AutoML-sklearn and torch
一、auto-sklearn
1.1 环境依赖
-
额外安装swig 第三方库
-
linux 支持, mac,windows不支持
1.2 示例代码
time_left_for_this_task 设定任务最大时间
per_run_time_limit 每个子任务最大训练时间
include 可以限制任务训练的模型
import autosklearn.classification
import sklearn.model_selection
from sklearn import datasets
import sklearn.metricsif __name__ == "__main__":X, y = datasets.load_breast_cancer(return_X_y=True)X_train, X_test, y_train, y_test = \sklearn.model_selection.train_test_split(X, y, random_state=1)automl = autosklearn.classification.AutoSklearnClassifier(time_left_for_this_task=120,per_run_time_limit=30,tmp_folder="/tmp/autosklearn_classification_example_tmp",include={'classifier': ["random_forest"],'feature_preprocessor': ["no_preprocessing"]})automl.fit(X_train, y_train)y_hat = automl.predict(X_test)automl.get_models_with_weights()print("Accuracy score", sklearn.metrics.accuracy_score(y_test, y_hat))print(automl.leaderboard())models_with_weights = automl.get_models_with_weights()with open('../../preprocess/models_report.txt', 'w') as f:for model in models_with_weights:f.write(str(model) + '\n')
结果展示:
可以展示参数任务cost值排列顺序
以及训练参数配置:
1.3 模块扩展
在不支持的训练模块,可以扩展及自定义模型进行自动调参
代码示例:
继承AutoSklearnClassificationAlgorithm 并重写子方法
autosklearn.pipeline.components.classification.add_classifier(MLPClassifier) 将自定义模块注册至模块中
include 参数添加既可调用
"""
====================================================
Extending Auto-Sklearn with Classification Component
====================================================The following example demonstrates how to create a new classification
component for using in auto-sklearn.
"""
from typing import Optional
from pprint import pprintfrom ConfigSpace.configuration_space import ConfigurationSpace
from ConfigSpace.hyperparameters import (CategoricalHyperparameter,UniformIntegerHyperparameter,UniformFloatHyperparameter,
)import sklearn.metricsfrom autosklearn.askl_typing import FEAT_TYPE_TYPE
import autosklearn.classification
import autosklearn.pipeline.components.classification
from autosklearn.pipeline.components.base import AutoSklearnClassificationAlgorithm
from autosklearn.pipeline.constants import (DENSE,SIGNED_DATA,UNSIGNED_DATA,PREDICTIONS,
)from sklearn.datasets import load_breast_cancer
from sklearn.model_selection import train_test_split############################################################################
# Create MLP classifier component for auto-sklearn
# ================================================class MLPClassifier(AutoSklearnClassificationAlgorithm):def __init__(self,hidden_layer_depth,num_nodes_per_layer,activation,alpha,solver,random_state=None,):self.hidden_layer_depth = hidden_layer_depthself.num_nodes_per_layer = num_nodes_per_layerself.activation = activationself.alpha = alphaself.solver = solverself.random_state = random_statedef fit(self, X, y):self.num_nodes_per_layer = int(self.num_nodes_per_layer)self.hidden_layer_depth = int(self.hidden_layer_depth)self.alpha = float(self.alpha)from sklearn.neural_network import MLPClassifierhidden_layer_sizes = tuple(self.num_nodes_per_layer for i in range(self.hidden_layer_depth))self.estimator = MLPClassifier(hidden_layer_sizes=hidden_layer_sizes,activation=self.activation,alpha=self.alpha,solver=self.solver,random_state=self.random_state,)self.estimator.fit(X, y)return selfdef predict(self, X):if self.estimator is None:raise NotImplementedError()return self.estimator.predict(X)def predict_proba(self, X):if self.estimator is None:raise NotImplementedError()return self.estimator.predict_proba(X)@staticmethoddef get_properties(dataset_properties=None):return {"shortname": "MLP Classifier","name": "MLP CLassifier","handles_regression": False,"handles_classification": True,"handles_multiclass": True,"handles_multilabel": False,"handles_multioutput": False,"is_deterministic": False,# Both input and output must be tuple(iterable)"input": [DENSE, SIGNED_DATA, UNSIGNED_DATA],"output": [PREDICTIONS],}@staticmethoddef get_hyperparameter_search_space(feat_type: Optional[FEAT_TYPE_TYPE] = None, dataset_properties=None):cs = ConfigurationSpace()hidden_layer_depth = UniformIntegerHyperparameter(name="hidden_layer_depth", lower=1, upper=3, default_value=1)num_nodes_per_layer = UniformIntegerHyperparameter(name="num_nodes_per_layer", lower=16, upper=216, default_value=32)activation = CategoricalHyperparameter(name="activation",choices=["identity", "logistic", "tanh", "relu"],default_value="relu",)alpha = UniformFloatHyperparameter(name="alpha", lower=0.0001, upper=1.0, default_value=0.0001)solver = CategoricalHyperparameter(name="solver", choices=["lbfgs", "sgd", "adam"], default_value="adam")cs.add_hyperparameters([hidden_layer_depth,num_nodes_per_layer,activation,alpha,solver,])return cs# Add MLP classifier component to auto-sklearn.
autosklearn.pipeline.components.classification.add_classifier(MLPClassifier)
cs = MLPClassifier.get_hyperparameter_search_space()
print(cs)############################################################################
# Data Loading
# ============
def get_local_csv():import pandas as pdimport numpy as npdf = pd.read_csv("/data/projects/example/auto_ml/Radiomics-2D/features.csv")label = pd.read_csv("/data/projects/example/auto_ml/Radiomics-2D/labels.csv")["label"]label = np.array([1 if l == "Positive" else 0 for l in label])return df.to_numpy(), label# local
X, y = get_local_csv()# breast cancer
# X, y = load_breast_cancer(return_X_y=True)X_train, X_test, y_train, y_test = train_test_split(X, y)############################################################################
# Fit MLP classifier to the data
# ==============================clf = autosklearn.classification.AutoSklearnClassifier(time_left_for_this_task=60,per_run_time_limit=30,include={"classifier": ["gradient_boosting", "adaboost", "MLPClassifier"],'feature_preprocessor': ["no_preprocessing"]},
)
clf.fit(X_train, y_train)############################################################################
# Print test accuracy and statistics
# ==================================y_pred = clf.predict(X_test)
print("accuracy: ", sklearn.metrics.accuracy_score(y_pred, y_test))
print(clf.sprint_statistics())
print(clf.leaderboard(detailed=False,top_k=30))
pprint(clf.show_models(), indent=4)models_with_weights = clf.get_models_with_weights()
with open('./models_report.txt', 'w') as f:for model in models_with_weights:f.write(str(model) + '\n')
二、auto-pytorch
1. 1 环境依赖
额外安装brew install cmake
lightgbm 库依赖第三方库 pip install lightgbm
brew install libomp
pip install autoPyTorch
mac 允许不限制memory, M1 芯片对内容限制的操作目前还有bug
1.2 支持用法
支持大量的表格型数据,图片数据支持少,且不支持扩展
代码示例:
用法比较固定,没有更多的文档来作为参考,且无法扩展。
import numpy as npimport sklearn.model_selectionimport torchvision.datasetsfrom autoPyTorch.pipeline.image_classification import ImageClassificationPipeline# Get the training data for tabular classification
trainset = torchvision.datasets.FashionMNIST(root='../datasets/', train=True, download=True)
data = trainset.data.numpy()
data = np.expand_dims(data, axis=3)
# Create a proof of concept pipeline!
dataset_properties = dict()
pipeline = ImageClassificationPipeline(dataset_properties=dataset_properties)# Train and test split
train_indices, val_indices = sklearn.model_selection.train_test_split(list(range(data.shape[0])),random_state=1,test_size=0.25,
)# Configuration space
pipeline_cs = pipeline.get_hyperparameter_search_space()
print("Pipeline CS:\n", '_' * 40, f"\n{pipeline_cs}")
config = pipeline_cs.sample_configuration()
print("Pipeline Random Config:\n", '_' * 40, f"\n{config}")
pipeline.set_hyperparameters(config)# Fit the pipeline
print("Fitting the pipeline...")pipeline.fit(X=dict(X_train=data,is_small_preprocess=True,dataset_properties=dict(mean=np.array([np.mean(data[:, :, :, i]) for i in range(1)]),std=np.array([np.std(data[:, :, :, i]) for i in range(1)]),num_classes=10,num_features=data.shape[1] * data.shape[2],image_height=data.shape[1],image_width=data.shape[2],is_small_preprocess=True),train_indices=train_indices,val_indices=val_indices,))# Showcase some components of the pipeline
print(pipeline)
相关文章:
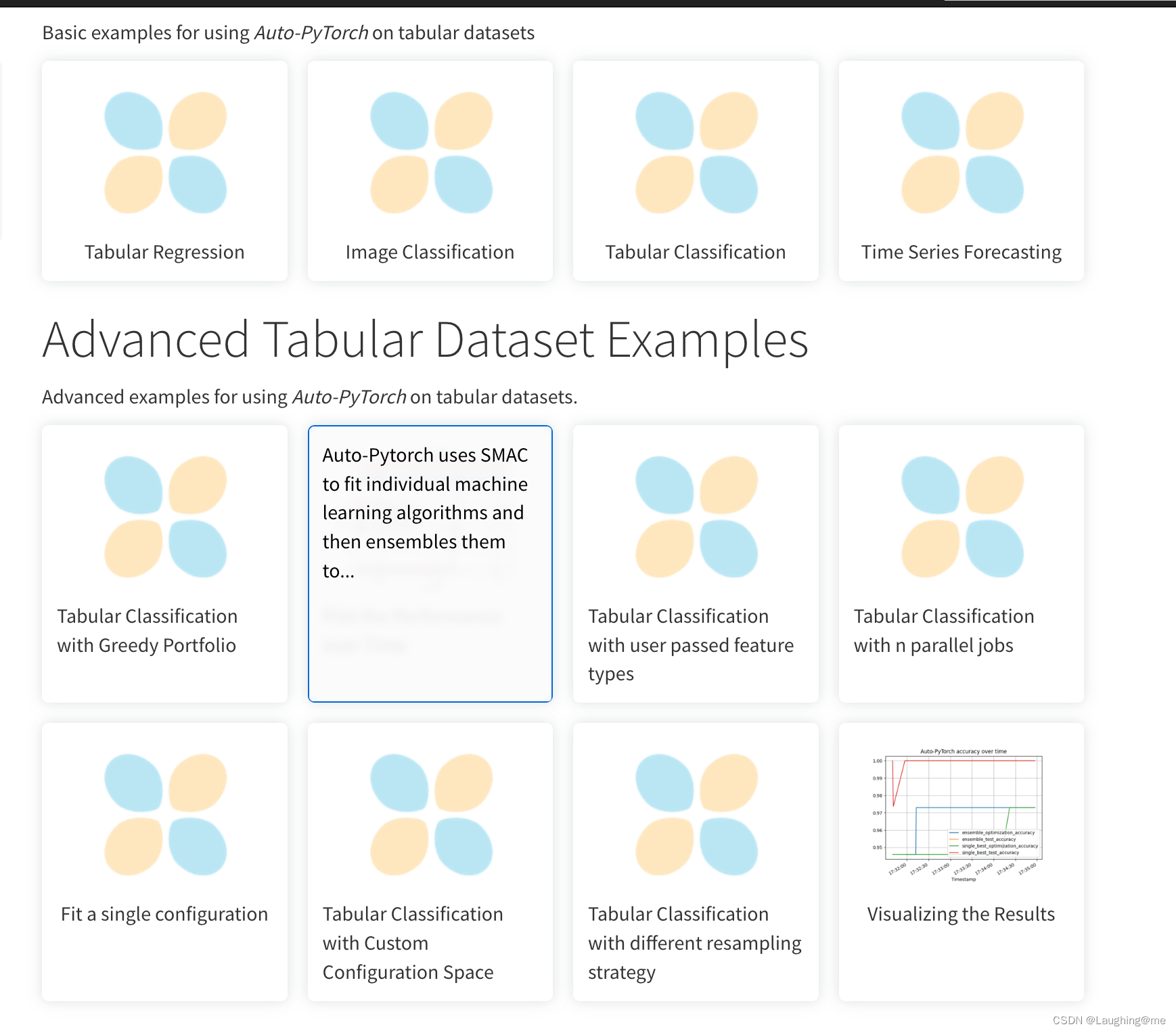
AutoML-sklearn and torch
一、auto-sklearn 1.1 环境依赖 额外安装swig 第三方库 linux 支持, mac,windows不支持 1.2 示例代码 time_left_for_this_task 设定任务最大时间 per_run_time_limit 每个子任务最大训练时间 include 可以限制任务训练的模型 import autosklearn.classific…...
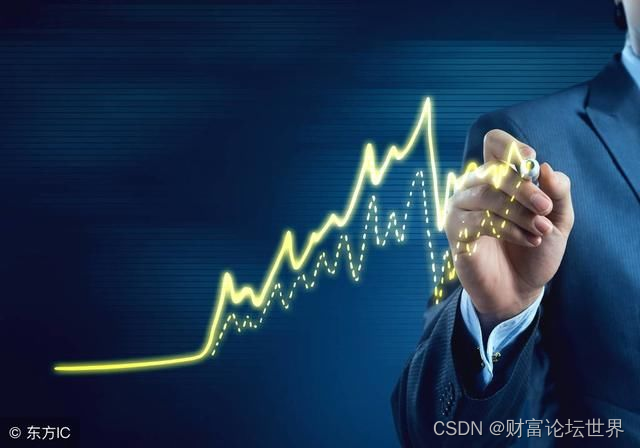
《扬帆优配》算力概念股大爆发,主力资金大扫货
3月22日,9股封单金额超亿元,工业富联、鸿博股份、鹏鼎控股分别为3.01亿元、2.78亿元、2.37亿元。 今日三大指数团体收涨,收盘共34股涨停,首要集中于数字经济方向,其间云核算、CPO大迸发。除去5只ST股,算计2…...
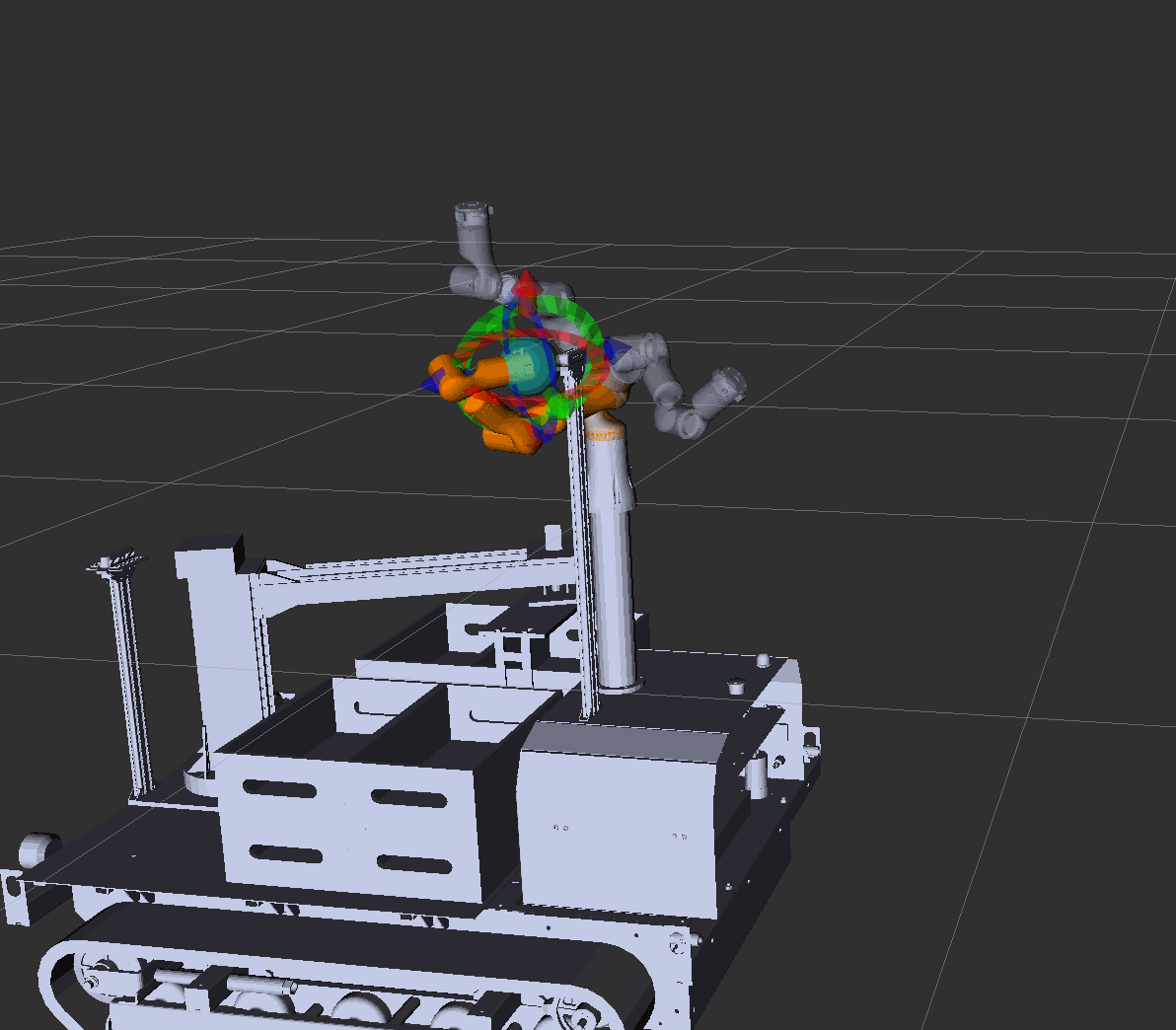
机械臂+底盘三维模型从solidworks到moveit配置功能包
文章目录 导出底盘STEP加载机械臂模型组合机械臂和底盘三维模型导出URDF在moveit中进行配置新建工作目录设置ROS工作空间的环境变量进入moveit setup加载URDF文件self-CollisionsPlanning groupsRobot posesControllersSimulationAuthor information生成配置包在rviz中进行可视…...
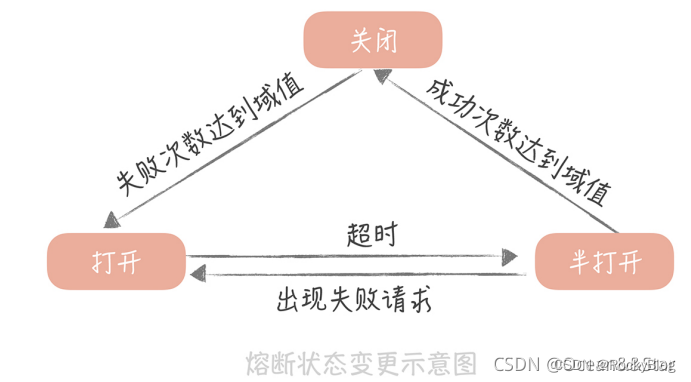
高并发系统设计:缓存、降级、限流、(熔断)
高并发系统设计:缓存、降级、限流、(熔断) 在开发高并发系统时有三把利器用来保护系统:缓存、降级和限流。 非核心服务可以采用降级、熔断,核心服务采用缓存和限流(隔离流量可以最大限度的保障业务无损)。 缓存 缓…...
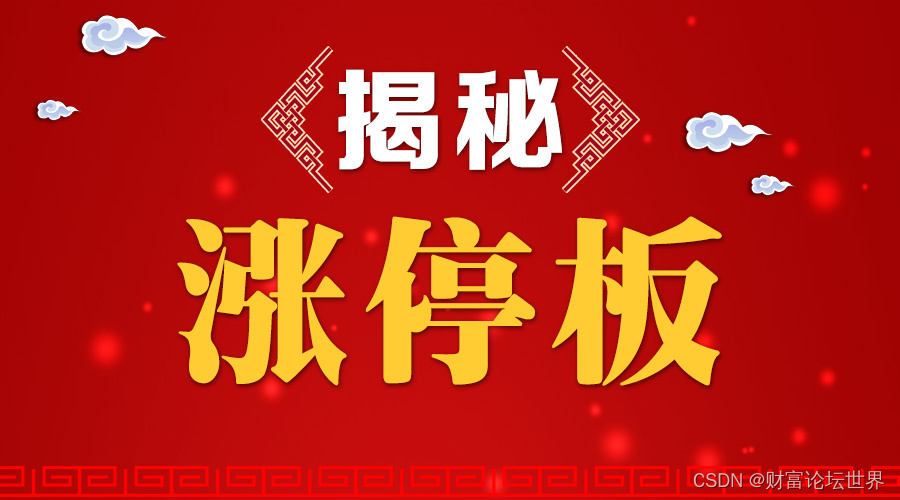
《辉煌优配》放量大涨,A股成交额重回万亿!PCB板块继续领跑
多只绩优PCB概念股超跌。 今日A股放量反弹,成交额从头站上万亿关口。芯片板块掀涨停潮,景嘉微、芯原股份20cm涨停,紫光国微、兆易创新、跃岭股份等封板;AI算力、存储器、光模块、云核算等板块全线拉升,板块内个股再度批…...
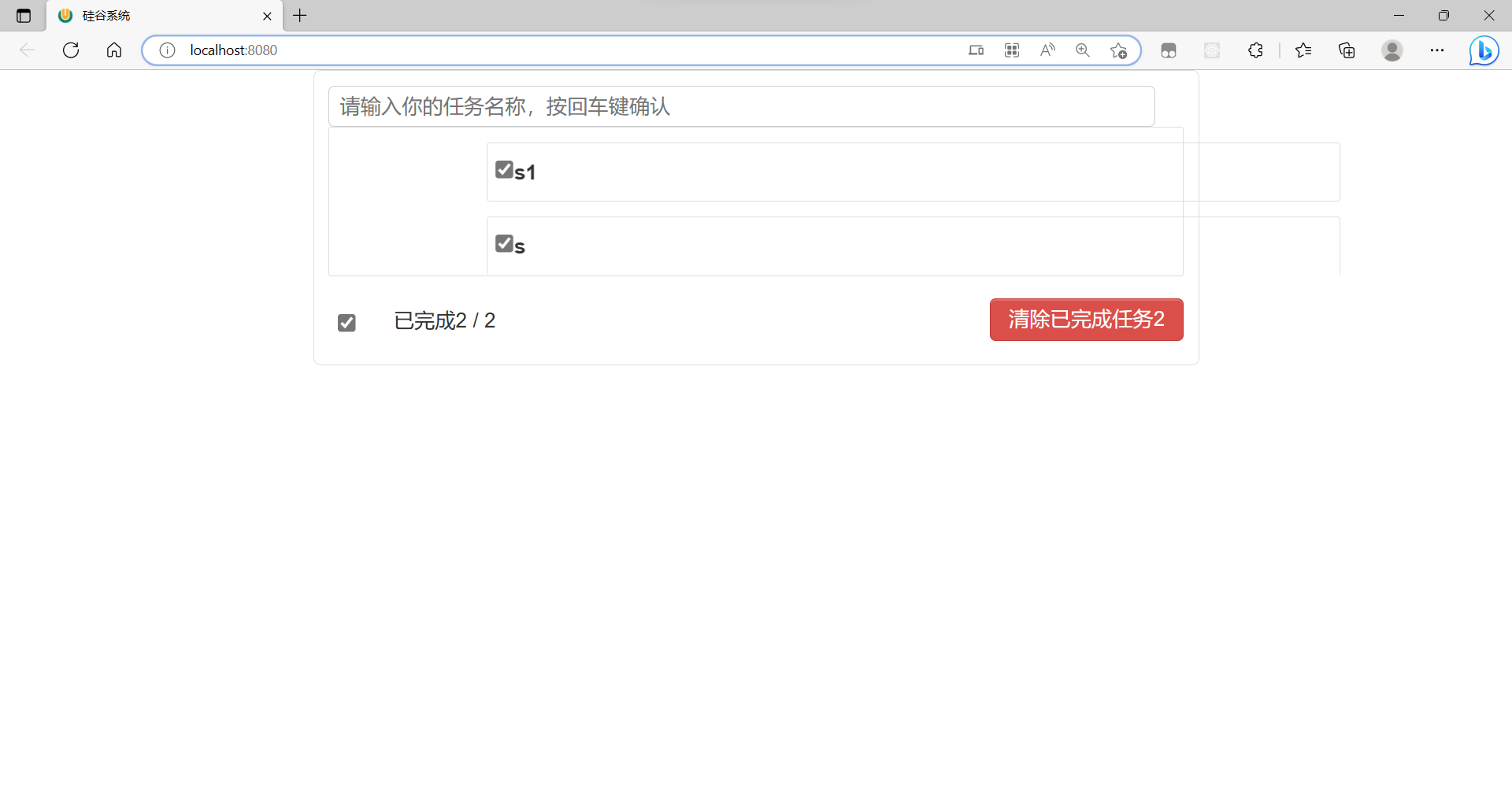
Vue封装的过度与动画
动画效果 先把样式封装好,然后设置一个动画 不需要vue也能实现的动画的效果,我们只需要判断一下,然后动态的添加和删除类名即可 那能不能不自己写动态,就靠vue 首先我们要靠<transition>标签把需要动画的包裹起来 vue中…...
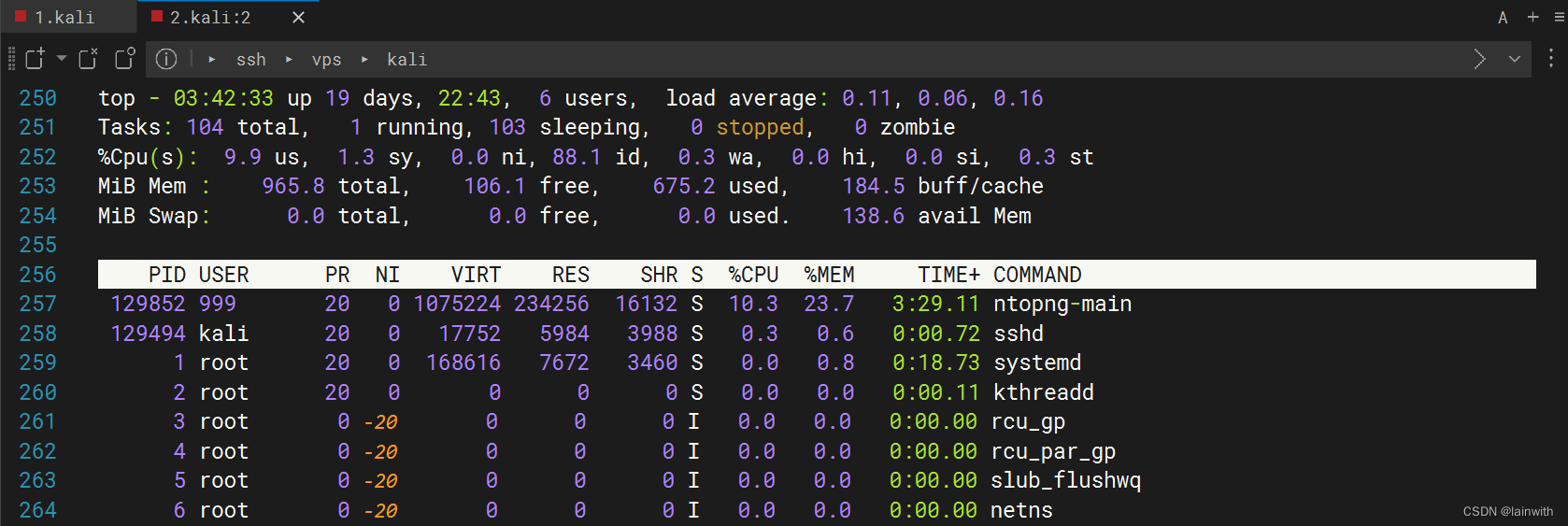
流量监控-ntopng
目录介绍安装使用介绍 ntopng是原始ntop的下一代版本,ntop是监视网络使用情况的网络流量探测器。ntopng基于libpcap,并且以可移植的方式编写,以便实际上可以在每个Unix平台,MacOSX和Windows上运行。 ntopng(是的&…...
C++ 21 set容器
目录 一、set容器 1.1 简介 1.2 构造和赋值 1.3 大小和交换 1.4 插入和删除 1.5 查找和统计 1.6 set和multiset区别 1.7 内置类型指定排序规则 1.8 自定义数据类型指定排序规则 一、set容器 1.1 简介 ① set容器中所有元素在插入时自动被排序。 ② set容器和multise…...
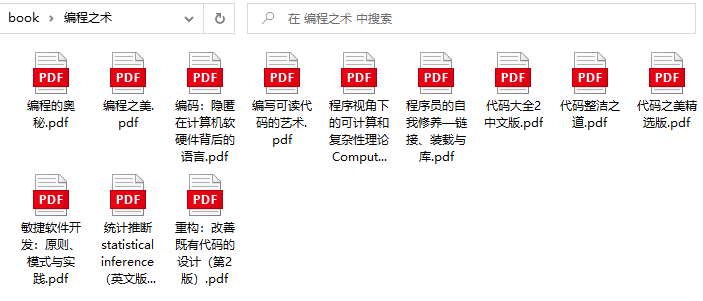
什么是JWT
JSON Web Token(缩写 JWT)是目前最流行的跨域认证解决方案。 传统的session认证 http协议本身是一种无状态的协议,而这就意味着如果用户向我们的应用提供了用户名和密码来进行用户认证,那么下一次请求时,用户还要再一…...
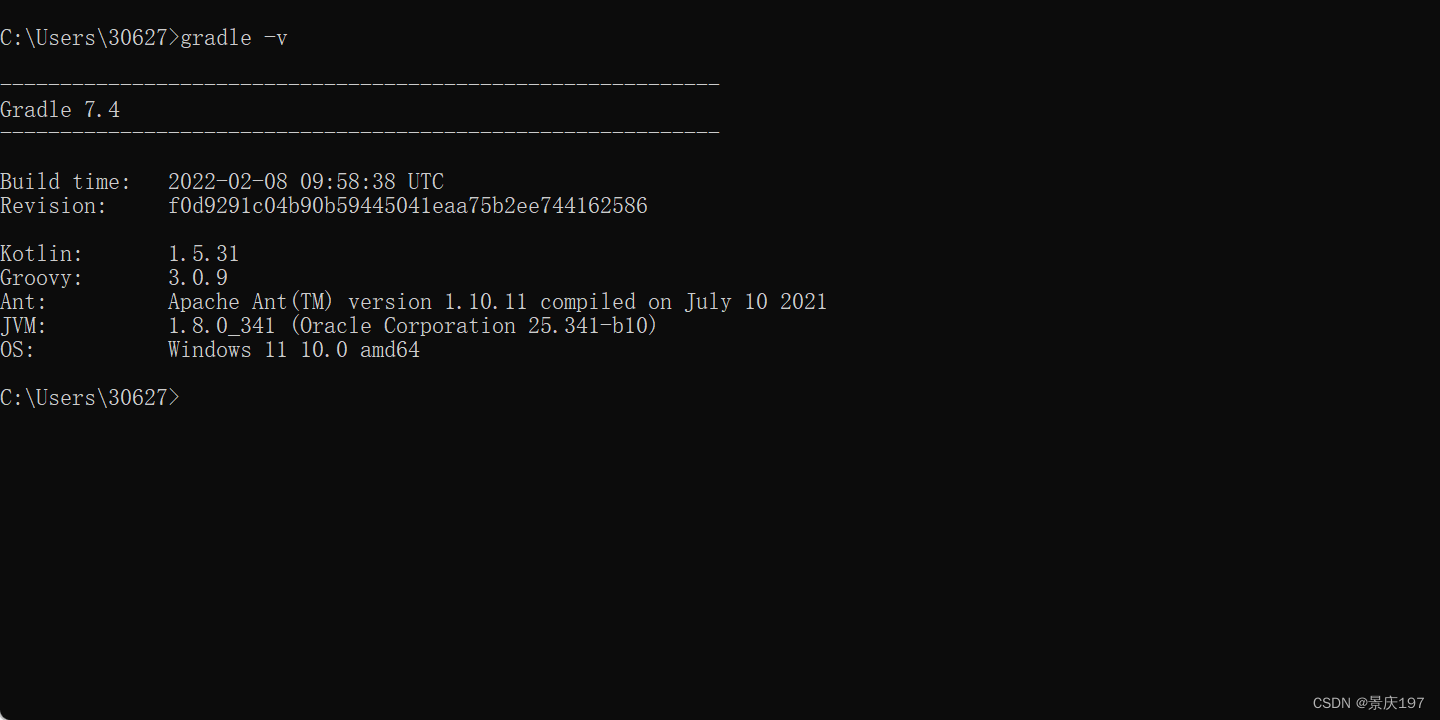
Gradle7.4安装
前置:本文基于IntelliJ IDEA 2022.2.1 、jdk1.8进行安装 目录 1.挑选Gradle版本 2.系统变量设置 1.挑选Gradle版本 gradle兼容性差, 1.跟idea会有版本问题。 2.跟springboot也有兼容问题Spring Boot Gradle Plugin Reference Guide 首先查询版本&…...
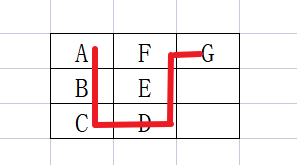
【华为OD机试 2023最新 】 箱子之字形摆放(C++ 100%)
文章目录 题目描述输入描述输出描述备注用例题目解析C++题目描述 有一批箱子(形式为字符串,设为str), 要求将这批箱子按从上到下以之字形的顺序摆放在宽度为 n 的空地,请输出箱子的摆放位置。 例如:箱子ABCDEFG,空地宽度为3,摆放结果如图: 则输出结果为: AFG BE CD …...
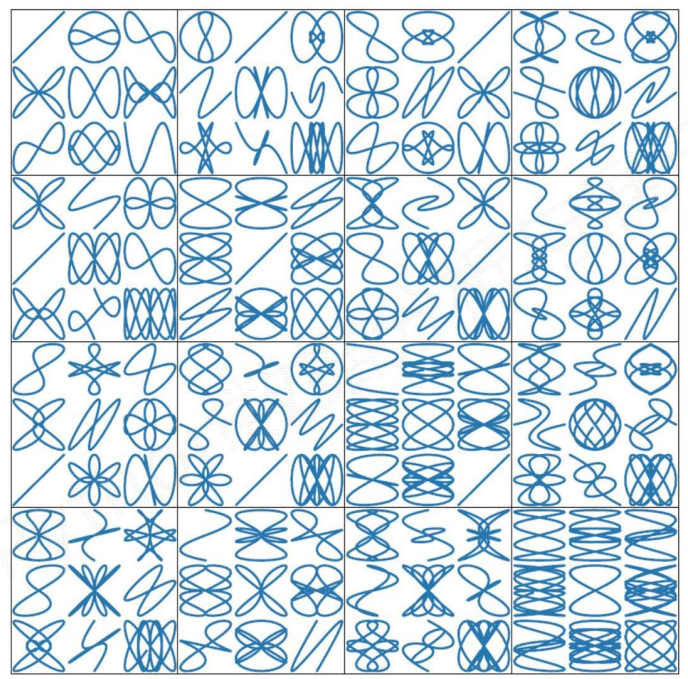
Matplotlib库入门
Matplotlib库的介绍 什么是Matplotlib库? Matplotlib是一个Python的数据可视化库,用于绘制各种类型的图表,包括线图、散点图、条形图、等高线图、3D图等等。它是一个非常强大和灵活的库,被广泛用于数据科学、机器学习、工程学、…...
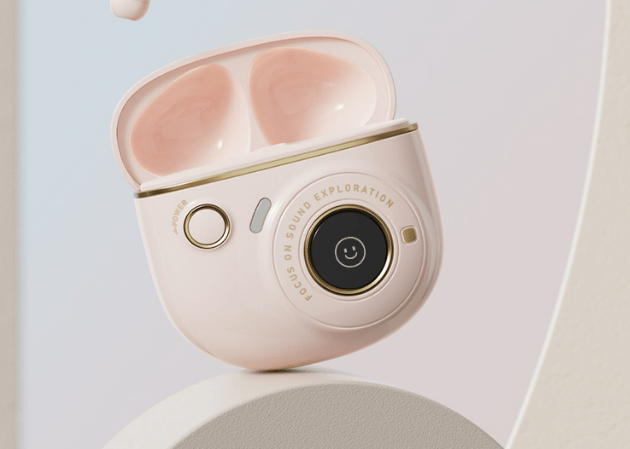
学生党用什么蓝牙耳机比较好?300内高性价比蓝牙耳机排行
随着蓝牙技术的发展,蓝牙耳机越来越普及,不同价位、不同性能的蓝牙耳机数不胜数。那么,学生党用什么蓝牙耳机比较好?下面,我来给大家推荐几款三百内高性价比蓝牙耳机,一起来看看吧。 一、南卡小音舱蓝牙耳…...
Lambda 表达式与函数式接口
函数式接口 如果一个接口,只有一个抽象方法,该接口即为函数式接口。函数式接口,即可使用 Lambda 表达式。 如下面的接口 public interface Translate {void translate();}目前该接口的抽象方法为无参数无返回值 Lambda 表达式 无参无返回值…...
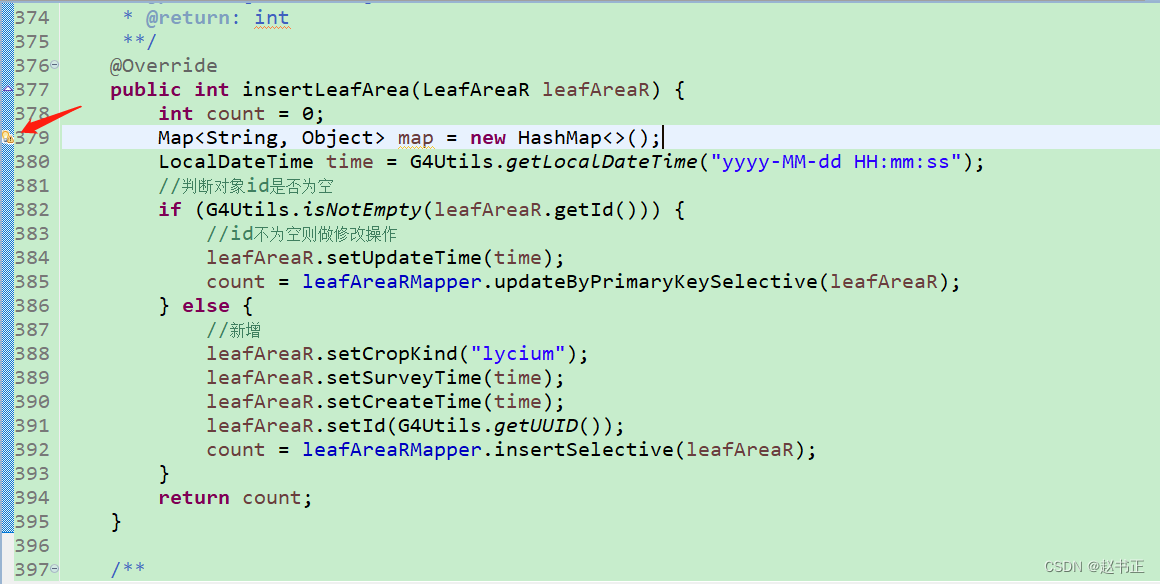
后端代码规范
1、报文入参尽量避免使用实体类(如果用实体类接受参数,一定要写好注解,具体用到了实体类的哪一个属性) /*** * Description: 新增玉米观测记录主表信息* param param params* param return 参数* return Result 返回类型* author…...
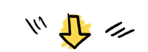
web自动化测试:Selenium+Python基础方法封装(建议收藏)
01、目的 web自动化测试作为软件自动化测试领域中绕不过去的一个“香饽饽”,通常都会作为广大测试从业者的首选学习对象,相较于C/S架构的自动化来说,B/S有着其无法忽视的诸多优势,从行业发展趋、研发模式特点、测试工具支持&…...
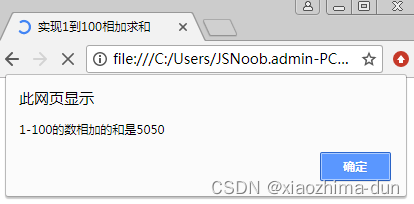
while实现1到100相加求和-课后程序(JavaScript前端开发案例教程-黑马程序员编著-第2章-课后作业)
【案例2-7】while实现1到100相加求和 一、案例描述 考核知识点 while循环语句 练习目标 掌握while循环语句。 需求分析 1-100之间的数相加求和,本案例通过while循环语句来实现。 案例分析 效果如图2-10所示。1-100所有数的和 具体实现步骤如下: 在&l…...
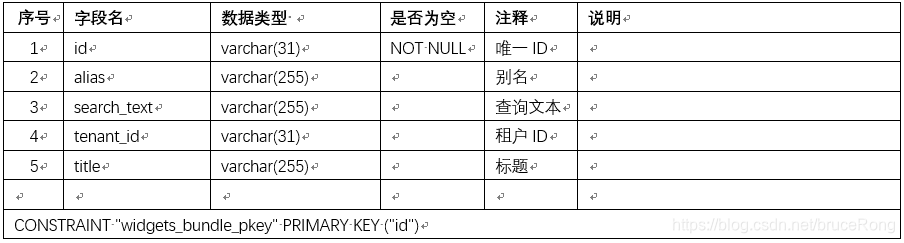
Thingsboard(2.4 postgresql版)数据库表结构说明
本文描述的表结构是根据thingsboard2.4(postgresql版)数据库中整理出来的,不一定完整,后续有新的发现再补充文档。 一、数据库E-R关系 Thingsboard2.4社区版共22个表,主要包括实体信息表、关系信息表、字典表和系统配…...
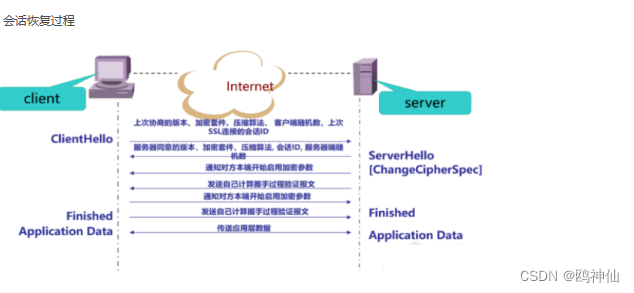
IDS反病毒与APT的具体介绍
文章目录一,IDS1. 什么是IDS?2. IDS和防火墙有什么不同?3. IDS工作原理?4. IDS的主要检测方法有哪些详细说明?5. IDS的部署方式有哪些?6. IDS的签名是什么意思?签名过滤器有什么作用?…...
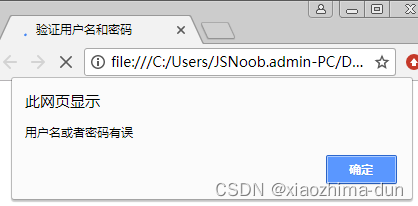
while do..while验证用户名和密码-课后程序(JavaScript前端开发案例教程-黑马程序员编著-第2章-课后作业)
【案例2-8】while do..while验证用户名和密码 一、案例描述 考核知识点 while、do…while循环语句 练习目标 掌握while语句。do…while循环语句。 需求分析 在网站上登录时会用到表单,让用户属于用户名和密码,输入正确才可以进入,本案例将…...

19c补丁后oracle属主变化,导致不能识别磁盘组
补丁后服务器重启,数据库再次无法启动 ORA01017: invalid username/password; logon denied Oracle 19c 在打上 19.23 或以上补丁版本后,存在与用户组权限相关的问题。具体表现为,Oracle 实例的运行用户(oracle)和集…...
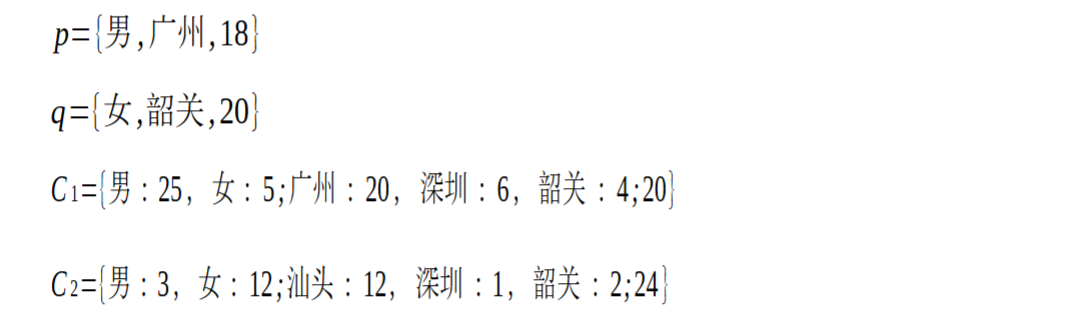
SCAU期末笔记 - 数据分析与数据挖掘题库解析
这门怎么题库答案不全啊日 来简单学一下子来 一、选择题(可多选) 将原始数据进行集成、变换、维度规约、数值规约是在以下哪个步骤的任务?(C) A. 频繁模式挖掘 B.分类和预测 C.数据预处理 D.数据流挖掘 A. 频繁模式挖掘:专注于发现数据中…...
如何为服务器生成TLS证书
TLS(Transport Layer Security)证书是确保网络通信安全的重要手段,它通过加密技术保护传输的数据不被窃听和篡改。在服务器上配置TLS证书,可以使用户通过HTTPS协议安全地访问您的网站。本文将详细介绍如何在服务器上生成一个TLS证…...
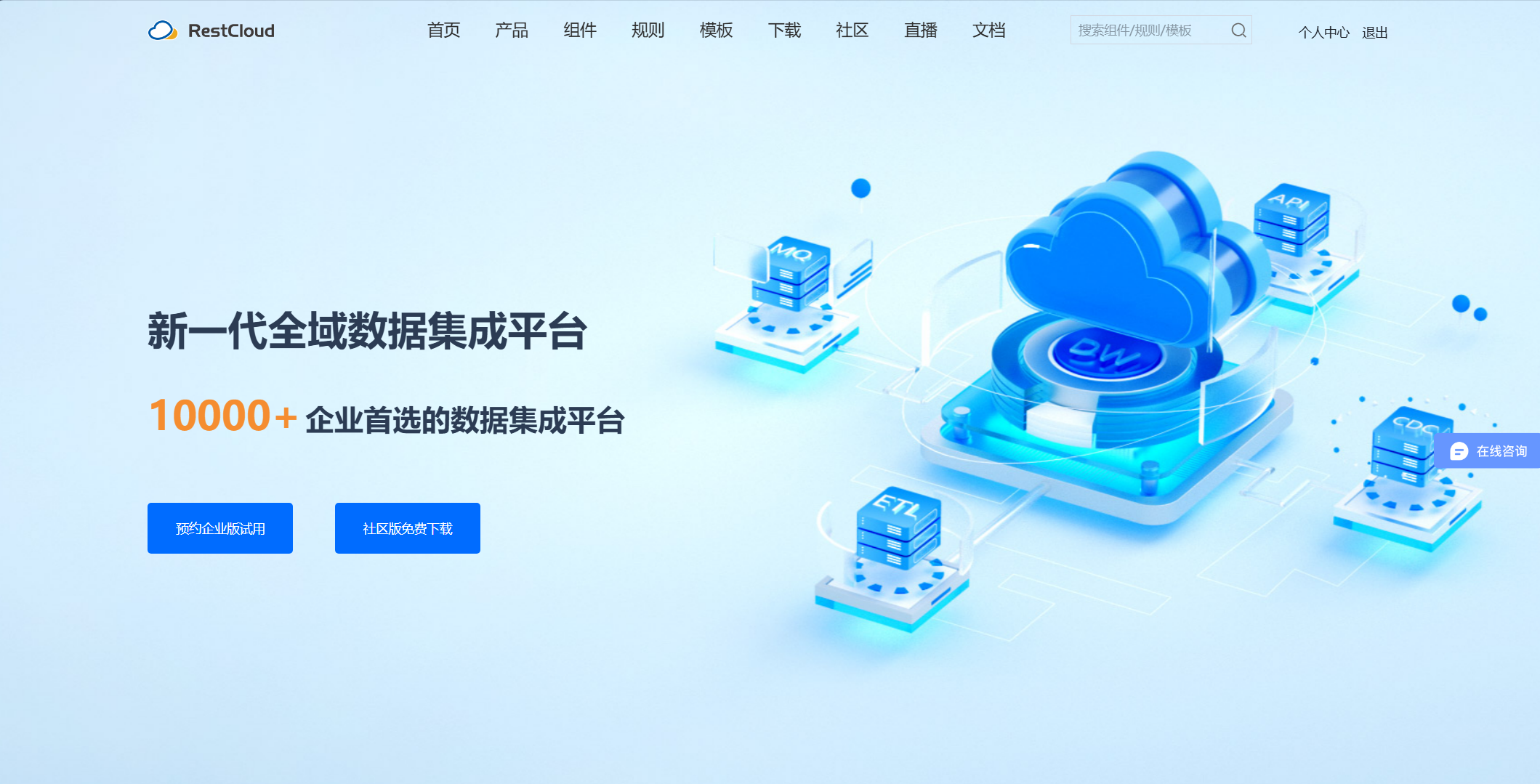
ETLCloud可能遇到的问题有哪些?常见坑位解析
数据集成平台ETLCloud,主要用于支持数据的抽取(Extract)、转换(Transform)和加载(Load)过程。提供了一个简洁直观的界面,以便用户可以在不同的数据源之间轻松地进行数据迁移和转换。…...
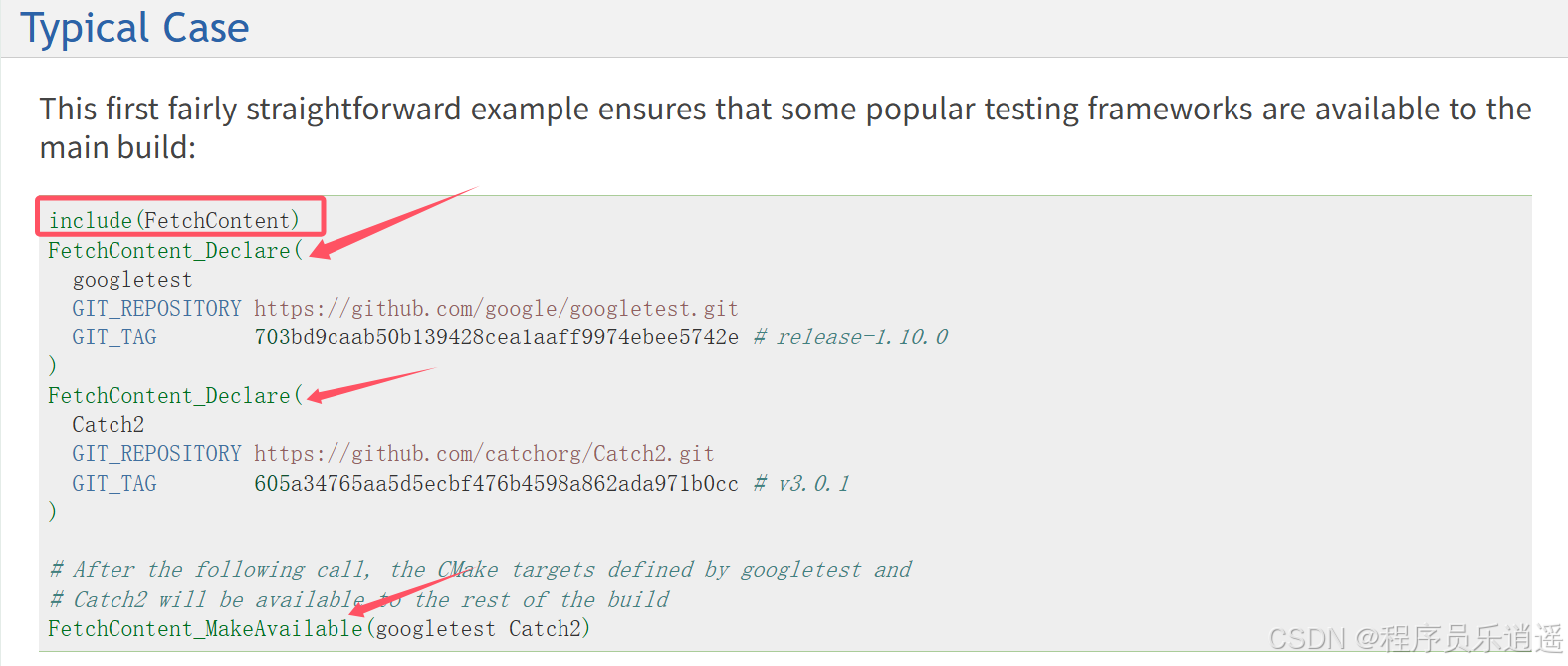
CMake 从 GitHub 下载第三方库并使用
有时我们希望直接使用 GitHub 上的开源库,而不想手动下载、编译和安装。 可以利用 CMake 提供的 FetchContent 模块来实现自动下载、构建和链接第三方库。 FetchContent 命令官方文档✅ 示例代码 我们将以 fmt 这个流行的格式化库为例,演示如何: 使用 FetchContent 从 GitH…...
【HTTP三个基础问题】
面试官您好!HTTP是超文本传输协议,是互联网上客户端和服务器之间传输超文本数据(比如文字、图片、音频、视频等)的核心协议,当前互联网应用最广泛的版本是HTTP1.1,它基于经典的C/S模型,也就是客…...
MySQL用户和授权
开放MySQL白名单 可以通过iptables-save命令确认对应客户端ip是否可以访问MySQL服务: test: # iptables-save | grep 3306 -A mp_srv_whitelist -s 172.16.14.102/32 -p tcp -m tcp --dport 3306 -j ACCEPT -A mp_srv_whitelist -s 172.16.4.16/32 -p tcp -m tcp -…...
Java求职者面试指南:计算机基础与源码原理深度解析
Java求职者面试指南:计算机基础与源码原理深度解析 第一轮提问:基础概念问题 1. 请解释什么是进程和线程的区别? 面试官:进程是程序的一次执行过程,是系统进行资源分配和调度的基本单位;而线程是进程中的…...
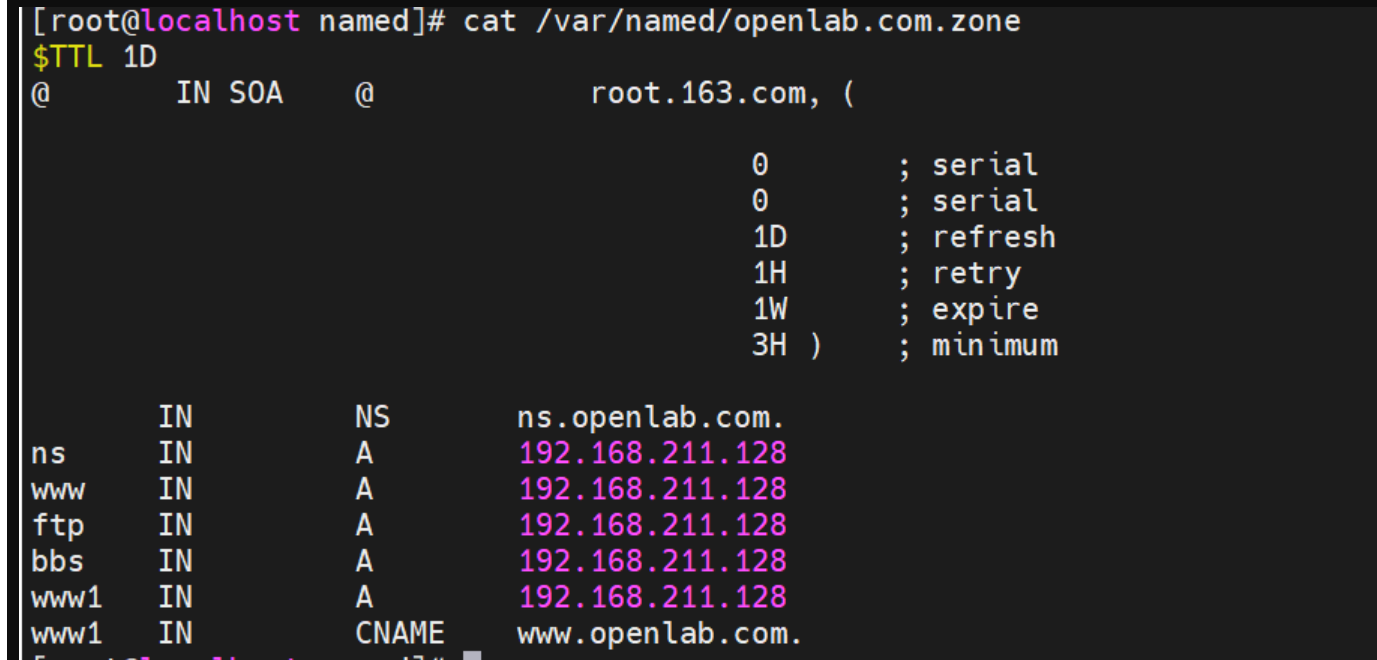
搭建DNS域名解析服务器(正向解析资源文件)
正向解析资源文件 1)准备工作 服务端及客户端都关闭安全软件 [rootlocalhost ~]# systemctl stop firewalld [rootlocalhost ~]# setenforce 0 2)服务端安装软件:bind 1.配置yum源 [rootlocalhost ~]# cat /etc/yum.repos.d/base.repo [Base…...
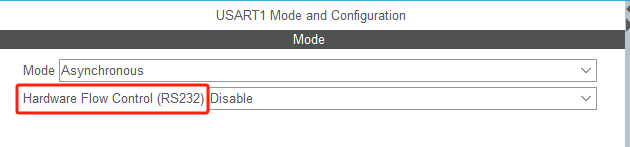
STM32HAL库USART源代码解析及应用
STM32HAL库USART源代码解析 前言STM32CubeIDE配置串口USART和UART的选择使用模式参数设置GPIO配置DMA配置中断配置硬件流控制使能生成代码解析和使用方法串口初始化__UART_HandleTypeDef结构体浅析HAL库代码实际使用方法使用轮询方式发送使用轮询方式接收使用中断方式发送使用中…...