使用 Python 进行自然语言处理第 5 部分:文本分类
一、说明
二、什么是文本分类?
- 文本分类是指将一段文本(例如,客户评论、电子邮件、网页、新闻文章)分类为一些预定义的类别或类。正面、负面或中立的评论评论、垃圾邮件或非垃圾邮件、作为个人或商业页面的网页、有关政治、体育或金融的新闻文章)
- 即,文本分类是为给定文本分配标签或类的任务。例如,将电子邮件归类为垃圾邮件。出于分类的目的,通常从输入文本中识别出一些信息量很大的特征。
- 监督机器学习和无监督学习可用于 NLP 中的文本分类。监督学习涉及在标记的数据集上训练分类器。无监督学习不需要标记的数据集,它使用数据的固有属性(相似性)将数据聚类到组中。高质量的标记数据,通常来自人类注释者,对于监督机器学习非常重要。标记的数据通常分为 3 个部分,训练集、验证集和测试集。分类器的性能使用准确率、精确度、召回率和 F1 分数等指标进行评估。
三、文本分类的重要用例:
- 情绪分析
- POS 标签
- 自然语言推理 — 推断两段文本之间的关系——前提和假设。这种关系的类型——蕴涵性、矛盾性和中性性。
- 蕴涵:假设由前提支持
- 矛盾:假设被前提否定
- 中性:假设和前提之间没有关系 例如,
- 前提:我现在正在看电影。
- 假设:我现在正在打板球。关系标签:矛盾
4. 检查语法正确性:可接受/不可接受。
四、使用 NLTK 进行文本分类
- 对于文本分类的第一个示例,我们将使用 nltk 库中内置的movie_reviews语料库。
- 您可以使用 nltk.download 函数下载 movie_reviews 包:
import nltk nltk.download("movie_reviews")
fileids() 方法允许我们访问 nltk.corpus 中数据集中所有文件的列表。movie_reviews数据集有 2000 个文本文件,每个文件都有一个 fileid。这些文件中的每一个都包含对电影的评论。其中 1000 个文件包含负面评论,1000 个包含正面评论。负面文件位于名为“neg”的文件夹中,所有包含正面评论的文件都位于名为“pos”的文件夹中。
#Required imports
import nltk
import random
from nltk.corpus import movie_reviews#Total no. of review files in corpus
##There are 1000 negative reviews, and 1000 positive reviews (one review per file)
len(movie_reviews.fileids())#separating filenames in two lists one for positive reviews, one for negative reviews(based on which folder they exists in corpus)
negative_fileids = movie_reviews.fileids('neg')
positive_fileids = movie_reviews.fileids('pos')# Now we will load all reviews and their labels (i.e., folder name pos or neg in which the review file is present)reviewswithcategory = [(list(movie_reviews.words(fileid)), category) for category in movie_reviews.categories()for fileid in movie_reviews.fileids(category)]
random.shuffle(reviewswithcategory)
接下来,让我们做一些文本预处理:
# Text pre-processing - lower casing, removing stop words and punctuation marks
import string
from nltk.corpus import stopwords
stop_words = stopwords.words('english')
def text_preprocessing(review):review = [w.lower() for w in review]review = [w.translate(str.maketrans('', '', string.punctuation)) for w in review]review = [w for w in review if w not in stop_words]review = list(filter(None, review)) #Remove empty stringsreturn reviewcleaned_reviewswithcategory = []
for review, cat in reviewswithcategory:cleanreview = text_preprocessing(review)cleaned_reviewswithcategory.append((cleanreview, cat))# Our variable cleaned_reviewswithcategory is a list of tuples,
# each tuple in it has a list of words and category label# here we all getting all words from all tuples into a single iterable
allcleanwords = list(itertools.chain(*cleaned_reviewswithcategory))
allcleanwordslist = []
for m in range(len(allcleanwords)):# traversing the inner listsfor n in range (len(allcleanwords[m])):# Add each element to the result listallcleanwordslist.append(allcleanwords[m][n])
接下来,我们从电影评论数据中清理过的单词列表中确定 5000 个最常见的单词
# Using NLTK FreqDist for computing word frequencies
freqd = nltk.FreqDist(allcleanwordslist)
# Identifying 5000 most frequent words from Frequency Distribution
frequent_words = list(freqd.keys())[:5000]
现在,我们将只使用这 5000 个单词。对于正面和负面类别中的每条评论,特征向量将包含这些常用词和一个布尔值 True(如果该词存在于该评论中),否则为 False。
# Identify the presence of these most frequent words in out positive and negative reviews.
# This function returns word and True if word is present, else it returns word and False. def extract_frequentwordfeatures(text):words = set(text) # computing all unique words (vocabulary) in input textfeatures = {}for w in frequent_words:features[w] = (w in words)return featuresreview_features = [(extract_frequentwordfeatures(review), category) for (review, category) in cleaned_reviewswithcategory]
现在,每个评论都由其特征表示,该特征是一个单词列表和一个布尔值 True 或 False,指示该单词是否存在于评论中。列表中共有 2000 条评论。接下来,我们将评审功能拆分为训练和测试部分。在 2000 条评论中,我们将使用 1800 条来训练来自 NLTK 的朴素贝叶斯分类器,并使用 200 条来测试其性能。
# Splitting the documents into training and test portions
train_data = review_features[:1800]
# set that we'll test against.
test_data = review_features[1800:]# use Naive Bayes classifier from NLTK to train
clf_nb = nltk.NaiveBayesClassifier.train(train_data)# After training, let us see the accuracy
print(" Accuracy:",(nltk.classify.accuracy(clf_nb, test_data)))
五、使用 sklearn 分类器对上述评论数据进行分类
from nltk.classify.scikitlearn import SklearnClassifier
from sklearn.linear_model import LogisticRegression
from sklearn.naive_bayes import MultinomialNB
from sklearn.svm import SVC
from sklearn.metrics import classification_report, accuracy_score, confusion_matrixnames = ['Logistic Regression','Multinomial Naive Bayes', 'Support Vector Machine']classifiers = [LogisticRegression(),MultinomialNB(),SVC(kernel='linear')]
models = zip(names, classifiers)text_features, labels = zip(*test_data)for name, model in models:nltk_clf = SklearnClassifier(model)nltk_clf.train(train_data)accuracy = nltk.classify.accuracy(nltk_clf, test_data)print("\n{} Classifier Accuracy: {}".format(name, accuracy))
六、使用 Keras 进行文本分类
在这部分,我们将使用来自 UCI 存储库的 Sentiment Labelled Sentences 数据集。此数据集包含标有正面或负面情绪的句子。情绪是分数的形式,分数是 1 表示积极情绪,0 表示消极情绪。这些句子来自三个不同的网站:imdb.com、amazon.com yelp.com 对于每个网站,存在 500 个正面句子和 500 个负面句子。在这里的示例中,我们将仅使用文件amazon_cells_labelled.txt中的亚马逊评论。
首先,我们将使用 pandas 将数据读入 pandas 数据帧。此数据有两列 — 句子和标签。句子是产品评论,标签是 0 或 1。标签 1 表示积极情绪,0 表示消极情绪。
import pandas as pd
df = pd.read_csv('amazon_cells_labelled.txt', names=['sentence', 'label'], sep='\t')
df.head()
接下来,我们对句子列中的文本进行预处理
# This process_text() function returns list of cleaned tokens of the text
import numpy
import re
import string
import unicodedata
from nltk.corpus import stopwords
from nltk.stem import WordNetLemmatizer
stop_words = stopwords.words('english')
lemmatizer = WordNetLemmatizer()def process_text(text):text = unicodedata.normalize('NFKD', text).encode('ascii', 'ignore').decode('utf-8', 'ignore')text = re.sub(r'[^a-zA-Z\s]', '', text)text = text.translate(str.maketrans('', '', string.punctuation))text = text.lower()text = " ".join([word for word in str(text).split() if word not in stop_words])text = " ".join([lemmatizer.lemmatize(word) for word in text.split()])return text
df['sentence'] = df['sentence'].apply(process_text)
df['sentence']
现在我们将数据分为训练和测试部分,在此之前,让我们将“句子”列中的文本和“标签”列中的标签分为两个pandas系列——“句子”和“标签”。
# Taking cleaned text and sentiment labels in two separate variables
sentences = df['sentence']
labels = df['label']# Splitting into train-test portions
from sklearn.model_selection import train_test_split
sentences_train, sentences_test, labels_train, labels_test = train_test_split(sentences, labels, test_size=0.25, random_state=1000)
接下来,我们使用 sklearn 中带有 CountVectorizer 的词袋模型以向量形式表示句子中的文本
# Converting sentences to vectors using Bag of words model with CountVectorizer
from sklearn.feature_extraction.text import CountVectorizer
cv = CountVectorizer()
cv.fit(sentences_train)vc_traindata = cv.transform(sentences_train)
vc_testdata = cv.transform(sentences_test)
vc_traindata
现在我们将使用 Keras 进行分类,因此应该在我们的计算机上安装 TensorFlow 和 Keras 库。可以使用 pip 命令从 Jupyter Notebook 中安装它们
!pip install tensorflow
!pip install keras
首先,我们将使用 Keras Tokenizer 来计算文本的单词嵌入
# Using Keras Tokenizer to compute embeddings for text
from keras.preprocessing.text import Tokenizer
tokenizer = Tokenizer(num_words=5000)
tokenizer.fit_on_texts(df['sentence'])# Take the sentence text in a variable X and labels in y.
X = df['sentence']
y = df['label']#Splitting X and y into train and test portions
X_train, X_test, y_train, y_test = train_test_split(X, y, test_size=0.25, random_state=1000)#converting sentences into vector sequences
X_train = tokenizer.texts_to_sequences(X_train)
X_test = tokenizer.texts_to_sequences(X_test)# Total number of unique words in our sentences
vocab_size = len(tokenizer.word_index) + 1 # Adding 1 because of reserved 0 index
print('Vocabulary size:' , vocab_size)
然后,我们将填充所有向量(代表产品评论的文本)的长度相同 — 50
# padding vector sequences to make them all of same length
from keras_preprocessing.sequence import pad_sequences
maxlen = 50
X_train = pad_sequences(X_train, padding='post', maxlen=maxlen)
X_test = pad_sequences(X_test, padding='post', maxlen=maxlen)
print(X_train[4, :])
七、使用 Keras 的嵌入层
我们现在将使用 Keras 的嵌入层,它采用先前计算的整数并将它们映射到嵌入的密集向量。它需要以下参数:
- input_dim:词汇量的大小
- output_dim: the size of the dense vector
- input_length: the length of the sequence
我们可以获取嵌入层的输出并将其插入密集层。为此,我们需要在中间添加一个 Flatten 层,为 Dense 层准备顺序输入。
在训练期间,要训练的参数数vacab_size乘以embedding_dim。嵌入层的权重是随机初始化的,然后在训练期间使用反向传播进行微调。该模型将按句子顺序出现的单词作为输入向量
在下面的代码中,我们使用嵌入层 GlobalMaxPool1D 进行扁平化,以及由 15 个神经元和一个输出层组成的密集层。我们编译了 keras 模型。
from keras.models import Sequential
from keras import layersembedding_dim = 100
maxlen = 50
model = Sequential()
model.add(layers.Embedding(input_dim=vocab_size, output_dim=embedding_dim, input_length=maxlen))
model.add(layers.GlobalMaxPool1D())
model.add(layers.Dense(15, activation='relu'))
model.add(layers.Dense(1, activation='sigmoid'))
model.compile(optimizer='adam',loss='binary_crossentropy',metrics=['accuracy'])
model.summary()
接下来,我们将模型拟合在 50 个 epoch 的训练数据上,并评估其性能。使用 matplotlib 绘制模型的准确性
import matplotlib.pyplot as plt
plt.figure(figsize=(9, 5))history = model.fit(X_train, y_train,epochs=50, verbose=False,validation_data=(X_test, y_test), batch_size=10)
loss, accuracy = model.evaluate(X_train, y_train, verbose=False)
print("Training Accuracy: {:.4f}".format(accuracy))
loss, accuracy = model.evaluate(X_test, y_test, verbose=False)
print("Testing Accuracy: {:.4f}".format(accuracy))acc = history.history['accuracy']
val_acc = history.history['val_accuracy']
loss = history.history['loss']
val_loss = history.history['val_loss']
x = range(1, len(acc) + 1)
plt.plot(x, acc, 'b', label='Training acc')
plt.plot(x, val_acc, 'r', label='Validation acc')
plt.legend()
plt.title('Training and validation accuracy')
plt.show()
在下一篇文章中,我们将看看变形金刚。代码出处尼姆里塔·库尔
参考和引用:
- https://developers.google.com/machine-learning/guides/text-classification
- Text Classification with Python and Scikit-Learn
- https://towardsdatascience.com/a-practitioners-guide-to-natural-language-processing-part-i-processing-understanding-text-9f4abfd13e72
- Text Classification using NLTK | Foundations of AI & ML
- Practical Text Classification With Python and Keras – Real Python
- Text Classification with NLTK | Chan`s Jupyter
- https://www.analyticsvidhya.com/blog/2018/04/a-comprehensive-guide-to-understand-and-implement-text-classification-in-python/
- https://medium.com/analytics-vidhya/nlp-tutorial-for-text-classification-in-python-8f19cd17b49e
- Practical Text Classification With Python and Keras – Real Python
相关文章:
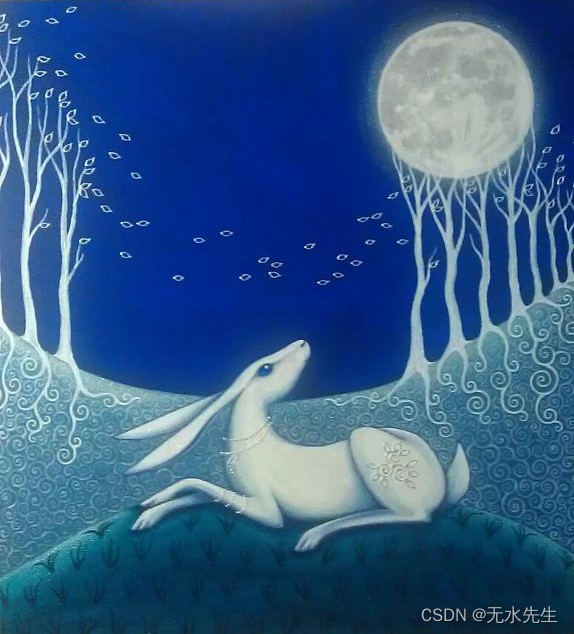
使用 Python 进行自然语言处理第 5 部分:文本分类
一、说明 关于文本分类,文章已经很多,本文这里有实操代码,明确而清晰地表述这种过程,是实战工程师所可以参照和依赖的案例版本。 本文是 2023 年 1 月的 WomenWhoCode 数据科学跟踪活动提供的会议系列文章中的一篇。 之前的文章在…...

uni-app---- 点击按钮拨打电话功能点击按钮调用高德地图进行导航的功能【安卓app端】
uniapp---- 点击按钮拨打电话功能&&点击按钮调用高德地图进行导航的功能【安卓app端】 先上效果图: 1. 在封装方法的文件夹下新建一个js文件,然后把这些功能进行封装 // 点击按钮拨打电话 export function getActionSheet(phone) {uni.showAct…...
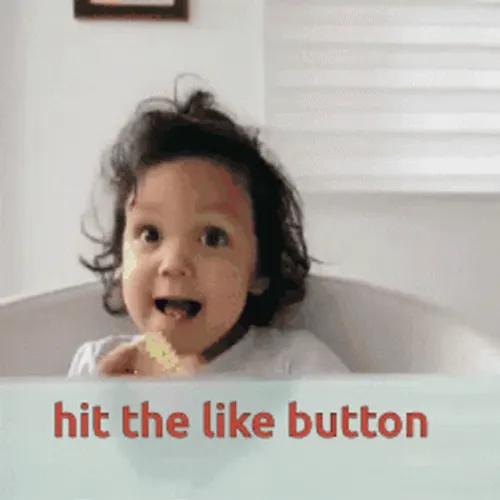
通讯录详解(静态版,动态版,文件版)
💓博客主页:江池俊的博客⏩收录专栏:C语言进阶之路👉专栏推荐:✅C语言初阶之路 ✅数据结构探索✅C语言刷题专栏💻代码仓库:江池俊的代码仓库🎉欢迎大家点赞👍评论&#x…...

在windows中搭建vue开发环境
1.环境搭建 具体环境搭建步骤参考链接 注意该博客中初始化命令: vue init webpack MyPortalProject需改为小写: vue init webpack myportalproject不然会报错 Warning: name can no longer contain capital letters2.创建第一个vueelement ui项目 …...
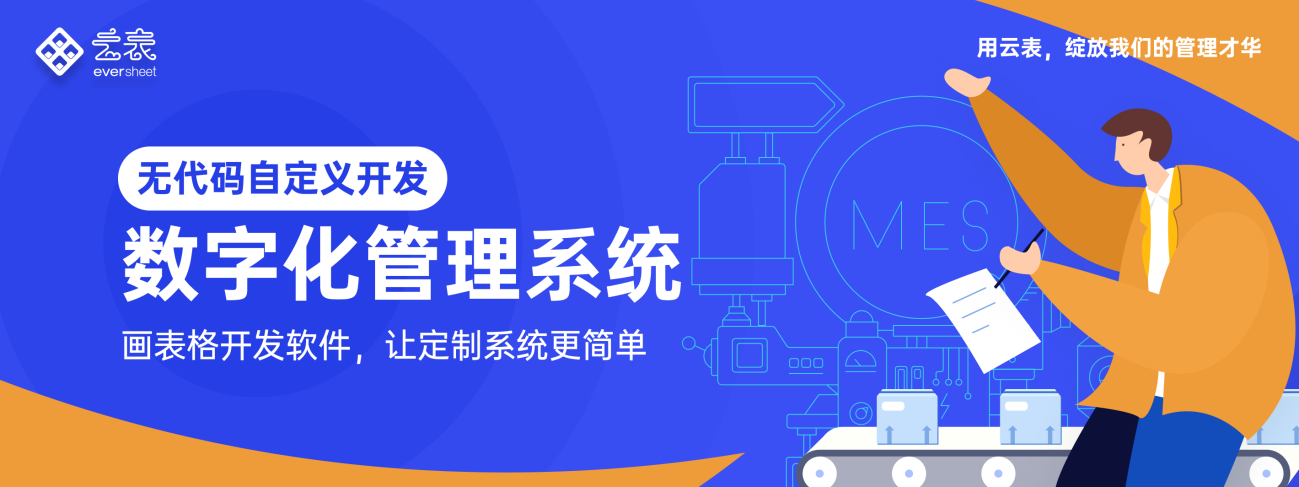
数字化转型:云表低代码开发助力制造业腾飞
数字化转型已成为制造业不可避免的趋势。为了应对市场快速变化、提高运营效率以及降低成本,制造业企业积极追求更加智能化、敏捷的生产方式。在这个转型过程中,低代码技术作为一种强大的工具,正逐渐崭露头角,有望加速制造业的数字…...
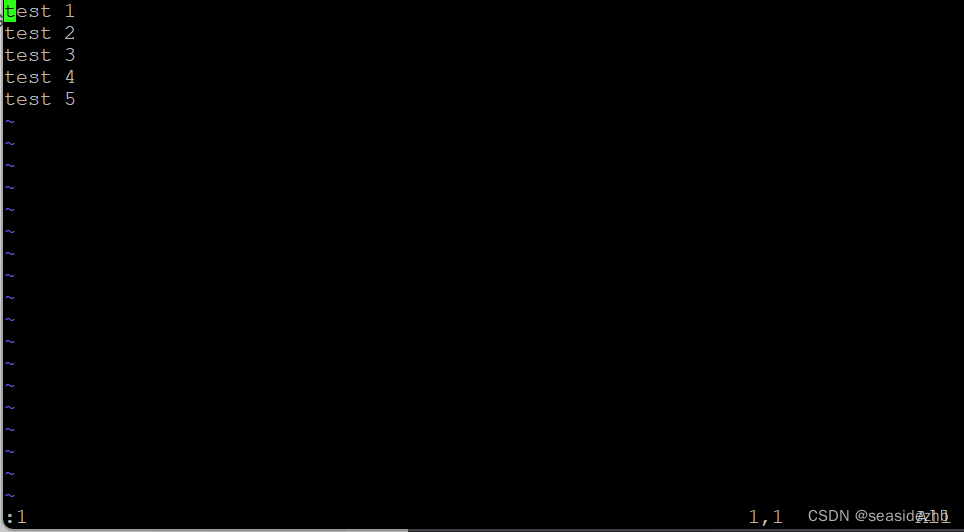
Linux学习之vim跳转到特定行数
参考的博客:《Vim跳到最后一行的方法》 《oeasy教您玩转vim - 14 - # 行头行尾》 《Linux:vim 中跳到首行和最后一行》 想要跳到特定行的话,可以在命令模式和正常模式进行跳转。要是对于vim的四种模式不太熟的话,可以到博客《Linu…...
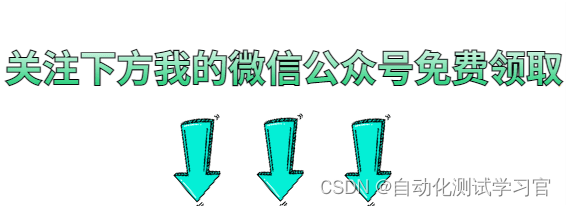
详解基于Android的Appium+Python自动化脚本编写
📢专注于分享软件测试干货内容,欢迎点赞 👍 收藏 ⭐留言 📝 如有错误敬请指正!📢交流讨论:欢迎加入我们一起学习!📢资源分享:耗时200小时精选的「软件测试」资…...
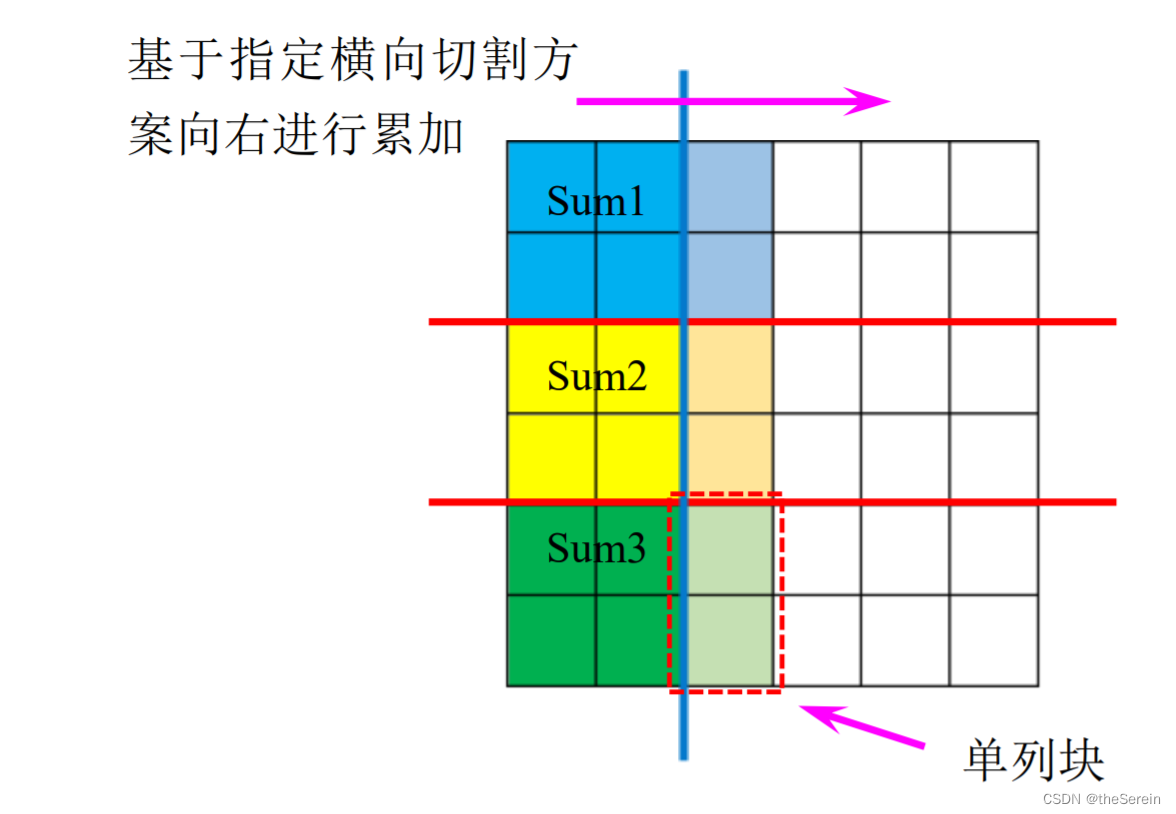
【马蹄集】—— 百度之星 2023
百度之星 2023 目录 BD202301 公园⭐BD202302 蛋糕划分⭐⭐⭐BD202303 第五维度⭐⭐ BD202301 公园⭐ 难度:钻石 时间限制:1秒 占用内存:64M 题目描述 今天是六一节,小度去公园玩,公园一共 N N N 个景点&am…...
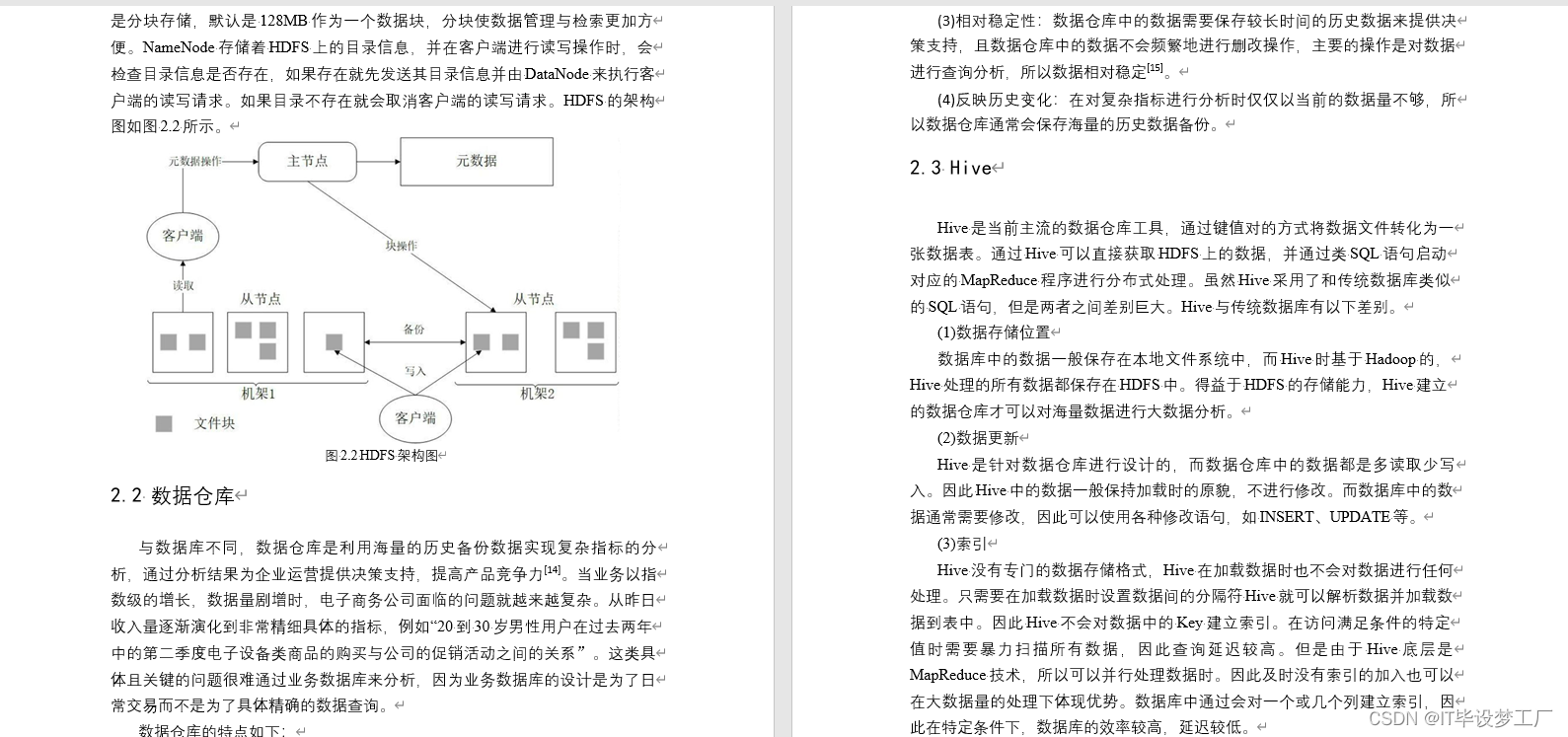
大数据毕业设计选题推荐-无线网络大数据平台-Hadoop-Spark-Hive
✨作者主页:IT毕设梦工厂✨ 个人简介:曾从事计算机专业培训教学,擅长Java、Python、微信小程序、Golang、安卓Android等项目实战。接项目定制开发、代码讲解、答辩教学、文档编写、降重等。 ☑文末获取源码☑ 精彩专栏推荐⬇⬇⬇ Java项目 Py…...

【jvm】虚拟机之本地方法接口与本地方法库
目录 一、本地方法1.1 说明1.2 代码示例1.3 为什么要使用native method 二、现状 一、本地方法 1.1 说明 1.一个Native Method就是一个Java调用非Java代码的接口。 2.一个Native Method是这样一个Java方法:该方法的实现由非Java语言实现,比如C。 3.这个…...
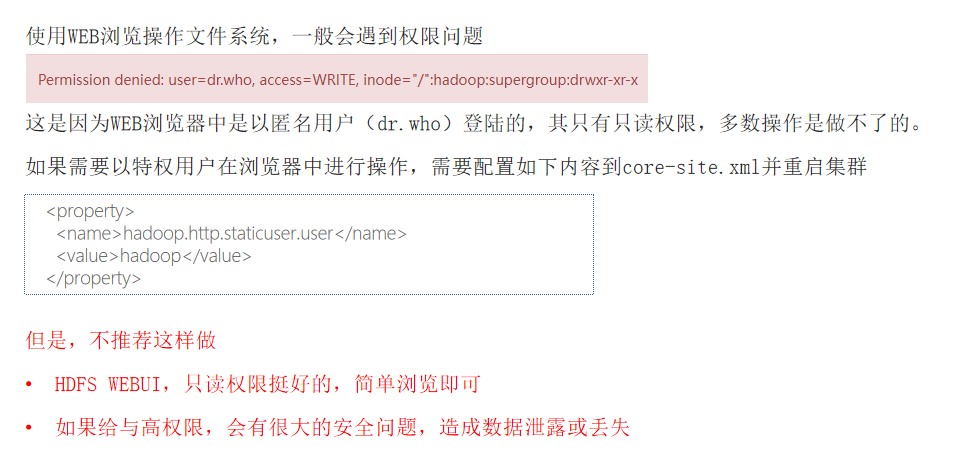
HDFS系统操作命令大全
一,前言 HDFS作为分布式存储的文件系统,有其对数据的路径表达方式 HDFS同linux系统一样,均是以/作为根目录的组织形式 linux:/usr/local/hello.txt HDFS:/usr/local/hello.txt 二,如何区分呢? L…...

雷尼绍探头编程 9810
9810 安全移动 使用参数 参数含义#9移动速度 F#117移动速度 F#148#24X 移动 终点绝对坐标#25Y 移动 终点绝对坐标#26Z 移动 终点绝对坐标#123机床移动到终点的绝对坐标 与 终点的理论值 的 差#5041当前绝对坐标 X 值#5042当前绝对坐标 Y 值#5043当前绝对坐标 Z 值#116刀具…...
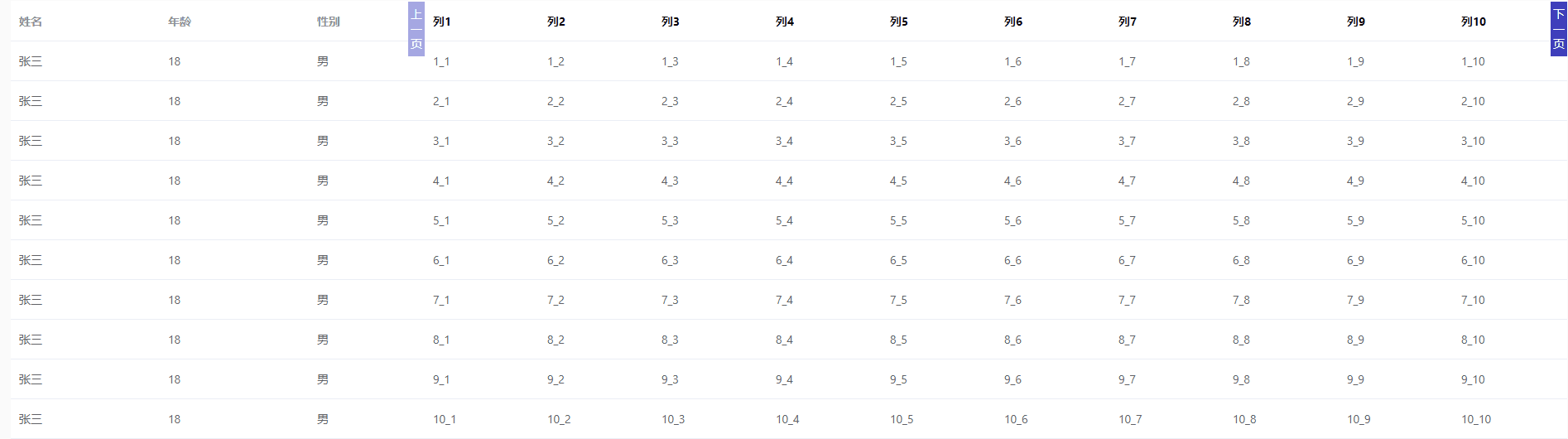
el-table 列分页
<template><div><el-table:data"tableData":key"tampTime"style"width: 100%"><el-table-columnprop"name"label"姓名"width"180"></el-table-column><el-table-columnprop&quo…...

APP攻防--ADB基础
进入app包 先使用 adb devices查看链接状态 手机连接成功的 adb shell 获取到手机的一个shell 此时想进入app包时没有权限的,APP包一般在data/data/下。没有执行权限,如图 Permission denied 权限被拒绝 此时需要手机root,root后输入 su …...
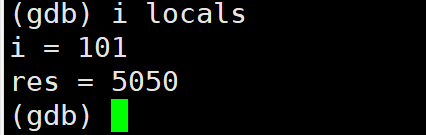
【Linux】第十站:git和gdb的基本使用
文章目录 一、git的基本操作1.gitee新建仓库注意事项2.git的安装3.git的克隆4.git的add5.git的commit6.git的push7.git log8.git status9. .gitignore 二、Linux调试器---gdb1.背景2.gdb安装、进入与退出3.list/l4.r/run运行程序5. break/b 打断点6.info/i b 查看断点7.delete/…...
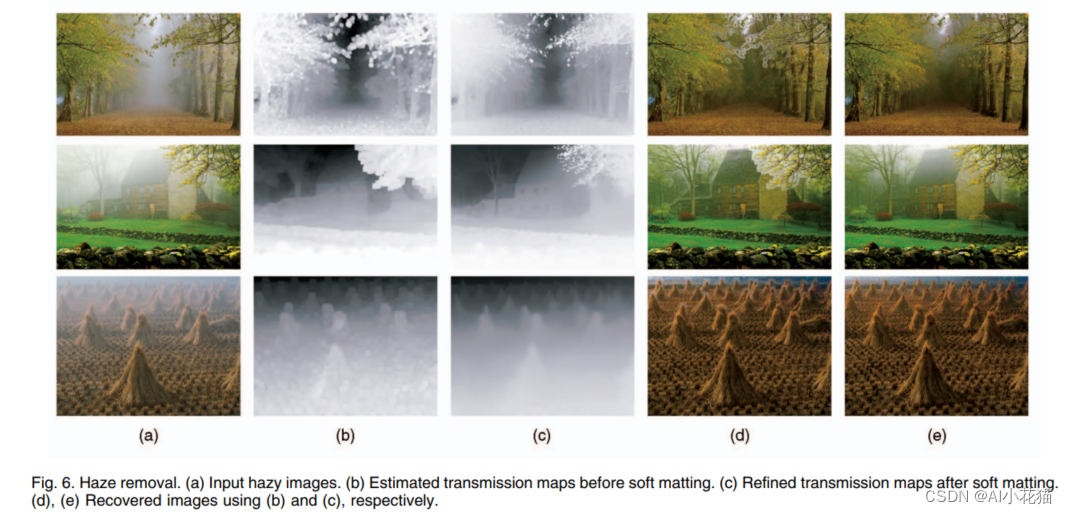
Single Image Haze Removal Using Dark Channel Prior(暗通道先验)
去雾算法都会依赖于很强的先验以及假设,并结合相应的物理模型,完成去雾过程。本文作者何凯明及其团队通过大量的无雾图像和有雾图像,归纳总结出无雾图像在其对应的暗通道图像上具有极低的强度值(趋近于0),并…...

力扣382.链表随机节点(java利用数组随机返回节点值)
Problem: 382. 链表随机节点 文章目录 思路解题方法复杂度Code 思路 注意链表与数组的特性,对于随机访问读取的操作利用数组可以较方便实现,所以我们可以将链表中的节点值先存入到数组中最后再取出随机生成节点位置的值。 解题方法 1.生成List集合与Rand…...
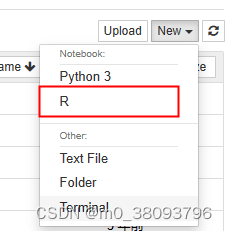
在jupyter中使用R
如果想在Jupyter Notebook中使用R语言,以下几个步骤操作可行: 1、启动Anaconda Prompt 2、进入R的安装位置,切换到R的安装位置:D:\Program Files\R\R-3.4.3\bin,启动R,具体代码操作步骤如下,在…...
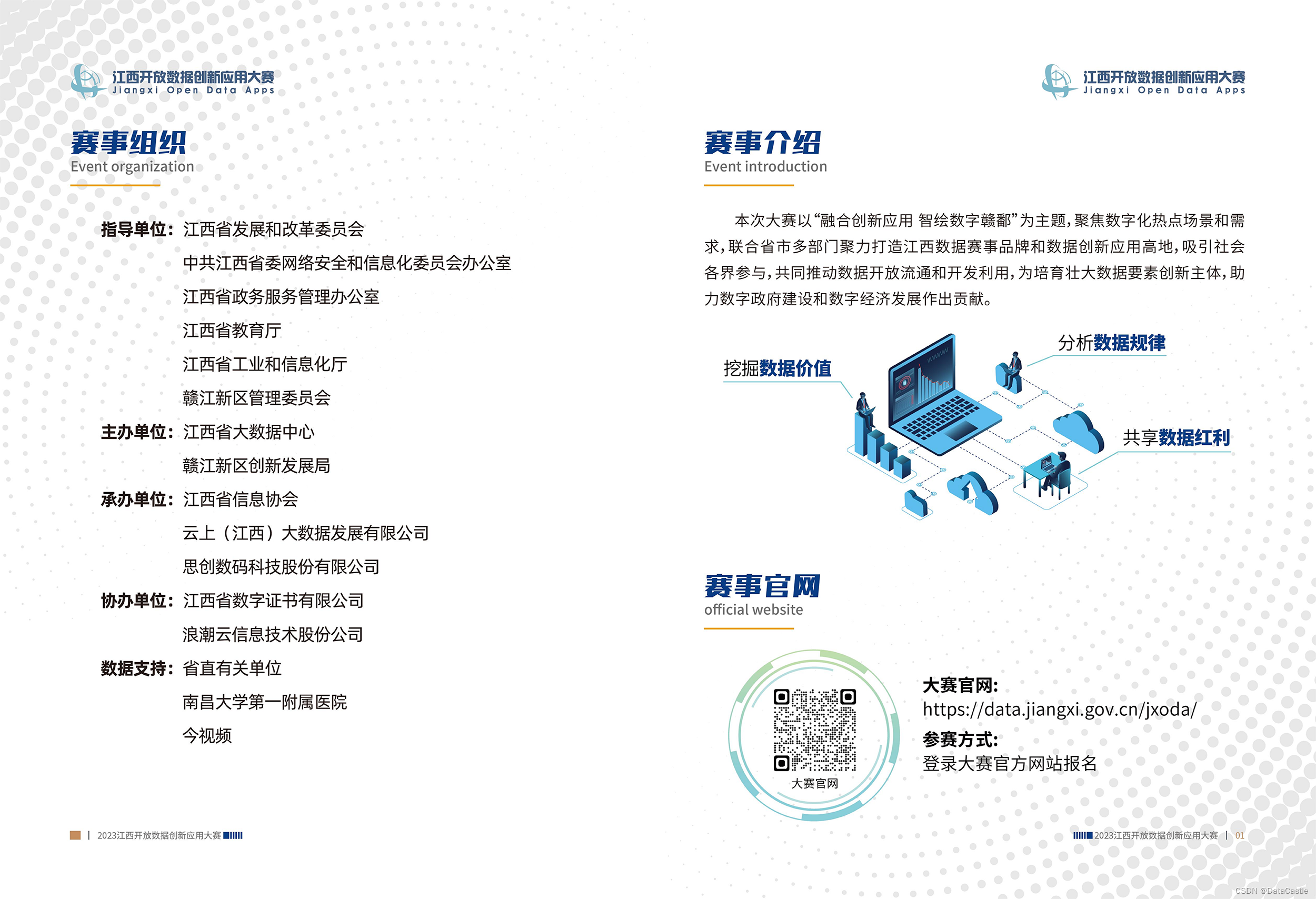
2023(第四届)江西开放数据创新应用大赛等你来挑战!
邀请函 这是一个友好的邀请。无论你是数据领域的专家、学生还是爱好者,我们都欢迎你加入这个平台。这不仅仅是一场比赛,更是一个交流、学习和展示自己的机会。 丰厚奖金:我们为参赛者准备了总计15W的奖金池,期待你的才华在这里得…...

2023-mac rz sz 安装
之前安装过一次,没问题,这次按照之前教程装了就不管上传下载都会卡住; step1: brew install lrzsz step2:在/usr/local/bin 路径下配置两个sh,之前从网上找到的直接用都不对,下面这个是调试过的正式可用的 iterm2…...
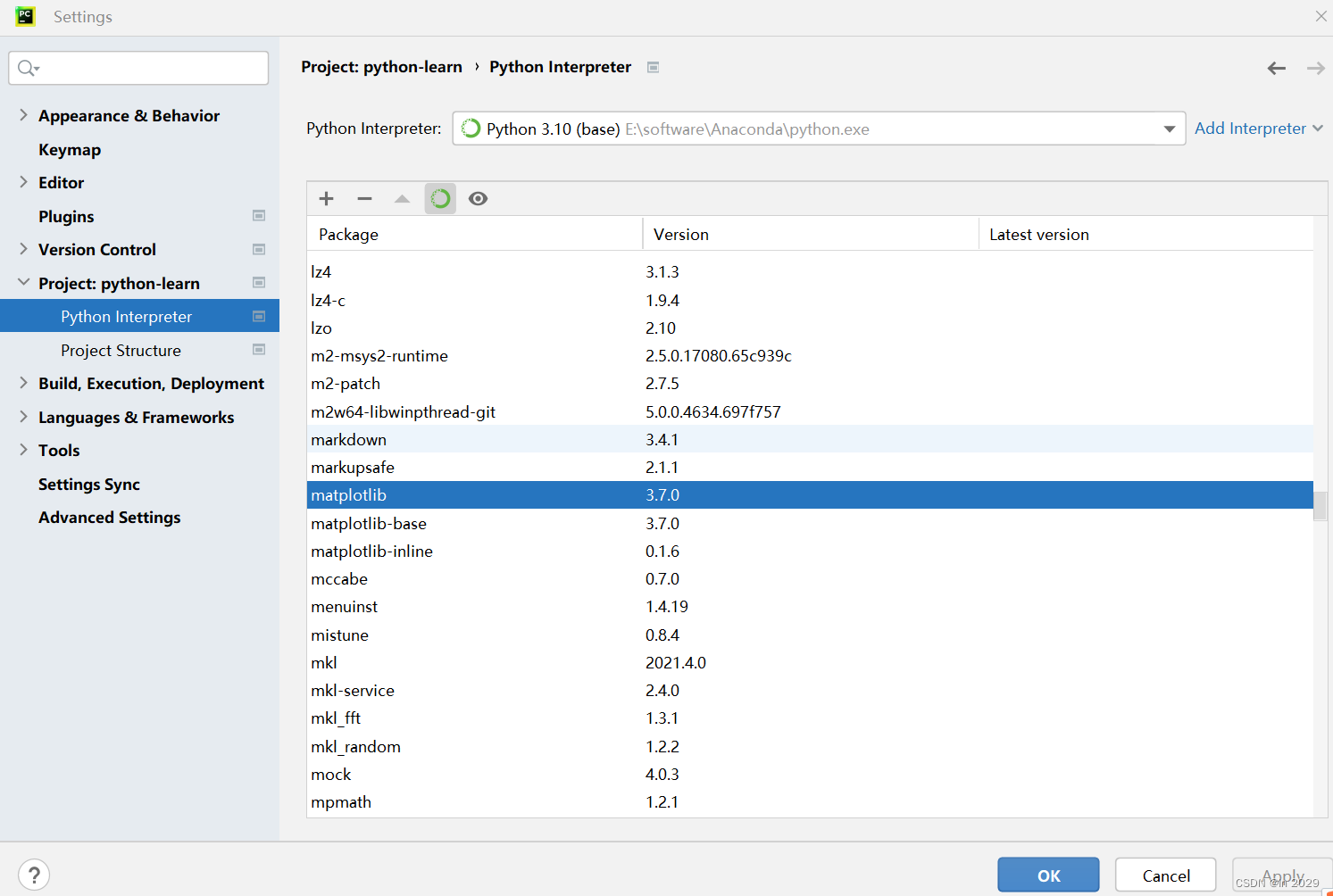
使用Matplotlib绘画3D图时运行不出结果,也不报错,图片是空白 !!
1.问题: 我使用如下代码运用matplotlib中的Axes3D绘画3D图,但是运行出来的结果是空白。 import numpy as np import matplotlib.pyplot as plt from mpl_toolkits.mplot3d import Axes3D #导入3D包 fig plt.figure() #窗口 #ax Axes3D(fig) # X, Y …...

Matlab函数——find
介绍 当你需要返回某个数组中符合指定条件的所有元素的索引时,可以使用 MATLAB 中的 find 函数。 find 函数语法: indices find(X) indices find(X, k) indices find(X, k, first) indices find(X, k, last) 其中,X 是一个数组…...
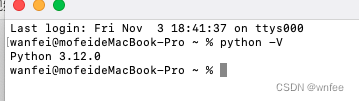
mac安装python3
文章目录 1. 安装1.1 brew安装(失败)2. 下载安装包 2. 查看版本3. 配置 1. 安装 1.1 brew安装(失败) brew install python3下载完成后报错:Error: python3.10: unknown or unsupported macOS version: :dunno 解决&a…...
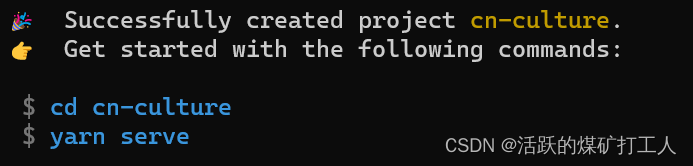
【星海出品】VUE(一)
Windows安装nvm控制器 Windows里找都PowerShell。右击点击管理员运行。 1.安装choco Set-ExecutionPolicy Bypass -Scope Process -Force; iex ((New-Object System.Net.WebClient).DownloadString(https://chocolatey.org/install.ps1))2.安装NVM choco install nvm 3.查看可…...
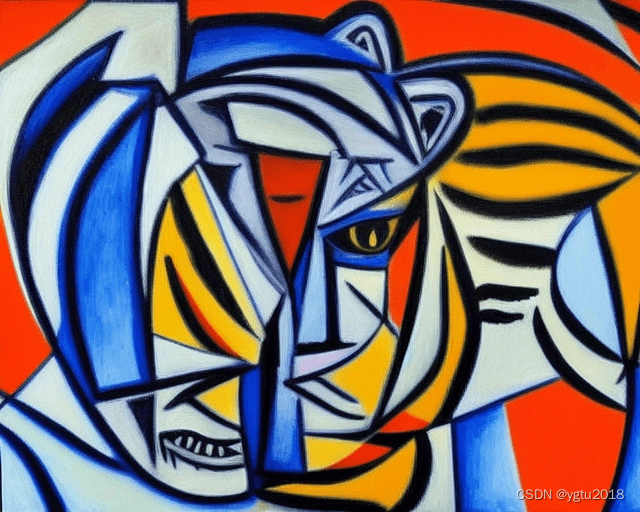
Stable Diffusion 的提示词使用技巧
推荐Stable Diffusion自动纹理工具: DreamTexture.js自动纹理化开发包 什么是提示语? 提示语是人工智能中的一个重要组成部分,尤其是自然语言处理 (NLP)。在AI自人工智能中,想要获得好的效果,简…...

Hook函数
在嵌入式系统中,hook函数(也被称为钩子函数)是一种特殊类型的函数,它会在特定的事件发生时被操作系统内部调用。例如,在实时操作系统(RTOS)中,如果删除了一个任务,就会调…...

USB简介系列-01
文章目录 USB简介一、电气USB简介 通用串行总线(USB)是由Compaq,Intel,Microsoft和NEC开发的规范,后来惠普,朗讯和飞利浦加入。这些公司成立了 USB Implementers Forum, Inc 作为一家非营利性公司,以发布规范并组织 USB 的进一步开发。 USB-IF的目的是为当时使用的PC…...

算法小白的心得笔记:比较小数点后五位,而不会受到浮点数精度问题的影响。
epsilon 来比较浮点数 double epsilon 1e-6; // for 6 decimal places for (const auto &ratio : colorRatio) {std::cout << "__" << inum << "__" << ratio << " ";if ((inum - 1) % 10 0){std::cout &l…...
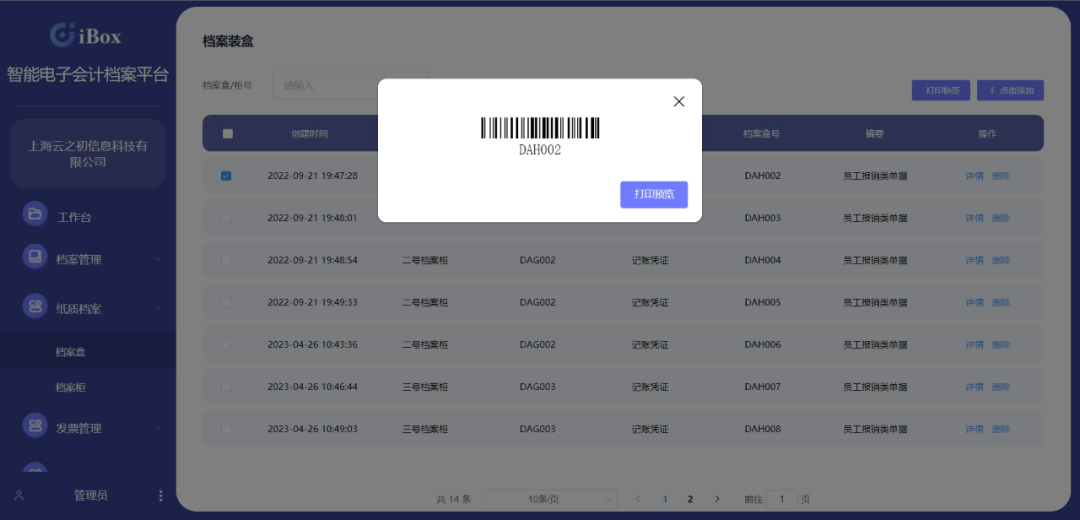
11月起,33个省份纳入数电票开票试点范围内,发票无纸化已是大势所趋!
10月底,北京、贵州、山东(不含青岛市)、湖南、宁夏5个地区相继发布开展数电票试点工作的通知,至此,全国已有33个省份纳入数电票开票试点范围内。根据上述5地区发布的相关公告,11月1日将正式推行“数电票”开…...
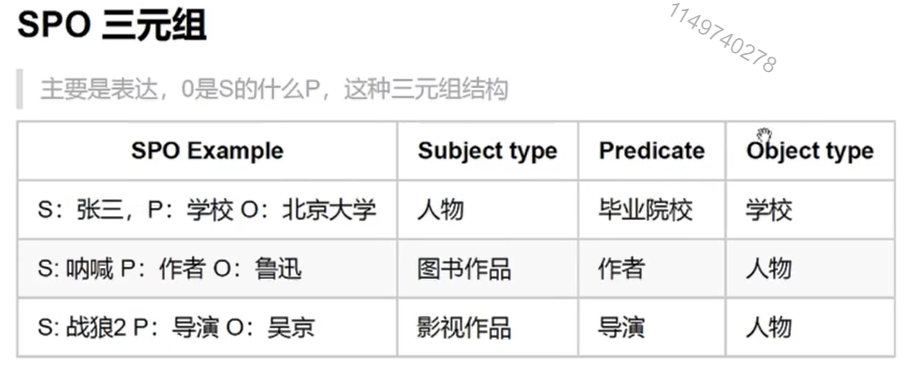
NLP之Bert多分类实现案例(数据获取与处理)
文章目录 1. 代码解读1.1 代码展示1.2 流程介绍1.3 debug的方式逐行介绍 3. 知识点 1. 代码解读 1.1 代码展示 import json import numpy as np from tqdm import tqdmbert_model "bert-base-chinese"from transformers import AutoTokenizertokenizer AutoToken…...