AI--构建检索增强生成 (RAG) 应用程序
LLM 所实现的最强大的应用之一是复杂的问答 (Q&A) 聊天机器人。这些应用程序可以回答有关特定源信息的问题。这些应用程序使用一种称为检索增强生成 (RAG) 的技术。
典型的 RAG 应用程序有两个主要组件
- 索引:从源中提取数据并对其进行索引的管道。这通常在线下进行。
- 检索和生成:实际的 RAG 链,它在运行时接受用户查询并从索引中检索相关数据,然后将其传递给模型。
从原始数据到答案最常见的完整序列如下:
- 加载:首先我们需要加载数据。这是通过DocumentLoaders完成的。
- 拆分:文本拆分器将大块内容拆分Documents成小块内容。这对于索引数据和将数据传递到模型都很有用,因为大块内容更难搜索,并且不适合模型的有限上下文窗口。
- 存储:我们需要一个地方来存储和索引我们的分割,以便以后可以搜索它们。这通常使用VectorStore和Embeddings模型来完成
检索和生成
4. 检索:根据用户输入,使用检索器从存储中检索相关分割。
5. 生成:ChatModel / LLM使用包含问题和检索到的数据的提示生成答案
#创建embedding 模型
from langchain.embeddings import HuggingFaceEmbeddings
from langchain_community.vectorstores import FAISS
from langchain_community.vectorstores.utils import DistanceStrategy
from config import EMBEDDING_PATH# init embedding model
model_kwargs = {'device': 'cuda'}
encode_kwargs = {'batch_size': 64, 'normalize_embeddings': True}embed_model = HuggingFaceEmbeddings(model_name=EMBEDDING_PATH,model_kwargs=model_kwargs,encode_kwargs=encode_kwargs)#导入相关库
from langchain_openai import ChatOpenAI
import bs4
from langchain import hub
from langchain_community.vectorstores import FAISS
from langchain_community.document_loaders import WebBaseLoader
from langchain_core.output_parsers import StrOutputParser
from langchain_core.runnables import RunnablePassthrough
from langchain_text_splitters import RecursiveCharacterTextSplitterchat = ChatOpenAI()loader = WebBaseLoader(web_paths=("https://lilianweng.github.io/posts/2023-06-23-agent/",),bs_kwargs=dict(parse_only=bs4.SoupStrainer(class_=("post-content", "post-title", "post-header"))),
)
docs = loader.load()documents = RecursiveCharacterTextSplitter(chunk_size=1000,chunk_overlap=200).split_documents(docs)vetorstors = FAISS.from_documents(documents,embed_model)retriever = vetorstors.as_retriever()promt = hub.pull("rlm/rag-prompt")promtdef format_docs(docs):return "\n\n".join(doc.page_content for doc in docs)#创建链
chain =({"context":retriever | format_docs ,"question":RunnablePassthrough()}| promt| chat| StrOutputParser()
)chain.invoke("What is Task Decomposition?")
输出结果
‘Task decomposition is the process of breaking down a problem into multiple thought steps to create a tree structure. It can be achieved through LLM with simple prompting, task-specific instructions, or human inputs. The goal is to transform big tasks into smaller and simpler steps to enhance model performance on complex tasks.’
首先:这些组件(retriever、prompt、chat等)中的每一个都是Runnable的实例。这意味着它们实现相同的方法——例如sync和async .invoke、、.stream或.batch——这使得它们更容易连接在一起。它们可以通过运算符|连接到RunnableSequence(另一个 Runnable)。
当遇到|操作符时,LangChain 会自动将某些对象转换为 Runnable。这里,format_docs转换为RunnableLambda"context" ,带有和的字典"question"转换为RunnableParallel。细节并不重要,重要的是,每个对象都是一个 Runnable。
让我们追踪一下输入问题如何流经上述可运行程序。
正如我们在上面看到的,输入prompt预计是一个带有键"context"和 的字典"question"。因此,该链的第一个元素构建了可运行对象,它将根据输入问题计算这两个值:
retriever | format_docs: 将文本传递给检索器,生成Document对象,然后将Document对象format_docs生成字符串;
RunnablePassthrough()不变地通过输入问题。
内置Chain
from langchain.chains import create_retrieval_chain
from langchain.chains.combine_documents import create_stuff_documents_chain
from langchain_core.prompts import ChatPromptTemplatesystem_prompt = ("You are an assistant for question-answering tasks. ""Use the following pieces of retrieved context to answer ""the question. If you don't know the answer, say that you ""don't know. Use three sentences maximum and keep the ""answer concise.""\n\n""{context}"
)prompt = ChatPromptTemplate.from_messages([("system", system_prompt),("human", "{input}"),]
)question_answer_chain = create_stuff_documents_chain(chat, prompt)
rag_chain = create_retrieval_chain(retriever, question_answer_chain)response = rag_chain.invoke({"input":"What is Task Decomposition?"})
print(response)
输出结果:
{‘input’: ‘What is Task Decomposition?’, ‘context’: [Document(page_content=‘Tree of Thoughts (Yao et al. 2023) extends CoT by exploring multiple reasoning possibilities at each step. It first decomposes the problem into multiple thought steps and generates multiple thoughts per step, creating a tree structure. The search process can be BFS (breadth-first search) or DFS (depth-first search) with each state evaluated by a classifier (via a prompt) or majority vote.\nTask decomposition can be done (1) by LLM with simple prompting like “Steps for XYZ.\n1.”, “What are the subgoals for achieving XYZ?”, (2) by using task-specific instructions; e.g. “Write a story outline.” for writing a novel, or (3) with human inputs.’, metadata={‘source’: ‘https://lilianweng.github.io/posts/2023-06-23-agent/’}), Document(page_content=‘Fig. 1. Overview of a LLM-powered autonomous agent system.\nComponent One: Planning#\nA complicated task usually involves many steps. An agent needs to know what they are and plan ahead.\nTask Decomposition#\nChain of thought (CoT; Wei et al. 2022) has become a standard prompting technique for enhancing model performance on complex tasks. The model is instructed to “think step by step” to utilize more test-time computation to decompose hard tasks into smaller and simpler steps. CoT transforms big tasks into multiple manageable tasks and shed lights into an interpretation of the model’s thinking process.’, metadata={‘source’: ‘https://lilianweng.github.io/posts/2023-06-23-agent/’}), Document(page_content=‘Fig. 2. Examples of reasoning trajectories for knowledge-intensive tasks (e.g. HotpotQA, FEVER) and decision-making tasks (e.g. AlfWorld Env, WebShop). (Image source: Yao et al. 2023).\nIn both experiments on knowledge-intensive tasks and decision-making tasks, ReAct works better than the Act-only baseline where Thought: … step is removed.\nReflexion (Shinn & Labash 2023) is a framework to equips agents with dynamic memory and self-reflection capabilities to improve reasoning skills. Reflexion has a standard RL setup, in which the reward model provides a simple binary reward and the action space follows the setup in ReAct where the task-specific action space is augmented with language to enable complex reasoning steps. After each action a t a_t at, the agent computes a heuristic h t h_t ht and optionally may decide to reset the environment to start a new trial depending on the self-reflection results.’, metadata={‘source’: ‘https://lilianweng.github.io/posts/2023-06-23-agent/’}), Document(page_content=‘Here are a sample conversation for task clarification sent to OpenAI ChatCompletion endpoint used by GPT-Engineer. The user inputs are wrapped in {{user input text}}.\n[\n {\n “role”: “system”,\n “content”: “You will read instructions and not carry them out, only seek to clarify them.\nSpecifically you will first summarise a list of super short bullets of areas that need clarification.\nThen you will pick one clarifying question, and wait for an answer from the user.\n”\n },\n {\n “role”: “user”,\n “content”: “We are writing {{a Super Mario game in python. MVC components split in separate files. Keyboard control.}}\n”\n },\n {\n “role”: “assistant”,’, metadata={‘source’: ‘https://lilianweng.github.io/posts/2023-06-23-agent/’})], ‘answer’: ‘Task decomposition involves breaking down a complex task into smaller and simpler steps to make it more manageable. This technique allows models or agents to utilize more computational resources at test time by thinking step by step. By decomposing tasks, models can better understand and interpret the thinking process involved in solving difficult problems.’}
create_stuff_documents_chain
def create_stuff_documents_chain(llm: LanguageModelLike,prompt: BasePromptTemplate,*,output_parser: Optional[BaseOutputParser] = None,document_prompt: Optional[BasePromptTemplate] = None,document_separator: str = DEFAULT_DOCUMENT_SEPARATOR,
) -> Runnable[Dict[str, Any], Any]:_validate_prompt(prompt)_document_prompt = document_prompt or DEFAULT_DOCUMENT_PROMPT_output_parser = output_parser or StrOutputParser()def format_docs(inputs: dict) -> str:return document_separator.join(format_document(doc, _document_prompt) for doc in inputs[DOCUMENTS_KEY])return (RunnablePassthrough.assign(**{DOCUMENTS_KEY: format_docs}).with_config(run_name="format_inputs")| prompt| llm| _output_parser).with_config(run_name="stuff_documents_chain")
从源代码看出来,就是chain
create_retrieval_chain
def create_retrieval_chain(retriever: Union[BaseRetriever, Runnable[dict, RetrieverOutput]],combine_docs_chain: Runnable[Dict[str, Any], str],
) -> Runnable:if not isinstance(retriever, BaseRetriever):retrieval_docs: Runnable[dict, RetrieverOutput] = retrieverelse:retrieval_docs = (lambda x: x["input"]) | retrieverretrieval_chain = (RunnablePassthrough.assign(context=retrieval_docs.with_config(run_name="retrieve_documents"),).assign(answer=combine_docs_chain)).with_config(run_name="retrieval_chain")return retrieval_chain
create_retrieval_chain调用过程就是先检索,然后调用combine_docs_chain
相关文章:
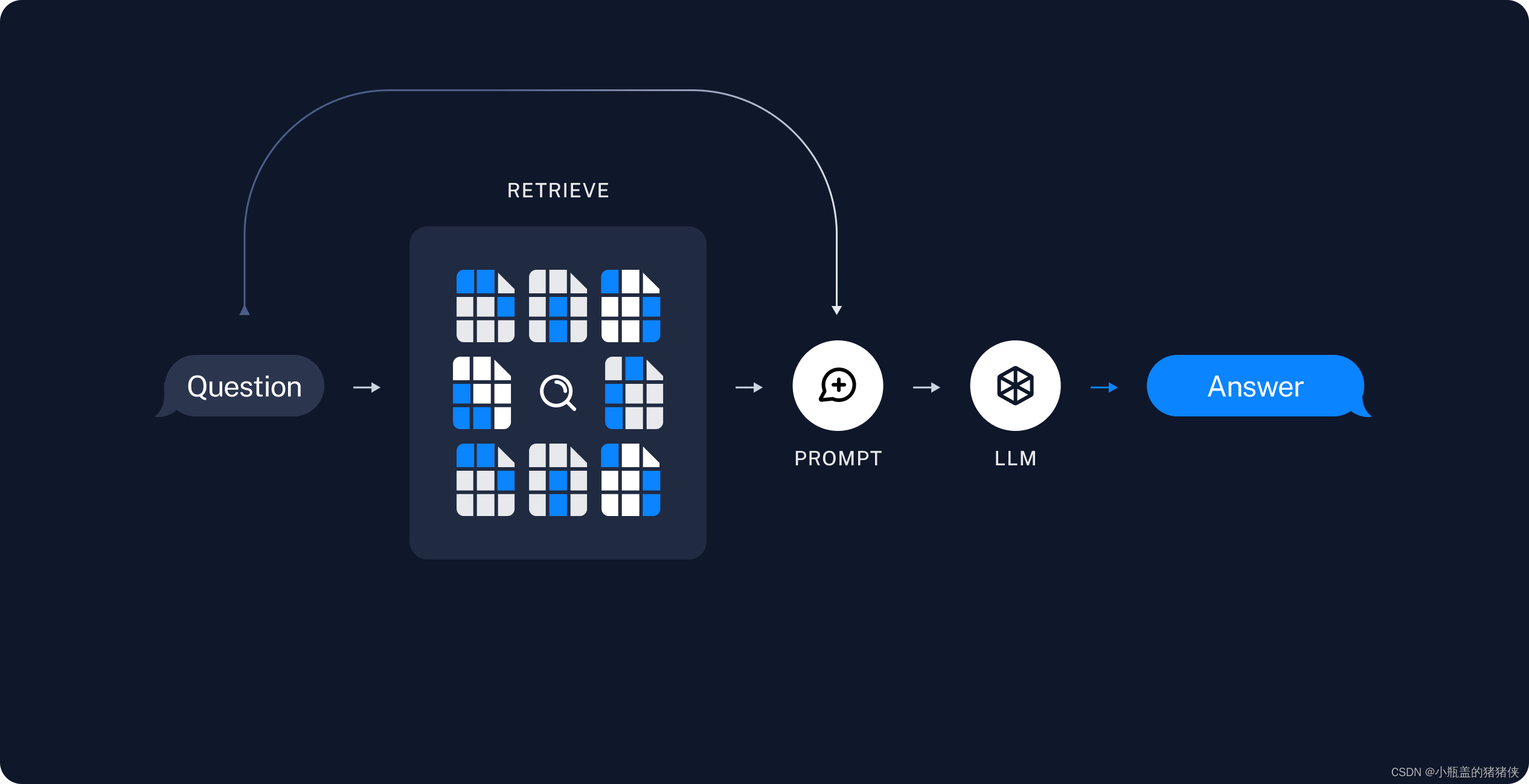
AI--构建检索增强生成 (RAG) 应用程序
LLM 所实现的最强大的应用之一是复杂的问答 (Q&A) 聊天机器人。这些应用程序可以回答有关特定源信息的问题。这些应用程序使用一种称为检索增强生成 (RAG) 的技术。 典型的 RAG 应用程序有两个主要组件 索引:从源中提取数据并对其进行索引的管道。这通常在线下…...
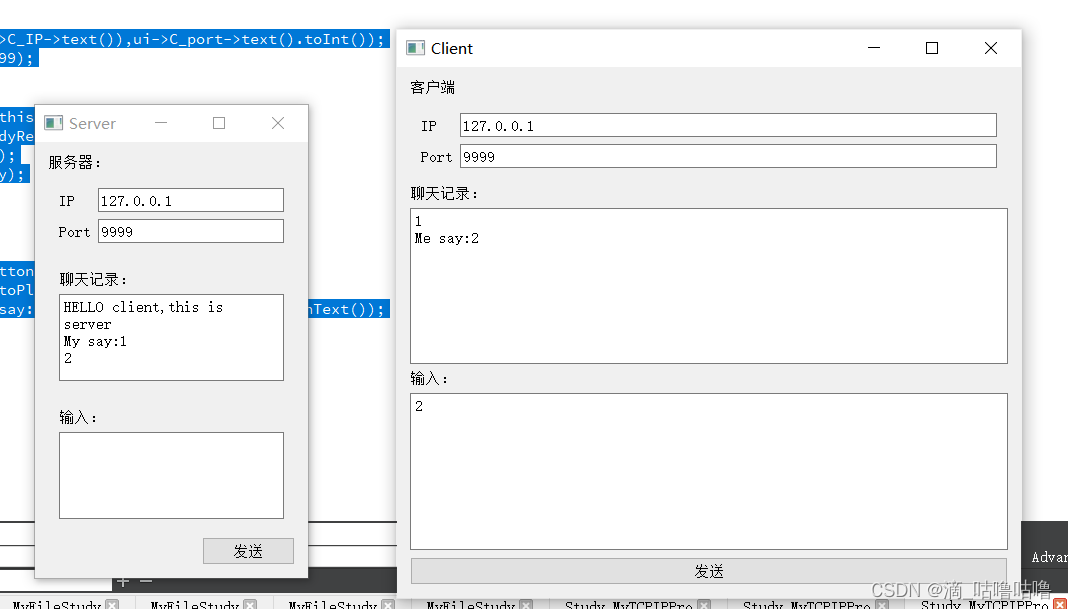
QT7_视频知识点笔记_4_文件操作,Socket通信:TCP/UDP
1.事件分发器,事件过滤器(重要程度:一般) event函数 2.文件操作(QFile) 实现功能:点击按钮,弹出对话框,并且用文件类读取出内容输出显示在控件上。 #include <QFi…...
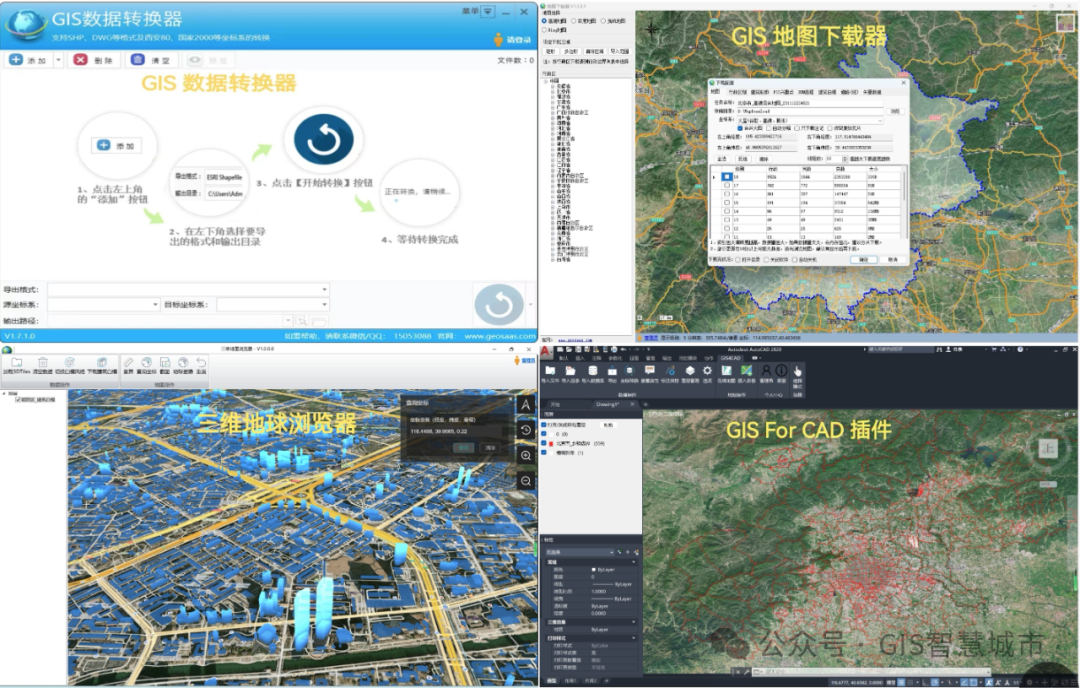
智慧社区管理系统:打造便捷、安全、和谐的新型社区生态
项目背景 在信息化、智能化浪潮席卷全球的今天,人们对于生活品质的需求日益提升,期待居住环境能与科技深度融合,实现高效、舒适、安全的生活体验。在此背景下,智慧社区管理系统应运而生,旨在借助现代信息技术手段&…...
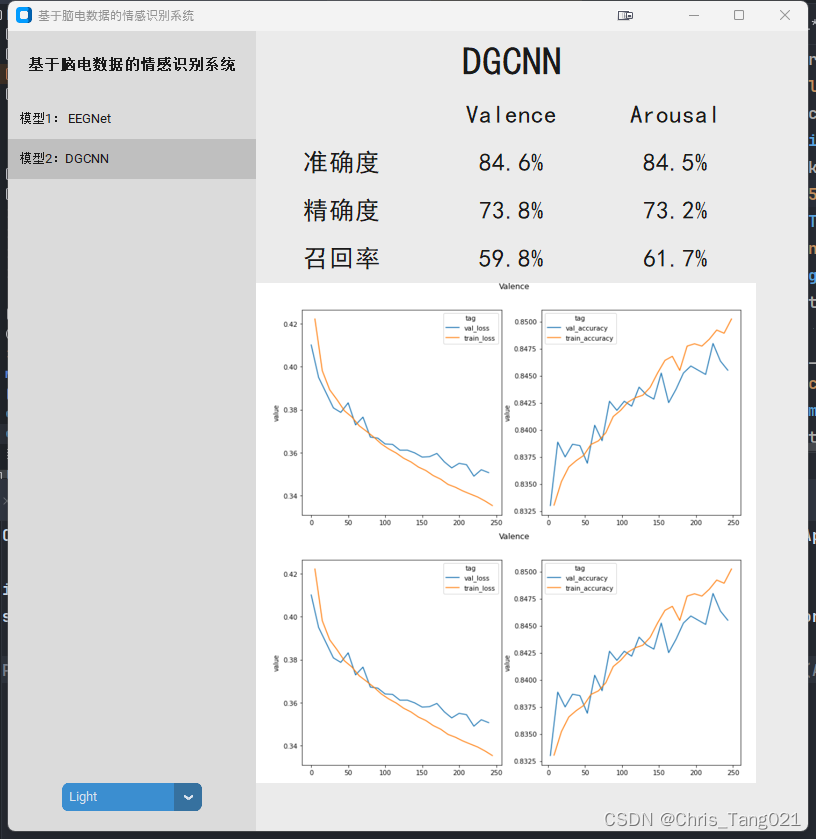
CustomTkinter:便捷美化Tkinter的UI界面(附模板)
CustomTkinter是一个基于Tkinter的Python用户界面库。 pip3 install customtkinter它提供了各种UI界面常见的小部件。这些小部件可以像正常的Tkinter小部件一样创建和使用,也可以与正常的Tkinter元素一起使用。 它的优势如下: CustomTkinter的小部件和…...
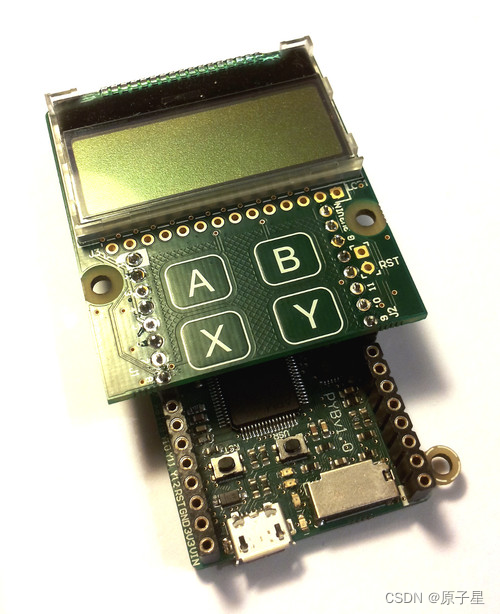
使用MicroPython和pyboard开发板(15):使用LCD和触摸传感器
使用LCD和触摸传感器 pybaord的pyb对LCD设备也进行了封装,可以使用官方的LCD显示屏。将LCD屏连接到开发板,连接后。 使用LCD 先用REPL来做个实验,在MicroPython提示符中输入以下指令。请确保LCD面板连接到pyboard的方式正确。 >>…...
c++20 std::jthread 源码简单赏析与应用
std::jthread 说明: std::jthread 是 C20 中引入的一个新特性,它是线程库中的一个类,专门用于处理 std::thread 与 std::stop_token 和 std::stop_source 之间的交互,以支持更优雅和安全的线程停止机制。 std::stop_source控制…...
自动化测试里的数据驱动和关键字驱动思路的理解
🍅 视频学习:文末有免费的配套视频可观看 🍅 点击文末小卡片,免费获取软件测试全套资料,资料在手,涨薪更快 初次接触自动化测试时,对数据驱动和关键字驱动不甚理解,觉得有点故弄玄须…...
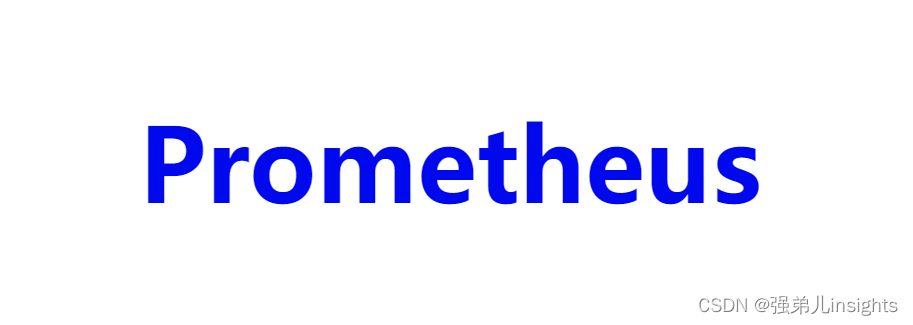
【30天精通Prometheus:一站式监控实战指南】第6天:mysqld_exporter从入门到实战:安装、配置详解与生产环境搭建指南,超详细
亲爱的读者们👋 欢迎加入【30天精通Prometheus】专栏!📚 在这里,我们将探索Prometheus的强大功能,并将其应用于实际监控中。这个专栏都将为你提供宝贵的实战经验。🚀 Prometheus是云原生和DevOps的…...
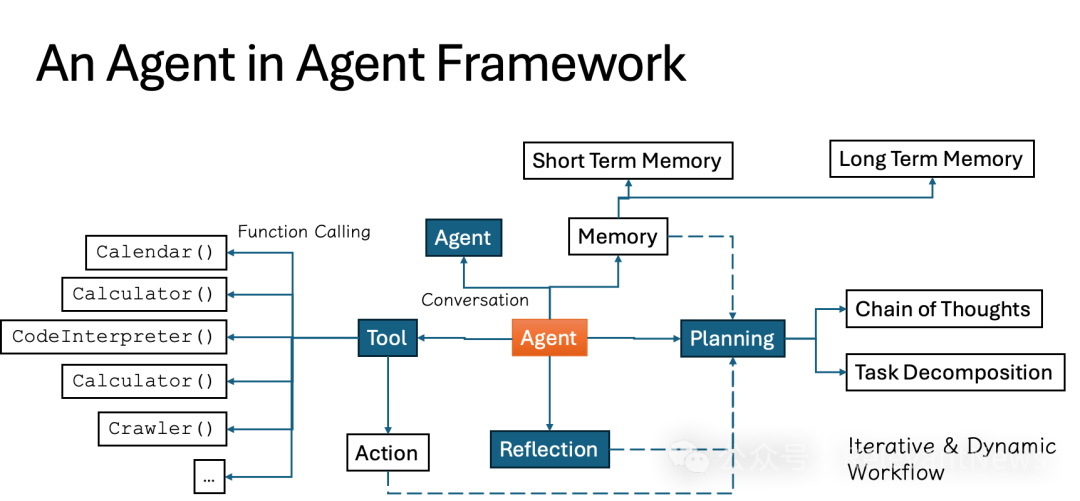
浅析智能体开发(第二部分):智能体设计模式和软件架构
大语言模型(LLM)驱动的智能体(AI Agent)展现出许多传统软件所不具备的特征。不仅与传统软件的设计理念、方法、工具和技术栈有显著的差异,AI原生(AI Native)的智能体还融入了多种新概念和技术。…...
Unity学习笔记---Transform组件
组件介绍 Transform组件在每个游戏对象中都存在,且只存在一个。该组件保存了游戏对象的位置、平移、旋转、缩放等信息。 组件相关方法 //获取当前游戏对象的Transform组件this.transform; getObject.transform; GetComponent<Transform>();//属性 gameObje…...
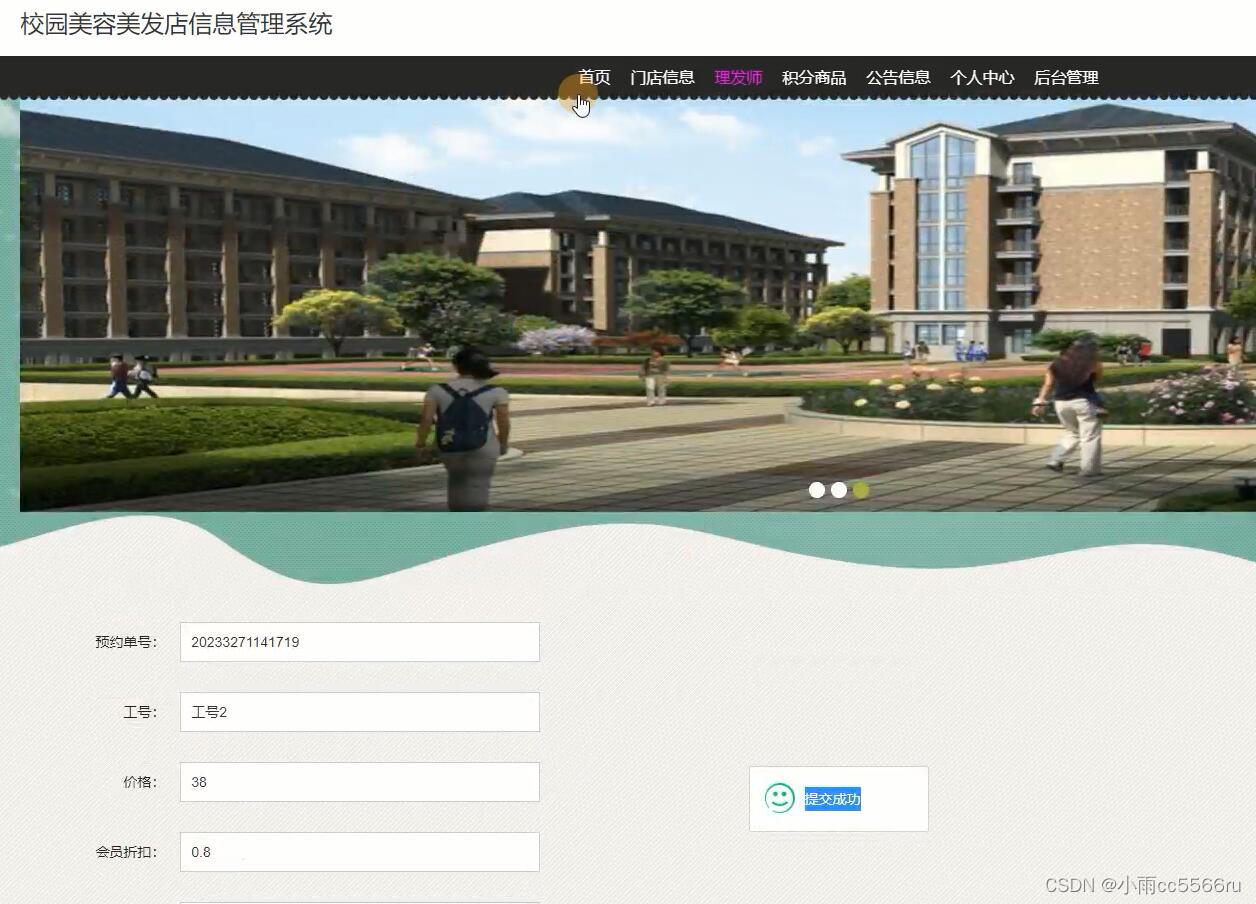
springboot+jsp校园理发店美容美发店信息管理系统0h29g
前台管理:会员管理、会员预定、开单点单、收银结帐、技师提成 后台管理:数据维护、物料管理、数据查询、报表分析、系统设置等 灵活的付款方式,支持现金、挂帐、会员卡,同时支持多种折扣方式并可按用户要求设置多种结帐类型善的充值卡管理模块:支持优惠卡…...
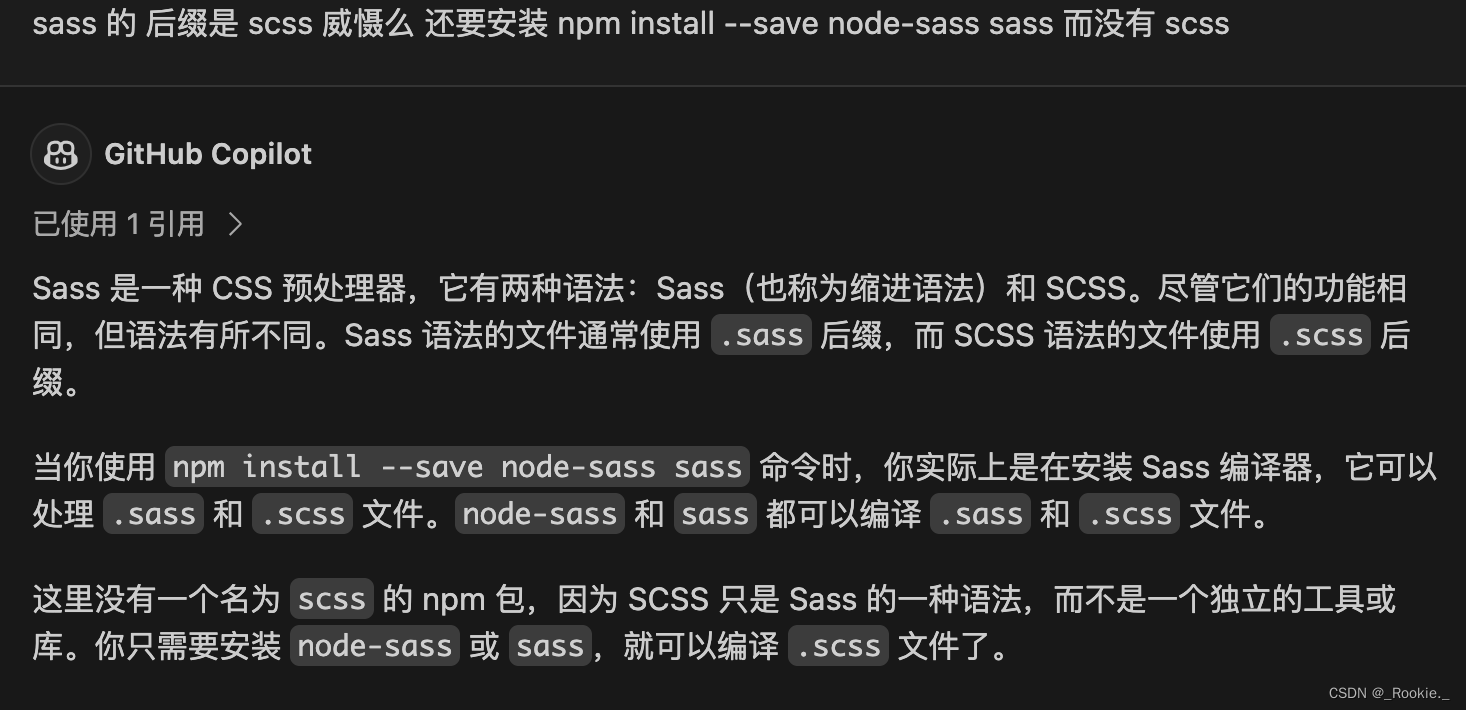
css - sass or scss ?
总的来说,Sass 和 SCSS 提供的功能是一样的,选择哪种语法主要取决于你的个人或团队的偏好。...
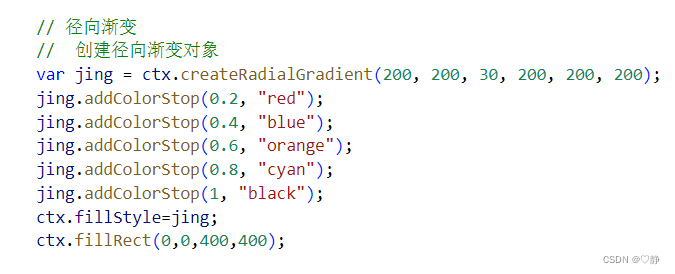
html5 笔记01
01 表单类型和属性 input的type属性 单行文本框: typetext 电子邮箱 : typeemail 地址路径 : type url 定义用于输入数字的字段: typenumber 手机号码: typetel 搜索框 : typesearch 定义颜色选择器 : typecolor 滑块控件 : typerange 定义日期 :typedate 定义输入时间的控件…...
E5063A是德科技e5063a网络分析仪
181-2461-8938产品概述: 简 述: E5063A 是低成本网络分析仪,可提供优化的性能和功能,适用于测试简单的无源器件,例如天线、电缆、滤波器和 PCB 等。它利用工业标准 ENA 系列始终如一的测量架构,能够极…...
【星海随笔】微信小程序(二)
WXML 模板语法 - 数据绑定 在data中定义页面的数据 在页面对应的 .js 文件中,把数据定义到 data 对象中即可: Page({data: {// 字符串类型的数据info: init data,// 数据类型的数据msgList: [{msg: hello},{msg: world}]} })Mustache 语法的格式 把 …...
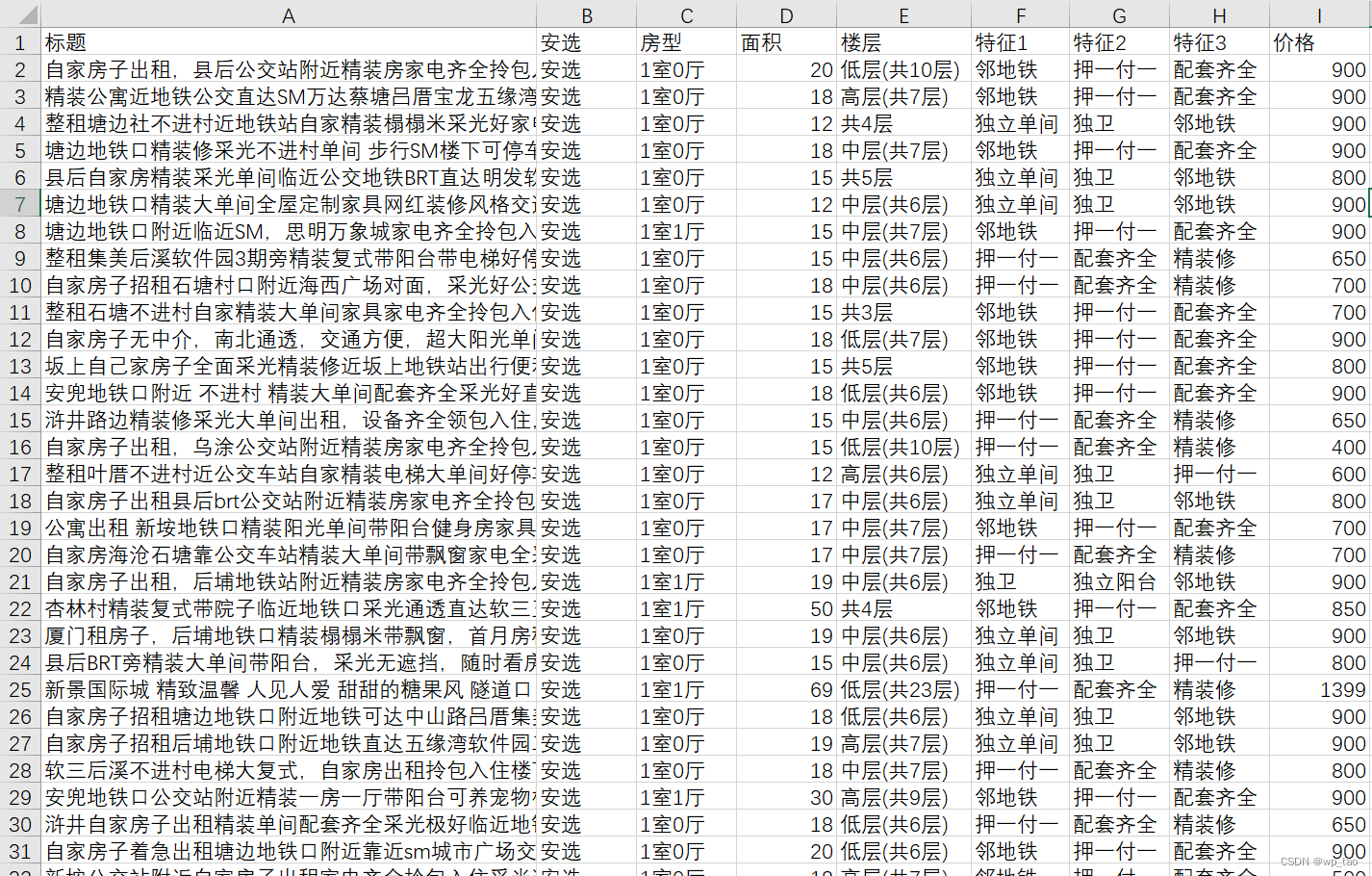
Python采集安居客租房信息
Python采集安居客租房信息 一、需求介绍二、完整代码一、需求介绍 本次采集的需求就是获取到页面中的所有信息: 将数据采集好之后保存为如下csv文件: 爬取的流程不再展开分析,完整代码附后。 二、完整代码 import csvimport requests from lxml import etreeclass Anju…...

Rust构造JSON和解析JSON
目录 一、Rust构造JSON和解析JSON 二、知识点 serde_json JSON 一、Rust构造JSON和解析JSON 添加依赖项 cargo add serde-json 代码: use serde_json::{Result, Value};fn main() -> Result<()>{//构造json结构 cpu_loadlet data r#"{"…...
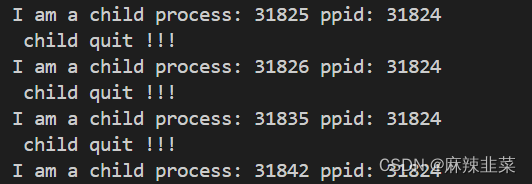
Linux 信号捕捉与处理
💓博主CSDN主页:麻辣韭菜💓 ⏩专栏分类:Linux知识分享⏪ 🚚代码仓库:Linux代码练习🚚 🌹关注我🫵带你学习更多Linux知识 🔝 目录 前言 1. 信号的处理时机 1.1用户…...
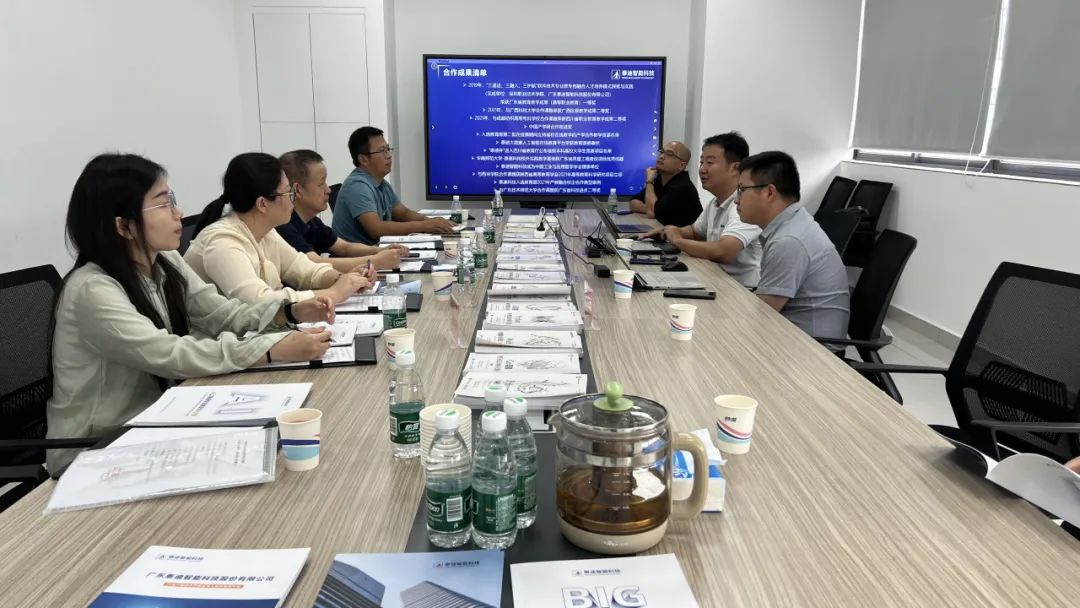
桂林电子科技大学计算机工程学院、广西北部湾大学计信学院莅临泰迪智能科技参观交流
5月18日,桂林电子科技大学计算机工程学院副院长刘利民、副书记杨美娜、毕业班辅导员黄秀娟、广西北部湾大学计信学院院长助理刘秀平莅临广东泰迪智能科技股份有限公司产教融合实训基地参观交流。泰迪智能科技副总经理施兴、广西分公司郑廷和、梁霜、培训业务部孙学镂…...
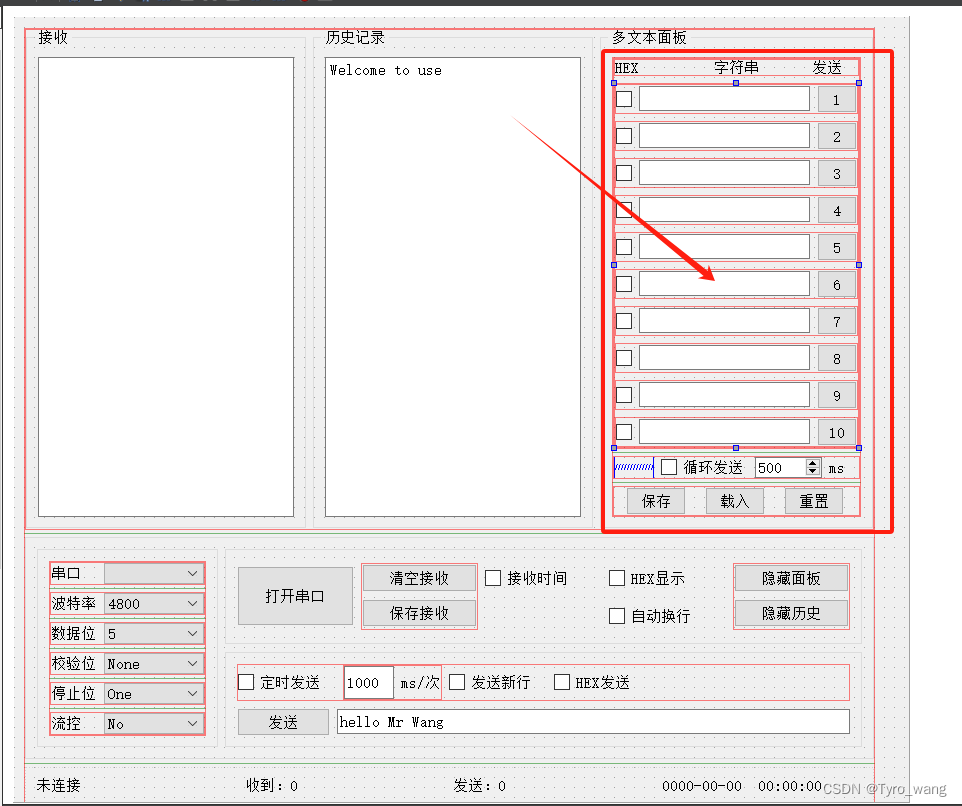
Qt笔记:动态处理多个按钮点击事件以更新UI
问题描述 在开发Qt应用程序时,经常需要处理多个按钮的点击事件,并根据点击的按钮来更新用户界面(UI),如下图。例如,你可能有一个包含多个按钮的界面,每个按钮都与一个文本框和一个复选框相关联…...
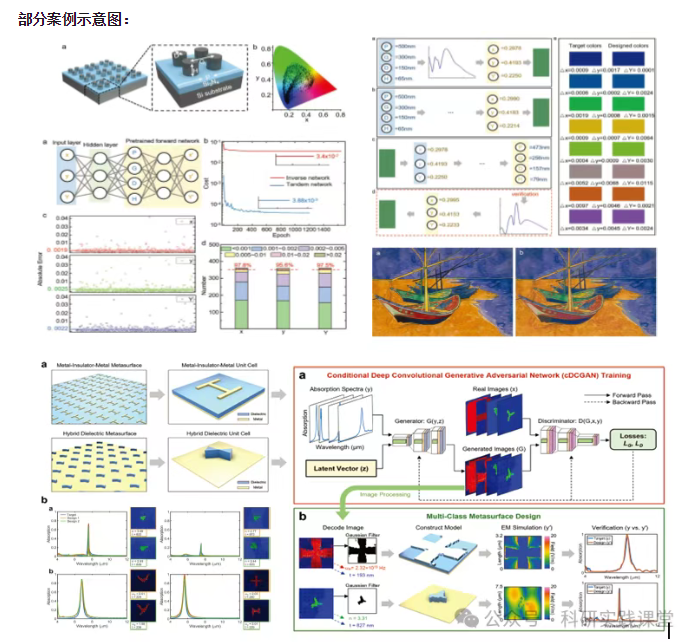
深度学习在微纳光子学中的应用
深度学习在微纳光子学中的主要应用方向 深度学习与微纳光子学的结合主要集中在以下几个方向: 逆向设计 通过神经网络快速预测微纳结构的光学响应,替代传统耗时的数值模拟方法。例如设计超表面、光子晶体等结构。 特征提取与优化 从复杂的光学数据中自…...
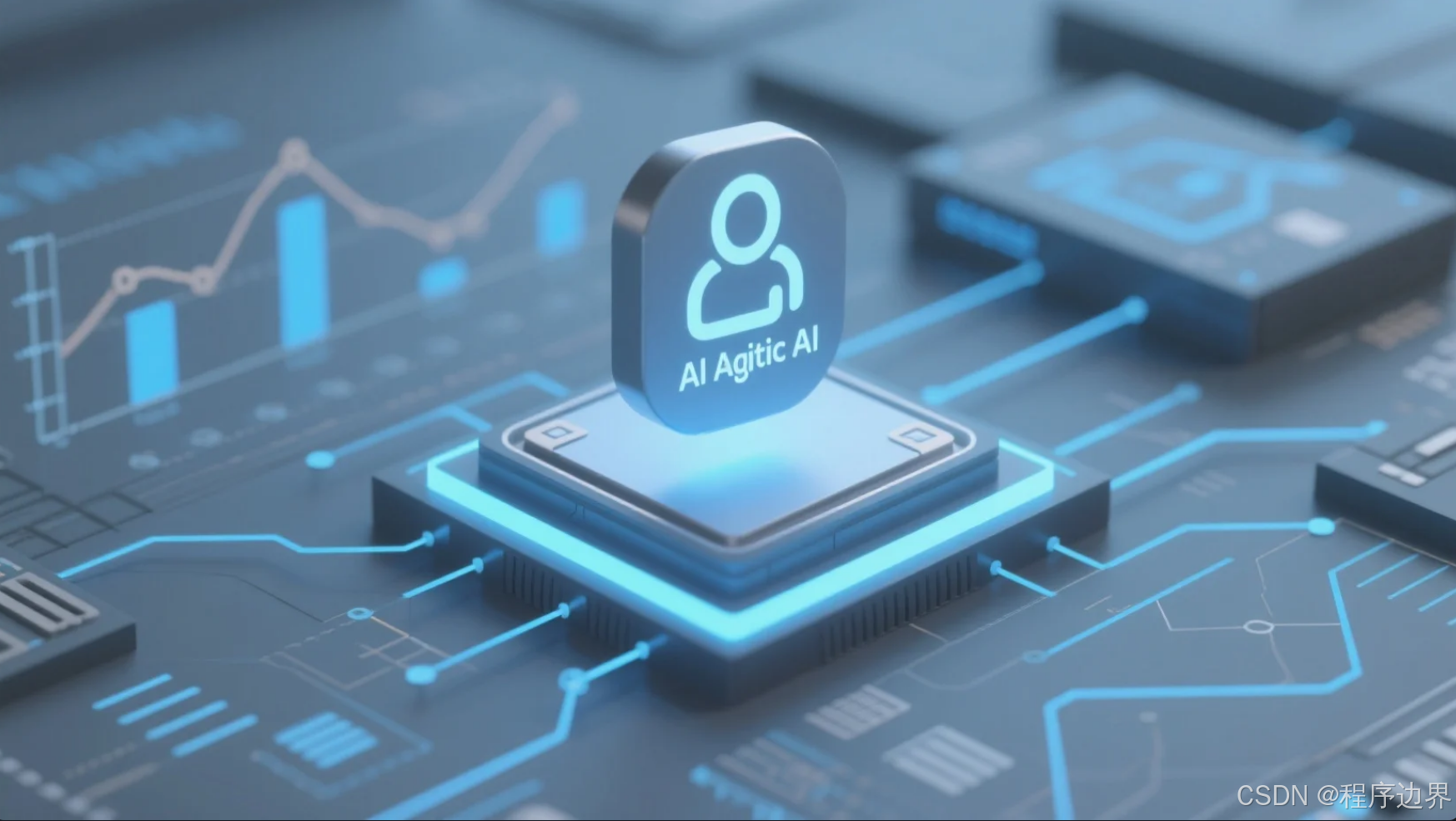
AI Agent与Agentic AI:原理、应用、挑战与未来展望
文章目录 一、引言二、AI Agent与Agentic AI的兴起2.1 技术契机与生态成熟2.2 Agent的定义与特征2.3 Agent的发展历程 三、AI Agent的核心技术栈解密3.1 感知模块代码示例:使用Python和OpenCV进行图像识别 3.2 认知与决策模块代码示例:使用OpenAI GPT-3进…...
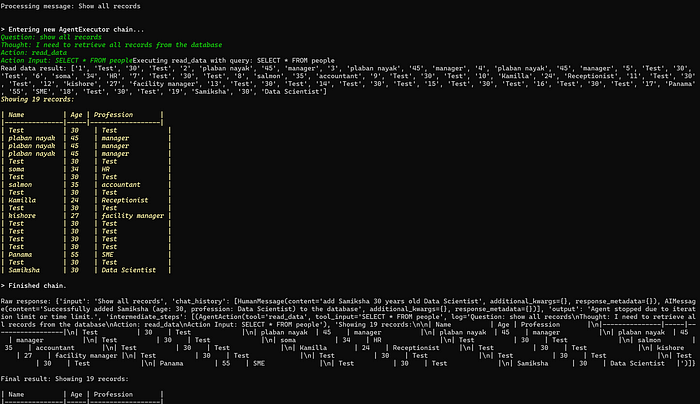
理解 MCP 工作流:使用 Ollama 和 LangChain 构建本地 MCP 客户端
🌟 什么是 MCP? 模型控制协议 (MCP) 是一种创新的协议,旨在无缝连接 AI 模型与应用程序。 MCP 是一个开源协议,它标准化了我们的 LLM 应用程序连接所需工具和数据源并与之协作的方式。 可以把它想象成你的 AI 模型 和想要使用它…...
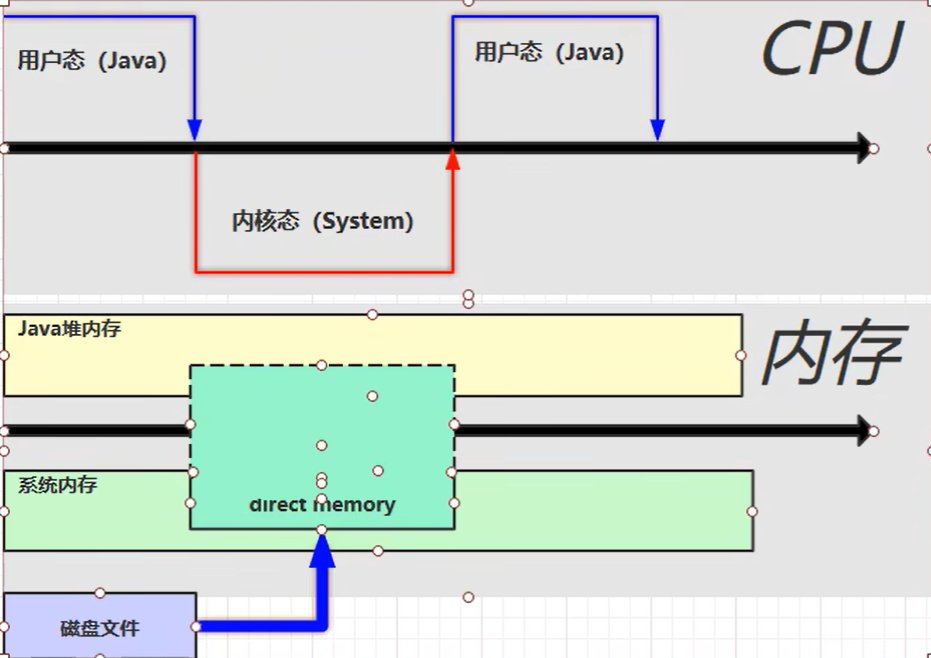
【JVM】- 内存结构
引言 JVM:Java Virtual Machine 定义:Java虚拟机,Java二进制字节码的运行环境好处: 一次编写,到处运行自动内存管理,垃圾回收的功能数组下标越界检查(会抛异常,不会覆盖到其他代码…...
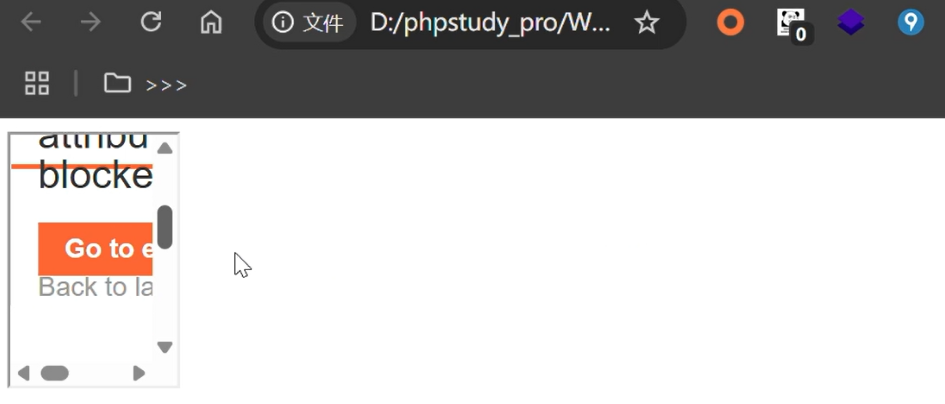
渗透实战PortSwigger靶场-XSS Lab 14:大多数标签和属性被阻止
<script>标签被拦截 我们需要把全部可用的 tag 和 event 进行暴力破解 XSS cheat sheet: https://portswigger.net/web-security/cross-site-scripting/cheat-sheet 通过爆破发现body可以用 再把全部 events 放进去爆破 这些 event 全部可用 <body onres…...
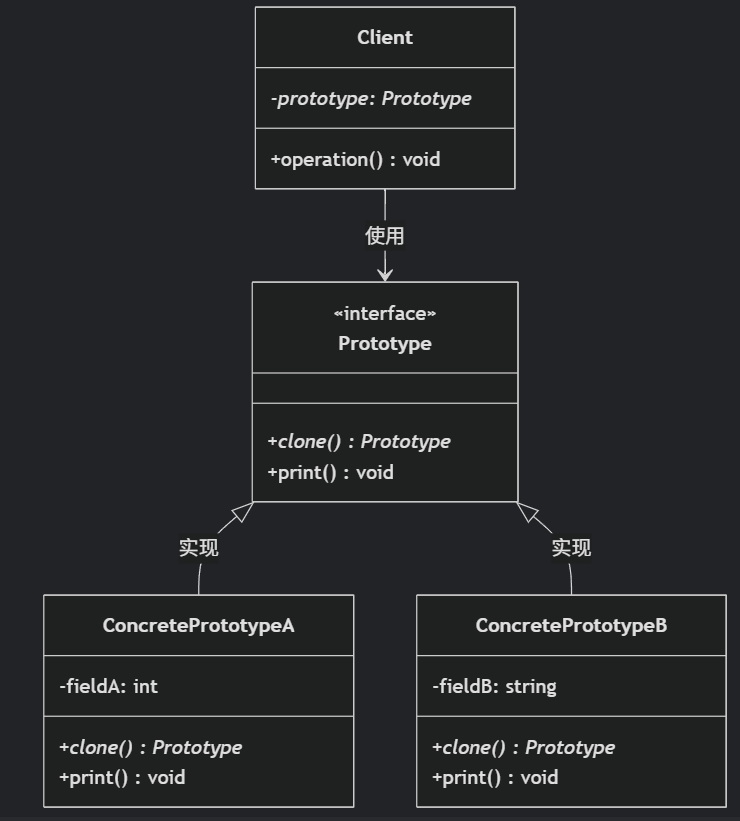
(二)原型模式
原型的功能是将一个已经存在的对象作为源目标,其余对象都是通过这个源目标创建。发挥复制的作用就是原型模式的核心思想。 一、源型模式的定义 原型模式是指第二次创建对象可以通过复制已经存在的原型对象来实现,忽略对象创建过程中的其它细节。 📌 核心特点: 避免重复初…...
python爬虫:Newspaper3k 的详细使用(好用的新闻网站文章抓取和解析的Python库)
更多内容请见: 爬虫和逆向教程-专栏介绍和目录 文章目录 一、Newspaper3k 概述1.1 Newspaper3k 介绍1.2 主要功能1.3 典型应用场景1.4 安装二、基本用法2.2 提取单篇文章的内容2.2 处理多篇文档三、高级选项3.1 自定义配置3.2 分析文章情感四、实战案例4.1 构建新闻摘要聚合器…...
【C语言练习】080. 使用C语言实现简单的数据库操作
080. 使用C语言实现简单的数据库操作 080. 使用C语言实现简单的数据库操作使用原生APIODBC接口第三方库ORM框架文件模拟1. 安装SQLite2. 示例代码:使用SQLite创建数据库、表和插入数据3. 编译和运行4. 示例运行输出:5. 注意事项6. 总结080. 使用C语言实现简单的数据库操作 在…...
【C++从零实现Json-Rpc框架】第六弹 —— 服务端模块划分
一、项目背景回顾 前五弹完成了Json-Rpc协议解析、请求处理、客户端调用等基础模块搭建。 本弹重点聚焦于服务端的模块划分与架构设计,提升代码结构的可维护性与扩展性。 二、服务端模块设计目标 高内聚低耦合:各模块职责清晰,便于独立开发…...
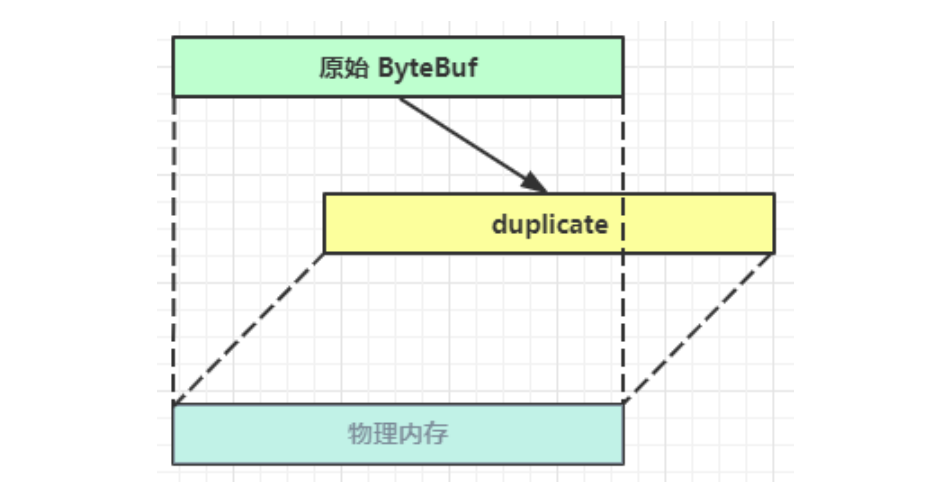
Netty从入门到进阶(二)
二、Netty入门 1. 概述 1.1 Netty是什么 Netty is an asynchronous event-driven network application framework for rapid development of maintainable high performance protocol servers & clients. Netty是一个异步的、基于事件驱动的网络应用框架,用于…...