", config=gpt4t)a2 = Action(name="Say", instruction="Say your opinion with emotion and don't repeat it")a3 = Action(name="Vote", instruction="Vote for the candidate, and say why you vote for him/her")# it will not work for a1 because the config is already setA = Role(name="A", profile="Democratic candidate", goal="Win the election", actions=[a1], watch=[a2], config=gpt4)# it will work for a2 because the config is not setB = Role(name="B", profile="Republican candidate", goal="Win the election", actions=[a2], watch=[a1], config=gpt4)# dittoC = Role(name="C", profile="Voter", goal="Vote for the candidate", actions=[a3], watch=[a1, a2], config=gpt35)env = Environment(desc="US election live broadcast")Team(investment=10.0, env=env, roles=[A, B, C])assert a1.llm.model == "gpt-4-turbo" if Path(home_dir / "gpt-4-turbo.yaml").exists() else "gpt-4-0613"assert a2.llm.model == "gpt-4-0613"assert a3.llm.model == "gpt35"await test_config_priority()
如果有任何问题,欢迎在评论区提问。
相关文章:
MetaGPT源码 (ContextMixin 类)
目录 理解 ContextMixin什么是 ContextMixin?主要组件实现细节 测试 ContextMixin示例:ModelX1. 配置优先级2. 多继承3. 多继承重写4. 配置优先级 在本文中,我们将探索 ContextMixin 类,它在多重继承场景中的集成及其在 Python 配…...
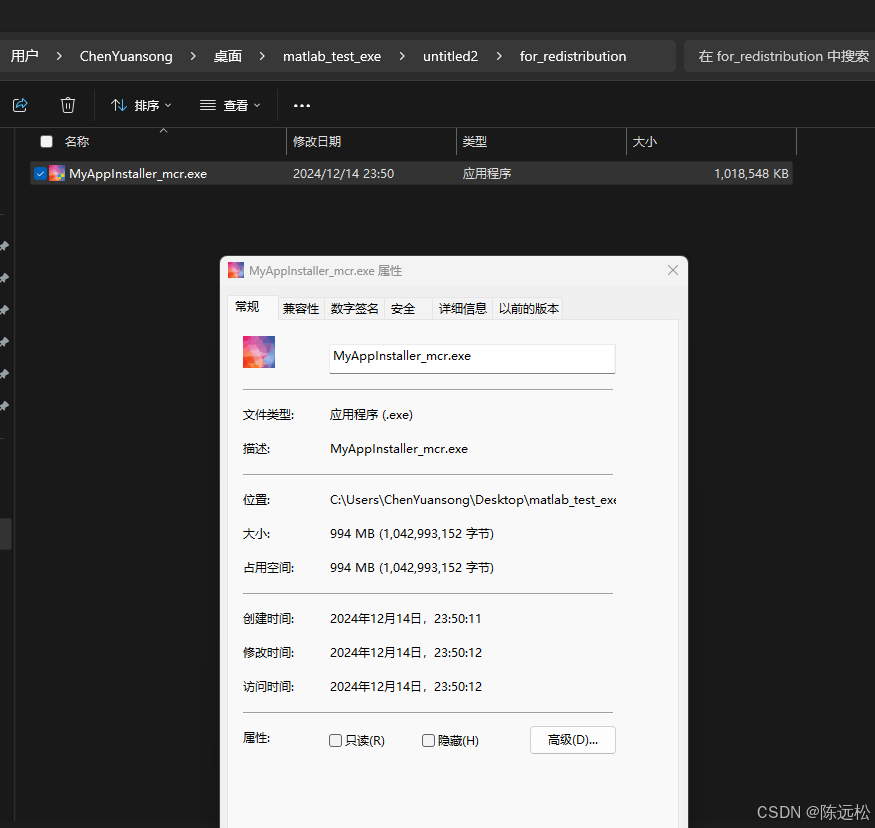
MATLAB生成.exe独立程序过程(常见问题解决方法)(2024.12.14)
本文只记录我执行过程中遇到的关键问题、以及解决方法,不讲诉整个流程。 电脑环境 win11系统 matlab 2024b 版本 整体流程 1.下载matlab运行时库,简写为MCR 2.配置MCR环境 3.打包程序 4.目标机器安装程序 一、下载MCR 下载这个折腾了大半天,大概问题就是…...
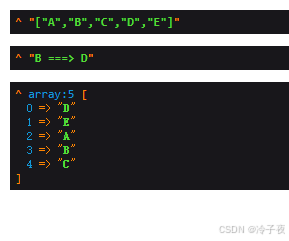
PHP排序算法:数组内有A~E,A移到C或者C移到B后排序,还按原顺序排序,循环
效果 PHP代码 public function demo($params){function moveNext($arr){$length count($arr);$lastElement $arr[$length - 1];for ($i $length - 1; $i > 0; $i--) {$arr[$i] $arr[$i - 1];}$arr[0] $lastElement;return $arr;}function moveAndReplace($array, $from…...
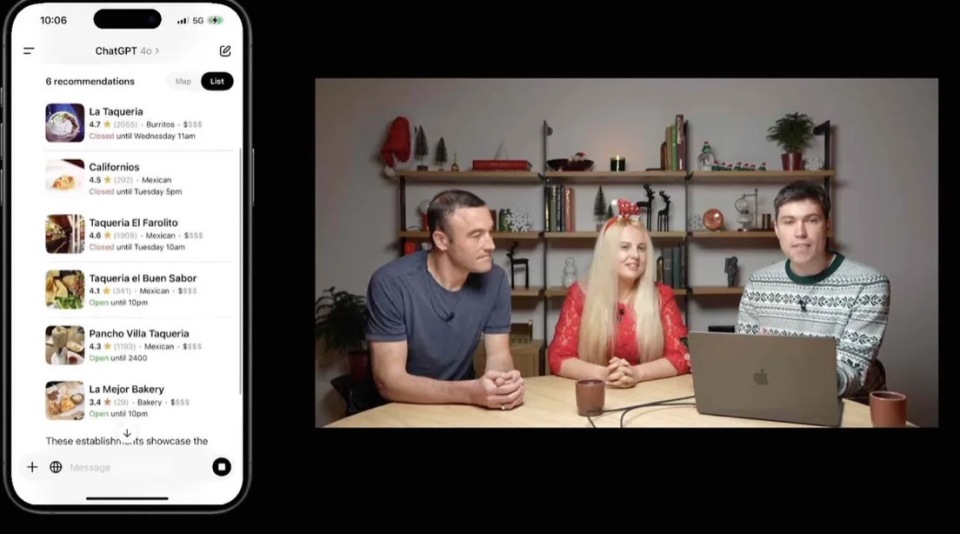
ChatGPT搜索全新升级,向全体用户开放,近屿智能助力AI行业发展
12月17日,OpenAI在第八天直播中正式宣布ChatGPT搜索功能全面升级,并即日起对所有ChatGPT用户开放。此次更新不仅带来了显著的性能提升,还引入了多项突破性功能,如更快的搜索速度、全新的地图体验以及YouTube视频嵌入,为…...

win10配置免密ssh登录远程的ubuntu
为了在终端ssh远程和使用VScode远程我的VM上的ubuntu不需要设置密码,需要在win10配置免密ssh登录远程的ubuntu。 在win10打开cmd,执行下面的代码生成密钥对(会提示进行设置,按照默认的配置就行,一直回车)&…...
skywalking 搭建 备忘录
基础环境 apache-skywalking-apm-9.6.0.tar.gz apache-skywalking-java-agent-9.1.0.tgz elasticsearch 7.14.1 采用dockers搭建 或者手动部署 kibana 可视化 应用 微服务版 consumer.jar eureka.jar 注册中心 provider.jar skywalking 地址 https://skywalkin…...
linux日常常用命令(AI向)
进程挂后台运行 nohup sh ./scripts/*****.sh > ./output/*****.log 2>&1 &删除***用户的所有python进程 pkill -u *** -f "^python"列出“***”用户的进程信息 ps aux --sort-%mem | grep ^***git add ./*git commit -m "注释"git push …...
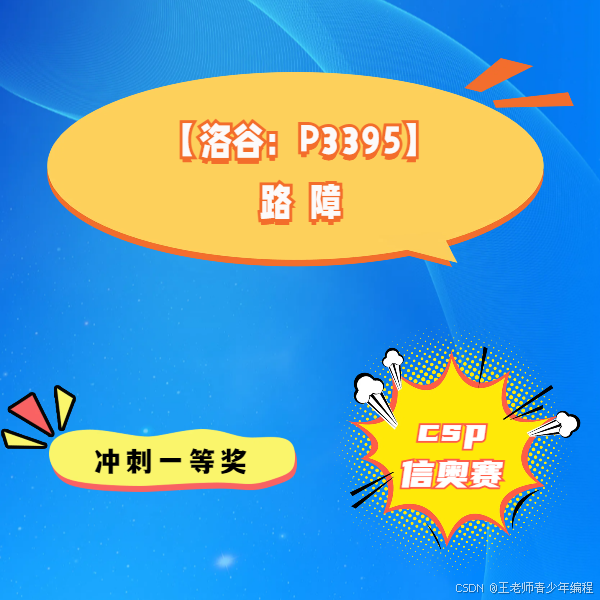
信奥赛CSP-J复赛集训(bfs专题)(5):洛谷P3395:路障
信奥赛CSP-J复赛集训(bfs专题-刷题题单及题解)(5):洛谷P3395:路障 题目描述 B 君站在一个 n n n\times n n...
《红队和蓝队在网络安全中的定义与分工》
网络安全中什么是红队蓝队 在网络安全领域,红队和蓝队是一种对抗性的演练机制,用于测试和提升网络安全防御能力。 红队(Red Team) 定义与目标 红队是扮演攻击者角色的团队。他们的主要任务是模拟真实的网络攻击,利用各…...
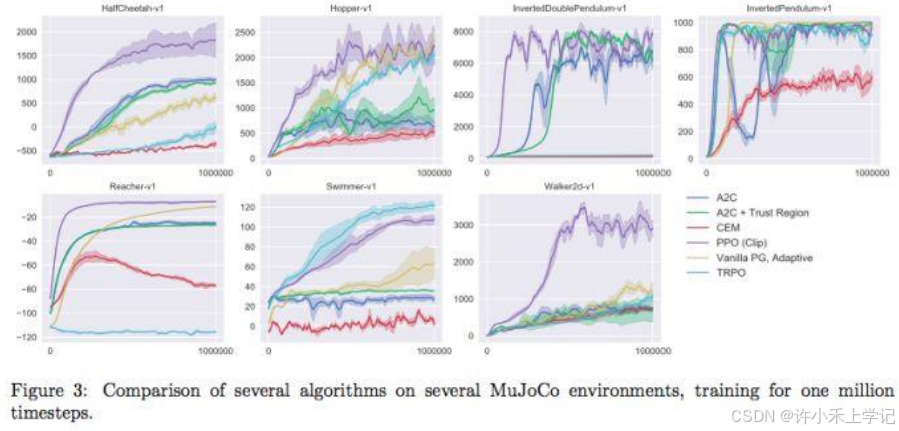
李宏毅深度强化学习入门笔记:PPO
李宏毅-深度强化学习-入门笔记:PPO 一、Policy Gradient(一)基本元素(二)Policy of Actor1. Policy π \pi π 是带有参数 θ \theta θ 的 network2. 例子:运行流程 (三)Actor, E…...
vue2项目中如何把rem设置为固定的100px
在 Vue 2 项目中,可以通过动态设置 html 元素的 font-size 来将 1rem 固定为 100px。以下是具体步骤: 在项目的入口文件 main.js 中添加以下代码,用于动态设置 html 的 font-size: // main.js function setHtmlFontSize() {cons…...
C++多线程常用方法
在 C 中,线程相关功能主要通过头文件提供的类和函数来实现,以下是一些常用的线程接口方法和使用技巧: std::thread类 构造函数: 可以通过传入可调用对象(如函数指针、函数对象、lambda 表达式等)来创建一…...

ubuntu+ros新手笔记(三):21讲没讲到的MoveIt2
1 安装MoveIt2 安装参照在ROS2中,通过MoveIt2控制Gazebo中的自定义机械手 安装 MoveIt2可以选择自己编译源码安装,或者直接从二进制安装。 个人建议直接二进制安装,可以省很多事。 sudo apt install ros-humble-moveitmoveit-setup-assistan…...
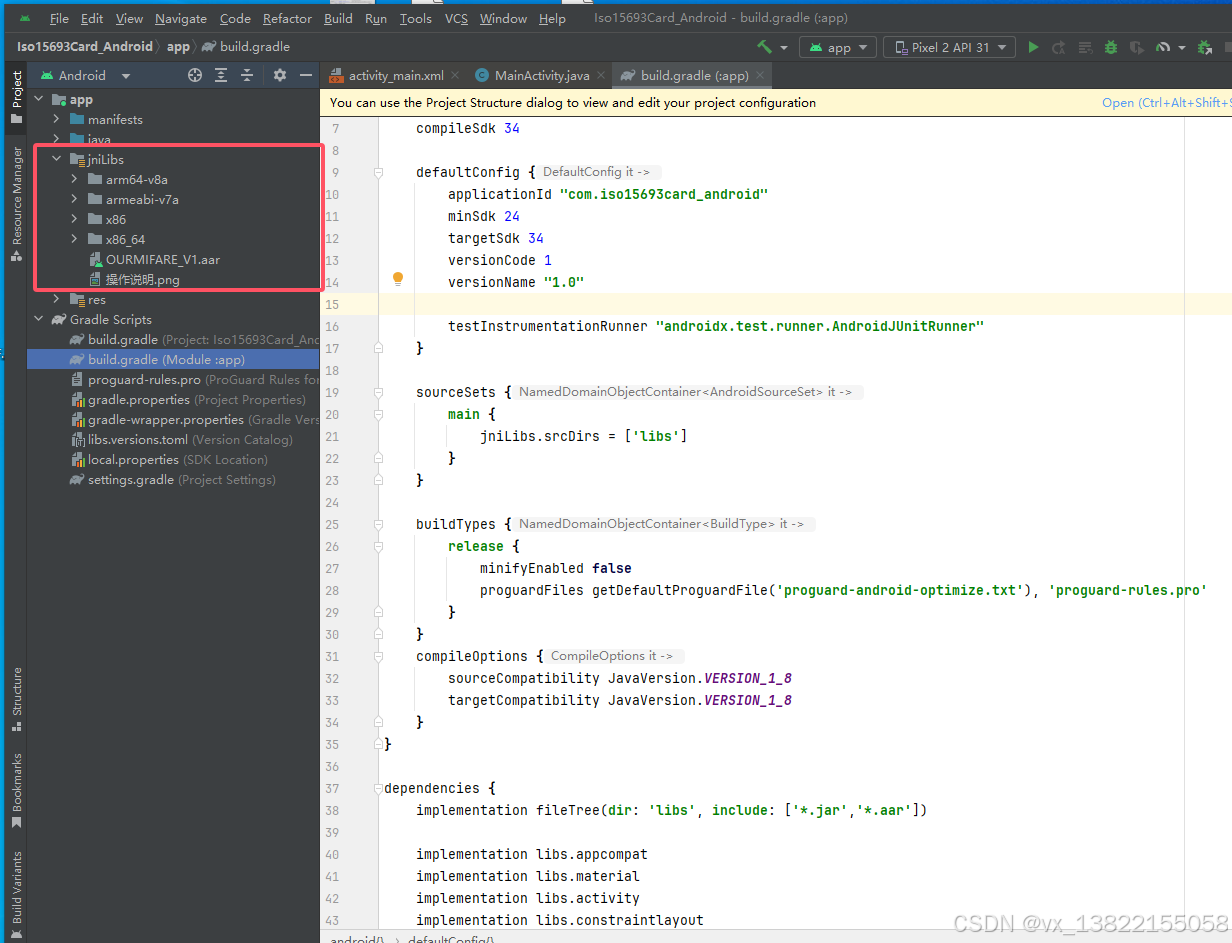
Android Studio创建新项目并引入第三方so外部aar库驱动NFC读写器读写IC卡
本示例使用设备:https://item.taobao.com/item.htm?spma21dvs.23580594.0.0.52de2c1bbW3AUC&ftt&id615391857885 一、打开Android Studio,点击 File> New>New project 菜单,选择 要创建的项目模版,点击 Next 二、输入项目名称…...
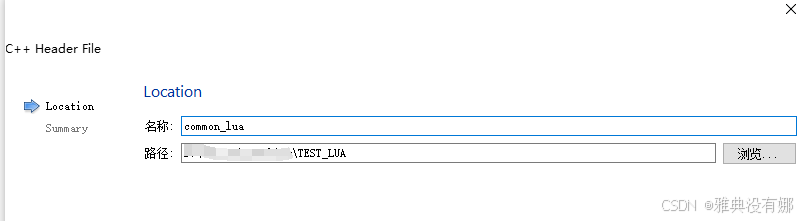
window QT/C++ 与 lua交互(mingw + lua + LuaBridge + luasocket)
一、环境与准备工作 测试环境:win10 编译器:mingw QT版本:QT5.12.3 下载三种源码: LuaBridge源码:https://github.com/vinniefalco/LuaBridge LUA源码(本测试用的是5.3.5):https://www.lua.org/download.html luasocket源码:https://github.com/diegonehab/luasocket 目…...
中阳科技:量化模型驱动的智能交易革命
在金融市场飞速发展的今天,量化交易作为科技与金融的深度融合,正推动市场格局向智能化转型。中阳科技凭借先进的数据分析技术与算法研发能力,探索量化模型的升级与优化,为投资者提供高效、智能的交易解决方案。 量化交易的本质与价…...
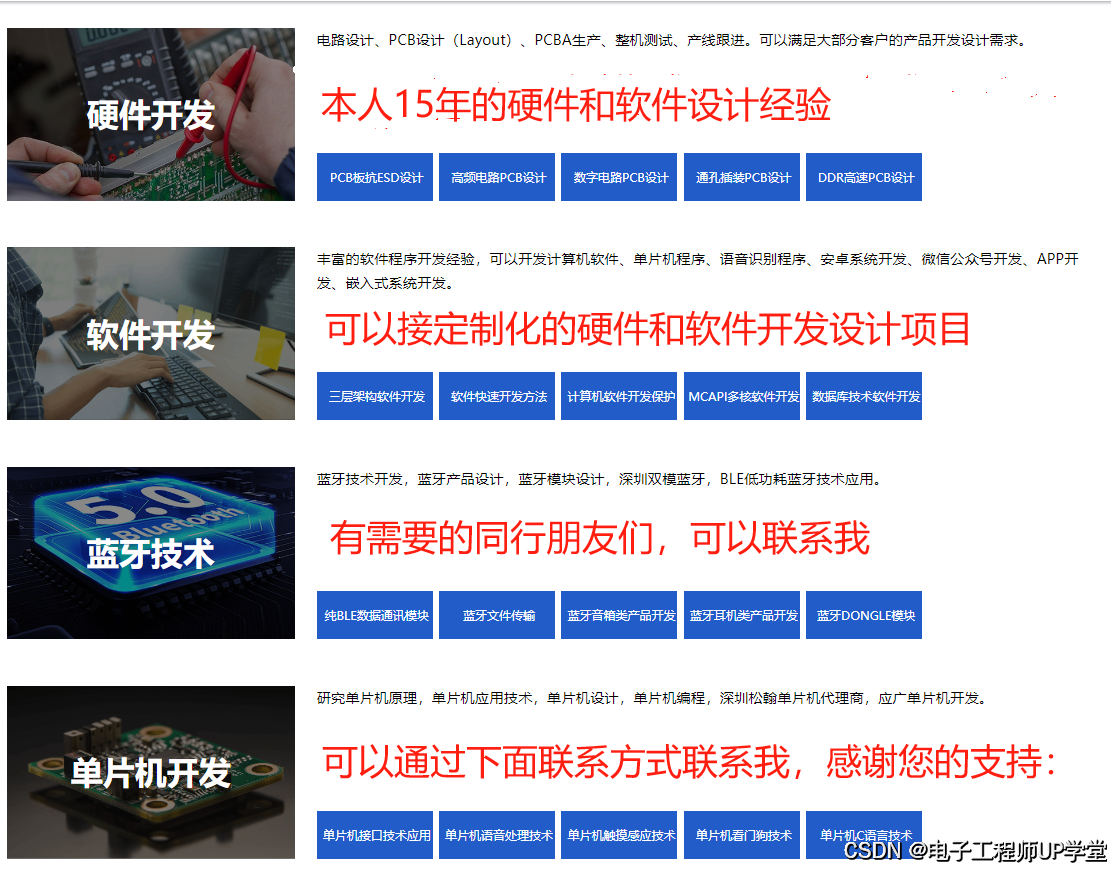
电子应用设计方案-56:智能书柜系统方案设计
智能书柜系统方案设计 一、引言 随着数字化时代的发展和人们对知识获取的需求增加,智能书柜作为一种创新的图书管理和存储解决方案,能够提供更高效、便捷和个性化的服务。本方案旨在设计一款功能齐全、智能化程度高的智能书柜系统。 二、系统概述 1. 系…...
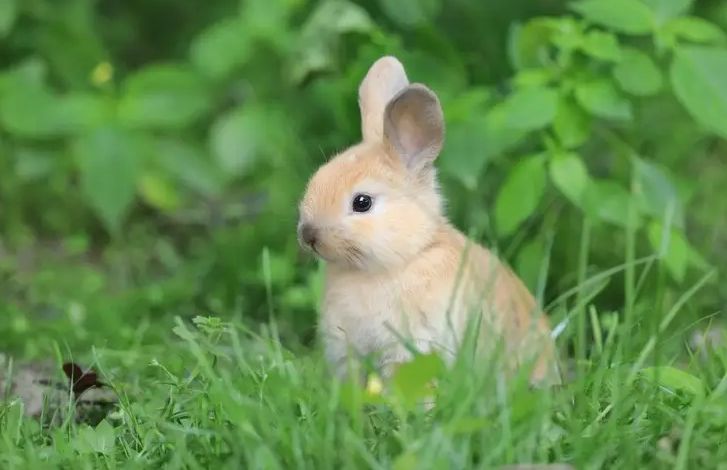
宠物兔需要洗澡吗?
在宠物兔的养护领域,“宠物兔需要洗澡吗” 这个问题一直备受争议。其实,这不能简单地一概而论,而要综合多方面因素考量。 兔子本身是爱干净的动物,它们日常会通过自我舔舐来打理毛发。从这个角度讲,如果兔子生活环境较…...
ubuntu升级python版本
Ubuntu升级Python版本 解压缩文件: 下载完成后,解压缩文件: tar -xf Python-3.12.0.tgz编译并安装: 进入解压后的目录,然后配置和安装Python: codecd Python-3.12.0 ./configure --enable-optimizations ma…...
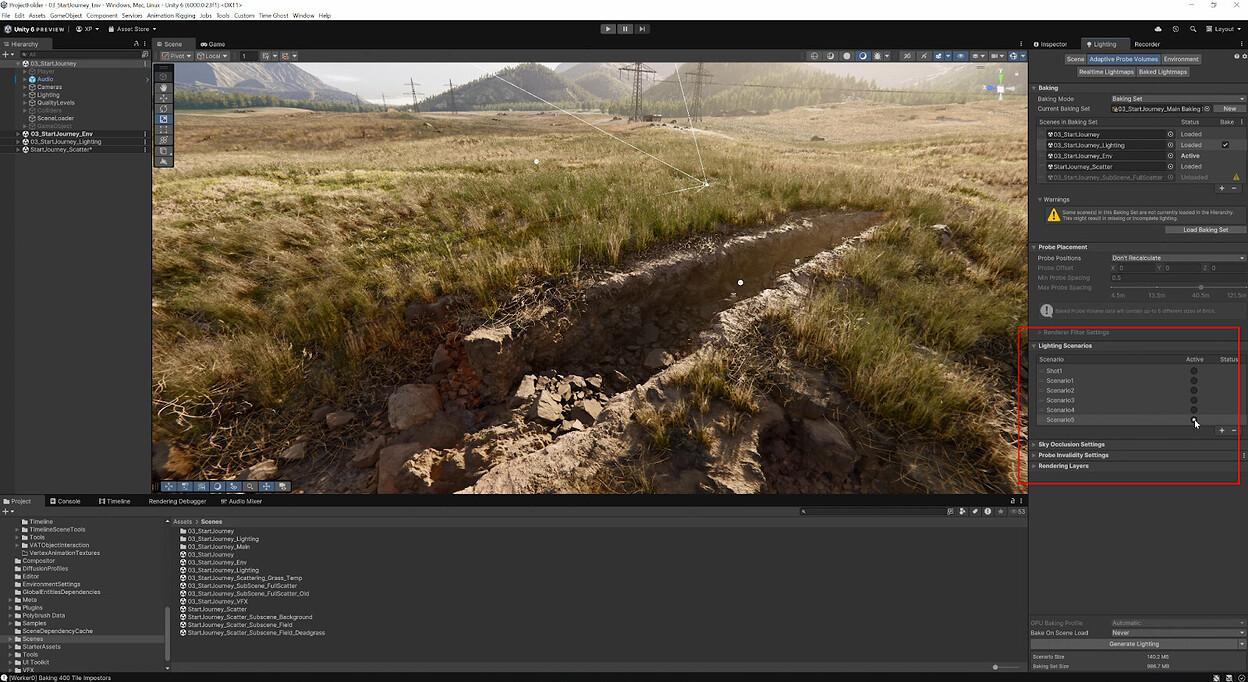
《Time Ghost》的制作:使用 DOTS ECS 制作更为复杂的大型环境
*基于 Unity 6 引擎制作的 demo 《Time Ghost》 开始《Time Ghost》项目时的目标之一是提升在 Unity 中构建大型户外环境的构建标准。为了实现这一目标,我们要有处理更为复杂的场景的能力、有足够的工具支持,同时它对引擎的核心图形、光照、后处理、渲染…...
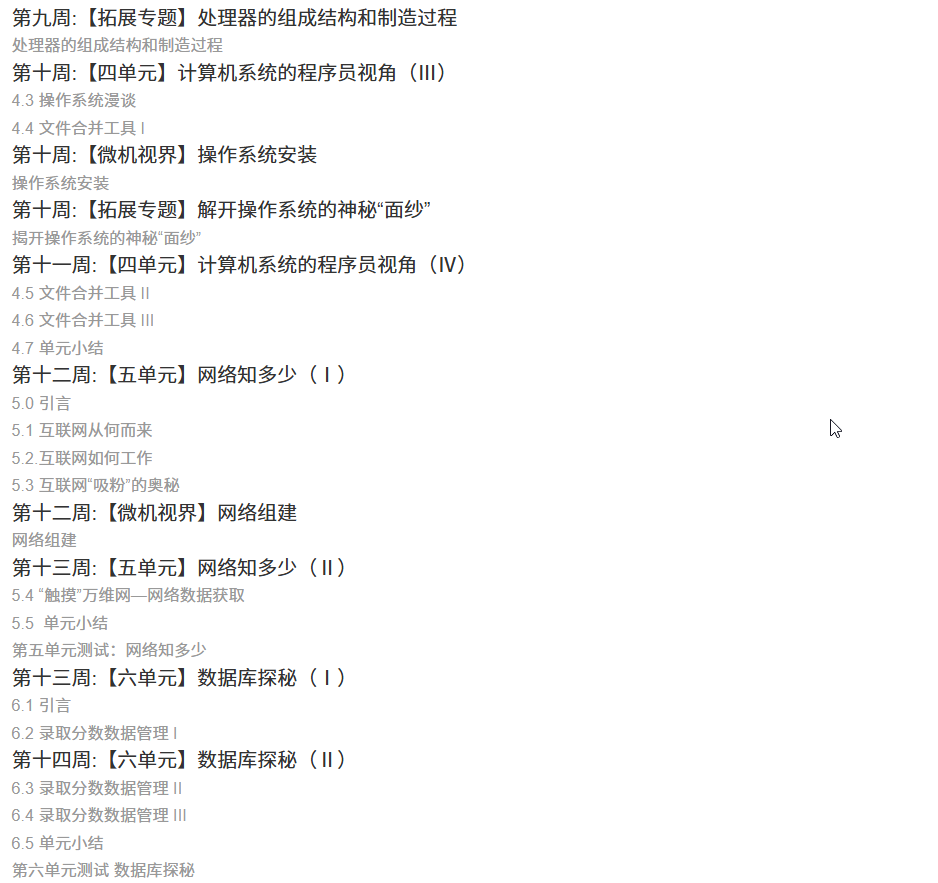
国防科技大学计算机基础课程笔记02信息编码
1.机内码和国标码 国标码就是我们非常熟悉的这个GB2312,但是因为都是16进制,因此这个了16进制的数据既可以翻译成为这个机器码,也可以翻译成为这个国标码,所以这个时候很容易会出现这个歧义的情况; 因此,我们的这个国…...
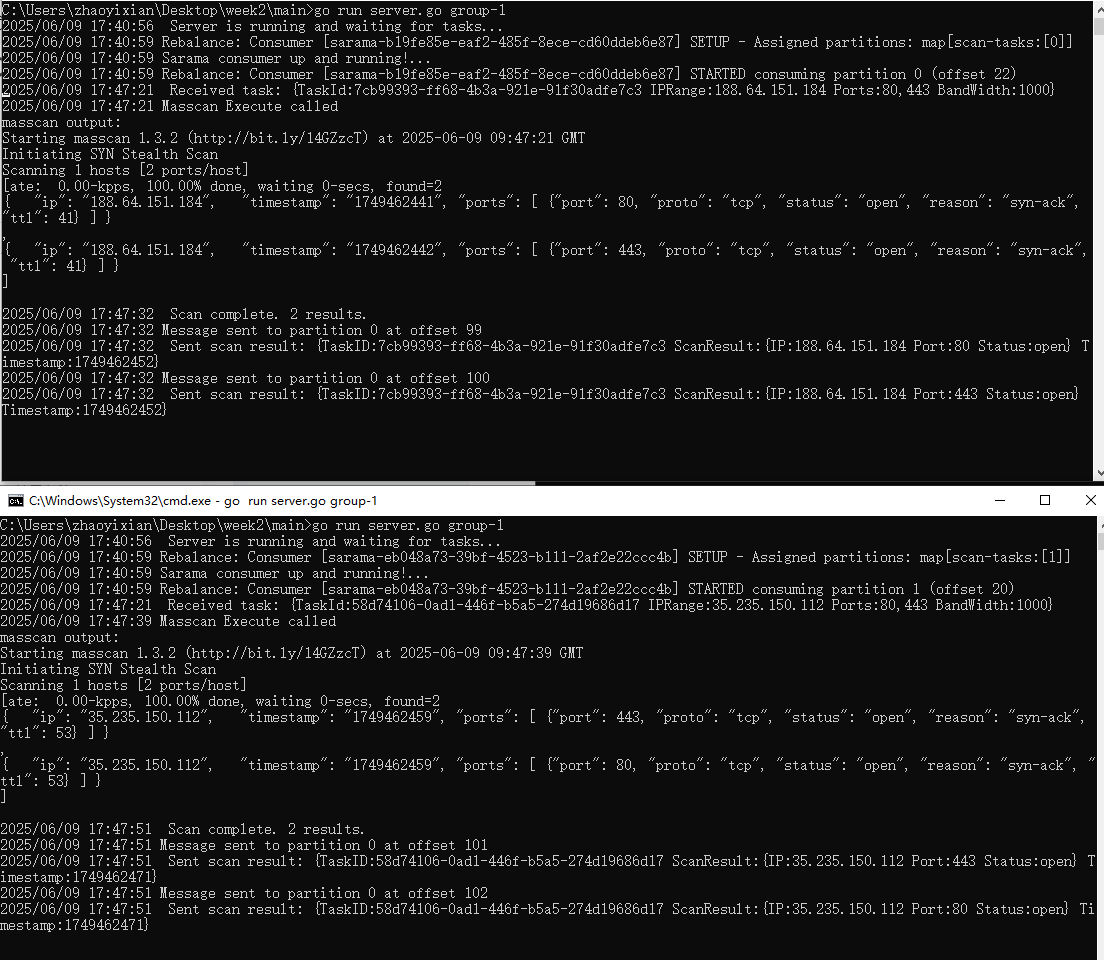
【kafka】Golang实现分布式Masscan任务调度系统
要求: 输出两个程序,一个命令行程序(命令行参数用flag)和一个服务端程序。 命令行程序支持通过命令行参数配置下发IP或IP段、端口、扫描带宽,然后将消息推送到kafka里面。 服务端程序: 从kafka消费者接收…...
ubuntu搭建nfs服务centos挂载访问
在Ubuntu上设置NFS服务器 在Ubuntu上,你可以使用apt包管理器来安装NFS服务器。打开终端并运行: sudo apt update sudo apt install nfs-kernel-server创建共享目录 创建一个目录用于共享,例如/shared: sudo mkdir /shared sud…...
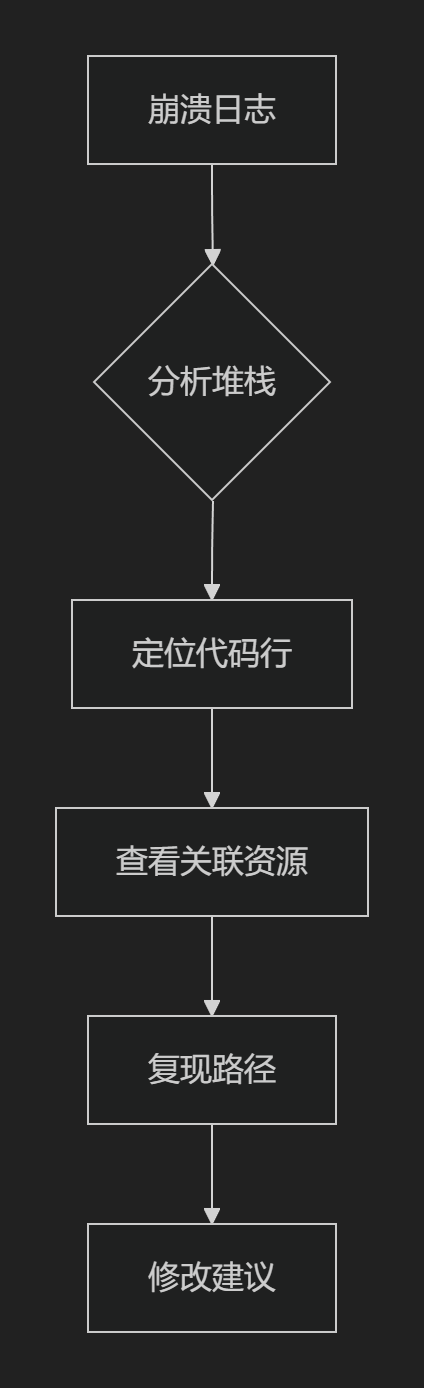
【HarmonyOS 5.0】DevEco Testing:鸿蒙应用质量保障的终极武器
——全方位测试解决方案与代码实战 一、工具定位与核心能力 DevEco Testing是HarmonyOS官方推出的一体化测试平台,覆盖应用全生命周期测试需求,主要提供五大核心能力: 测试类型检测目标关键指标功能体验基…...
1688商品列表API与其他数据源的对接思路
将1688商品列表API与其他数据源对接时,需结合业务场景设计数据流转链路,重点关注数据格式兼容性、接口调用频率控制及数据一致性维护。以下是具体对接思路及关键技术点: 一、核心对接场景与目标 商品数据同步 场景:将1688商品信息…...
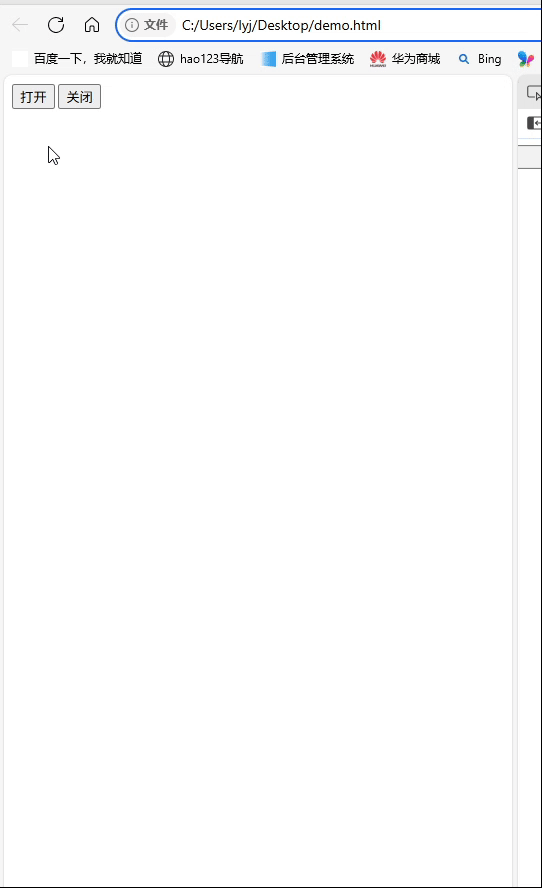
深入理解JavaScript设计模式之单例模式
目录 什么是单例模式为什么需要单例模式常见应用场景包括 单例模式实现透明单例模式实现不透明单例模式用代理实现单例模式javaScript中的单例模式使用命名空间使用闭包封装私有变量 惰性单例通用的惰性单例 结语 什么是单例模式 单例模式(Singleton Pattern&#…...
【论文笔记】若干矿井粉尘检测算法概述
总的来说,传统机器学习、传统机器学习与深度学习的结合、LSTM等算法所需要的数据集来源于矿井传感器测量的粉尘浓度,通过建立回归模型来预测未来矿井的粉尘浓度。传统机器学习算法性能易受数据中极端值的影响。YOLO等计算机视觉算法所需要的数据集来源于…...
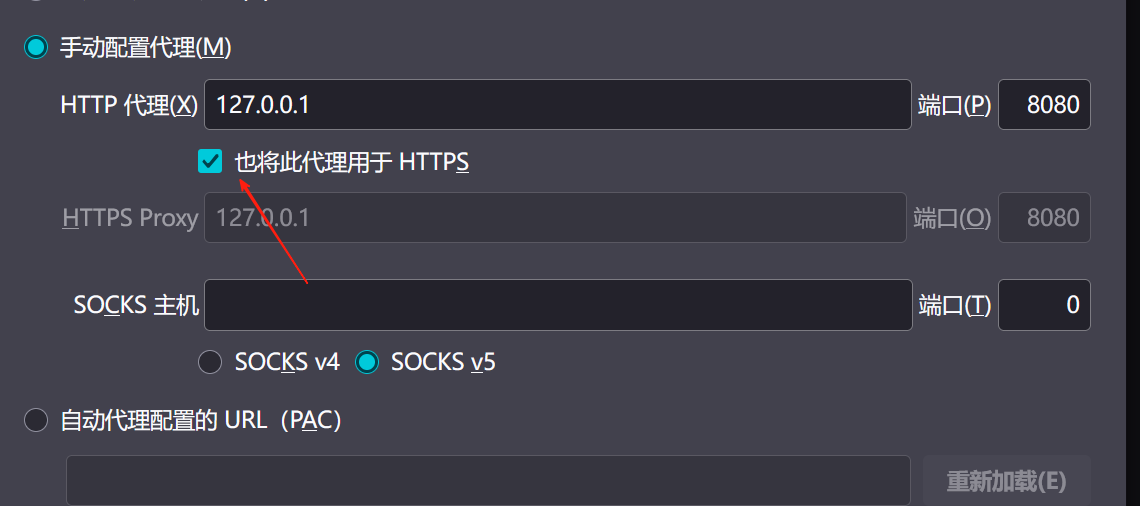
【2025年】解决Burpsuite抓不到https包的问题
环境:windows11 burpsuite:2025.5 在抓取https网站时,burpsuite抓取不到https数据包,只显示: 解决该问题只需如下三个步骤: 1、浏览器中访问 http://burp 2、下载 CA certificate 证书 3、在设置--隐私与安全--…...

涂鸦T5AI手搓语音、emoji、otto机器人从入门到实战
“🤖手搓TuyaAI语音指令 😍秒变表情包大师,让萌系Otto机器人🔥玩出智能新花样!开整!” 🤖 Otto机器人 → 直接点明主体 手搓TuyaAI语音 → 强调 自主编程/自定义 语音控制(TuyaAI…...
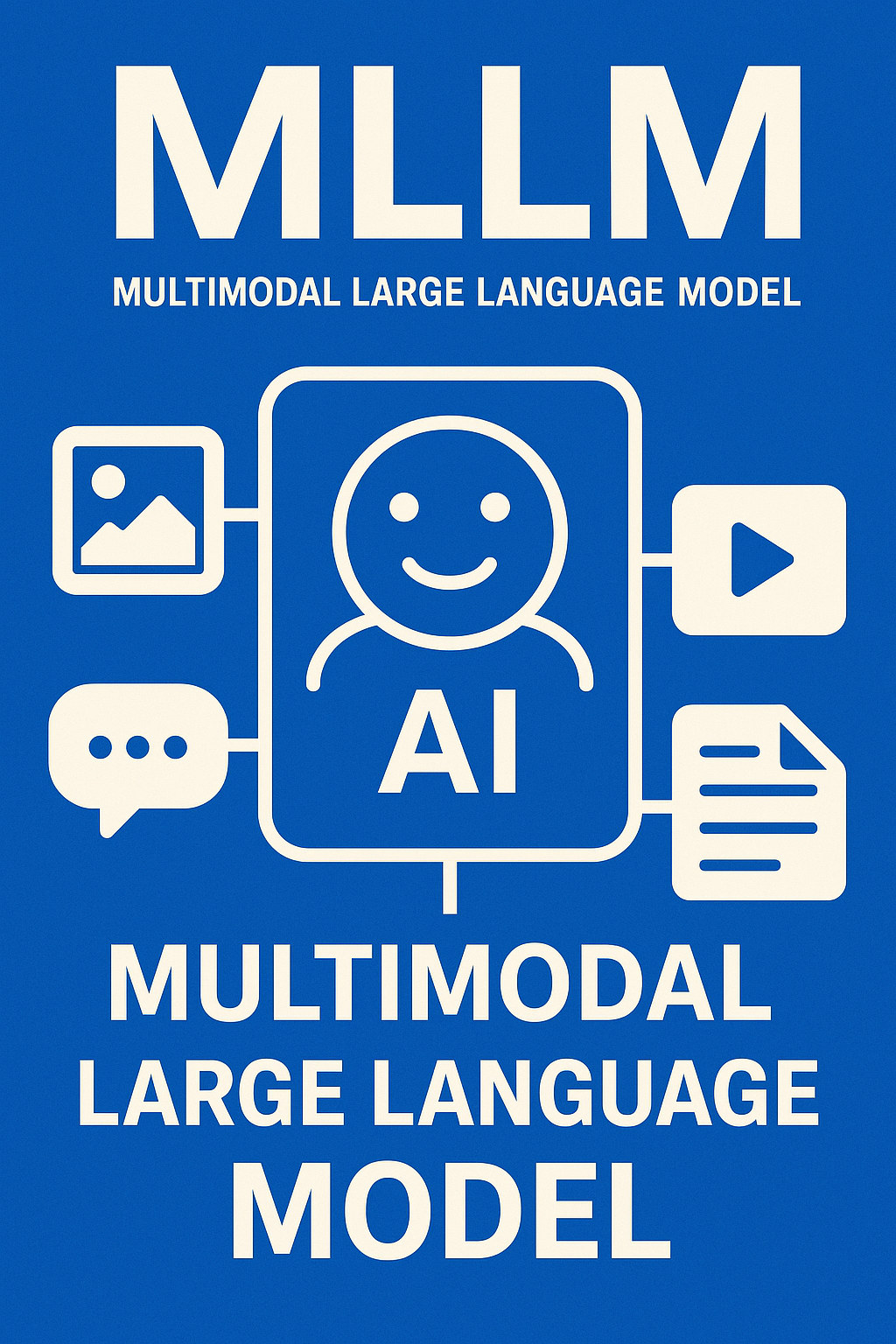
多模态大语言模型arxiv论文略读(108)
CROME: Cross-Modal Adapters for Efficient Multimodal LLM ➡️ 论文标题:CROME: Cross-Modal Adapters for Efficient Multimodal LLM ➡️ 论文作者:Sayna Ebrahimi, Sercan O. Arik, Tejas Nama, Tomas Pfister ➡️ 研究机构: Google Cloud AI Re…...