应用最优化方法及MATLAB实现——第3章代码实现
一、概述
在阅读最优方法及MATLAB实现后,想着将书中提供的代码自己手敲一遍,来提高自己对书中内容理解程度,巩固一下。
这部分内容主要针对第3章的内容,将其所有代码实现均手敲一遍,中间部分代码自己根据其公式有些许的调整,但最后运算结果与书中所给结果一致。
修改的部分我会在下面进行单独说明并标注上我这样做的原因。
二、具体代码
(一)计算函数文件
因为书中所给出的示例代码,所使用的函数都是一样的。所以将其单独罗列出来。
其中,f_test1.m如下所示。
function y = f_test1(x)
% 该函数的主要作用是编写函数,用于计算函数输出值
% x为函数输入值
% y为函数输出值y = 2 * x ^2 - x - 1;
end
f_test2.m如下所示。
function y = f_test2(x)
% 该函数的主要作用是编写函数,用于计算函数输出值
% x为函数输入值
% y为函数输出值x1 = x(1);x2 = x(2);y = x1 ^2 + x2 ^2 - 1;
end
f_test3.m如下所示。
function y = f_test3(x)
% 该函数的主要作用是编写函数,用于计算函数输出值
% x为函数输入值
% y为函数输出值if(x <= 2)y = -x + 3;elsey = x / 2;end
end
这些文件每个方法使用的都是一样的。
(二)对分搜索法
1.对分搜索法函数文件
这个文件主要参照书中的,没有改动。
function [alpha_star, x_next, f_next, k] = Dichotomous_search(f_test, x_current, d_current, alpha_lower, alpha_upper, tolerance)
% 该函数针对对分搜索法的实现
% 输入参数说明
% f_test, 目标函数
% x_current, x在向量空间中的当前点(已确定)
% d_current, f_test在向量空间中的搜索方向(已确定)
% alpha_lower, 从x_current出发,沿着d_current得到一个步长的单谷区间,该单谷区间的初始下届
% alpha_upper, 从x_current出发,沿着d_current得到一个步长的单谷区间,该单谷区间的初始上届
% tolerance, 最终区间的要求宽度,精度不能小于扰动量% 输出参数说明
% alpha_star, 完成对分搜索后输出的步长
% x_next, x_next = x_current + alpha_star * d_current;局部最优点
% f_next, 局部最优点对应的函数值
% k,迭代次数disturbance_quantity = 0;if(tolerance >= 1e-8)disturbance_quantity = 1e-9;elsedisturbance_quantity = 0.1 * tolerance;end% 初始值k = 0;alpha_lower_k = alpha_lower;alpha_upper_k = alpha_upper;alpha_left_k = (1/2)*(alpha_lower_k + alpha_upper_k) - disturbance_quantity;alpha_right_k = (1/2)*(alpha_lower_k + alpha_upper_k) + disturbance_quantity;x_alpha_left_k = x_current + alpha_left_k * d_current;x_alpha_right_k = x_current + alpha_right_k * d_current;f_alpha_left_k = f_test(x_alpha_left_k);f_alpha_right_k = f_test(x_alpha_right_k);% 进入循环while(abs(alpha_upper_k - alpha_lower_k) > tolerance)if(f_alpha_right_k > f_alpha_left_k)alpha_upper_k = alpha_right_k;elseif(f_alpha_right_k < f_alpha_left_k)alpha_lower_k = alpha_left_k;elsealpha_upper_k = alpha_right_k;alpha_lower_k = alpha_left_k;endalpha_left_k = (1/2)*(alpha_lower_k + alpha_upper_k) - disturbance_quantity;alpha_right_k = (1/2)*(alpha_lower_k + alpha_upper_k) + disturbance_quantity;x_alpha_left_k = x_current + alpha_left_k * d_current;x_alpha_right_k = x_current + alpha_right_k * d_current;f_alpha_left_k = f_test(x_alpha_left_k);f_alpha_right_k = f_test(x_alpha_right_k);k = k + 1;endalpha_star = (alpha_right_k + alpha_left_k) / 2;x_next = x_current + alpha_star * d_current;f_next = f_test(x_next);
end
2.对应的主运行文件
放开相应的注释即可。
% 这个文件作为第3章的主文件% 清空所有
close;
clear;
clc;% 对分搜索法实现
% 第一个示例
% x_current = -0.5;
% d_current = 1;
% alpha_lower = 0;
% alpha_upper = 1;
% tolerance = 1e-4;
% [alpha_star, x_next, f_next, k] = Dichotomous_search(@f_test1, x_current, d_current, alpha_lower, alpha_upper, tolerance);% 第二个示例
% x_current = [2, 2];
% d_current = [-1, -1];
% alpha_lower = 0;
% alpha_upper = 2;
% tolerance = 1e-6;
% [alpha_star, x_next, f_next, k] = Dichotomous_search(@f_test2, x_current, d_current, alpha_lower, alpha_upper, tolerance);% 第三个示例
x_current = 3;
d_current = -1;
alpha_lower = 0;
alpha_upper = 2;
tolerance = 1e-4;
[alpha_star, x_next, f_next, k] = Dichotomous_search(@f_test3, x_current, d_current, alpha_lower, alpha_upper, tolerance);
(三)三点等间隔搜索法
1.三点等间隔搜索法
function [alpha_star, x_next, f_next, k] = Trisection_search(f_test, x_current, d_current, alpha_lower, alpha_upper, tolerance)
% 该函数针对三点等间隔的实现
% 输入参数说明
% f_test, 目标函数
% x_current, x在向量空间中的当前点(已确定)
% d_current, f_test在向量空间中的搜索方向(已确定)
% alpha_lower, 从x_current出发,沿着d_current得到一个步长的单谷区间,该单谷区间的初始下届
% alpha_upper, 从x_current出发,沿着d_current得到一个步长的单谷区间,该单谷区间的初始上届
% tolerance, 最终区间的要求宽度,精度不能小于扰动量% 输出参数说明
% alpha_star, 完成对分搜索后输出的步长
% x_next, x_next = x_current + alpha_star * d_current;局部最优点
% f_next, 局部最优点对应的函数值
% k,迭代次数% 初始化
k = 0;
% 这两个是每次迭代过程中的上下界
alpha_lower_k = alpha_lower;
alpha_upper_k = alpha_upper;alpha_left_k = alpha_lower_k + (1/4) * (alpha_upper_k - alpha_lower_k);
alpha_middle_k = alpha_lower_k + (1/2) * (alpha_upper_k - alpha_lower_k);
alpha_right_k = alpha_lower_k + (3/4) * (alpha_upper_k - alpha_lower_k);x_alpha_left_k = x_current + alpha_left_k * d_current;
x_alpha_middle_k = x_current + alpha_middle_k * d_current;
x_alpha_right_k = x_current + alpha_right_k * d_current;f_alpha_left_k = f_test(x_alpha_left_k);
f_alpha_middle_k = f_test(x_alpha_middle_k);
f_alpha_right_k = f_test(x_alpha_right_k);% 这部分代码书写是一种计算方法,不太精准,部分信息没有使用到
% while abs(alpha_upper_k -alpha_lower_k) > tolerance
% if (f_alpha_left_k < f_alpha_middle_k) && (f_alpha_left_k < f_alpha_right_k)
% % alpha_lower_k = alpha_lower;
% alpha_upper_k = alpha_middle_k;
% elseif (f_alpha_middle_k < f_alpha_left_k) && (f_alpha_middle_k < f_alpha_right_k)
% alpha_lower_k = alpha_left_k;
% alpha_upper_k = alpha_right_k;
% else
% alpha_lower_k = alpha_middle_k;
% % alpha_upper_k = alpha_upper
% end
% k = k + 1;
% alpha_left_k = alpha_lower_k + (1/4) * (alpha_upper_k - alpha_lower_k);
% alpha_middle_k = alpha_lower_k + (1/2) * (alpha_upper_k - alpha_lower_k);
% alpha_right_k = alpha_lower_k + (3/4) * (alpha_upper_k - alpha_lower_k);
%
% x_alpha_left_k = x_current + alpha_left_k * d_current;
% x_alpha_middle_k = x_current + alpha_middle_k * d_current;
% x_alpha_right_k = x_current + alpha_right_k * d_current;
%
% f_alpha_left_k = f_test(x_alpha_left_k);
% f_alpha_middle_k = f_test(x_alpha_middle_k);
% f_alpha_right_k = f_test(x_alpha_right_k);
% end% 第二种方法,可以重复利用三点等间隔搜索法的信息
while abs(alpha_upper_k -alpha_lower_k) > toleranceif (f_alpha_left_k < f_alpha_middle_k) && (f_alpha_left_k < f_alpha_right_k)% alpha_lower_k = alpha_lower;alpha_upper_k = alpha_middle_k;alpha_left_k = alpha_lower_k + (1/4) * (alpha_upper_k - alpha_lower_k);alpha_middle_k = alpha_left_k;alpha_right_k = alpha_lower_k + (3/4) * (alpha_upper_k - alpha_lower_k);x_alpha_left_k = x_current + alpha_left_k * d_current;x_alpha_middle_k = x_current + alpha_middle_k * d_current;x_alpha_right_k = x_current + alpha_right_k * d_current;f_alpha_left_k = f_test(x_alpha_left_k);f_alpha_middle_k = f_alpha_left_k;f_alpha_right_k = f_test(x_alpha_right_k);elseif (f_alpha_middle_k < f_alpha_left_k) && (f_alpha_middle_k < f_alpha_right_k)alpha_lower_k = alpha_left_k;alpha_upper_k = alpha_right_k;alpha_left_k = alpha_lower_k + (1/4) * (alpha_upper_k - alpha_lower_k);% alpha_middle_k = alpha_left_k;alpha_right_k = alpha_lower_k + (3/4) * (alpha_upper_k - alpha_lower_k);x_alpha_left_k = x_current + alpha_left_k * d_current;% x_alpha_middle_k = x_current + alpha_middle_k * d_current;x_alpha_right_k = x_current + alpha_right_k * d_current;f_alpha_left_k = f_test(x_alpha_left_k);% f_alpha_middle_k = f_alpha_left_k;f_alpha_right_k = f_test(x_alpha_right_k);else alpha_lower_k = alpha_middle_k;% alpha_upper_k = alpha_upperalpha_left_k = alpha_lower_k + (1/4) * (alpha_upper_k - alpha_lower_k);alpha_middle_k = alpha_right_k;alpha_right_k = alpha_lower_k + (3/4) * (alpha_upper_k - alpha_lower_k);x_alpha_left_k = x_current + alpha_left_k * d_current;x_alpha_middle_k = x_current + alpha_middle_k * d_current;x_alpha_right_k = x_current + alpha_right_k * d_current;f_alpha_left_k = f_test(x_alpha_left_k);f_alpha_middle_k = f_alpha_right_k;f_alpha_right_k = f_test(x_alpha_right_k);end
k = k + 1;
endalpha_star = (alpha_left_k + alpha_right_k) / 2;
x_next = x_current + alpha_star * d_current;
f_next = f_test(x_next);
end
2.主函数运行文件
放开相应注释即可。
% 这个是三点等间隔搜索法的主程序% 清空变量
close;
clear;
clc;% 示例一
% x_current = -0.5;
% d_current = 1;
% alpha_lower = 0;
% alpha_upper = 1;
% tolerance = 1e-4;
% [alpha_star, x_next, f_next, k] = Trisection_search(@f_test1, x_current, d_current, alpha_lower, alpha_upper, tolerance);% 示例二
% x_current = [2, 2];
% d_current = [-1, -1];
% alpha_lower = 0;
% alpha_upper = 2;
% tolerance = 1e-6;
% [alpha_star, x_next, f_next, k] = Trisection_search(@f_test2, x_current, d_current, alpha_lower, alpha_upper, tolerance);% 示例三
x_current = 3;
d_current = -1;
alpha_lower = 0;
alpha_upper = 2;
tolerance = 1e-4;
[alpha_star, x_next, f_next, k] = Trisection_search(@f_test3, x_current, d_current, alpha_lower, alpha_upper, tolerance);
3.一些说明
在三点搜索法的实现过程中,目前放开注释的是比较符合书中思路的,在前面有一部分注释掉的实现方式,如图所示。
使用这一部分代码时候,也是可以运行成功的,但是计算效率不高,因为三点搜索法的一些条件没有利用到,重新计算函数值这些会浪费一定时间,但是代码简单,比较好理解,也好书写。
(四) Fibonacci搜索法
1.Fibonacci搜索法函数文件
function [alpha_star, x_next, f_next, k] = Fibonacci_search(f_test, x_current, d_current, alpha_lower, alpha_upper, tolerance)
% 该函数针对Fibonacci搜索法的实现
% 输入参数说明
% f_test, 目标函数
% x_current, x在向量空间中的当前点(已确定)
% d_current, f_test在向量空间中的搜索方向(已确定)
% alpha_lower, 从x_current出发,沿着d_current得到一个步长的单谷区间,该单谷区间的初始下届
% alpha_upper, 从x_current出发,沿着d_current得到一个步长的单谷区间,该单谷区间的初始上届
% tolerance, 最终区间的要求宽度,精度不能小于扰动量% 输出参数说明
% alpha_star, 完成对分搜索后输出的步长
% x_next, x_next = x_current + alpha_star * d_current;局部最优点
% f_next, 局部最优点对应的函数值
% k,迭代次数% 计算斐波那契数列数列需要多少个
Fibonacci_series_upper = (alpha_upper - alpha_lower) / tolerance;
% 这里不需要第1个,实际上是从第二个开始
Fibonacci_series = [1, 2];
n = 2;
while (Fibonacci_series(n) <= Fibonacci_series_upper)n = n + 1;Fibonacci_series(n) = Fibonacci_series(n-1) + Fibonacci_series(n-2);
end% 使用斐波那契数列进行搜索,注意到第n-2位置
k = 0;
alpha_lower_k = alpha_lower;
alpha_upper_k = alpha_upper;
Length_k = (Fibonacci_series(n - 1) / Fibonacci_series(n)) * (alpha_upper_k - alpha_lower_k);
alpha_left_k = alpha_upper_k - Length_k;
alpha_right_k = alpha_lower_k + Length_k;
x_alpha_left_k = x_current + alpha_left_k * d_current;
x_alpha_right_k = x_current + alpha_right_k * d_current;
f_alpha_left_k = f_test(x_alpha_left_k);
f_alpha_right_k = f_test(x_alpha_right_k);
while k < n - 2k = k + 1;if(f_alpha_left_k < f_alpha_right_k)% alpha_lower_k = alpha_lower;alpha_upper_k = alpha_right_k;Length_k = (Fibonacci_series(n - k - 1) / Fibonacci_series(n - k)) * (alpha_upper_k - alpha_lower_k);alpha_right_k = alpha_left_k;alpha_left_k = alpha_upper_k - Length_k;% alpha_right_k = alpha_lower_k + Length_k; x_alpha_left_k = x_current + alpha_left_k * d_current;x_alpha_right_k = x_current + alpha_right_k * d_current;f_alpha_left_k = f_test(x_alpha_left_k);f_alpha_right_k = f_test(x_alpha_right_k);elsealpha_lower_k = alpha_left_k;% alpha_upper_k = alpha_right_k;Length_k = (Fibonacci_series(n - k - 1) / Fibonacci_series(n - k)) * (alpha_upper_k - alpha_lower_k);alpha_left_k = alpha_right_k;% alpha_left_k = alpha_upper_k - Length_k;alpha_right_k = alpha_lower_k + Length_k; x_alpha_left_k = x_current + alpha_left_k * d_current;x_alpha_right_k = x_current + alpha_right_k * d_current;f_alpha_left_k = f_test(x_alpha_left_k);f_alpha_right_k = f_test(x_alpha_right_k);end
endk = k + 1;
disturbance_quantity = 0.1 * tolerance;
alpha_left_k = (1/2)*(alpha_lower_k + alpha_upper_k) - disturbance_quantity;
alpha_right_k = (1/2)*(alpha_lower_k + alpha_upper_k) + disturbance_quantity;
x_alpha_left_k = x_current + alpha_left_k * d_current;
x_alpha_right_k = x_current + alpha_right_k * d_current;
f_alpha_left_k = f_test(x_alpha_left_k);
f_alpha_right_k = f_test(x_alpha_right_k);if (f_alpha_left_k < f_alpha_right_k)alpha_upper_k = alpha_right_k;
elseif (f_alpha_left_k > f_alpha_right_k)alpha_lower_k = alpha_left_k;
elsealpha_upper_k = alpha_right_k;alpha_lower_k = alpha_left_k;
endalpha_star = (alpha_lower_k + alpha_upper_k) / 2;
x_next = x_current + alpha_star * d_current;
f_next = f_test(x_next);
end
2.主函数运行文件
放开相应注释即可。
% 这个文件主要用来进行斐波那契搜索法的运行close;
clear;
clc;% 示例一
% x_current = -0.5;
% d_current = 1;
% alpha_lower = 0;
% alpha_upper = 1;
% tolerance = 1e-4;
% [alpha_star, x_next, f_next, k] = Fibonacci_search(@f_test1, x_current, d_current, alpha_lower, alpha_upper, tolerance);% 示例二
x_current = [2, 2];
d_current = [-1, -1];
alpha_lower = 0;
alpha_upper = 2;
tolerance = 1e-6;
[alpha_star, x_next, f_next, k] = Fibonacci_search(@f_test2, x_current, d_current, alpha_lower, alpha_upper, tolerance);% 示例三
% x_current = 3;
% d_current = -1;
% alpha_lower = 0;
% alpha_upper = 2;
% tolerance = 1e-4;
% [alpha_star, x_next, f_next, k] = Fibonacci_search(@f_test3, x_current, d_current, alpha_lower, alpha_upper, tolerance);
(五)黄金分割法
1.黄金分割法函数文件
function [alpha_star, x_next, f_next, k] = Golden_section_search(f_test, x_current, d_current, alpha_lower, alpha_upper, tolerance)
% 该函数针对黄金分割法的实现
% 输入参数说明
% f_test, 目标函数
% x_current, x在向量空间中的当前点(已确定)
% d_current, f_test在向量空间中的搜索方向(已确定)
% alpha_lower, 从x_current出发,沿着d_current得到一个步长的单谷区间,该单谷区间的初始下届
% alpha_upper, 从x_current出发,沿着d_current得到一个步长的单谷区间,该单谷区间的初始上届
% tolerance, 最终区间的要求宽度,精度不能小于扰动量% 输出参数说明
% alpha_star, 完成对分搜索后输出的步长
% x_next, x_next = x_current + alpha_star * d_current;局部最优点
% f_next, 局部最优点对应的函数值
% k,迭代次数% 黄金分割点
golden_ratio = 1 / (0.5 * (1 + sqrt(5)));% 使用斐波那契数列进行搜索,注意到第n-2位置
k = 0;
alpha_lower_k = alpha_lower;
alpha_upper_k = alpha_upper;
Length_k = golden_ratio * (alpha_upper_k - alpha_lower_k);
alpha_left_k = alpha_upper_k - Length_k;
alpha_right_k = alpha_lower_k + Length_k;
x_alpha_left_k = x_current + alpha_left_k * d_current;
x_alpha_right_k = x_current + alpha_right_k * d_current;
f_alpha_left_k = f_test(x_alpha_left_k);
f_alpha_right_k = f_test(x_alpha_right_k);
while abs(alpha_upper_k - alpha_lower_k) > tolerancek = k + 1;if(f_alpha_left_k < f_alpha_right_k)% alpha_lower_k = alpha_lower;alpha_upper_k = alpha_right_k;Length_k = golden_ratio * (alpha_upper_k - alpha_lower_k);alpha_right_k = alpha_left_k;alpha_left_k = alpha_upper_k - Length_k;% alpha_right_k = alpha_lower_k + Length_k; x_alpha_left_k = x_current + alpha_left_k * d_current;x_alpha_right_k = x_current + alpha_right_k * d_current;f_alpha_left_k = f_test(x_alpha_left_k);f_alpha_right_k = f_test(x_alpha_right_k);elsealpha_lower_k = alpha_left_k;% alpha_upper_k = alpha_right_k;Length_k = golden_ratio * (alpha_upper_k - alpha_lower_k);alpha_left_k = alpha_right_k;% alpha_left_k = alpha_upper_k - Length_k;alpha_right_k = alpha_lower_k + Length_k; x_alpha_left_k = x_current + alpha_left_k * d_current;x_alpha_right_k = x_current + alpha_right_k * d_current;f_alpha_left_k = f_test(x_alpha_left_k);f_alpha_right_k = f_test(x_alpha_right_k);end
endalpha_star = (alpha_lower_k + alpha_upper_k) / 2;
x_next = x_current + alpha_star * d_current;
f_next = f_test(x_next);
end
2.主函数运行文件
% 这个文件主要用来进行黄金分割法的运行close;
clear;
clc;% 示例一
% x_current = -0.5;
% d_current = 1;
% alpha_lower = 0;
% alpha_upper = 1;
% tolerance = 1e-4;
% [alpha_star, x_next, f_next, k] = Golden_section_search(@f_test1, x_current, d_current, alpha_lower, alpha_upper, tolerance);% 示例二
x_current = [2, 2];
d_current = [-1, -1];
alpha_lower = 0;
alpha_upper = 2;
tolerance = 1e-6;
[alpha_star, x_next, f_next, k] = Golden_section_search(@f_test2, x_current, d_current, alpha_lower, alpha_upper, tolerance);% 示例三
% x_current = 3;
% d_current = -1;
% alpha_lower = 0;
% alpha_upper = 2;
% tolerance = 1e-4;
% [alpha_star, x_next, f_next, k] = Golden_section_search(@f_test3, x_current, d_current, alpha_lower, alpha_upper, tolerance);
3.一些说明
这里我稍微更改了一下实现方式,主要是针对于更新后的边界。书中原有方法如下所示,由于黄金分割法跟Fibonacci搜索法基本原理相似,所以,我就采用跟Fibonacci搜索法相似的更新方法。
更新方法如下所示。写的比较粗糙,但原理一致。
(六)三点二次插值法
1.三点二次插值法
function [alpha_star, x_next, f_next, k] = Quadratic3points_search(f_test, x_current, d_current, alpha_lower, alpha_upper, tolerance)
% 该函数针对三点二次插值法的实现
% 输入参数说明
% f_test, 目标函数
% x_current, x在向量空间中的当前点(已确定)
% d_current, f_test在向量空间中的搜索方向(已确定)
% alpha_lower, 从x_current出发,沿着d_current得到一个步长的单谷区间,该单谷区间的初始下届
% alpha_upper, 从x_current出发,沿着d_current得到一个步长的单谷区间,该单谷区间的初始上届
% tolerance, 最终区间的要求宽度,精度不能小于扰动量% 输出参数说明
% alpha_star, 完成对分搜索后输出的步长
% x_next, x_next = x_current + alpha_star * d_current;局部最优点
% f_next, 局部最优点对应的函数值
% k,迭代次数% 初始值
k = 0;
alpha_lower_k = alpha_lower;
alpha_upper_k = alpha_upper;alpha_left_k = alpha_lower_k;
alpha_right_k = alpha_upper_k;
alpha_middle_k = (alpha_left_k + alpha_right_k) / 2;x_alpha_left_k = x_current + alpha_left_k * d_current;
x_alpha_right_k = x_current + alpha_right_k * d_current;
x_alpha_middle_k = x_current + alpha_middle_k * d_current;f_alpha_left_k = f_test(x_alpha_left_k);
f_alpha_right_k = f_test(x_alpha_right_k);
f_alpha_middle_k = f_test(x_alpha_middle_k);% 用来保证算法能够启动,因为需要|ak+1-ak|作为判断条件,但第一次无法
% 计算这个,通过100 * tolerance保证能够进入循环进行运算,之后再进行
% 更新
gap_k = 100 * tolerance;
alpha_interpolation_previous = -100;while gap_k > tolerancek = k + 1;a1 = (f_alpha_left_k - f_alpha_middle_k) * (alpha_middle_k - alpha_right_k) * (alpha_right_k - alpha_left_k);a2 = f_alpha_left_k * (alpha_middle_k - alpha_right_k) + f_alpha_middle_k * (alpha_right_k - alpha_left_k) + f_alpha_right_k * (alpha_left_k - alpha_middle_k);alpha_interpolation_k = (1/2) * ((alpha_left_k + alpha_middle_k) + (a1 / a2));x_alpha_interpolation_k = x_current + alpha_interpolation_k * d_current;f_alpha_interpolation_k = f_test(x_alpha_interpolation_k);alpha_interpolation_current = alpha_interpolation_k;alpha_middle_previous = alpha_middle_k;f_alpha_middle_previous = f_alpha_middle_k;if ((alpha_left_k < alpha_interpolation_k) && (alpha_interpolation_k < alpha_middle_k))if (f_alpha_interpolation_k <= f_alpha_middle_k)alpha_right_k = alpha_middle_k;f_alpha_right_k = f_alpha_middle_k;alpha_middle_k = alpha_interpolation_k;f_alpha_middle_k = f_alpha_interpolation_k;elsealpha_left_k = alpha_interpolation_k;f_alpha_left_k = f_alpha_interpolation_k;endendif ((alpha_middle_k < alpha_interpolation_k) && (alpha_interpolation_k < alpha_right_k))if (f_alpha_interpolation_k <= f_alpha_middle_k)alpha_left_k = alpha_middle_k;f_alpha_left_k = f_alpha_middle_k;alpha_middle_k = alpha_interpolation_k;f_alpha_middle_k = f_alpha_interpolation_k;elsealpha_right_k = alpha_interpolation_k;f_alpha_right_k = f_alpha_interpolation_k;endendgap_k = abs(alpha_interpolation_current - alpha_interpolation_previous);alpha_interpolation_previous = alpha_interpolation_current;
endf_alpha_interpolation_current = f_alpha_interpolation_k;
if (f_alpha_interpolation_current <= f_alpha_interpolation_k)alpha_star = alpha_interpolation_k;
elsealpha_star = alpha_middle_previous;
endx_next = x_current + alpha_star * d_current;
f_next = f_test(x_next);
end
2.主函数运行文件
% 这个文件主要用来进行斐波那契搜索法的运行close;
clear;
clc;% 示例一
% x_current = -0.5;
% d_current = 1;
% alpha_lower = 0;
% alpha_upper = 1;
% tolerance = 1e-4;
% [alpha_star, x_next, f_next, k] = Quadratic3points_search(@f_test1, x_current, d_current, alpha_lower, alpha_upper, tolerance);% 示例二
% x_current = [2, 2];
% d_current = [-1, -1];
% alpha_lower = 0;
% alpha_upper = 2;
% tolerance = 1e-6;
% [alpha_star, x_next, f_next, k] = Quadratic3points_search(@f_test2, x_current, d_current, alpha_lower, alpha_upper, tolerance);% 示例三
x_current = 3;
d_current = -1;
alpha_lower = 0;
alpha_upper = 2;
tolerance = 1e-4;
[alpha_star, x_next, f_next, k] = Quadratic3points_search(@f_test3, x_current, d_current, alpha_lower, alpha_upper, tolerance);
3.一些说明
如果按照文中代码,如图所示红色部分圈出来的。在针对第一个和第二个示例的时,运行结果跟书中一致,跟前面几种方法是相同的。
但如果运行第三个示例的话,按照书中这种方法,则不会运行处正确结果。原因是因为这样计算出来的gap_k永远为0,程序只会迭代一次就结束。
更改完成的代码如上面所示,主要是提前将alpha_interpolation_previous进行初始化,这个值我设置为了-100,可以设置不可能达到的数字,这样能够保证每次运行都可以成功。其次,我将alpha_interpolation_previous的更新放在循环的最后,这样解决了上述问题。
相关文章:
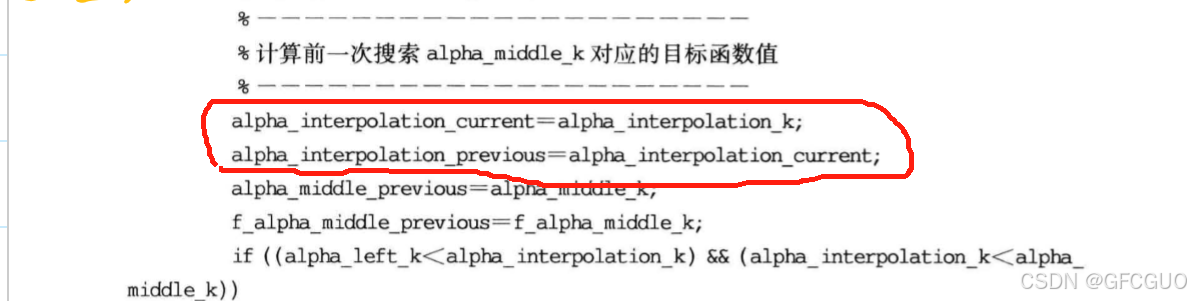
应用最优化方法及MATLAB实现——第3章代码实现
一、概述 在阅读最优方法及MATLAB实现后,想着将书中提供的代码自己手敲一遍,来提高自己对书中内容理解程度,巩固一下。 这部分内容主要针对第3章的内容,将其所有代码实现均手敲一遍,中间部分代码自己根据其公式有些许的…...
django的增删改查,排序,分组等常用的ORM操作
Django 的 ORM(对象关系映射)提供了一种方便的方式来与数据库进行交互。 1. Django模型 在 myapp/models.py 中定义一个示例模型:python from django.db import modelsclass Person(models.Model):name models.CharField(max_length100)age…...
Leetcode Java学习记录——树、二叉树、二叉搜索树
文章目录 树的定义树的遍历中序遍历代码 二叉搜索树 常见二维数据结构:树/图 树和图的区别就在于有没有环。 树的定义 public class TreeNode{public int val;public TreeNode left,right;public TreeNode(int val){this.val val;this.left null;this.right nu…...
华为HCIP Datacom H12-821 卷30
1.单选题 以下关于OSPF协议报文说法错误的是? A、OSPF报文采用UDP报文封装并且端口号是89 B、OSPF所有报文的头部格式相同 C、OSPF协议使用五种报文完成路由信息的传递 D、OSPF所有报文头部都携带了Router-ID字段 正确答案:A 解析: OSPF用IP报文直接封装协议报文,…...
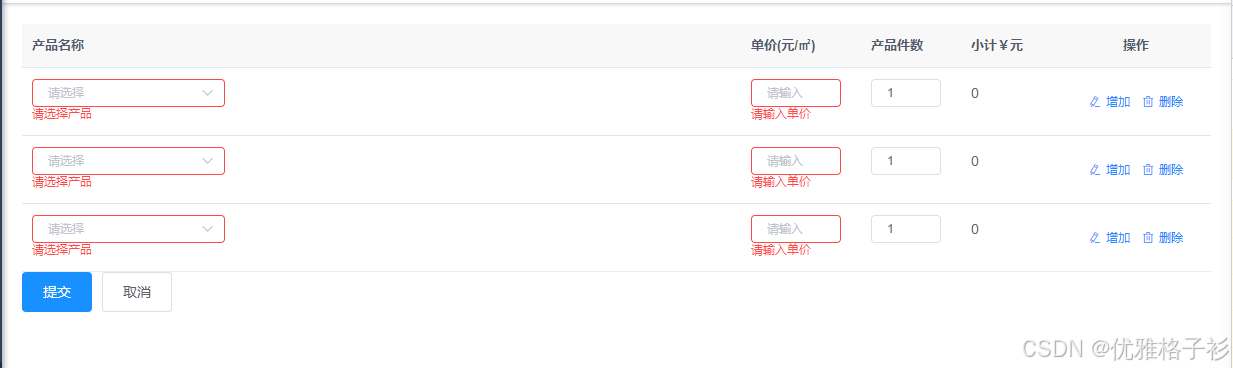
element el-table实现表格动态增加/删除/编辑表格行,带校验规则
本篇文章记录el-table增加一行可编辑的数据列,进行增删改。 1.增加空白行 直接在页面mounted时对form里面的table列表增加一行数据,直接使用push() 方法增加一列数据这个时候也可以设置一些默认值。比如案例里面的 产品件数 。 mounted() {this.$nextTi…...
QT调节屏幕亮度
1、目标 利用QT实现调节屏幕亮度功能:在无屏幕无触控时,将屏幕亮度调低,若有触控则调到最亮。 2、调节亮度命令 目标装置使用嵌入式Linux系统,调节屏幕亮度的指令为: echo x > /sys/class/backlight/backlight/…...
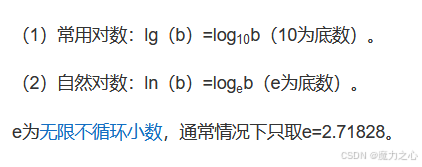
实变函数精解【3】
文章目录 点集求导集 闭集参考文献 点集 求导集 例1 E { 1 / n 1 / m : n , m ∈ N } 1. lim n → ∞ ( 1 / n 1 / m ) 1 / m 2. lim n , m → ∞ ( 1 / n 1 / m ) 0 3. E ′ { 0 , 1 , 1 / 2 , 1 / 3 , . . . . } E\{1/n1/m:n,m \in N\} \\1.\lim_{n \rightar…...

JVM:SpringBoot TomcatEmbeddedWebappClassLoader
文章目录 一、介绍二、SpringBoot中TomcatEmbeddedWebappClassLoader与LaunchedURLClassLoader的关系 一、介绍 TomcatEmbeddedWebappClassLoader 是 Spring Boot 在其内嵌 Tomcat 容器中使用的一个类加载器(ClassLoader)。在 Spring Boot 应用中&#…...
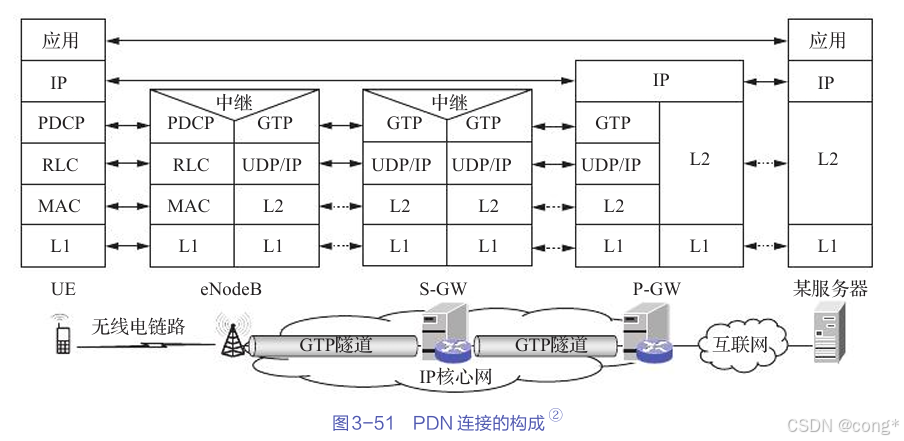
蜂窝互联网接入:连接世界的无缝体验
通过Wi—Fi,人们可以方便地接入互联网,但无线局域网的覆盖范围通常只有10~100m。当我们携带笔记本电脑在外面四处移动时,并不是在所有地方都能找到可接入互联网的Wi—Fi热点,这时候蜂窝移动通信系统可以为我们提供广域…...

Sprint Boot 2 核心功能(一)
核心功能 1、配置文件 application.properties 同基础入门篇的application.properties用法一样 Spring Boot 2 入门基础 application.yaml(或application.yml) 基本语法 key: value;kv之间有空格大小写敏感使用缩进表示层级关系缩进不允…...
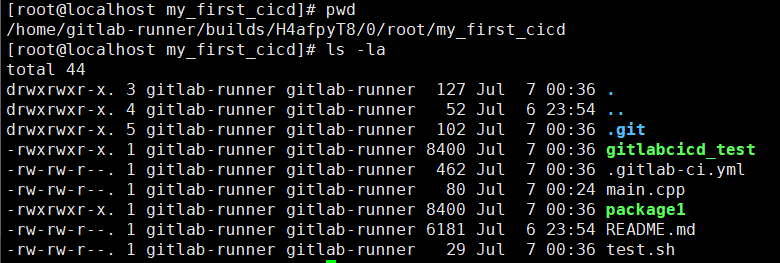
GitLab CI/CD实现项目自动化部署
1 GitLab CI/CD介绍 GitLab CI/CD 是 GitLab 中集成的一套用于软件开发的持续集成(Continuous Integration)、持续交付(Continuous Delivery)和持续部署(Continuous Deployment)工具。这套系统允许开发团队…...
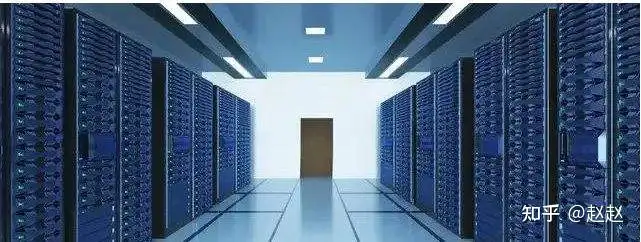
阿里云调整全球布局关停澳洲云服务器,澳洲服务器市场如何选择稳定可靠的云服务?
近日,阿里云宣布将关停澳大利亚地域的数据中心服务,这一决定引发了全球云计算行业的广泛关注。作为阿里云的重要海外市场之一,澳洲的数据中心下架对于当地的企业和个人用户来说无疑是一个不小的挑战。那么,在阿里云调整全球布局的…...
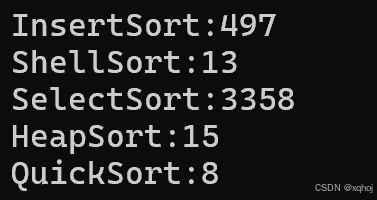
排序(二)——快速排序(QuickSort)
欢迎来到繁星的CSDN,本期内容包括快速排序(QuickSort)的递归版本和非递归版本以及优化。 一、快速排序的来历 快速排序又称Hoare排序,由霍尔 (Sir Charles Antony Richard Hoare) ,一位英国计算机科学家发明。霍尔本人是在发现冒泡排序不够快…...
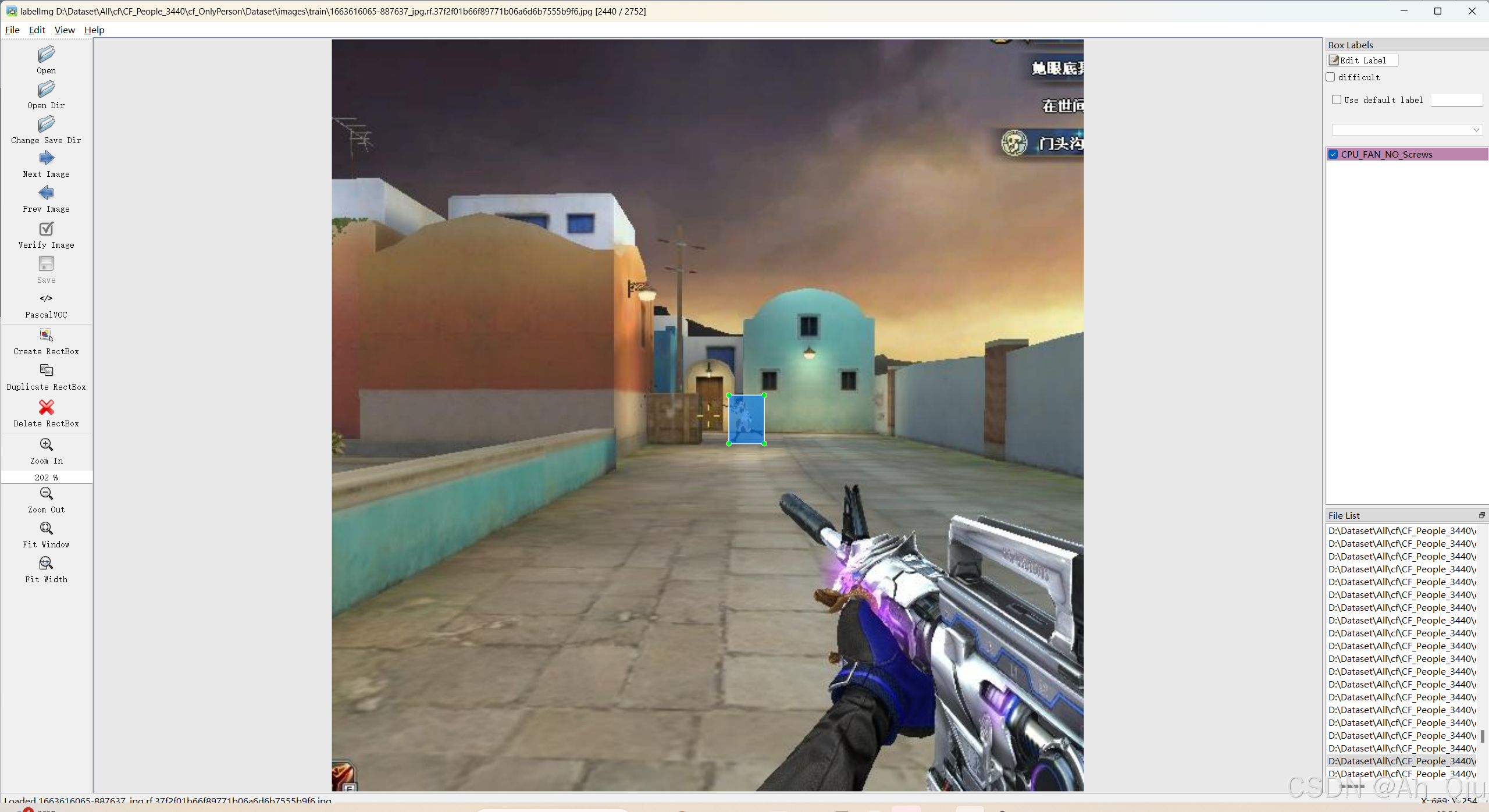
<数据集>穿越火线cf人物识别数据集<目标检测>
数据集格式:VOCYOLO格式 图片数量:3440张 标注数量(xml文件个数):3440 标注数量(txt文件个数):3440 标注类别数:1 标注类别名称:[person] 使用标注工具:labelImg 标注规则:对…...
a+=1和a=a+1的区别
文章目录 a1 和a a1的区别一、实例代码二、代码解释三、总结 a1 和a a1的区别 一、实例代码 public class Test {public static void main(String[] args) {byte a 10; // a a 1; // a (byte) (a 1);a 1;System.out.println(a);} }上面的对变量a进行加一操作时&a…...
设计模式使用场景实现示例及优缺点(结构型模式——桥接模式)
结构型模式 桥接模式(Bridge Pattern) 桥接模式(Bridge Pattern)是一种结构型设计模式,其主要目的是“将抽象与实现解耦,使得两者可以独立地变化”。这种模式通过提供抽象化和实现化之间的桥接结构&#…...
Spring——自动装配Bean
自动装配是Spring满足bean依赖的一种方式 Spring会在上下文中自动寻找,并自动给bean装配属性 在Spring中有三种装配的方式: 1. 在xml中显示配置 2. 在java中显示配置 3. 隐式的自动装配bean【重要】 测试 记得创建Cat、Dog、People类 public clas…...
云端典藏:iCloud中个人收藏品目录的智能存储方案
云端典藏:iCloud中个人收藏品目录的智能存储方案 在数字化生活不断推进的今天,个人收藏品的管理也趋向于电子化和云端化。iCloud作为苹果公司提供的云服务,为个人收藏品目录的存储和管理提供了一个安全、便捷、跨设备的解决方案。本文将详细…...
安全开发基础篇-数据溢出
上一节我们简单讲解了多语言的数据类型,我们只需要知道这个概念,并且在不同语言有不同的规矩就好。这节讲数据溢出,严格说应该是字符串溢出和整数溢出。 在软件开发中,字符串和整数溢出漏洞是常见的安全问题,它们可能…...
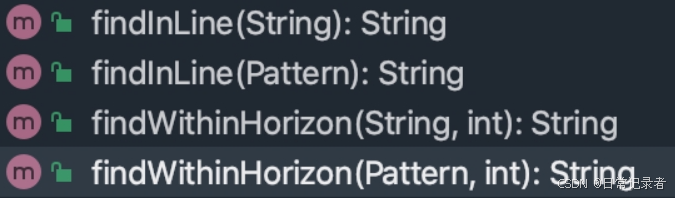
Scanner工具类
扫描控制台输入 1.nextLine nextLine() 方法会扫描输入流中的字符,直到遇到行末尾的换行符 \n,然后将该行的内容作为字符串返回,同时,nextLine() 会将 Scanner 对象的位置移动到下一行的开头,以便下一次读取数据时从下…...
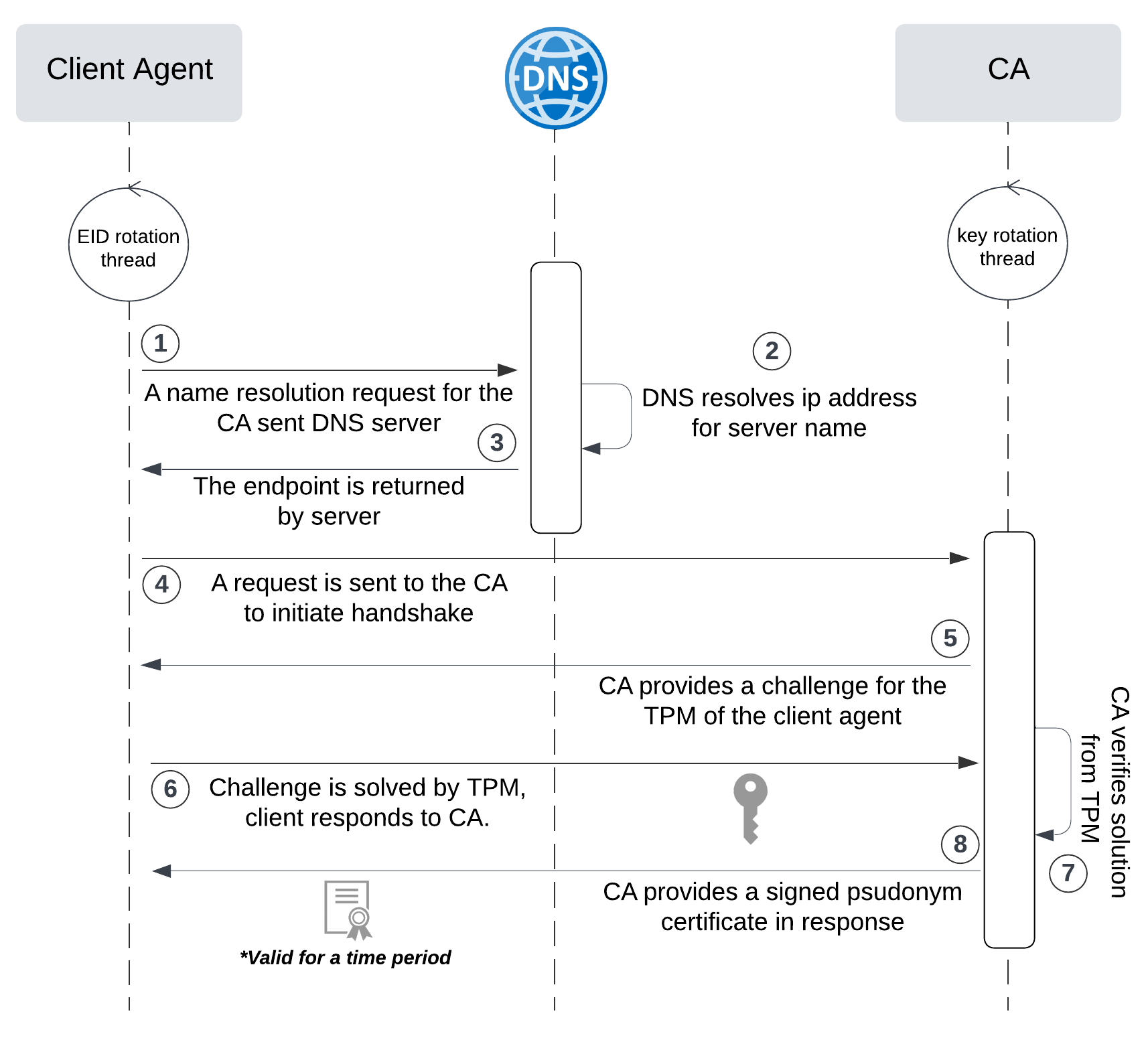
网络六边形受到攻击
大家读完觉得有帮助记得关注和点赞!!! 抽象 现代智能交通系统 (ITS) 的一个关键要求是能够以安全、可靠和匿名的方式从互联车辆和移动设备收集地理参考数据。Nexagon 协议建立在 IETF 定位器/ID 分离协议 (…...
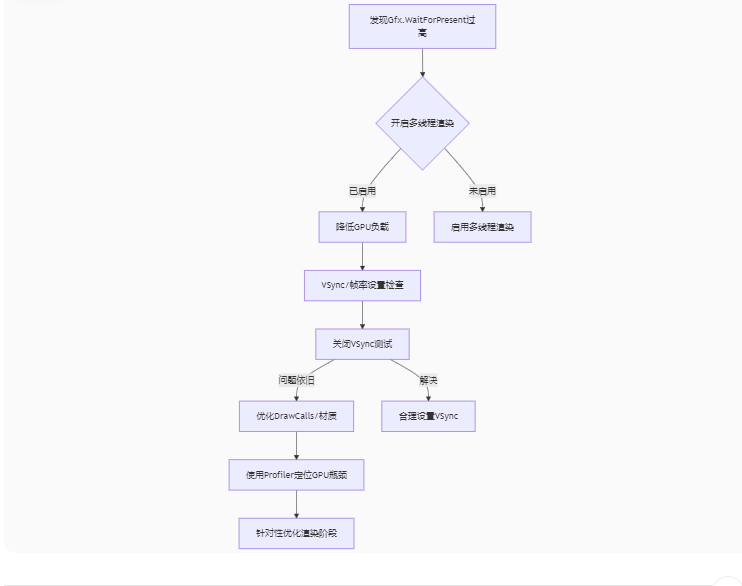
Unity3D中Gfx.WaitForPresent优化方案
前言 在Unity中,Gfx.WaitForPresent占用CPU过高通常表示主线程在等待GPU完成渲染(即CPU被阻塞),这表明存在GPU瓶颈或垂直同步/帧率设置问题。以下是系统的优化方案: 对惹,这里有一个游戏开发交流小组&…...
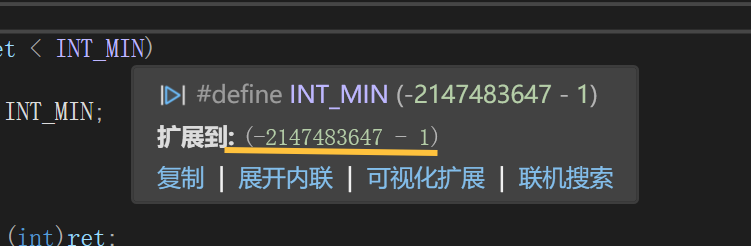
练习(含atoi的模拟实现,自定义类型等练习)
一、结构体大小的计算及位段 (结构体大小计算及位段 详解请看:自定义类型:结构体进阶-CSDN博客) 1.在32位系统环境,编译选项为4字节对齐,那么sizeof(A)和sizeof(B)是多少? #pragma pack(4)st…...
AtCoder 第409场初级竞赛 A~E题解
A Conflict 【题目链接】 原题链接:A - Conflict 【考点】 枚举 【题目大意】 找到是否有两人都想要的物品。 【解析】 遍历两端字符串,只有在同时为 o 时输出 Yes 并结束程序,否则输出 No。 【难度】 GESP三级 【代码参考】 #i…...
五年级数学知识边界总结思考-下册
目录 一、背景二、过程1.观察物体小学五年级下册“观察物体”知识点详解:由来、作用与意义**一、知识点核心内容****二、知识点的由来:从生活实践到数学抽象****三、知识的作用:解决实际问题的工具****四、学习的意义:培养核心素养…...
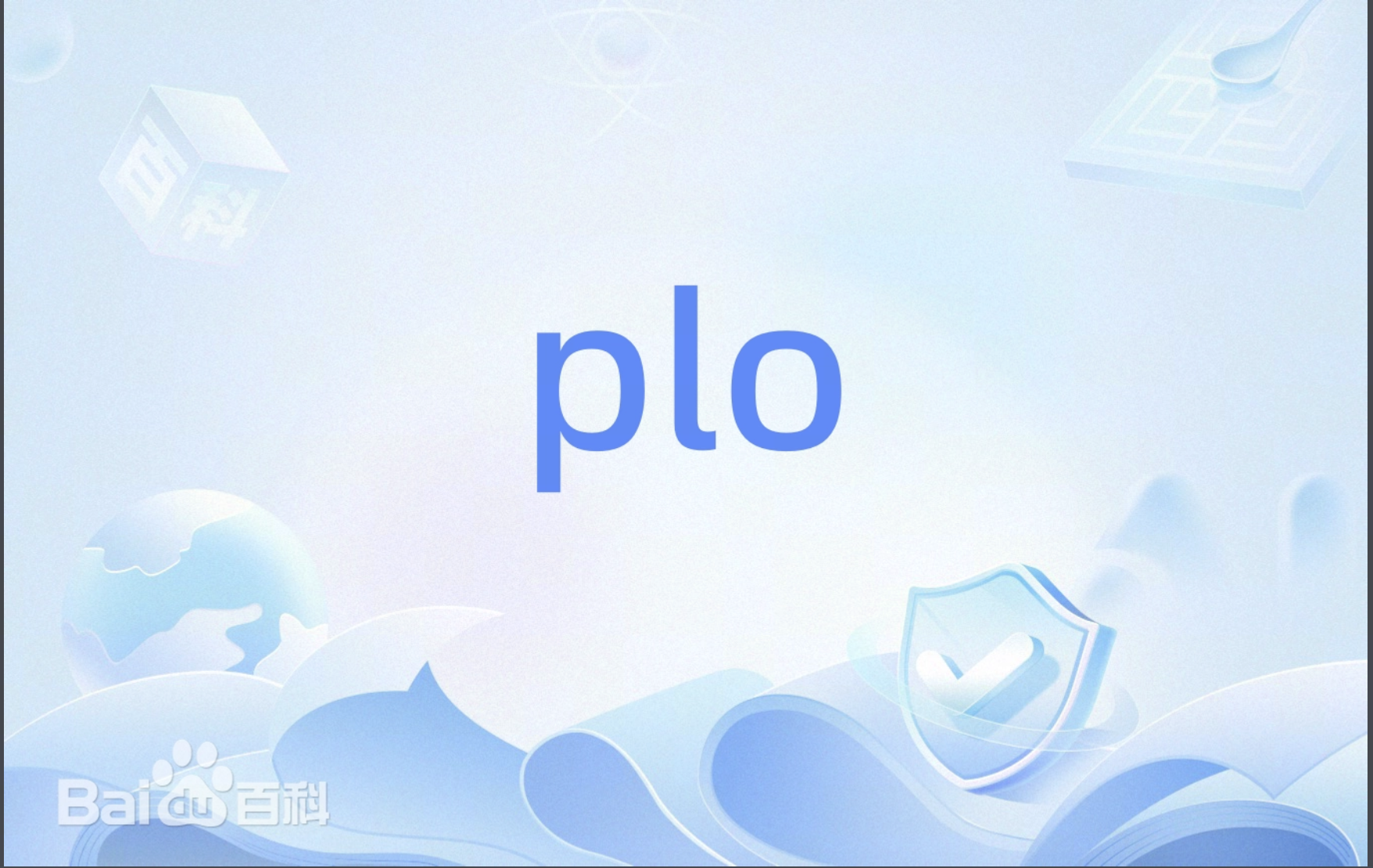
PL0语法,分析器实现!
简介 PL/0 是一种简单的编程语言,通常用于教学编译原理。它的语法结构清晰,功能包括常量定义、变量声明、过程(子程序)定义以及基本的控制结构(如条件语句和循环语句)。 PL/0 语法规范 PL/0 是一种教学用的小型编程语言,由 Niklaus Wirth 设计,用于展示编译原理的核…...

涂鸦T5AI手搓语音、emoji、otto机器人从入门到实战
“🤖手搓TuyaAI语音指令 😍秒变表情包大师,让萌系Otto机器人🔥玩出智能新花样!开整!” 🤖 Otto机器人 → 直接点明主体 手搓TuyaAI语音 → 强调 自主编程/自定义 语音控制(TuyaAI…...
数据库分批入库
今天在工作中,遇到一个问题,就是分批查询的时候,由于批次过大导致出现了一些问题,一下是问题描述和解决方案: 示例: // 假设已有数据列表 dataList 和 PreparedStatement pstmt int batchSize 1000; // …...
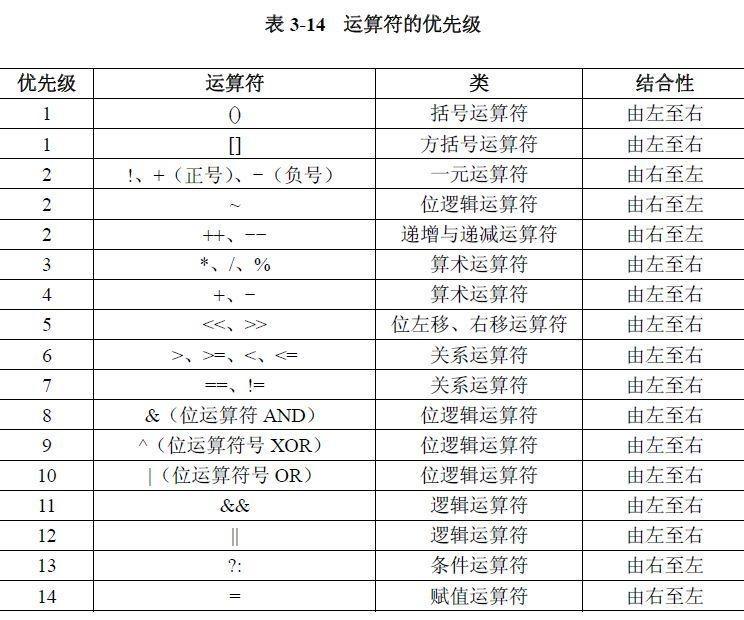
02.运算符
目录 什么是运算符 算术运算符 1.基本四则运算符 2.增量运算符 3.自增/自减运算符 关系运算符 逻辑运算符 &&:逻辑与 ||:逻辑或 !:逻辑非 短路求值 位运算符 按位与&: 按位或 | 按位取反~ …...
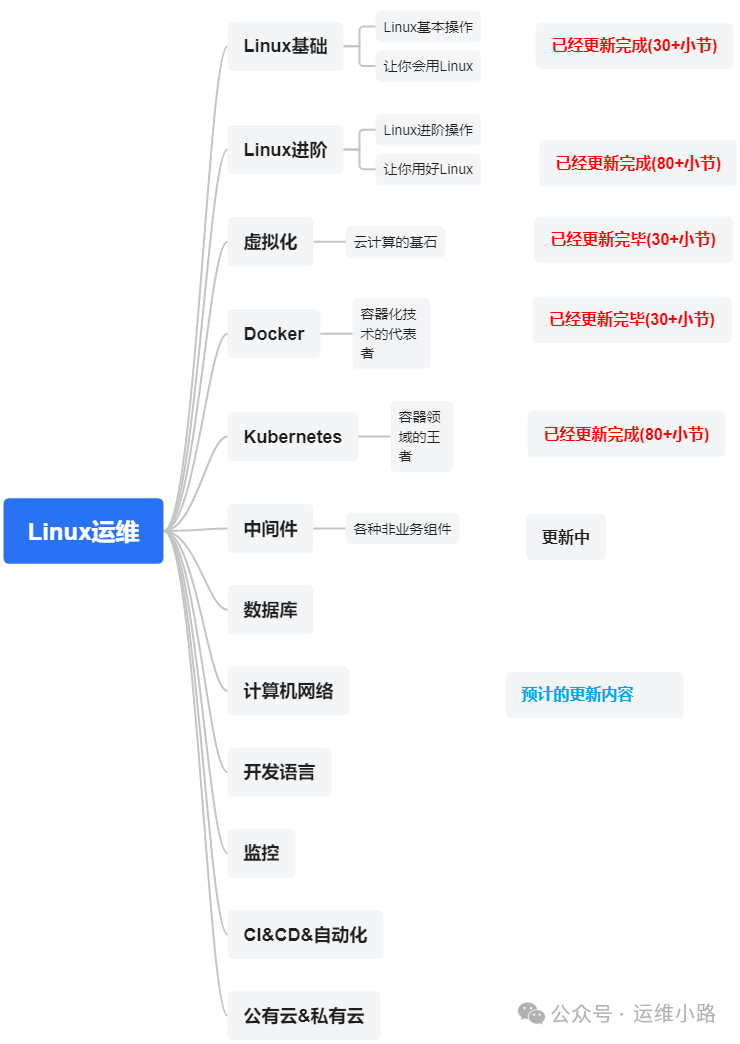
代理服务器-LVS的3种模式与调度算法
作者介绍:简历上没有一个精通的运维工程师。请点击上方的蓝色《运维小路》关注我,下面的思维导图也是预计更新的内容和当前进度(不定时更新)。 我们上一章介绍了Web服务器,其中以Nginx为主,本章我们来讲解几个代理软件:…...