x光下危险物品/违禁物品目标识别的模型训练与推理代码
前言
1.安检在公共场合的重要性不言而喻,保障群众人身安全是其首要任务。在各种场合,安检都是不可或缺的环节。x光安检机作为安检的重要工具,尽管其具有人工监控判断成像的特性,但是其局限性也十分明显。
为了解决这一局限性为出发点,让人工智能介入,这里在Torch框架下使用YOLO算法实现了对x光图像中刀具、气罐、鞭炮等10类危险物品的目标检测功能。
2.源码地址:https://download.csdn.net/download/matt45m/88178088
数据集
1.数据集定义
每个场景的安检违禁品都是一样的,但从法律法规的角度来度,违禁品可以分10大类,60多种小类,具体如下:
- 枪支类:运动枪、机枪、防暴枪、电击枪、发令枪、麻醉注射枪、气枪、步枪、冲锋枪、射钉枪、消防灭火枪、猎枪、玩具枪、仿真枪、催泪枪、道具枪、钢珠枪、手枪
- 五金工具类:大扳手、铁锤子、铲子、警棍、斧子、臂力器、甩棍
- 宠物类:猫咪、仓鼠、水獭、蛇、刺猬、龙猫、兔子、狗狗(导盲犬除外)、乌龟、蜥蜴
- 消毒用品类:医用酒精、84消毒液、酒精免洗凝胶、酒精喷雾、过氧乙酸消毒液、双氧水消毒液、酒精消毒液
- 毒品、腐蚀物:盐酸、硒粉、农药、水银、氰化物、砒霜、苯酚、硫酸、老鼠药、硝酸、氢氧化钾、杀虫药、氢氧化钠
- 燃料、气体:煤油、乙烷、乙烯、天然气、甲烷、柴油、丙烯、乙炔、液化石油气、丁烷、汽油、氢气、一氧化碳
- 易燃物:红磷、固体酒精、白磷、赛璐珞、镁铝粉、钾、锂、钠、油漆、稀料、油纸、乙醚、黄磷、松香油、碳化钙(电石)、丙酮、苯、闪光粉
- 食品类:未密封的榴莲、自嗨锅、活鱼、活虾、自热米饭、活螃蟹、臭豆腐、自热火锅
- 爆炸物品类:燃烧弹、毒气弹、引火线、导火索、雷管、催泪弹、炸药、炸弹、照明弹、手雷、信号弹、各种烟花爆竹、手榴弹、烟幕弹、烟火药。
- 刀具用品类:美工刀、屠宰刀、尖头剪刀、陶瓷刀、水果刀、餐刀、瑞士军刀、菜刀。
2.数据集收集
X光的数据有两种形式,一种彩色的成像,一种是黑白成像,相对于彩色成像,黑白成像对于人类视觉,更难于分出违禁品。
3.数据标注
数据集使用了网上开源的数据和私人收集的部分数据集,数据集涵盖了基本上所有的违禁品,但由于人手与算力了的问题,只挑了4000张彩色成像的x光图像,标注了10个我国常见的违禁品,分别是有:‘lighter’,‘scissors’,‘powerbank’,‘pressure’,‘knife’,‘zippooil’,‘handcuffs’,‘slingshot’,‘firecrackers’,‘nailpolish’。数据标注LabelImg,标注格式是YOLO。
模型训练
训练和开发环境是win10,显卡RTX3080;cuda10.2,cudnn7.1;OpenCV4.5;yolov5用的是5s的模型,Anaconda 3.5。
1.创建环境
conda create --name yolov5 python=3.8activate yolov5git clone https://github.com/ultralytics/yolov5.gitcd yolov5pip install -r requirements.txt
或者
conda create --name yolov5 python=3.8
activate yolov5
git clone https://github.com/ultralytics/yolov5.git
cd yolov5
conda install pytorch torchvision cudatoolkit=10.2 -c pytorch
pip install cython matplotlib tqdm opencv-python tensorboard scipy pillow onnx pyyaml pandas seaborn
2.训练
打开model/yolov5s.yaml,更改nc数目。
# parameters
nc: 10 # 检测总类别
depth_multiple: 0.33 # model depth multiple 网络的深度系数
width_multiple: 0.50 # layer channel multiple 卷积核的系数# anchors 候选框,可以改成自己目标的尺寸,也可以增加候选框
anchors:- [10,13, 16,30, 33,23] # P3/8- [30,61, 62,45, 59,119] # P4/16- [116,90, 156,198, 373,326] # P5/32# YOLOv5 backbone
backbone: #特征提取模块# [from, number, module, args]# from - 输入是什么,-1:上一层的输出结果;# number - 该层的重复的次数,要乘以系数,小于1则等于1 源码( n = max(round(n * gd), 1) if n > 1 else n)# module - 层的名字# args - 卷积核的个数[[-1, 1, Focus, [64, 3]], # 0-P1/2 # 64要乘以卷积核的个数 64*0.5 = 32个特征图[-1, 1, Conv, [128, 3, 2]], # 1-P2/4[-1, 3, BottleneckCSP, [128]],[-1, 1, Conv, [256, 3, 2]], # 3-P3/8[-1, 9, BottleneckCSP, [256]],[-1, 1, Conv, [512, 3, 2]], # 5-P4/16[-1, 9, BottleneckCSP, [512]],[-1, 1, Conv, [1024, 3, 2]], # 7-P5/32[-1, 1, SPP, [1024, [5, 9, 13]]],[-1, 3, BottleneckCSP, [1024, False]], # 9]# YOLOv5 head
head:[[-1, 1, Conv, [512, 1, 1]],[-1, 1, nn.Upsample, [None, 2, 'nearest']],[[-1, 6], 1, Concat, [1]], # cat backbone P4[-1, 3, BottleneckCSP, [512, False]], # 13[-1, 1, Conv, [256, 1, 1]],[-1, 1, nn.Upsample, [None, 2, 'nearest']],[[-1, 4], 1, Concat, [1]], # cat backbone P3[-1, 3, BottleneckCSP, [256, False]], # 17 (P3/8-small)[-1, 1, Conv, [256, 3, 2]],[[-1, 14], 1, Concat, [1]], # cat head P4[-1, 3, BottleneckCSP, [512, False]], # 20 (P4/16-medium)[-1, 1, Conv, [512, 3, 2]],[[-1, 10], 1, Concat, [1]], # cat head P5[-1, 3, BottleneckCSP, [1024, False]], # 23 (P5/32-large)[[17, 20, 23], 1, Detect, [nc, anchors]], # Detect(P3, P4, P5) [17,20,23] #17层、20层、23层;]
在data目录下添加一个dangerous.yaml训练数据配置文件,文件内容如下:
# download command/URL (optional)
download: bash data/scripts/get_voc.sh# 训练集txt与验证集txt路径
train: data/xxx/train.txt
val: data/xxx/val.txt# 总类别数
nc: 10# 类名
names: ['lighter','scissors','powerbank','pressure','knife','zippooil','handcuffs','slingshot','firecrackers','nailpolish']
开始训练
训练命令
单卡:
python train.py --cfg models/yolov5s.yaml --data data/ODID.yaml --hyp data/hyps/hyp.scratch.yaml --epochs 100 --multi-scale --device 0
多卡:
python train.py --cfg models/yolov5s.yaml --data data/ODID.yaml --hyp data/hyps/hyp.scratch.yaml --epochs 100 --multi-scale --device 0,1
测试模型
python test.py --weights runs/train/exp/weights/best.pt --data data/ODID.yaml --device 0 --verbose
--weights: 训练得到的模型
--data:数据配置文件.txt
--device:选择gpu进行评测
--verbose:是否打印每一类的评测指标
模型推理
对于简单的项目需求,可以使用Gradio来快速部署。但是,当项目的复杂性增加,界面布局变得更复杂时,使用Gradio来管理和设置各个控件的位置会变得非常困难。在这种情况下,就可以考虑使用Qt。PyQt是一个Python绑定的Qt框架,它利用Qt的布局和设计工具,将拖放和设置好的界面转换为Python代码,从而简化了项目开发过程。
py qt5安装
pip install PyQt5
pip install PyQt5-tools
安装好PyQt5之后就可以开始编写推理代码了,pyqt能快速创建一个一推理的UI界面,项目分三种输入,图像、摄像头、视频。
#! /usr/bin/env python
# -*- coding: utf-8 -*-
"""
Run a YOLO_v3 style detection model on test images.
"""
import numpy as np
import argparseimport cv2
import torch
import torch.backends.cudnn as cudnn
from numpy import randomfrom models.experimental import attempt_load
from utils.general import check_img_size, non_max_suppression, scale_coords
from utils.plots import plot_one_box
from utils.torch_utils import select_device
import sys
from PyQt5 import QtCore, QtGui, QtWidgets
from PyQt5.QtWidgets import *
flag = Falsedef letterbox(img, new_shape=(640, 640), color=(114, 114, 114), auto=True, scaleFill=False, scaleup=True):# Resize image to a 32-pixel-multiple rectangle https://github.com/ultralytics/yolov3/issues/232shape = img.shape[:2] # current shape [height, width]shape = img.shape[:2] # current shape [height, width]if isinstance(new_shape, int):new_shape = (new_shape, new_shape)# Scale ratio (new / old)r = min(new_shape[0] / shape[0], new_shape[1] / shape[1])if not scaleup: # only scale down, do not scale up (for better test mAP)r = min(r, 1.0)# Compute paddingratio = r, r # width, height ratiosnew_unpad = int(round(shape[1] * r)), int(round(shape[0] * r))dw, dh = new_shape[1] - new_unpad[0], new_shape[0] - new_unpad[1] # wh paddingif auto: # minimum rectangledw, dh = np.mod(dw, 32), np.mod(dh, 32) # wh paddingelif scaleFill: # stretchdw, dh = 0.0, 0.0new_unpad = (new_shape[1], new_shape[0])ratio = new_shape[1] / shape[1], new_shape[0] / shape[0] # width, height ratiosdw /= 2 # divide padding into 2 sidesdh /= 2if shape[::-1] != new_unpad: # resizeimg = cv2.resize(img, new_unpad, interpolation=cv2.INTER_LINEAR)top, bottom = int(round(dh - 0.1)), int(round(dh + 0.1))left, right = int(round(dw - 0.1)), int(round(dw + 0.1))img = cv2.copyMakeBorder(img, top, bottom, left, right, cv2.BORDER_CONSTANT, value=color) # add borderreturn img, ratio, (dw, dh)
# with torch.no_grad():
# detect()
class Ui_MainWindow(QtWidgets.QWidget):def __init__(self, parent=None):super(Ui_MainWindow, self).__init__(parent)self.timer_camera = QtCore.QTimer()self.timer_camera_capture = QtCore.QTimer()self.cap = cv2.VideoCapture()self.CAM_NUM = 0self.set_ui()self.slot_init()# self.detect_image(self.image)self.__flag_work = 0self.x = 0parser = argparse.ArgumentParser()parser.add_argument('--weights', nargs='+', type=str, default='weights/dangerous-best.pt', help='model.pt path(s)')parser.add_argument('--source', type=str, default='images', help='source') # file/folder, 0 for webcamparser.add_argument('--img-size', type=int, default=640, help='inference size (pixels)')parser.add_argument('--conf-thres', type=float, default=0.5, help='object confidence threshold')parser.add_argument('--iou-thres', type=float, default=0.45, help='IOU threshold for NMS')parser.add_argument('--device', default='', help='cuda device, i.e. 0 or 0,1,2,3 or cpu')parser.add_argument('--view-img', action='store_true', help='display results')parser.add_argument('--save-txt', action='store_true', help='save results to *.txt')parser.add_argument('--save-conf', action='store_true', help='save confidences in --save-txt labels')parser.add_argument('--save-dir', type=str, default='results', help='directory to save results')parser.add_argument('--classes', nargs='+', type=int, help='filter by class: --class 0, or --class 0 2 3')parser.add_argument('--agnostic-nms', action='store_true', help='class-agnostic NMS')parser.add_argument('--augment', action='store_true', help='augmented inference')parser.add_argument('--update', action='store_true', help='update all models')self.opt = parser.parse_args()print(self.opt)ut, source, weights, view_img, save_txt, imgsz = \self.opt.save_dir, self.opt.source, self.opt.weights, self.opt.view_img, self.opt.save_txt, self.opt.img_sizewebcam = source.isnumeric() or source.startswith(('rtsp://', 'rtmp://', 'http://')) or source.endswith('.txt')self.device = select_device(self.opt.device)# if os.path.exists(out): # output dir# shutil.rmtree(out) # delete dir# os.makedirs(out) # make new dirself.half = self.device.type != 'cpu' # half precision only supported on CUDA# Load modelself.model = attempt_load(weights,device=self.device) # load FP32 modelself.imgsz = check_img_size(imgsz, s=self.model.stride.max()) # check img_sizeif self.half:self.model.half() # to FP16cudnn.benchmark = True # set True to speed up constant image size inference# Get names and colorsself.names = self.model.module.names if hasattr(self.model, 'module') else self.model.namesself.colors = [[random.randint(0, 255) for _ in range(3)] for _ in range(len(self.names))]def set_ui(self):self.__layout_main = QtWidgets.QHBoxLayout()self.__layout_fun_button = QtWidgets.QVBoxLayout()self.__layout_data_show = QtWidgets.QVBoxLayout()self.openimage = QtWidgets.QPushButton(u'图片')self.opencameras = QtWidgets.QPushButton(u'摄像头')self.train = QtWidgets.QPushButton(u'视频')# self.Openvideo = QtWidgets.QPushButton(u'打开视频')self.openimage.setMinimumHeight(50)self.opencameras.setMinimumHeight(50)self.train.setMinimumHeight(50)# self.Openvideo.setMinimumHeight(50)# self.lineEdit = QtWidgets.QLineEdit(self) # 创建 QLineEdit# self.lineEdit.textChanged.connect(self.text_changed)# self.lineEdit.setMinimumHeight(50)self.openimage.move(10, 30)self.opencameras.move(10, 50)self.train.move(15,70)# 信息显示self.showimage = QtWidgets.QLabel()# self.label_move = QtWidgets.QLabel()# self.lineEdit.setFixedSize(70, 30)self.showimage.setFixedSize(641, 481)self.showimage.setAutoFillBackground(False)self.__layout_fun_button.addWidget(self.openimage)self.__layout_fun_button.addWidget(self.opencameras)self.__layout_fun_button.addWidget(self.train)# self.__layout_fun_button.addWidget(self.Openvideo)self.__layout_main.addLayout(self.__layout_fun_button)self.__layout_main.addWidget(self.showimage)self.setLayout(self.__layout_main)# self.label_move.raise_()self.setWindowTitle(u'X光下目标识别0.1版本')def slot_init(self):self.openimage.clicked.connect(self.button_open_image_click)self.opencameras.clicked.connect(self.button_opencameras_click)self.timer_camera.timeout.connect(self.show_camera)# self.timer_camera_capture.timeout.connect(self.capture_camera)self.train.clicked.connect(self.button_train_click)# self.Openvideo.clicked.connect(self.Openvideo_click)def button_open_image_click(self):imgName, imgType = QFileDialog.getOpenFileName(self, "打开图片", "", "*.jpg;;*.png;;All Files(*)")img = cv2.imread(imgName)print(imgName)showimg = imgwith torch.no_grad():img = letterbox(img, new_shape=self.opt.img_size)[0]# Convertimg = img[:, :, ::-1].transpose(2, 0, 1) # BGR to RGB, to 3x416x416img = np.ascontiguousarray(img)img = torch.from_numpy(img).to(self.device)img = img.half() if self.half else img.float() # uint8 to fp16/32img /= 255.0 # 0 - 255 to 0.0 - 1.0if img.ndimension() == 3:img = img.unsqueeze(0)# Inferencepred = self.model(img, augment=self.opt.augment)[0]# Apply NMSpred = non_max_suppression(pred, self.opt.conf_thres, self.opt.iou_thres, classes=self.opt.classes, agnostic=self.opt.agnostic_nms)# Process detectionsfor i, det in enumerate(pred): # detections per imageif det is not None and len(det):# Rescale boxes from img_size to im0 sizedet[:, :4] = scale_coords(img.shape[2:], det[:, :4], showimg.shape).round()# Write resultsfor *xyxy, conf, cls in reversed(det):label = '%s %.2f' % (self.names[int(cls)], conf)plot_one_box(xyxy, showimg, label=label, color=self.colors[int(cls)], line_thickness=3)self.result = cv2.cvtColor(showimg, cv2.COLOR_BGR2BGRA)self.result = cv2.resize(self.result, (640, 480), interpolation=cv2.INTER_AREA)self.QtImg = QtGui.QImage(self.result.data, self.result.shape[1], self.result.shape[0],QtGui.QImage.Format_RGB32)# 显示图片到label中;self.showimage.setPixmap(QtGui.QPixmap.fromImage(self.QtImg))def button_train_click(self):global flagself.timer_camera_capture.stop()self.cap.release()if flag == False:flag = TrueimgName, imgType = QFileDialog.getOpenFileName(self, "打开视频", "", "*.mp4;;*.avi;;All Files(*)")flag = self.cap.open(imgName)if flag == False:msg = QtWidgets.QMessageBox.warning(self, u"Warning", u"打开视频失败",buttons=QtWidgets.QMessageBox.Ok,defaultButton=QtWidgets.QMessageBox.Ok)else:self.timer_camera.start(30)self.train.setText(u'关闭识别')else:flag = Falseself.timer_camera.stop()self.cap.release()self.showimage.clear()self.train.setText(u'打开视频')def button_opencameras_click(self):self.timer_camera_capture.stop()self.cap.release()if self.timer_camera.isActive() == False:flag = self.cap.open(self.CAM_NUM)if flag == False:msg = QtWidgets.QMessageBox.warning(self, u"Warning", u"请检测相机与电脑是否连接正确",buttons=QtWidgets.QMessageBox.Ok,defaultButton=QtWidgets.QMessageBox.Ok)else:self.timer_camera.start(30)self.opencameras.setText(u'关闭识别')else:self.timer_camera.stop()self.cap.release()self.showimage.clear()self.opencameras.setText(u'打开摄像头')def show_camera(self):flag, img = self.cap.read()if img is not None:showimg = imgwith torch.no_grad():img = letterbox(img, new_shape=self.opt.img_size)[0]# Convertimg = img[:, :, ::-1].transpose(2, 0, 1) # BGR to RGB, to 3x416x416img = np.ascontiguousarray(img)img = torch.from_numpy(img).to(self.device)img = img.half() if self.half else img.float() # uint8 to fp16/32img /= 255.0 # 0 - 255 to 0.0 - 1.0if img.ndimension() == 3:img = img.unsqueeze(0)# Inferencepred = self.model(img, augment=self.opt.augment)[0]# Apply NMSpred = non_max_suppression(pred, self.opt.conf_thres, self.opt.iou_thres, classes=self.opt.classes,agnostic=self.opt.agnostic_nms)# Process detectionsfor i, det in enumerate(pred): # detections per imageif det is not None and len(det):# Rescale boxes from img_size to im0 sizedet[:, :4] = scale_coords(img.shape[2:], det[:, :4], showimg.shape).round()# Write resultsfor *xyxy, conf, cls in reversed(det):label = '%s %.2f' % (self.names[int(cls)], conf)print(label)plot_one_box(xyxy, showimg, label=label, color=self.colors[int(cls)], line_thickness=3)show = cv2.resize(showimg, (640, 480))self.result = cv2.cvtColor(show, cv2.COLOR_BGR2RGB)showImage = QtGui.QImage( self.result.data, self.result.shape[1], self.result.shape[0], QtGui.QImage.Format_RGB888)self.showimage.setPixmap(QtGui.QPixmap.fromImage(showImage))else:flag = Falseself.timer_camera.stop()self.cap.release()self.showimage.clear()self.train.setText(u'打开视频')if __name__ == '__main__':app = QtWidgets.QApplication(sys.argv)ui = Ui_MainWindow()ui.show()sys.exit(app.exec_())
然后运行main.py
后记
1.违禁品中有要检测的危险品是小巧的打火机,考虑到其在复杂拥挤环境中的易遮挡性,有时候是很难精确检测到的,我这个用的是S模型,检测效果还可以,如果对精度有更高的要求,除了加大训练数据之外还可以选择更大的模型或者使用YOLOv8。
2.在安检这个场景中,出现漏检要比出现错检所触发的问题更严重,为了优化漏检率,可以适当放大置信度和加入一些相近的样本,还有场景负样本。
3.如果对该项目感兴趣或者在安装的过程中遇到什么错误的的可以加我的企鹅群:487350510,大家一起探讨。
相关文章:
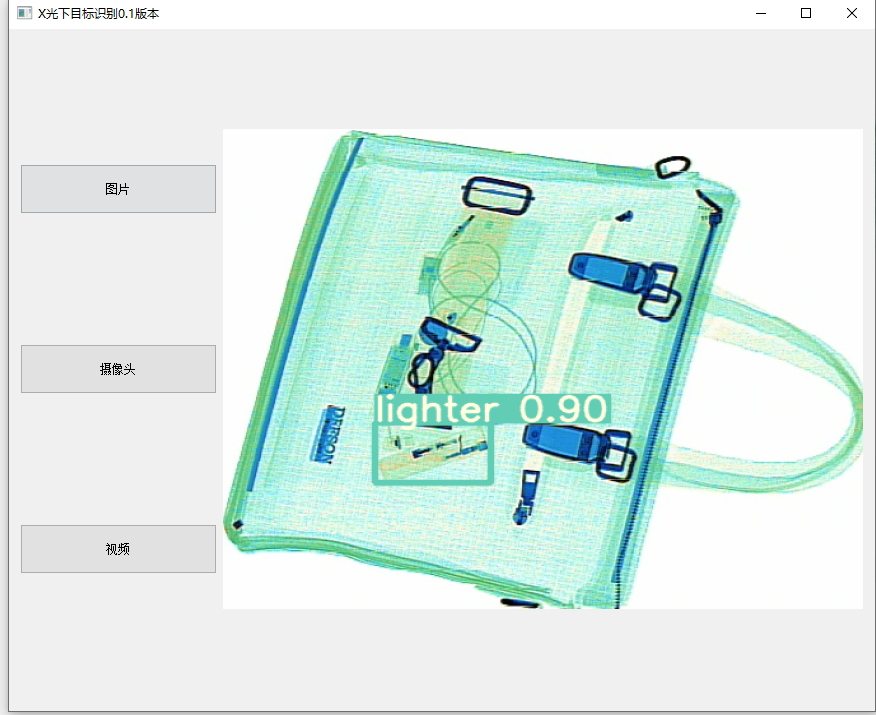
x光下危险物品/违禁物品目标识别的模型训练与推理代码
前言 1.安检在公共场合的重要性不言而喻,保障群众人身安全是其首要任务。在各种场合,安检都是不可或缺的环节。x光安检机作为安检的重要工具,尽管其具有人工监控判断成像的特性,但是其局限性也十分明显。 为了解决这一局限性为出…...

基于Matlab实现图像融合技术(附上多个仿真源码+数据)
图像融合技术是一种将多幅图像融合为一幅图像的方法,使得这幅融合图像包含原始图像的所有信息。近年来,图像融合技术已经广泛应用于图像分割、变换和裁剪等领域。本文将介绍如何使用Matlab实现图像融合技术。 实现步骤 首先,我们需要了解图…...
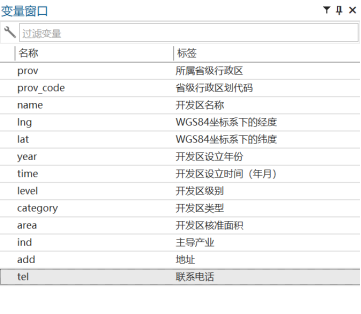
国家级与省级开发区设立超长时间段数据(1970-2022年)
在基于因果推断方法的政策评估备受经济学研究欢迎的今天,将一个重要政策作为外生冲击进行计量建模这一做法,是很多顶刊论文的“宠儿”,大家整理分享的国家级与省级开发区设立超长时间段数据正是其中的代表。 我国各级人民ZF均将开发区设立作为…...
数据结构 10-排序4 统计工龄 桶排序/计数排序(C语言)
给定公司名员工的工龄,要求按工龄增序输出每个工龄段有多少员工。 输入格式: 输入首先给出正整数(≤),即员工总人数;随后给出个整数,即每个员工的工龄,范围在[0, 50]。 输出格式: 按工龄的递…...
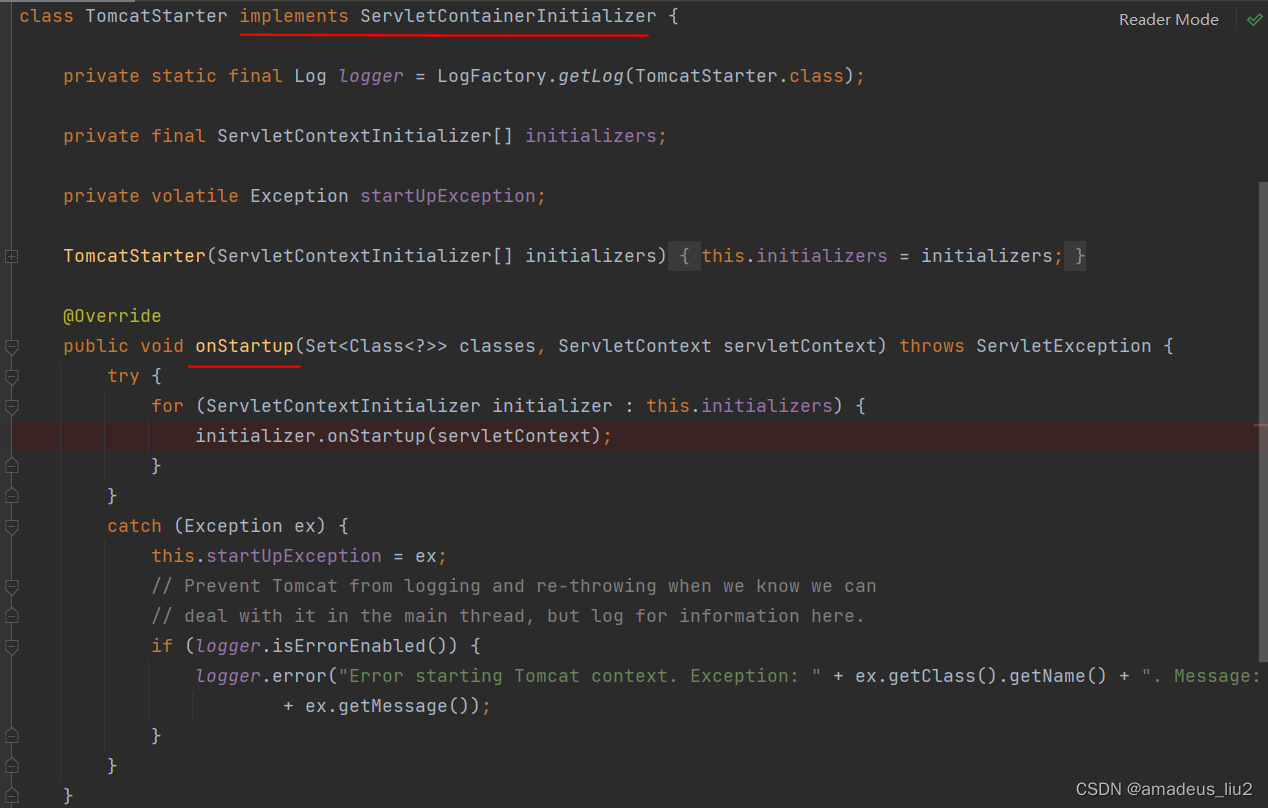
SpringBoot复习:(16)TomcatStarter
直接在idea里运行SpringBoot程序时,内嵌的tomcat容器会调用TomcatStarter这个类的onStartup方法。TomcatStarter继承自ServletContainerInitializer 其onStartup方法会调用ServletContextInitializer(不是ServletContainerInitializer)的onStartup方法.…...
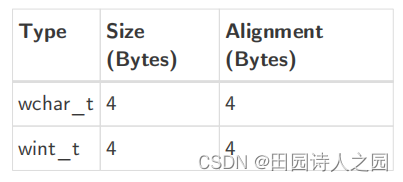
RISCV 5 RISC-V调用规则
RISCV 5 RISC-V调用规则 1 Register Convention1.1 Integer Register Convention1.2 Floating-point Register Convention 2. Procedure Calling Convention2.1 Integer Calling Convention2.2 Hardware Floating-point Calling Convention2.3 ILP32E Calling Convention2.4 Na…...
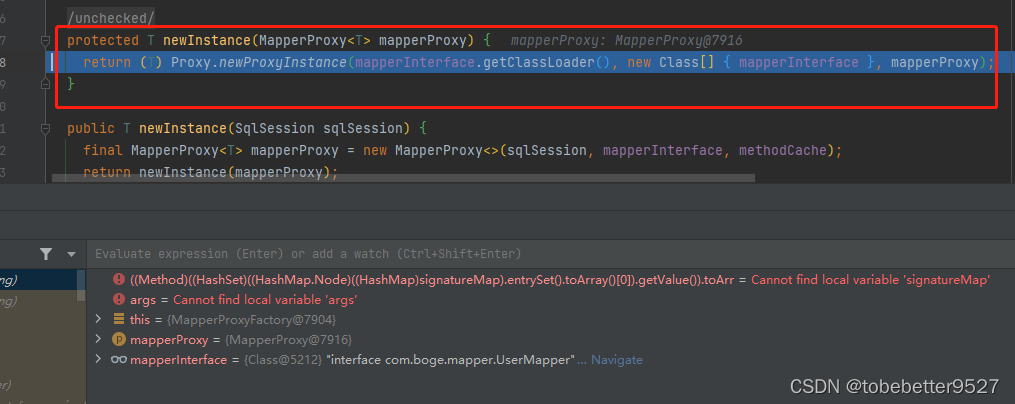
Spring Boot如何整合mybatis
文章目录 1. 相关配置和代码2. 整合原理2.1 springboot自动配置2.2 MybatisAutoConfiguration2.3 debug过程2.3.1 AutoConfiguredMapperScannerRegistrar2.3.2 MapperScannerConfigurer2.3.4 创建MapperFactoryBean2.3.5 创建MybatisAutoConfiguration2.3.6 创建sqlSessionFact…...

TypeScript中 interface 和 type 的区别
区别1 使用 interface 和 type 都是表示给定数据结构的常用方法。定义的方式略有不同。type 定义的时候有 “” 符号 interface User {name: string,age: number } type User {name: string,age: number }区别2 interface 可以多次声明同一接口。它们将合并在一起形成一个接…...

题解 | #B.Distance# 2023牛客暑期多校6
B.Distance 贪心(?) 题目大意 对于两个大小相同的多重集 A , B \mathbb{A},\mathbb{B} A,B ,可以选择其中任一元素 x x x 执行操作 x x 1 xx1 xx1 任意次数,最少的使得 A , B \mathbb{A},\mathbb{B} A,B 相同的操作次数记为 C ( A , B ) C(\m…...

【flink】开启savepoint
先启动一个任务 flink run -c com.yang.flink.CDCJob test-cdc.jar开启savepoint 命令: flink savepoint JobID 文件地址 flink savepoint e929a11d79bdc5e6f140f2cfb92e1335 file:///workspace/flinkSavepoints/backend这样就开启好了 操作中的错误 详细信…...
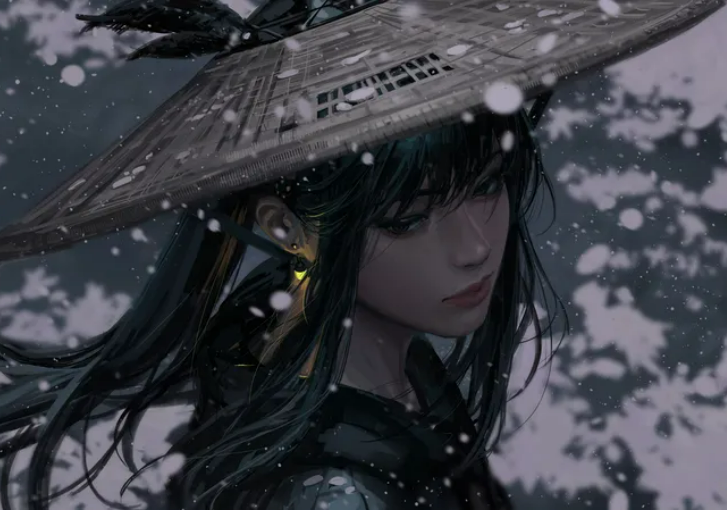
【C++】开源:事件驱动网络库libevent配置使用
😏★,:.☆( ̄▽ ̄)/$:.★ 😏 这篇文章主要介绍事件驱动库libevent配置使用。 无专精则不能成,无涉猎则不能通。——梁启超 欢迎来到我的博客,一起学习,共同进步。 喜欢的朋友可以关注一下…...

业务测试——历史数据
业务测试历史数据的必要性 1.保留上一版本的呈现效果以及数据正确性 2.做发版前后数据、样式一致性校验 3.后端处理历史数据,覆盖各类场景,保证客户的现有数据不会被影响,造成线上事务 4.为测试过程的覆盖度以及产品迭代的质量保驾护航 如何…...
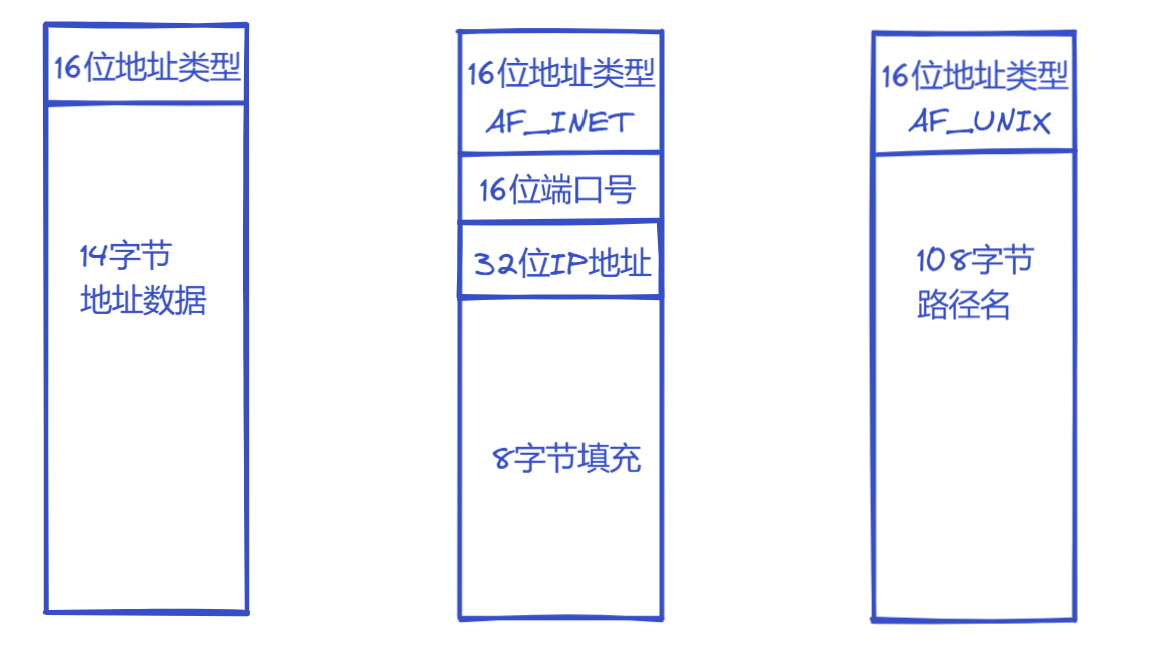
【Linux】计算机网络套接字编写
文章目录 前言TCP协议和UDP协议网络字节序socket接口sockaddr结构1.创建套接字 cs2.绑定端口号 s3.监听socket s4.接受请求 s5.建立连接 c 地址转换函数字符串转in_addrin_addr转字符串 recvfrom和sendto 前言 上篇文章我们学习了计算机网络分层,了解了网络通信的本…...
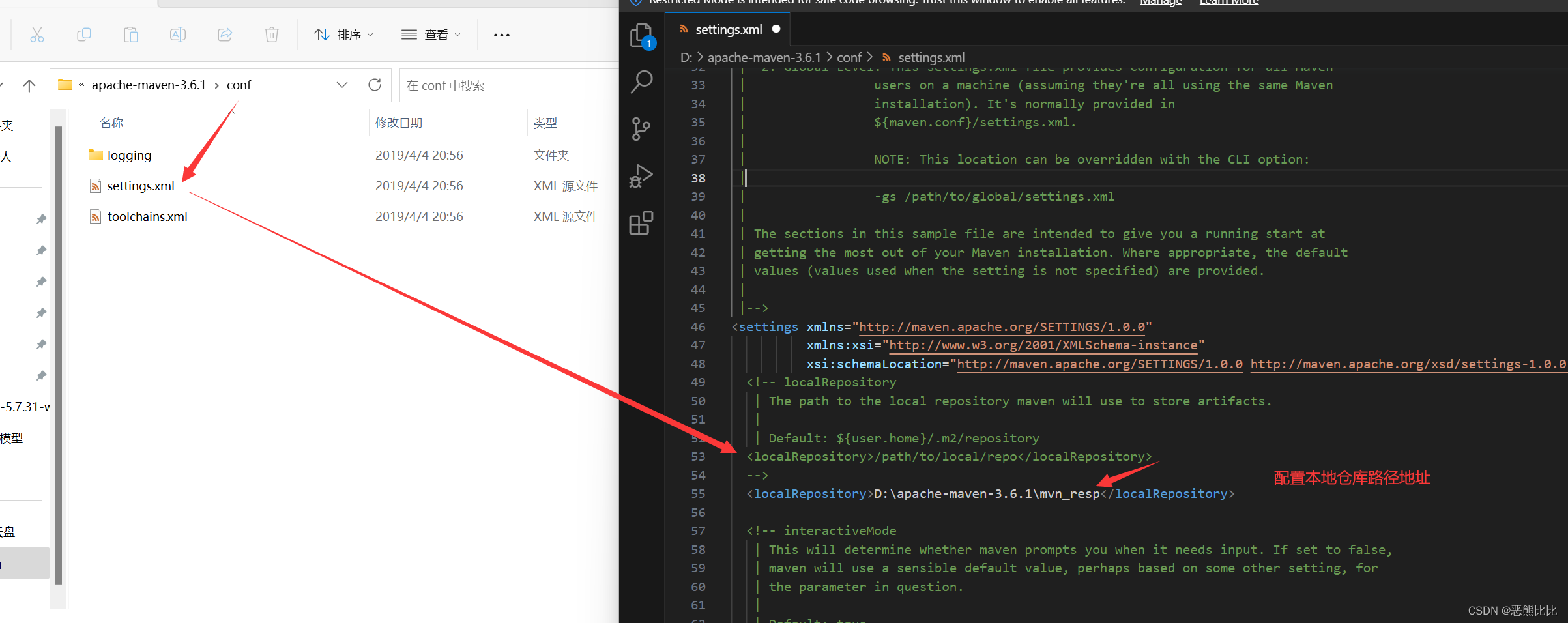
Maven-学习笔记
文章目录 1. Maven简介2.Maven安装和基础配置3.Maven基本使用4.Maven坐标介绍 1. Maven简介 概念 Maven是专门用于管理和构建Java项目的工具 主要功能有: 提供了一套标准化的项目结构提供了一套标准化的构建流程(编译,测试,打包,…...
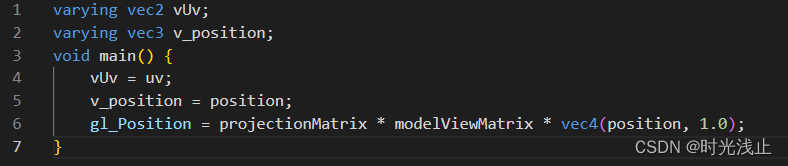
WebGL Shader着色器GLSL语言
在2D绘图中的坐标系统,默认情况下是与窗口坐标系统相同,它以canvas的左上角为坐标原点,沿X轴向右为正值,沿Y轴向下为正值。其中canvas坐标的单位都是’px’。 WebGL使用的是正交右手坐标系,且每个方向都有可使用的值的…...

【Codeforces】 CF468C Hack it!
题目链接 CF方向 Luogu方向 题目解法 令 ∑ i 1 1 e 18 f ( i ) ≡ g ( g < a ) ( m o d a ) \sum_{i1}^{1e18}f(i)\equiv g(g<a)(mod \;a) ∑i11e18f(i)≡g(g<a)(moda) 那么 ∑ i 2 1 e 18 1 f ( i ) ≡ g 1 \sum_{i2}^{1e181}f(i)\equiv g1 ∑i21e181f…...
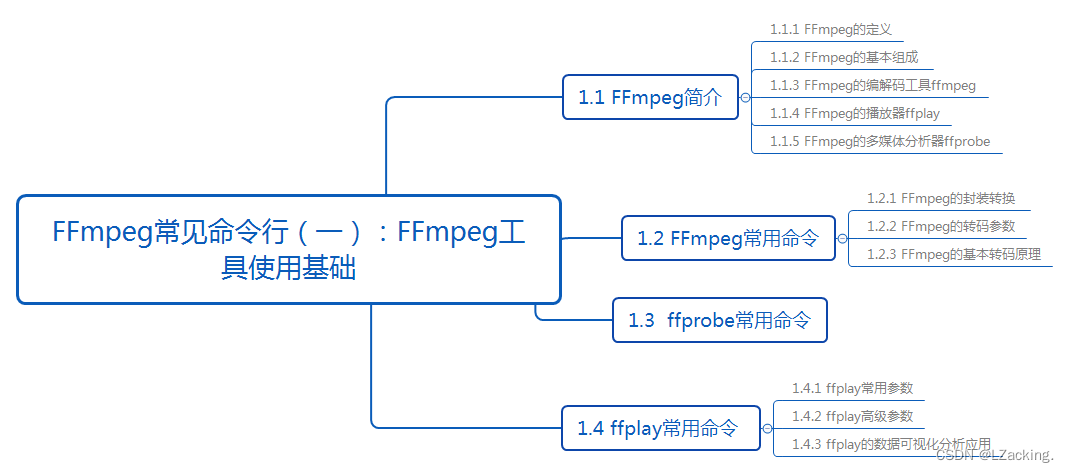
FFmpeg常见命令行(一):FFmpeg工具使用基础
前言 在Android音视频开发中,网上知识点过于零碎,自学起来难度非常大,不过音视频大牛Jhuster提出了《Android 音视频从入门到提高 - 任务列表》。本文是Android音视频任务列表的其中一个, 对应的要学习的内容是:FFmpe…...
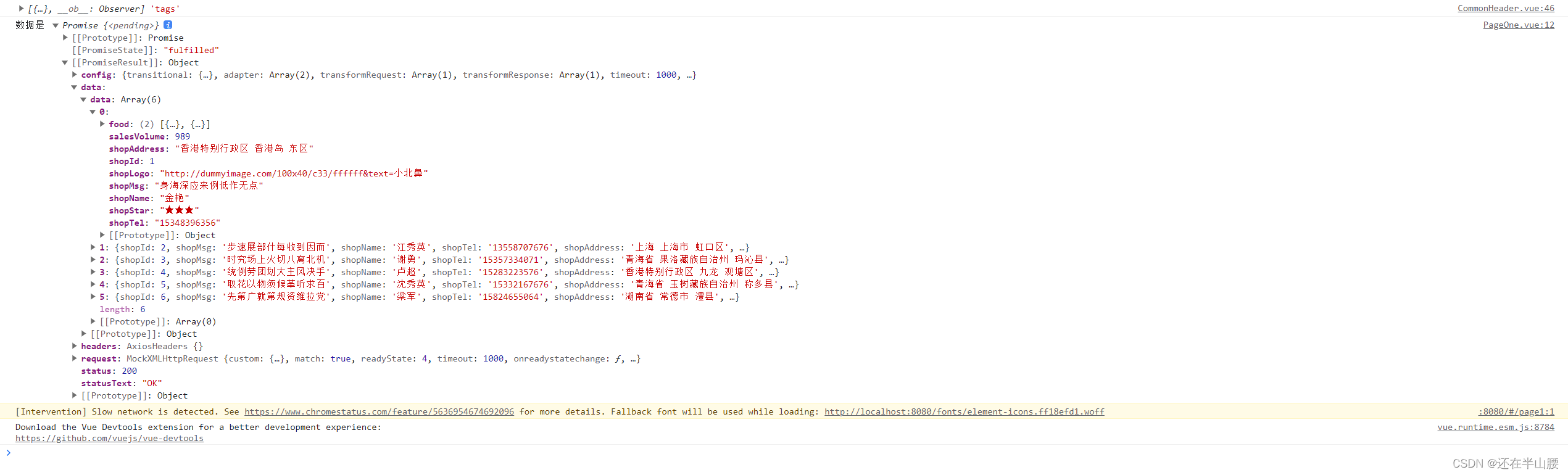
Mock.js的基本使用方法
官网网址:Mock.js (mockjs.com) 当前端工程师需要独立于后端并行开发时,后端接口还没有完成,那么前端怎么获取数据? 这时可以考虑前端搭建web server自己模拟假数据,这里我们选第三方库mockjs用来生成随机数据…...
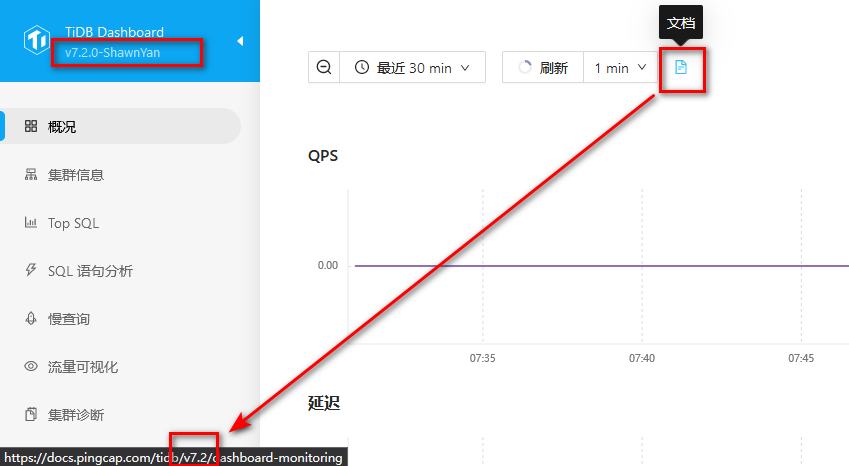
TiDB 源码编译之 PD/TiDB Dashboard 篇
作者: ShawnYan 原文来源: https://tidb.net/blog/a16b1d46 TiDB TiDB 是 PingCAP 公司自主设计、研发的开源分布式关系型数据库,是一款同时支持在线事务处理与在线分析处理 (Hybrid Transactional and Analytical Processing, HTAP) 的融…...
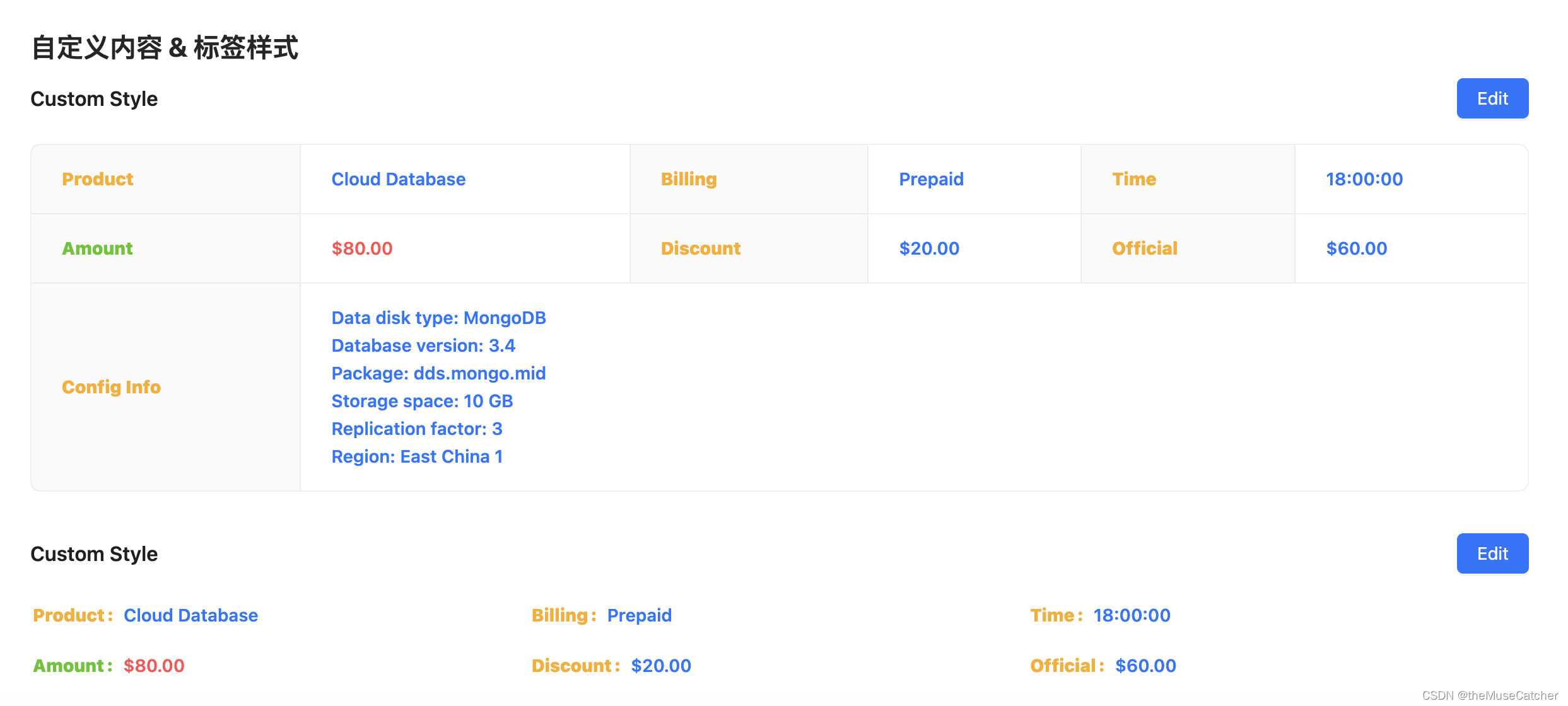
Vue3描述列表(Descriptions)
😁 整体功能效果与 ant design vue 保持高度一致 😁 包含两种组件:Descriptions 和 DescriptionsItem(必须搭配使用!) 效果如下图:在线预览 APIs Descriptions 参数说明类型默认值必传title…...
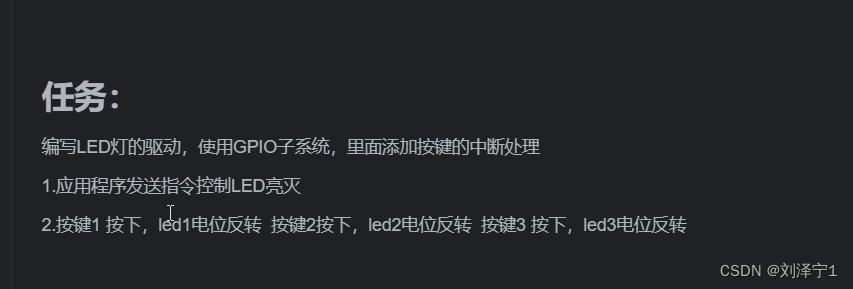
【驱动开发day8作业】
作业1: 应用层代码 #include <stdlib.h> #include <stdio.h> #include <sys/types.h> #include <sys/stat.h> #include <fcntl.h> #include <unistd.h> #include <string.h> #include <sys/ioctl.h>int main(int…...

yxBUG记录
1、 原因:前端参数method方法名写错。 2、Field ‘REC_ID‘ doesn‘t have a default value 问题是id的生成问题。 项目的表不是自增。项目有封装好的方法。调用方法即可。 params.put("rec_id",getSequence("表名")) 3、sql语句有问题 检…...
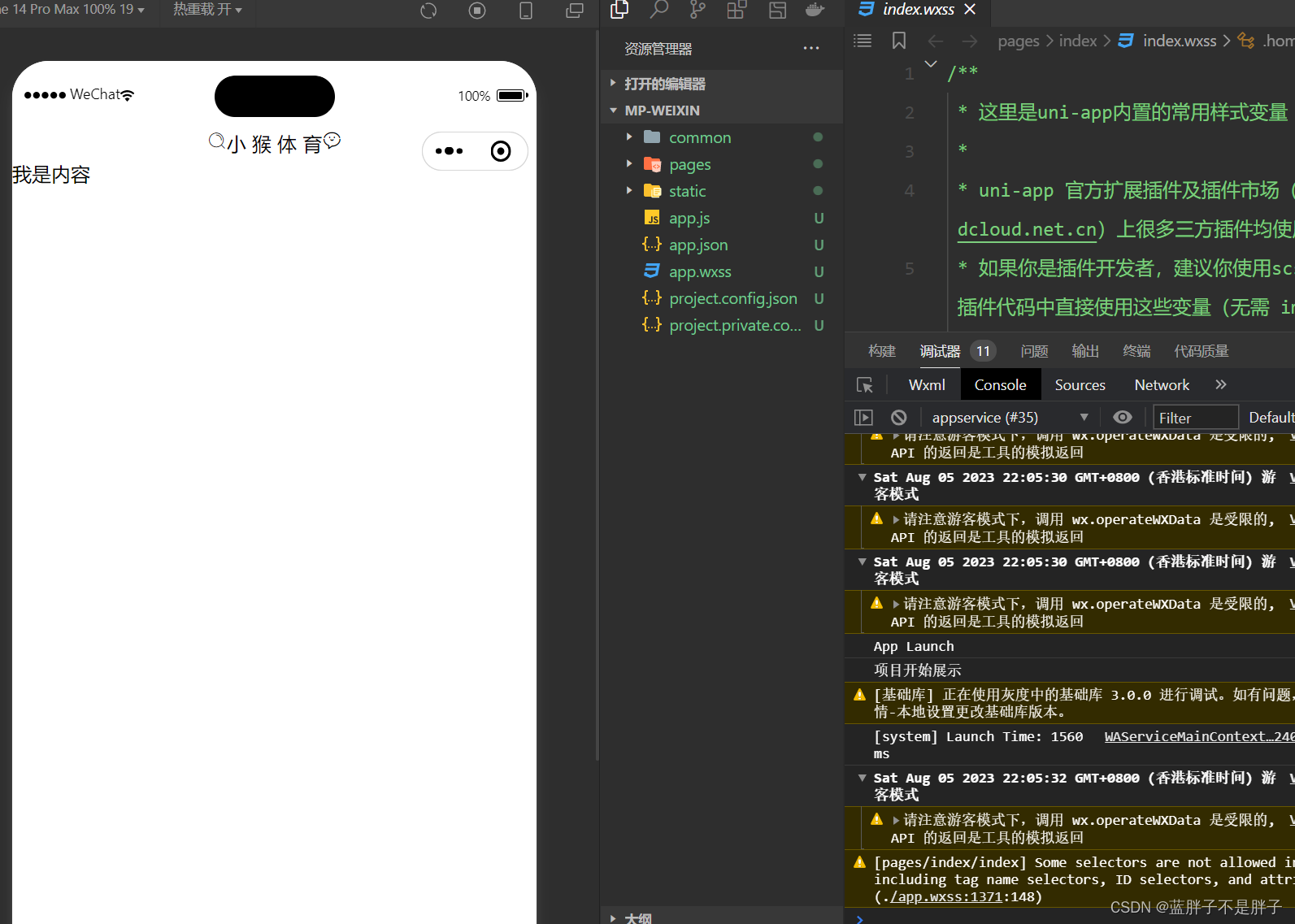
uniapp引入inconfont自定义导航栏
app,h5端引入 uniapp本身的全局设置中有个iconfontsrc属性 所以只需要 1.iconfont将需要的icon添加至项目 2.下载到本地解压后,将其中的ttf文件,放在static静态目录下 3.在page.json中对全局文件进行配置tabBar(导航图标) “iconfontSrc”: “static/font/iconfont.ttf”, …...
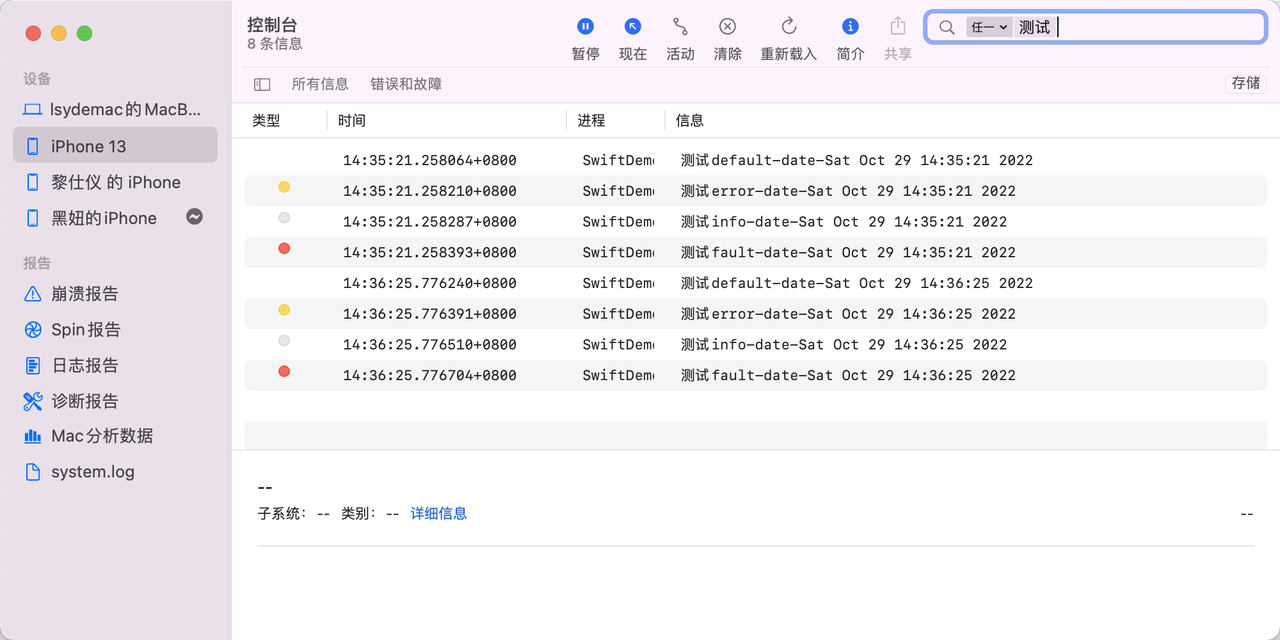
OSLog与NSLog对比
NSLog: NSLog的文档,第一句话就说:Logs an error message to the Apple System Log facility.,所以首先,NSLog就不是设计作为普通的debug log的,而是error log;其次,NSLog也并非是printf的简单…...
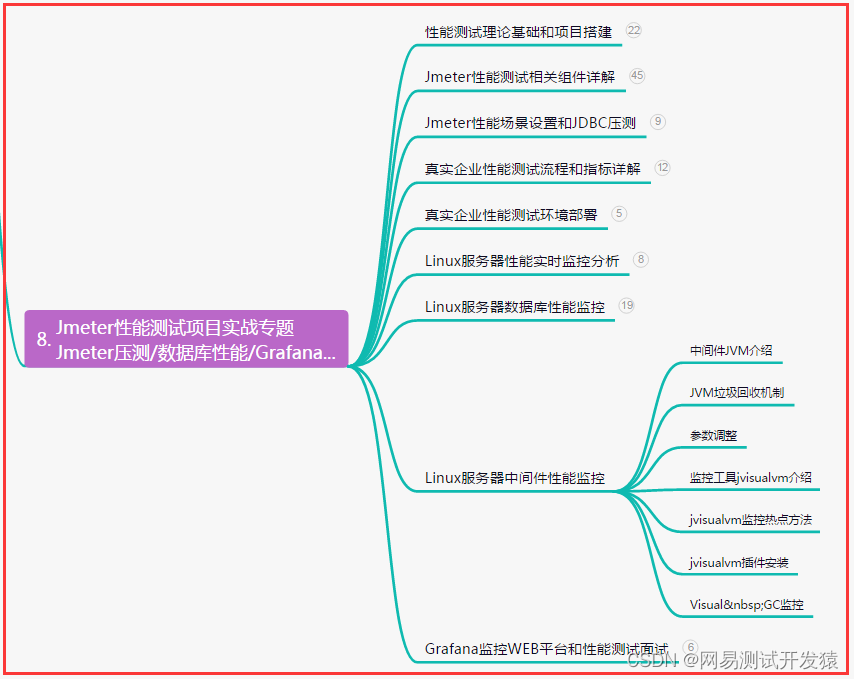
全网最细,Fiddler修改接口返回数据详细步骤实战,辅助接口测试...
目录:导读 前言一、Python编程入门到精通二、接口自动化项目实战三、Web自动化项目实战四、App自动化项目实战五、一线大厂简历六、测试开发DevOps体系七、常用自动化测试工具八、JMeter性能测试九、总结(尾部小惊喜) 前言 在测试的过程中&a…...
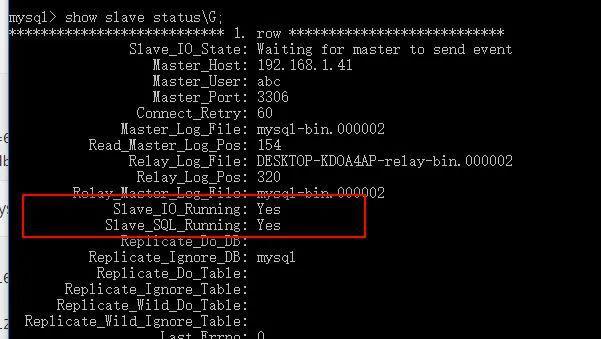
Mysql自动同步的详细设置步骤
以下步骤是真实的测试过程,将其记录下来,与大家共同学习。 一、环境说明: 1、主数据库: (1)操作系统:安装在虚拟机中的CentOS Linux release 7.4.1708 (Core) [rootlocalhost ~]# cat /etc/redh…...
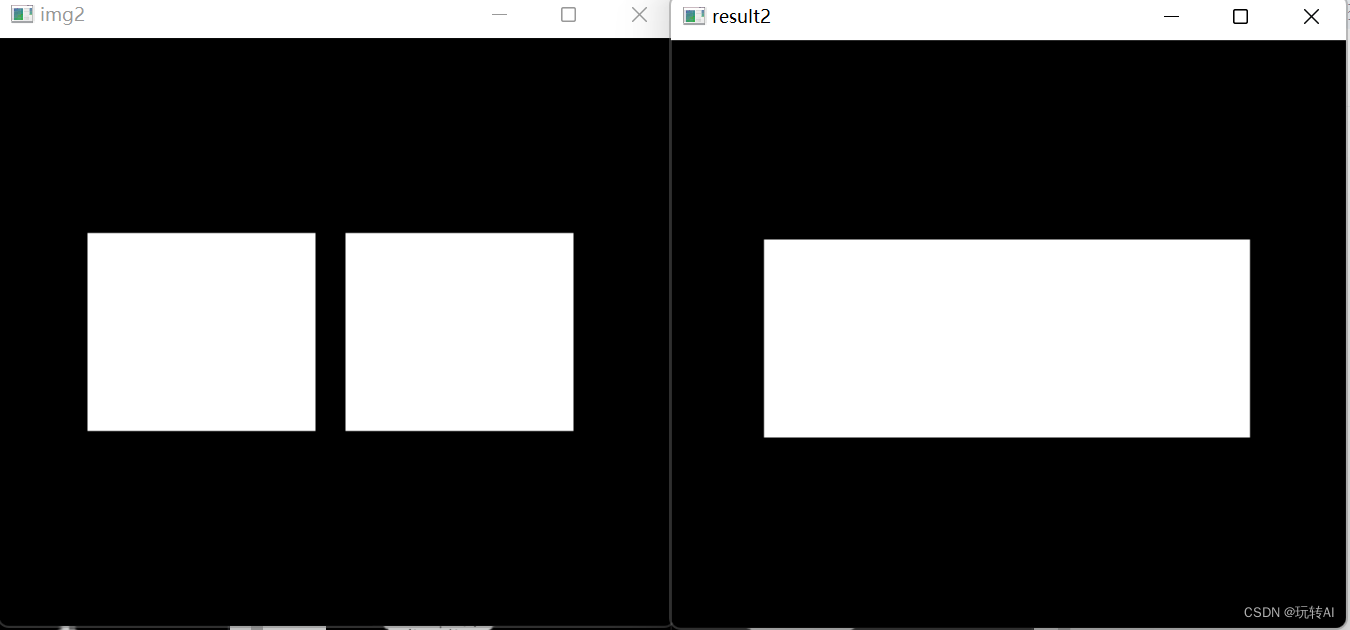
opencv-38 形态学操作-闭运算(先膨胀,后腐蚀)cv2.morphologyEx(img, cv2.MORPH_CLOSE, kernel)
闭运算是先膨胀、后腐蚀的运算,它有助于关闭前景物体内部的小孔,或去除物体上的小黑点,还可以将不同的前景图像进行连接。 例如,在图 8-17 中,通过先膨胀后腐蚀的闭运算去除了原始图像内部的小孔(内部闭合的…...
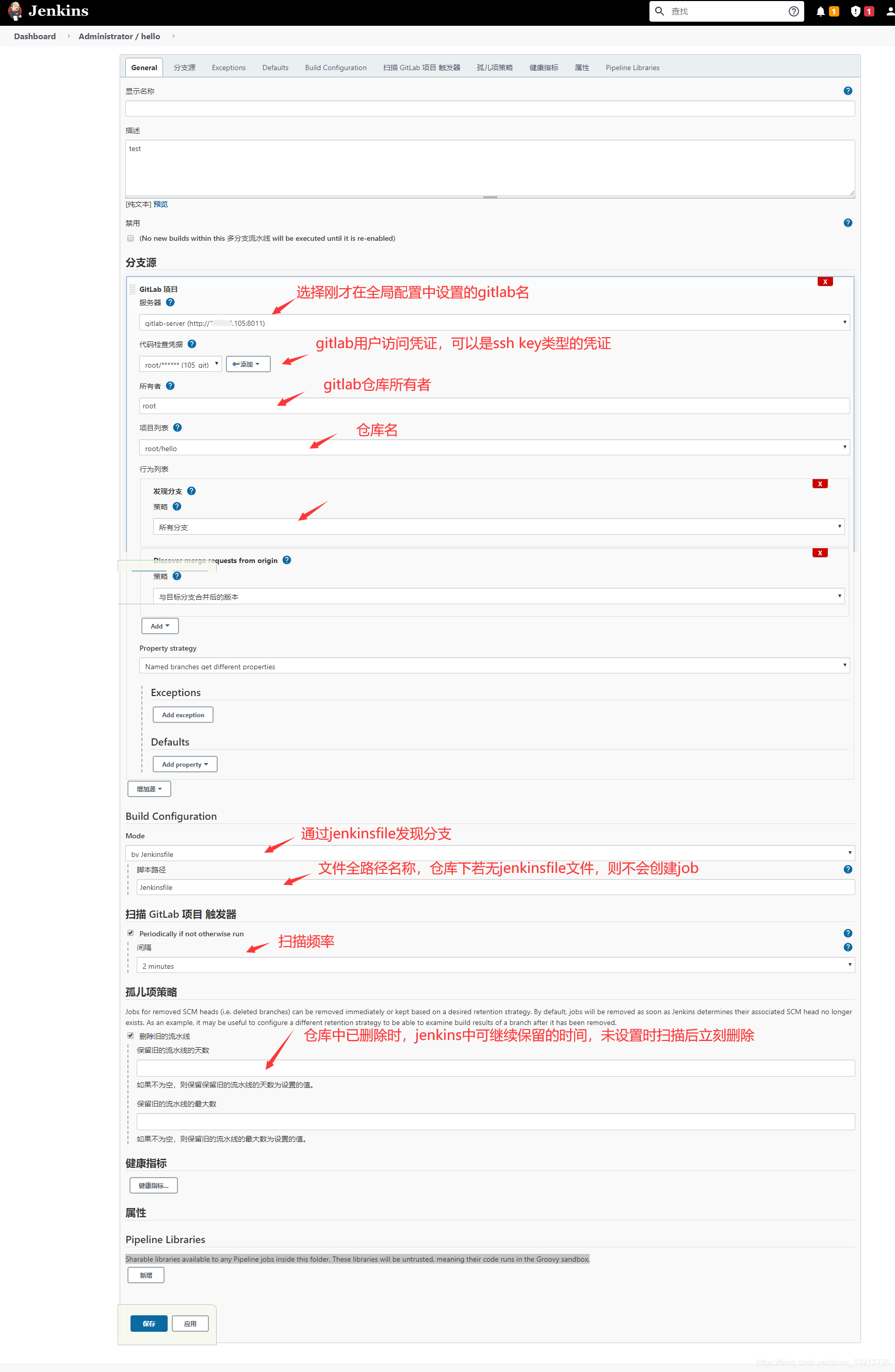
jenkins gitlab多分支构建发布
内容背景介绍 这个是新手教程,普及概念为主 公司现在还使用单分支发布测试环境和生产,多人协同开发同一个项目导致测试环境占用等待等情况 测试环境占用等待问题 测试环境代码直接合并到 master,容易导致误发布到生产的情况 避免多版本同时发布测试不完善的情况出现 中间件…...
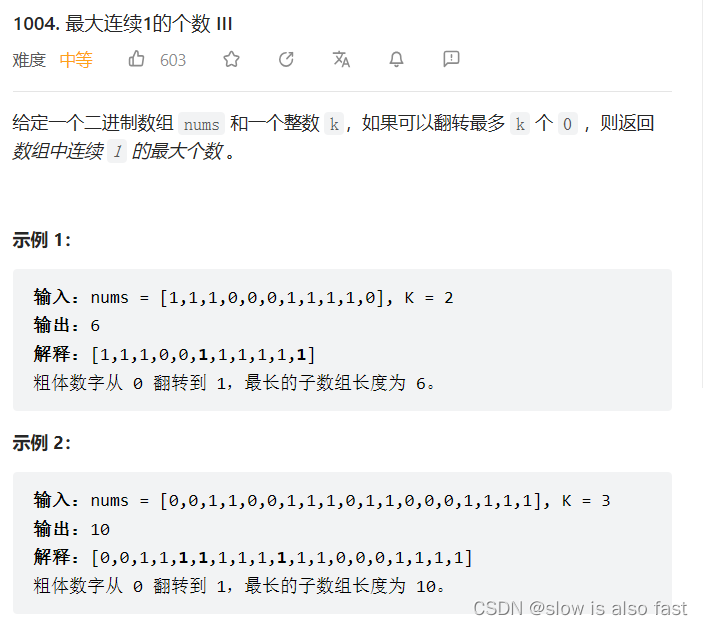
刷题笔记 day8
1004 最大连续1的个数 III 这道题要求将原数组中的0翻转成1,求出最大元素全是1的子数组长度,看这道题第一感觉还要将里面的0变成1,感觉这道题解决起来很麻烦,但是我们可以转变思路,找出其最大子数组,使得子…...

C 语言的表达式
表达式 expression 表达式由运算符和运算对象组成。 最简单的表达式是一个单独的运算对象,以此为基础可以建立复杂的表达式。 一些表达式由子表达式(subexpression)组成。子表达式即较小的表达式。 这些都是一些表达式: -4 a…...