Reinforcement Learning with Code 【Chapter 8. Value Funtion Approximation】
Reinforcement Learning with Code
This note records how the author begin to learn RL. Both theoretical understanding and code practice are presented. Many material are referenced such as ZhaoShiyu’s Mathematical Foundation of Reinforcement Learning, .
文章目录
- Reinforcement Learning with Code
- Chapter 8. Value Funtion Approximation
- 8.1 State value: MC/TD learning with function approximation
- 8.2 Action value: Sarsa with funtion approximation
- 8.3 Optimal action value: Q-learning with function approximation
- 8.4 Deep Q-learning (DQN)
- Reference
Chapter 8. Value Funtion Approximation
As so far in this note, state and action values are represented in tabular fashion. There are two problems that first although tabular representation is intuitive, it would encounter some problems when the state action space is large. Second since the value of a state is updated only if it is visited, the values of unvisited states cannot be estimated.
We can sovle the problems using a parameterized function v ^ ( s , w ) \hat{v}(s,w) v^(s,w) to approximate the value funtion, where w ∈ R m w\in\mathbb{R}^m w∈Rm is the parameter vector. On the one hand, we only need to store the parameter w w w instead of all states s s s, which is much smaller. On the other hand, when a state s s s is visited, the parameter w w w is updated so that the values of some other unvisited states can also be estimated.
We usually use neural network to approximate the value function. This problem basically is a regression problem. We can find the optimal parameter w w w by minimize some objective funtions, which we will introduce next.
8.1 State value: MC/TD learning with function approximation
Let v π ( s ) v_\pi(s) vπ(s) and v ^ ( s , w ) \hat{v}(s,w) v^(s,w) be the true value and approximated state value of s ∈ S s\in\mathcal{S} s∈S. The objective funtion considered in value function approximation is usually
J ( w ) = E [ ( v π ( S ) − v ^ ( S , w ) ) 2 ] \textcolor{red}{J(w) = \mathbb{E}\Big[ \big( v_\pi(S)-\hat{v}(S,w) \big)^2 \Big]} J(w)=E[(vπ(S)−v^(S,w))2]
which is also called mean square error in deep learning field. Where S , S ′ S,S^\prime S,S′ denote random variable of state s s s.
Next, we discuss the distribution of random variable S S S. We often use the stationary distribution, which describeds the long-run behavior of a Markov process. Let { d π ( s ) } s ∈ S \textcolor{blue}{\{d_\pi(s)\}_{s\in\mathcal{S}}} {dπ(s)}s∈S donte the stationary distribution of random variable S S S. By definition, d π ( s ) ≥ 0 d_\pi(s)\ge 0 dπ(s)≥0 and ∑ s ∈ S d π ( s ) = 1 \sum_{s\in\mathcal{S}} d_\pi(s)=1 ∑s∈Sdπ(s)=1. Hence, the objective funciton can be rewritten as
J ( w ) = ∑ s ∈ S d π ( s ) [ v π ( s ) − v ^ ( s , w ) ] 2 \textcolor{red}{J(w) = \sum_{s\in\mathcal{S}} d_\pi(s) \Big[v_\pi(s)-\hat{v}(s,w) \Big]^2} J(w)=s∈S∑dπ(s)[vπ(s)−v^(s,w)]2
This objective function is a weighted squared error. How to compute the stationary distribution of random variable S S S? We often use the equation
d π T = d π T P π d_\pi^T = d_\pi^T P_\pi dπT=dπTPπ
As a result, d π d_\pi dπ is the left eigenvector of P π P_\pi Pπ associated with the eigenvalue of 1 1 1. The proof is omitted.
Recall the spirit of gradient descent (GD). We can use it to minimize the objective function as
w k + 1 = w k − α k ∇ w E [ ( v π ( S ) − v ^ ( S , w ) ) 2 ] = w k − α k E [ ∇ w ( v π ( S ) − v ^ ( S , w ) ) 2 ] = w k − 2 α k E [ ( v π ( S ) − v ^ ( S , w ) ) ∇ w ( − v ^ ( S , w ) ) ] = w k + 2 α k E [ v π ( S ) − v ^ ( S , w ) ∇ w v ^ ( S , w ) ] \begin{aligned} w_{k+1} & = w_k - \alpha_k \nabla_w \mathbb{E}\Big[ (v_\pi(S)-\hat{v}(S,w))^2 \Big] \\ & = w_k - \alpha_k \mathbb{E} \Big[ \nabla_w(v_\pi(S)-\hat{v}(S,w))^2 \Big] \\ & = w_k - 2\alpha_k \mathbb{E}\Big[ (v_\pi(S)-\hat{v}(S,w)) \nabla_w(-\hat{v}(S,w)) \Big] \\ & = w_k + 2\alpha_k \mathbb{E} \Big[v_\pi(S)-\hat{v}(S,w) \nabla_w\hat{v}(S,w) \Big] \end{aligned} wk+1=wk−αk∇wE[(vπ(S)−v^(S,w))2]=wk−αkE[∇w(vπ(S)−v^(S,w))2]=wk−2αkE[(vπ(S)−v^(S,w))∇w(−v^(S,w))]=wk+2αkE[vπ(S)−v^(S,w)∇wv^(S,w)]
where without loss of generality the cofficient 2 before α k \alpha_k αk can be dropped. By the spirit of stochastic gradient descent (SGD), we can remove the expectation operation to obtain
w t + 1 = w t + a t ( v π ( s t ) − v ^ ( s t , w t ) ) ∇ w v ^ ( s t , w t ) w_{t+1} = w_t + a_t (v_\pi(s_t) - \hat{v}(s_t,w_t))\nabla_w \hat{v}(s_t,w_t) wt+1=wt+at(vπ(st)−v^(st,wt))∇wv^(st,wt)
However this equation can’t be implemented. Because it requires the true state value v π v_\pi vπ, which is the unknown to be esitmated. Hence, we can use the idea of Monte Carlo or TD learning to estimate it.
By Monte Carlo learning spirit, we can use the g t g_t gt to denote the discounted return that
g t = r t + 1 + γ r t + 2 + γ 2 r t + 3 + ⋯ g_t = r_{t+1} + \gamma r_{t+2} + \gamma^2 r_{t+3} + \cdots gt=rt+1+γrt+2+γ2rt+3+⋯
Then, g t g_t gt can be used as an approximation of v π ( s ) v_\pi(s) vπ(s). The algorithm becomes
w t + 1 = w t + a t ( g t − v ^ ( s t , w t ) ) ∇ w v ^ ( s t , w t ) w_{t+1} = w_t + a_t (\textcolor{red}{g_t} - \hat{v}(s_t,w_t))\nabla_w \hat{v}(s_t,w_t) wt+1=wt+at(gt−v^(st,wt))∇wv^(st,wt)
By TD learning spirit, we can use the r t + 1 + γ v ^ ( s t + 1 , w t ) r_{t+1}+\gamma \hat{v}(s_{t+1},w_t) rt+1+γv^(st+1,wt) as the approximation of v π ( s ) v_\pi(s) vπ(s). The algorithm becomes
w t + 1 = w t + a t ( r t + 1 + γ v ^ ( s t + 1 , w ) − v ^ ( s t , w t ) ) ∇ w v ^ ( s t , w t ) w_{t+1} = w_t + a_t (\textcolor{red}{r_{t+1}+\gamma \hat{v}(s_{t+1},w)} - \hat{v}(s_t,w_t))\nabla_w \hat{v}(s_t,w_t) wt+1=wt+at(rt+1+γv^(st+1,w)−v^(st,wt))∇wv^(st,wt)
Pesudocode:
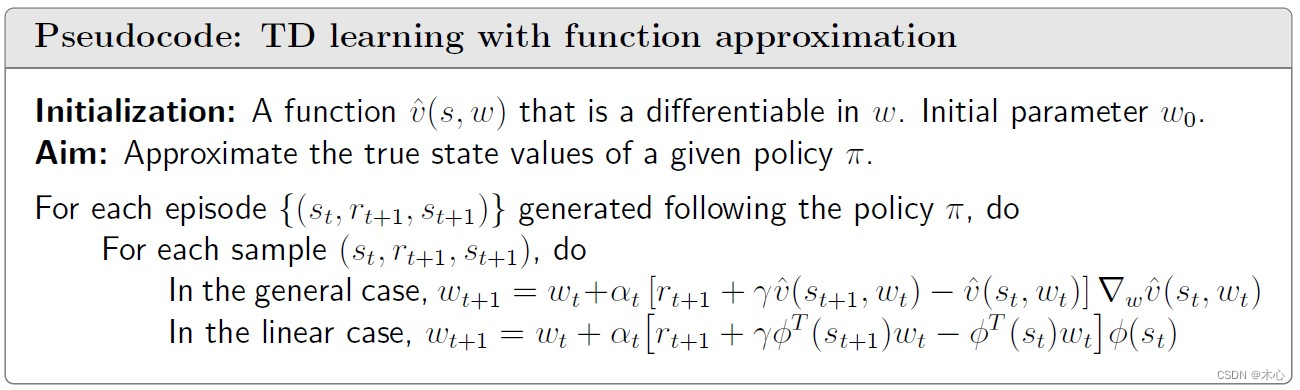
8.2 Action value: Sarsa with funtion approximation
To seach for optimal policies, we need to estimate action values. This section introduces how to estimate action values using Sarsa in the presence of value function approximation.
The action value q π ( s , a ) q_\pi(s,a) qπ(s,a) is described by a function q ^ ( s , a , w ) \hat{q}(s,a,w) q^(s,a,w) parameterized by w w w. The objective funtion considered in action value approximation is usually selected as
J ( w ) = E [ ( q π ( S , A ) − q ^ ( S , A , w ) ) 2 ] \textcolor{red}{J(w) = \mathbb{E}[(q_\pi(S,A) - \hat{q}(S,A,w))^2]} J(w)=E[(qπ(S,A)−q^(S,A,w))2]
Use the stochasitic gradient descent to minimize the objective function
w k + 1 = w k − α k ∇ w E [ ( q π ( S , A ) − q ^ ( S , A , w ) ) 2 ] = w k + 2 a k E [ q π ( S , A ) − q ^ ( S , A , w ) ] ∇ w q ^ ( S , A , w ) = w k + α k ( q π ( s , a ) − q ^ ( s , a , w ) ) ∇ w q ^ ( s , a , w ) \begin{aligned} w_{k+1} & = w_k - \alpha_k \nabla_w \mathbb{E}[(q_\pi(S,A) - \hat{q}(S,A,w))^2] \\ & = w_k + 2a_k \mathbb{E}[q_\pi(S,A) - \hat{q}(S,A,w)]\nabla_w \hat{q}(S,A,w) \\ & = w_k + \alpha_k (q_\pi(s,a) - \hat{q}(s,a,w))\nabla_w\hat{q}(s,a,w) \end{aligned} wk+1=wk−αk∇wE[(qπ(S,A)−q^(S,A,w))2]=wk+2akE[qπ(S,A)−q^(S,A,w)]∇wq^(S,A,w)=wk+αk(qπ(s,a)−q^(s,a,w))∇wq^(s,a,w)
where without loss of generality the cofficient 2 before α k \alpha_k αk can be dropped.
By Sarsa spirit, we use the r + γ q ^ ( s ′ , a ′ , w ) r+\gamma \hat{q}(s^\prime,a^\prime,w) r+γq^(s′,a′,w) to approximate ture action value q π ( s , a ) q_\pi(s,a) qπ(s,a). Hence we have
w k + 1 = w k + α k ( r + γ q ^ ( s ′ , a ′ , w ) − q ^ ( s , a , w ) ) ∇ w q ^ ( s , a , w ) w_{k+1} = w_k + \alpha_k (r+\gamma \hat{q}(s^\prime,a^\prime,w) - \hat{q}(s,a,w))\nabla_w\hat{q}(s,a,w) wk+1=wk+αk(r+γq^(s′,a′,w)−q^(s,a,w))∇wq^(s,a,w)
The sampled data ( s , a , r k , s k ′ , a k ′ ) (s,a,r_k,s^\prime_k,a^\prime_k) (s,a,rk,sk′,ak′) is changed to ( s t , a t , r t + 1 , s t + 1 , a t + 1 ) (s_t,a_t,r_{t+1},s_{t+1},a_{t+1}) (st,at,rt+1,st+1,at+1). Hence
w t + 1 = w t + α t [ r t + 1 + γ q ^ ( s t + 1 , a t + 1 , w t ) − q ^ ( s t , a t , w t ) ] ∇ w q ^ ( s t , a t , w t ) w_{t+1} = w_t + \alpha_t \Big[ \textcolor{red}{r_{t+1}+\gamma \hat{q}(s_{t+1},a_{t+1},w_t)} - \hat{q}(s_t,a_t,w_t) \Big]\nabla_w \hat{q}(s_t,a_t,w_t) wt+1=wt+αt[rt+1+γq^(st+1,at+1,wt)−q^(st,at,wt)]∇wq^(st,at,wt)
Pseudocode:
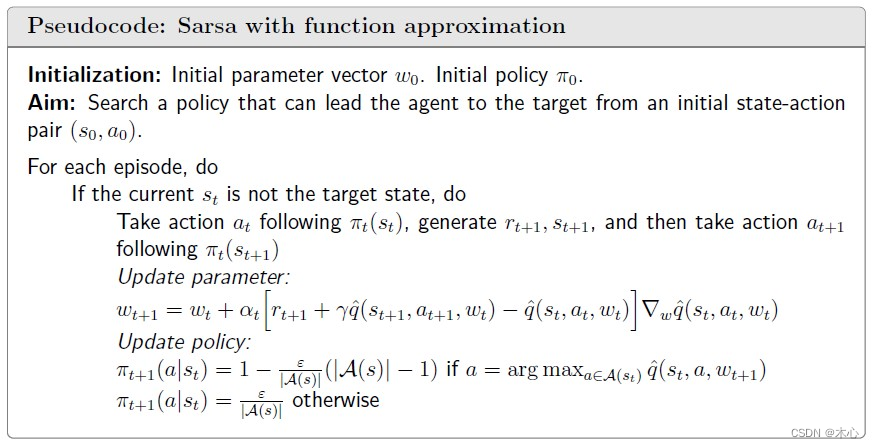
8.3 Optimal action value: Q-learning with function approximation
Similar to Sarsa, tabular Q-learning can also be extended to the case of value function approximation.
By the spirit of Q-learning, the update rule is
w t + 1 = w t + α t [ r t + 1 + γ max a ∈ A ( s t + 1 ) q ^ ( s t + 1 , a , w t ) − q ^ ( s t , a t , w t ) ] ∇ w q ^ ( s t , a t , w t ) w_{t+1} = w_t + \alpha_t \Big[ \textcolor{red}{r_{t+1}+\gamma \max_{a\in\mathcal{A}(s_{t+1})} \hat{q}(s_{t+1},a,w_t)} - \hat{q}(s_t,a_t,w_t) \Big]\nabla_w \hat{q}(s_t,a_t,w_t) wt+1=wt+αt[rt+1+γa∈A(st+1)maxq^(st+1,a,wt)−q^(st,at,wt)]∇wq^(st,at,wt)
which is the same as Sarsa expect that q ^ ( s t + 1 , a t + 1 , w t ) \hat{q}(s_{t+1},a_{t+1},w_t) q^(st+1,at+1,wt) is replaced by max a ∈ A ( s t + 1 ) q ^ ( s t + 1 , a , w t ) \max_{a\in\mathcal{A}(s_{t+1})} \hat{q}(s_{t+1},a,w_t) maxa∈A(st+1)q^(st+1,a,wt).
Pseudocode:
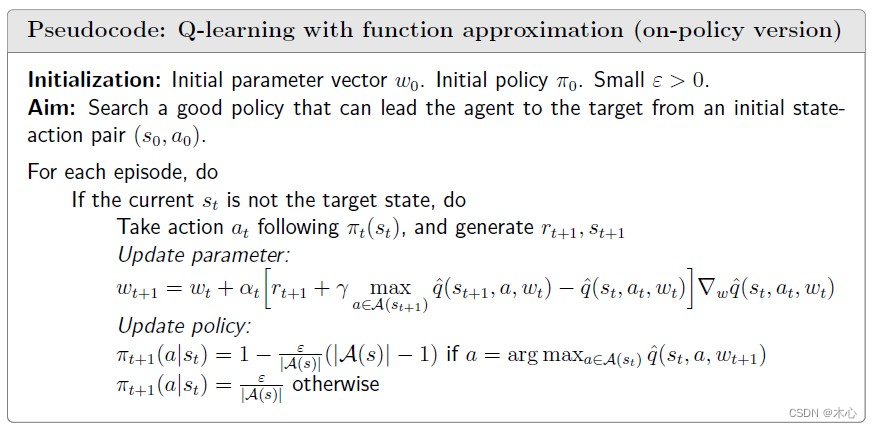
8.4 Deep Q-learning (DQN)
We can introduce deep neural networks into Q-learning to obtain deep Q-learning or deep Q-network (DQN).
Mathematically, deep Q-learning aims to minimize the objective funtion
J ( w ) = E [ ( R + γ max a ∈ A ( S ′ ) q ^ ( S ′ , a , w ) − q ^ ( S , A , w ) ) 2 ] \textcolor{red}{J(w) = \mathbb{E} \Big[ \Big( R+\gamma \max_{a\in\mathcal{A}(S^\prime)} \hat{q}(S^\prime, a, w) - \hat{q}(S,A,w) \Big)^2 \Big]} J(w)=E[(R+γa∈A(S′)maxq^(S′,a,w)−q^(S,A,w))2]
where ( S , A , R , S ′ ) (S,A,R,S^\prime) (S,A,R,S′) are random variables representing a state, an action taken at that state, the immediate reward, and the next state. This objective funtion can be viewed as the Bellman opitmality error. That is because
q ( s , a ) = E [ R t + 1 + γ max a ∈ A ( S t + 1 ) q ( S t + 1 , a ) ∣ S t = s , A t = a ] q(s,a) = \mathbb{E} \Big[ R_{t+1} + \gamma \max_{a\in\mathcal{A}(S_{t+1})} q(S_{t+1},a) | S_t = s, A_t= a \Big] q(s,a)=E[Rt+1+γa∈A(St+1)maxq(St+1,a)∣St=s,At=a]
is the Bellman optimality equation in terms of action value.
Then we can use the stochastic gradient to minimize the objective funtion. However, it is noted that the parameter w w w not only apperas in q ^ ( S , A , w ) \hat{q}(S,A,w) q^(S,A,w) but also in y ≜ R + γ max a ∈ A ( S ′ ) q ^ ( S ′ , a , w ) y\triangleq R+\gamma \max_{a\in\mathcal{A}(S^\prime)}\hat{q}(S^\prime,a,w) y≜R+γmaxa∈A(S′)q^(S′,a,w). For the sake of simplicity, we can assume that w w w in y y y is fixed (at least for a while) when we calculate the gradient. To do that, we can introduce two networks. One is a *main networ*k representing q ^ ( s , a , w ) \hat{q}(s,a,w) q^(s,a,w) and the other is a target network q ^ ( s , a , w T ) \hat{q}(s,a,w_T) q^(s,a,wT). The objective function in this case degenerates to
J ( w ) = E [ ( R + γ max a ∈ A ( S ′ ) q ^ ( S ′ , a , w T ) − q ^ ( S , A , w ) ) 2 ] J(w) = \mathbb{E} \Big[ \Big( R+\gamma \max_{a\in\mathcal{A}(S^\prime)} \hat{q}(S^\prime, a, w_T) - \hat{q}(S,A,w) \Big)^2 \Big] J(w)=E[(R+γa∈A(S′)maxq^(S′,a,wT)−q^(S,A,w))2]
where w T w_T wT is the target network parameter and w w w is the main network parameter. When w T w_T wT is fixed, the gradient of J ( w ) J(w) J(w) is
∇ w J = − 2 ∗ E [ ( R + γ max a ∈ A ( S ′ ) q ^ ( S ′ , a , w T ) − q ^ ( S ′ , A , w ) ) ∇ w q ^ ( S , A , w ) ] \nabla_{w}J = -2*\mathbb{E} \Big[ \Big( R + \gamma \max_{a\in\mathcal{A}(S^\prime)} \hat{q}(S^\prime,a,w_T) - \hat{q}(S^\prime,A,w) \Big) \nabla_{w}\hat{q}(S,A,w) \Big] ∇wJ=−2∗E[(R+γa∈A(S′)maxq^(S′,a,wT)−q^(S′,A,w))∇wq^(S,A,w)]
There are two techniques should be noticed.
When the target network is fixed, using stochastic gradient descent (SGD) we can obtain
w t + 1 = w t + α t [ r t + 1 + γ max a ∈ A ( s t + 1 ) q ^ ( s t + 1 , a , w T ) − q ^ ( s t , a t , w ) ] ∇ w q ^ ( s t , a t , w ) \textcolor{red}{w_{t+1} = w_{t} + \alpha_t \Big[ r_{t+1} + \gamma \max_{a\in\mathcal{A}(s_{t+1})} \hat{q}(s_{t+1},a,w_T) - \hat{q}(s_t,a_t,w) \Big] \nabla_w \hat{q}(s_t,a_t,w)} wt+1=wt+αt[rt+1+γa∈A(st+1)maxq^(st+1,a,wT)−q^(st,at,w)]∇wq^(st,at,w)
One technique is experience replay. This is, after we have collected some experience samples, we don’t use these samples in the order they were collected. Instead, we store them in a data set, called replay buffer, and draw a batch of samples randomly to train the neureal network. In particular, let ( s , a , r , s ′ ) (s,a,r,s^\prime) (s,a,r,s′) be an experience sample and B ≜ { ( s , a , r , s ′ ) } \mathcal{B}\triangleq \{(s,a,r,s^\prime) \} B≜{(s,a,r,s′)} be the replay buffer. Every time we train the neural network, we can draw a mini-batch of random samples from the reply buffer. The draw of samples, or called experience replay, should follow a uniform distribution.
Another technique is use two networks. A main network parameterized by w w w, and a target network parameterized by w T w_T wT. The two network parameters are set to be the same initially. The target output is y T ≜ r + γ max a ∈ A ( s ′ ) q ^ ( s ′ , a , w T ) y_T \triangleq r + \gamma \max_{a\in\mathcal{A}(s^\prime)}\hat{q}(s^\prime,a,w_T) yT≜r+γmaxa∈A(s′)q^(s′,a,wT). Then, we directly minimize the TD error or called loss function ( y − q ^ ( s , a , w , ) ) 2 (y-\hat{q}(s,a,w,))^2 (y−q^(s,a,w,))2 over the mini-batch { ( s , a , y T ) } \{(s,a,y_T)\} {(s,a,yT)} instead of a single sample to improve efficiency and stability.
The parameter of the main network is updated in every interation. By contrast, the target network is set to be the same as the main network every a certain number of iterations to meet the assumption that w T w_T wT is fixed when calculating the gradient.
Pseudocode:
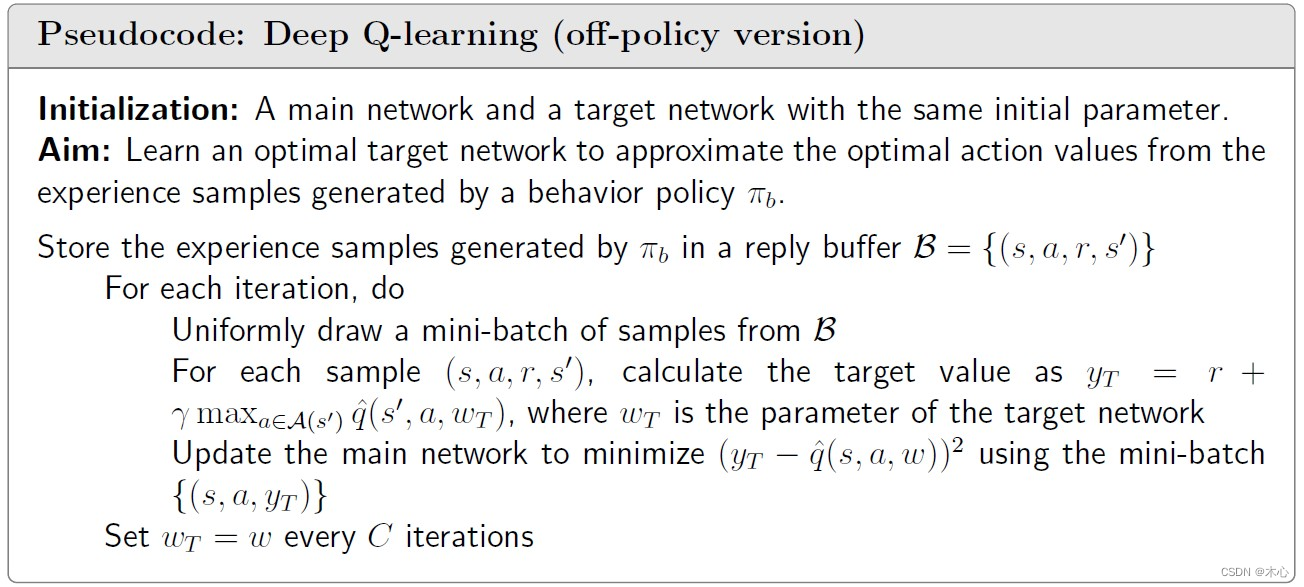
Reference
赵世钰老师的课程
相关文章:
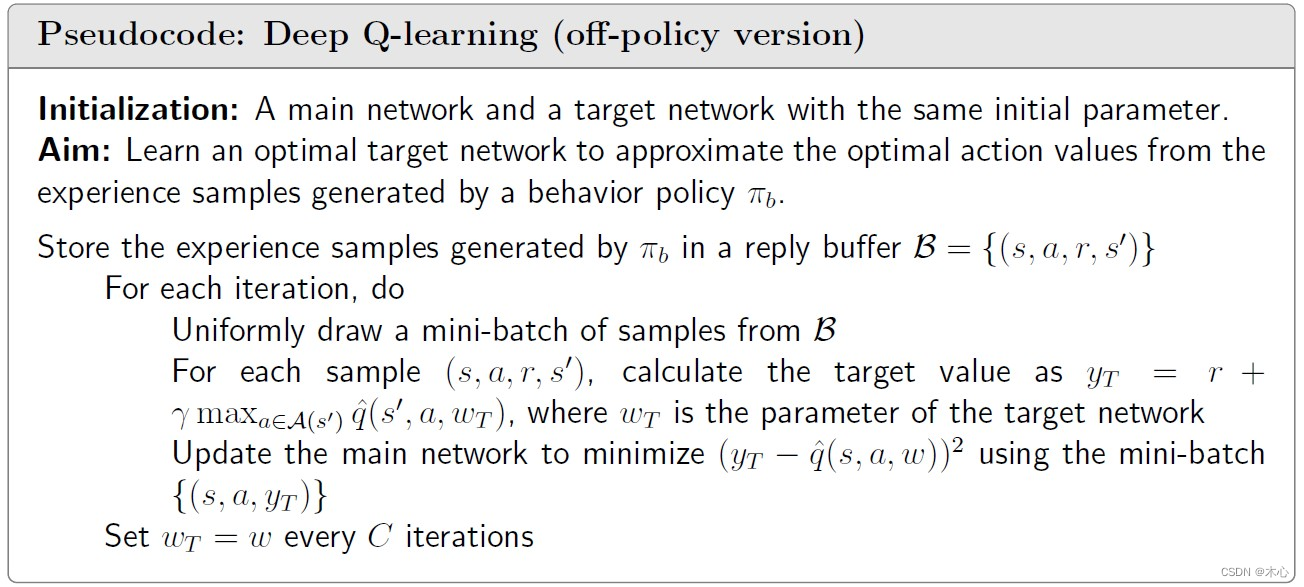
Reinforcement Learning with Code 【Chapter 8. Value Funtion Approximation】
Reinforcement Learning with Code This note records how the author begin to learn RL. Both theoretical understanding and code practice are presented. Many material are referenced such as ZhaoShiyu’s Mathematical Foundation of Reinforcement Learning, . 文章…...

常用InnoDB参数介绍
常用InnoDB参数介绍 1 状态参数1.1 InnoDB 缓冲池状态监控1.1.1 Innodb_buffer_pool_pages_total1.1.2 Innodb_buffer_pool_pages_data1.1.3 Innodb_buffer_pool_bytes_data1.1.4 Innodb_buffer_pool_pages_dirty1.1.5 Innodb_buffer_pool_bytes_dirty1.1.6 Innodb_buffer_pool…...
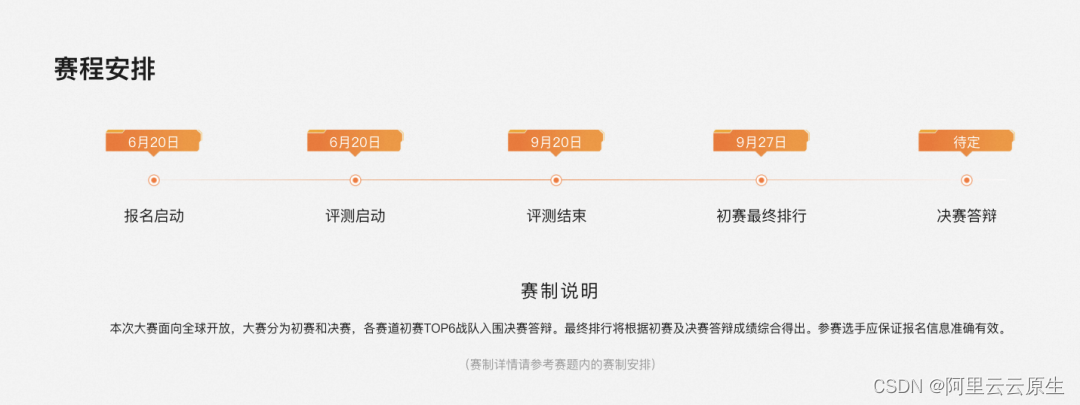
云原生网关部署新范式丨 Higress 发布 1.1 版本,支持脱离 K8s 部署
作者:澄潭 版本特性 Higress 1.1.0 版本已经 Release,K8s 环境下可以使用以下命令将 Higress 升级到最新版本: kubectl apply -f https://github.com/alibaba/higress/releases/download/v1.1.0/customresourcedefinitions.gen.yaml helm …...
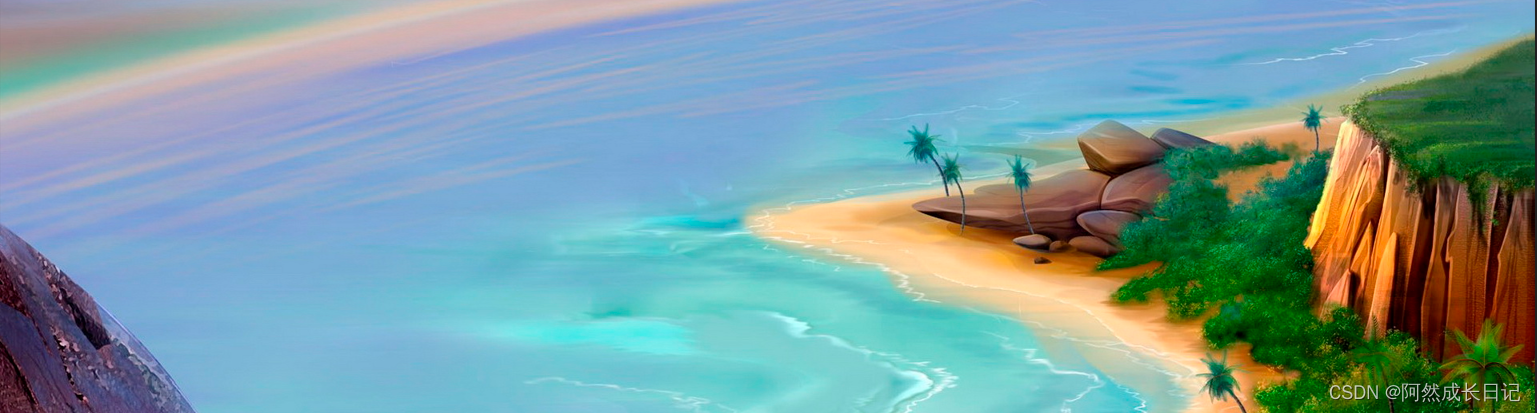
【通讯录】--C语言
💐 🌸 🌷 🍀 🌹 🌻 🌺 🍁 🍃 🍂 🌿 🍄🍝 🍛 🍤 📃个人主页 :阿然成长日记 …...
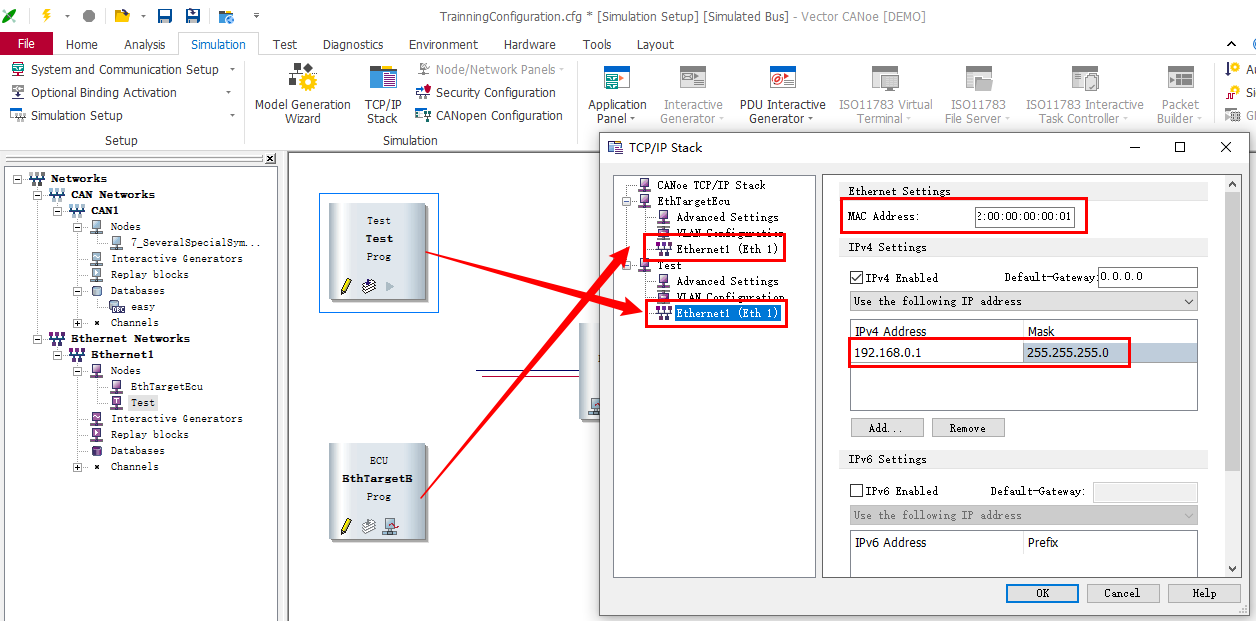
通过两种实现方式理解CANoe TC8 demo是如何判断接收的以太网报文里的字段的
假设有一个测试用例,需求是:编写一个测试用例,发送一条icmpv4 echo request报文给DUT,identifier字段设置为10。判断DUT能够回复icmpv4 echo reply报文,且identifier字段值为10。 实现:在canoe的simulation setup界面插入一个test节点,ip地址为:192.168.0.1,mac地址为…...
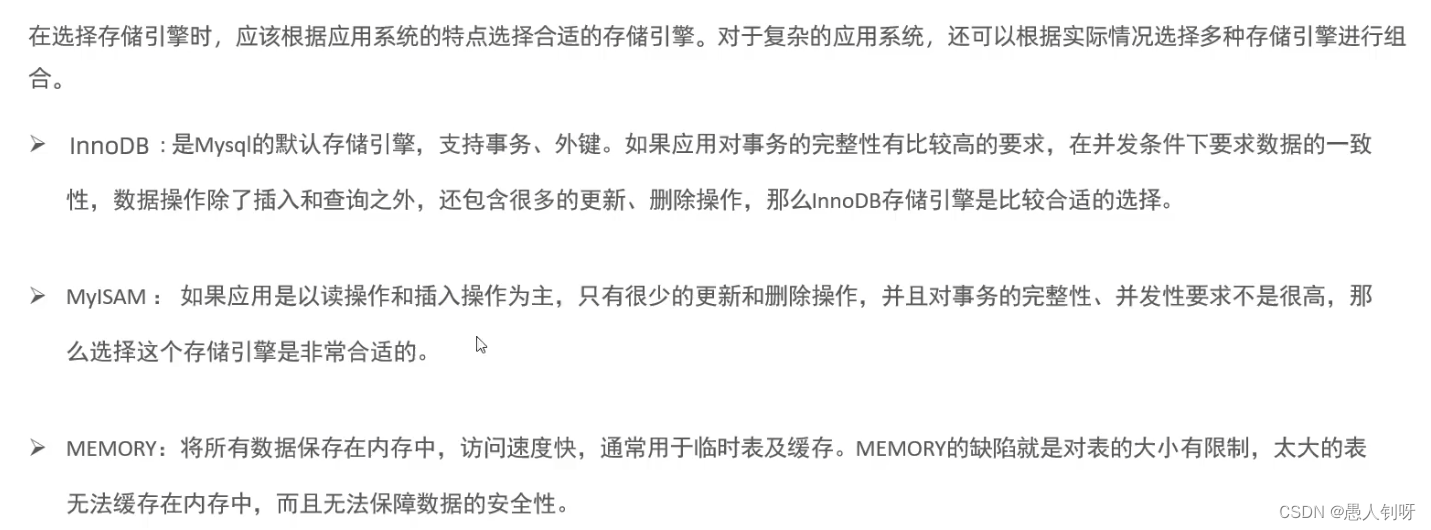
Mysql- 存储引擎
目录 1.Mysql体系结构 2.存储引擎简介 3.存储引擎特点 InnoDB MyISAM Memory 4.存储引擎选择 1.Mysql体系结构 MySQL整体的逻辑结构可以分为4层: 连接层:进行相关的连接处理、权限控制、安全处理等操作 服务层:服务层负责与客户层进行…...
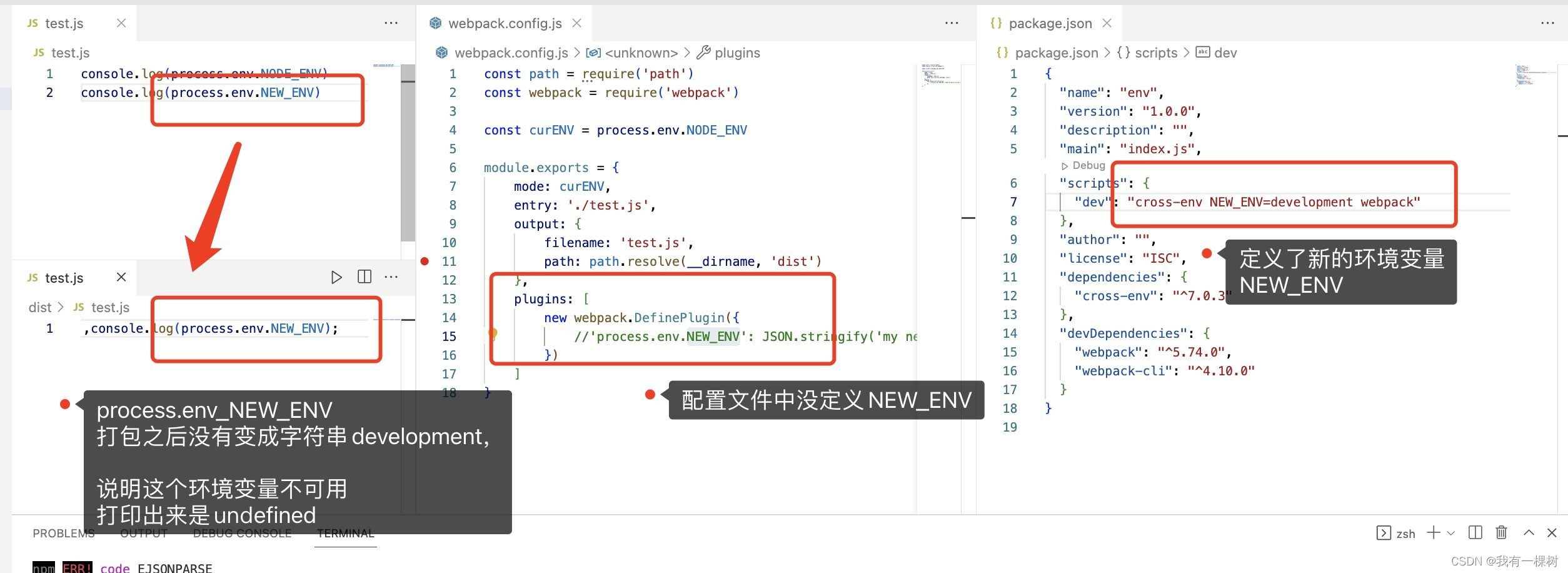
vite / nuxt3 项目使用define配置/自定义,可以使用process.env.xxx获取的环境变量
每日鸡汤:每个你想要学习的瞬间,都是未来的你向自己求救 首先可以看一下我的这篇文章了解一下关于 process.env 的环境变量。 对于vite项目,在我们初始化项目之后,在浏览器中打印 process.env,只有 NODE_ENV这个变量&…...

在Linux、Ubuntu中跨平台编译ARM(AARCH64)平台的binutils
Binutils 是GNU(https://www.gnu.org/)提供的一组二进制工具的集合。通常,在已经安装了Linux操作系统的个人电脑上,系统就已经自带了这个工具集。但在进行嵌入式开发的时候,可能会用到支持ARM64平台的Binutils,这时就需要用到交叉编译。 此前,在【1】我们已经介绍过Ubun…...
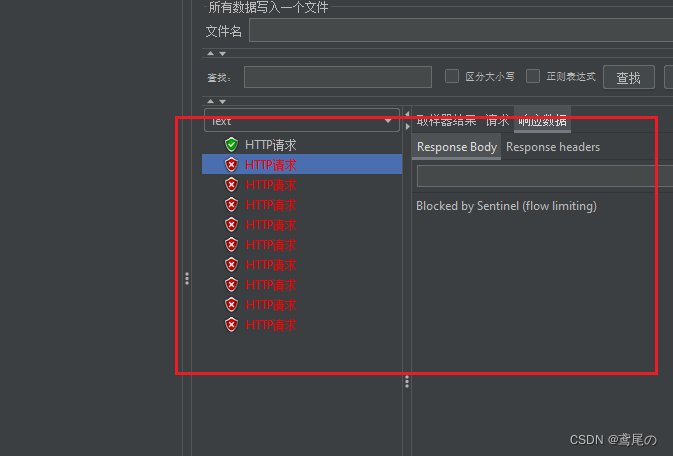
SpringCloudAlibaba微服务实战系列(五)Sentinel1.8.5+Nacos持久化
Sentinel数据持久化 前面介绍Sentinel的流控、熔断降级等功能,同时Sentinel应用也在面临着一个问题:我们在Sentinel后台管理界面中配置了一堆流控、降级规则,但是Sentinel一重启,这些规则全部消失了。那么我们就要考虑Sentinel的持…...

pytest中conftest的用法以及钩子基本使用
一、conftest是什么? conftest是pytest进阶中的高级应用,最近正好用到这一块儿,研究之后,向大家分享该高级应用。 二、使用步骤 1.conftest代码块 以全局性使用driver为主,只启动一次浏览器: pytest.fi…...

数据结构---顺序栈、链栈
特点 typedef struct Stack { int* base; //栈底 int* top;//栈顶 int stacksize //栈的容量; }SqStack; typedef struct StackNode { int data;//数据域 struct StackNode* next; //指针域 }StackNode,*LinkStack; 顺序栈 #define MaxSize 100 typedef struct Stack { int*…...
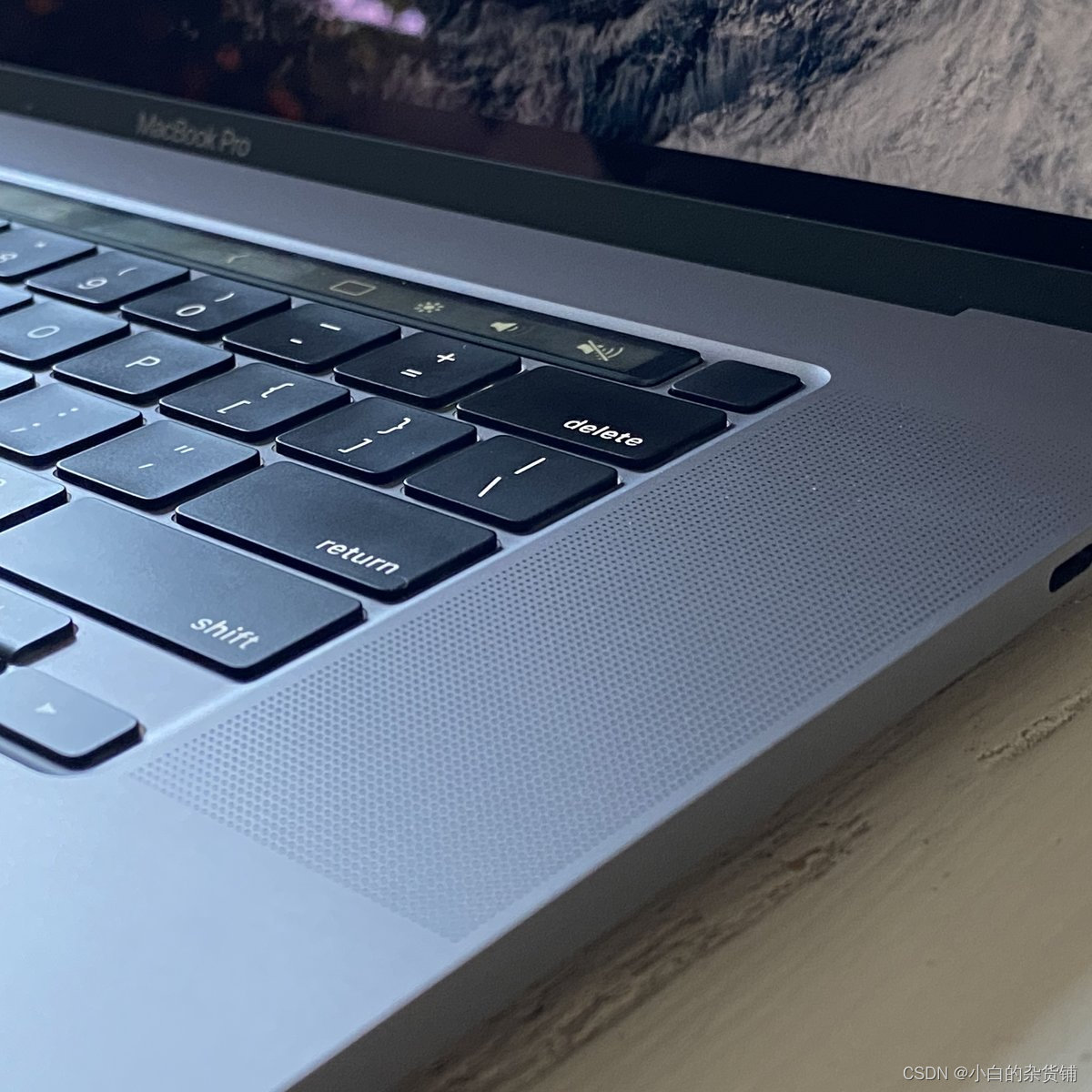
我的MacBook Pro:维护心得与实用技巧
文章目录 我的MacBook Pro:维护心得与实用技巧工作电脑概况:MacBook Pro 2019款 16 寸日常维护措施个人维护技巧其他建议 我的MacBook Pro:维护心得与实用技巧 无论是学习还是工作,电脑都是IT人必不可少的重要武器。一台好电脑除…...
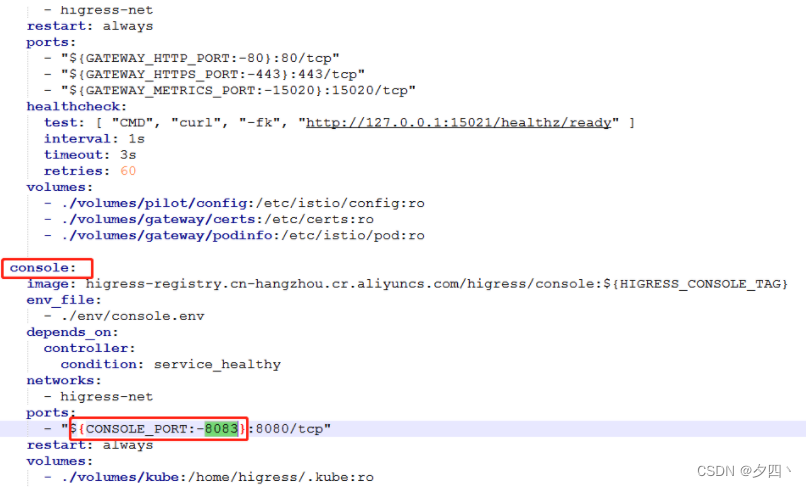
Higress非K8S安装
Higress非K8S安装 文章目录 Higress非K8S安装环境安装安装higress进入到higress 的目录下修改下nacos的地址启动Higress登录higress管理页面 Higress 是基于阿里内部构建的下一代云原生网关,官网介绍:https://higress.io/zh-cn/docs/overview/what-is-hi…...
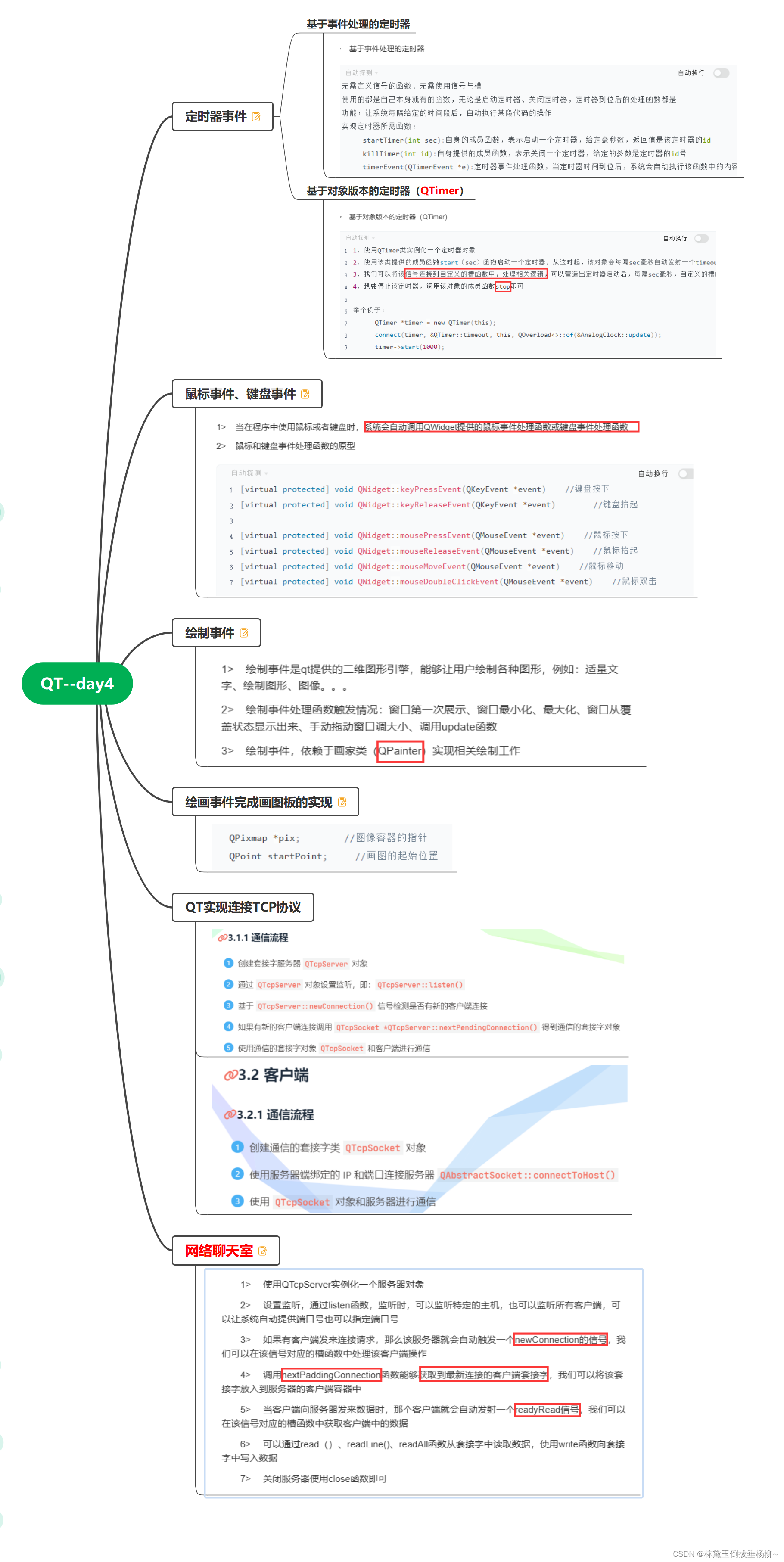
QT--day4(定时器事件、鼠标事件、键盘事件、绘制事件、实现画板、QT实现TCP服务器)
QT实现tcpf服务器代码:(源文件) #include "widget.h" #include "ui_widget.h"Widget::Widget(QWidget *parent): QWidget(parent), ui(new Ui::Widget) {ui->setupUi(this);//给服务器指针实例化空间server new QTc…...

hjm家族信托科技研究报告
目录 绪论 研究背景与意义 一、选题背景 二、选题意义 研究内容与主要研究方法 一、本文内容 二、研究方法 创新与不足 一、创新 二、不足之处 文献综述与理论基础 文献综述 国外研究现状国内研究现状国内外研究综述 理论基础 金融创新理论组合投资理论生命周期理论…...

[SQL挖掘机] - 视图相关操作
创建视图: create view view_name as select column1, column2, ... from table_name where condition;以上语句创建了一个名为view_name的视图,它基于table_name表格,并选择了列column1、column2等作为结果集。可以使用where子句来指定条件。 注意: 视…...
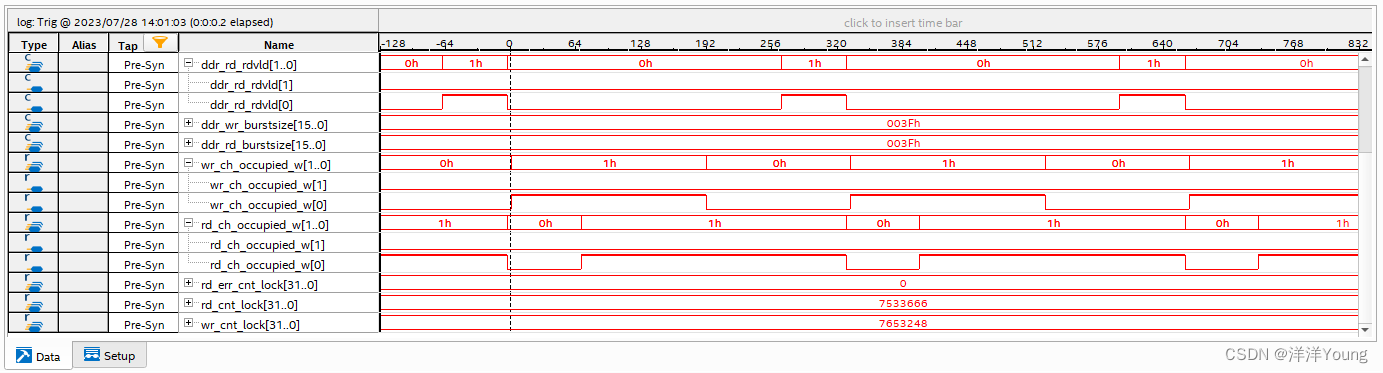
【Quartus FPGA】EMIF DDR3 读写带宽测试
在通信原理中,通信系统的有效性用带宽来衡量,带宽定义为每秒传输的比特数,单位 b/s,或 bps。在 DDR3 接口的产品设计中,DDR3 读/写带宽是设计者必须考虑的指标。本文主要介绍了 Quartus FPGA 平台 EMIF 参数配置&#…...
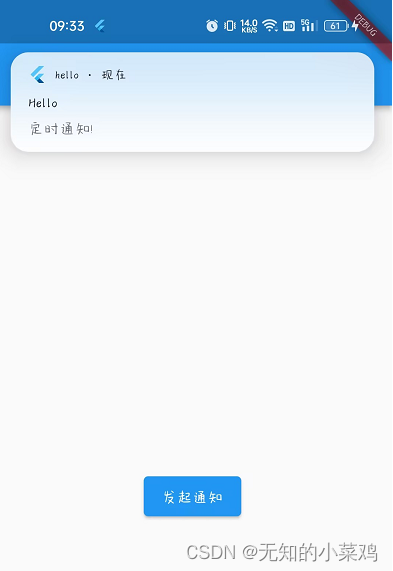
Flutter:flutter_local_notifications——消息推送的学习
前言 注: 刚开始学习,如果某些案例使用时遇到问题,可以自行百度、查看官方案例、官方github。 简介 Flutter Local Notifications是一个用于在Flutter应用程序中显示本地通知的插件。它提供了一个简单而强大的方法来在设备上发送通知&#…...
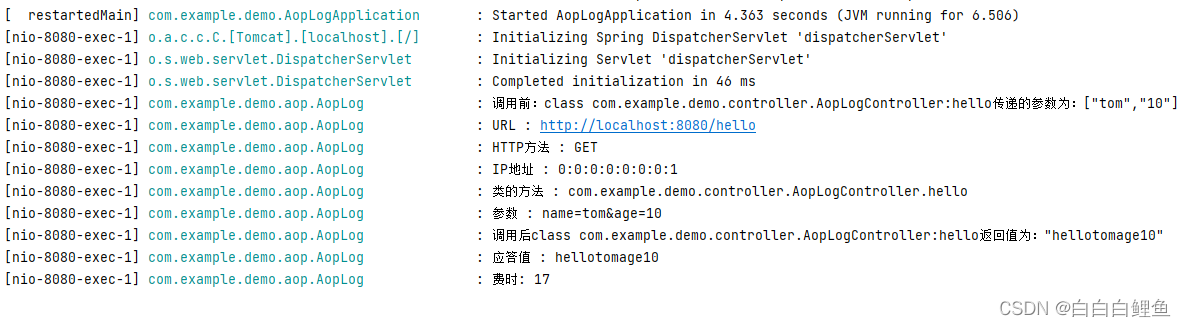
Spring AOP (面向切面编程)原理与代理模式—实例演示
一、AOP介绍和应用场景 Spring 中文文档 (springdoc.cn) Spring | Home 官网 1、AOP介绍(为什么会出现AOP ?) Java是一个面向对象(OOP)的语言,但它有一些弊端。虽然使用OOP可以通过组合或继承的方…...
什么是SCRUM认证体系 ?
Scrum认证是一个针对个人职业发展的认证体系,基础级认证主要面向Scrum的三个角色:Scrum Master、Scrum Product Owner 和 Developers。Scrum认证体系由Scrum官方机构—国际Scrum联盟(ScrumAlliance.org)制定和维护,Scr…...
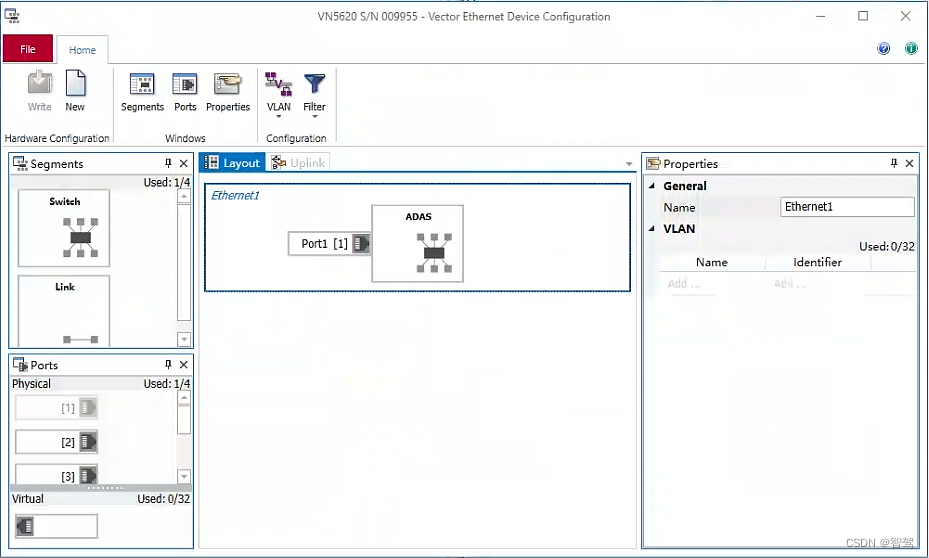
DoIP学习笔记系列:(二)VN5620 DoIP测试配置实践笔记
文章目录 1. 添加.cdd2. CAPL中调用接口发送DoIP请求3. “Ethernet Packet Builder”的妙用4. CANoe也可以做交互界面在进行测试前,先检查车载以太网硬件连线是否正确,需要注意连接两端的Master、Slave,100M、1000M等基本情况,在配置VN5620的时候就可以灵活处理了。成功安装…...
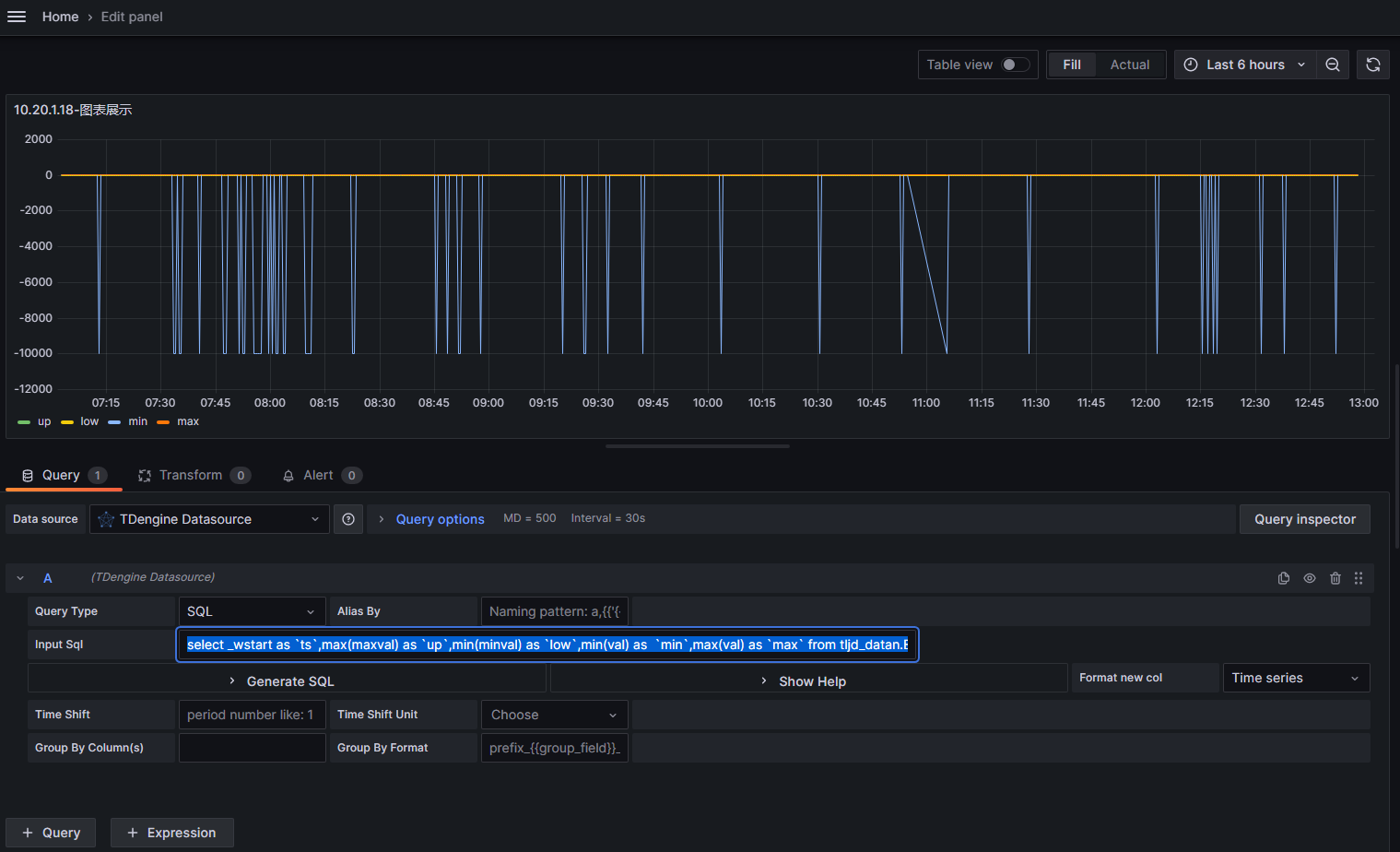
Grafana - TDEngine搭建数据监测报警系统
TDengine 与开源数据可视化系统 Grafana 快速集成搭建数据监测报警系统 一、介绍二、前置条件三、Grafana 安装及配置3.1 下载3.2 安装3.2.1 windows安装 - 图形界面3.2.2 linux安装 - 安装脚本 四、Grafana的TDEngine配置及使用4.1 登录4.2 安装 Grafana Plugin 并配置数据源4…...
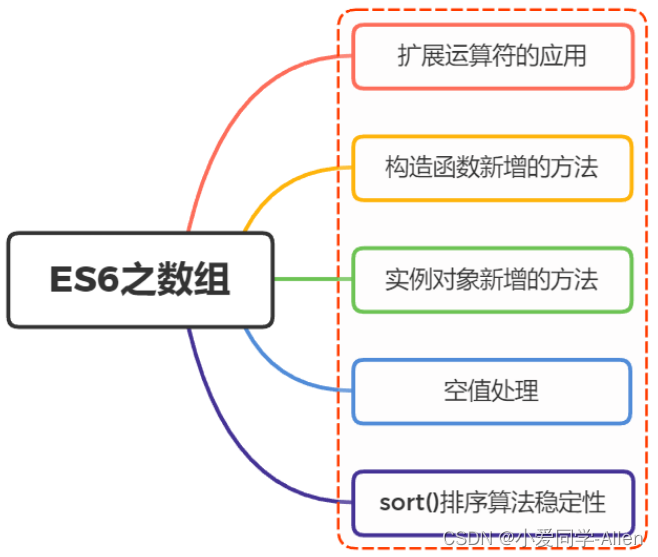
ES6基础知识二:ES6中数组新增了哪些扩展?
一、扩展运算符的应用 ES6通过扩展元素符…,好比 rest 参数的逆运算,将一个数组转为用逗号分隔的参数序列 console.log(...[1, 2, 3]) // 1 2 3console.log(1, ...[2, 3, 4], 5) // 1 2 3 4 5[...document.querySelectorAll(div)] // [<div>, &l…...
使用CRM分析数据有哪些功能?
CRM数据分析软件可以帮助企业增强竞争力,并更好地了解客户需求及市场变化,助力企业数据分析,并提供实时更新的数据和分析结果,CRM数据分析软件的主要特点是什么?包括以下6个特点。 CRM数据分析软件的主要功能通常包括…...
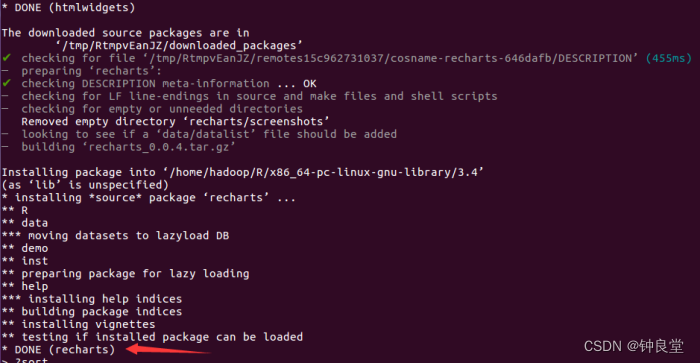
大数据课程综合实验案例---课设问题汇总
最近翻看两年前的大数据课设,感觉这个大数据课设实验当时答辩 在大数据课设实验过程中,我遇到了很多问题,在这里做出汇总: 1、MySQL启动报错 首先,我的MySQL有时候启动不了,当我输入这个命令的时候&#…...
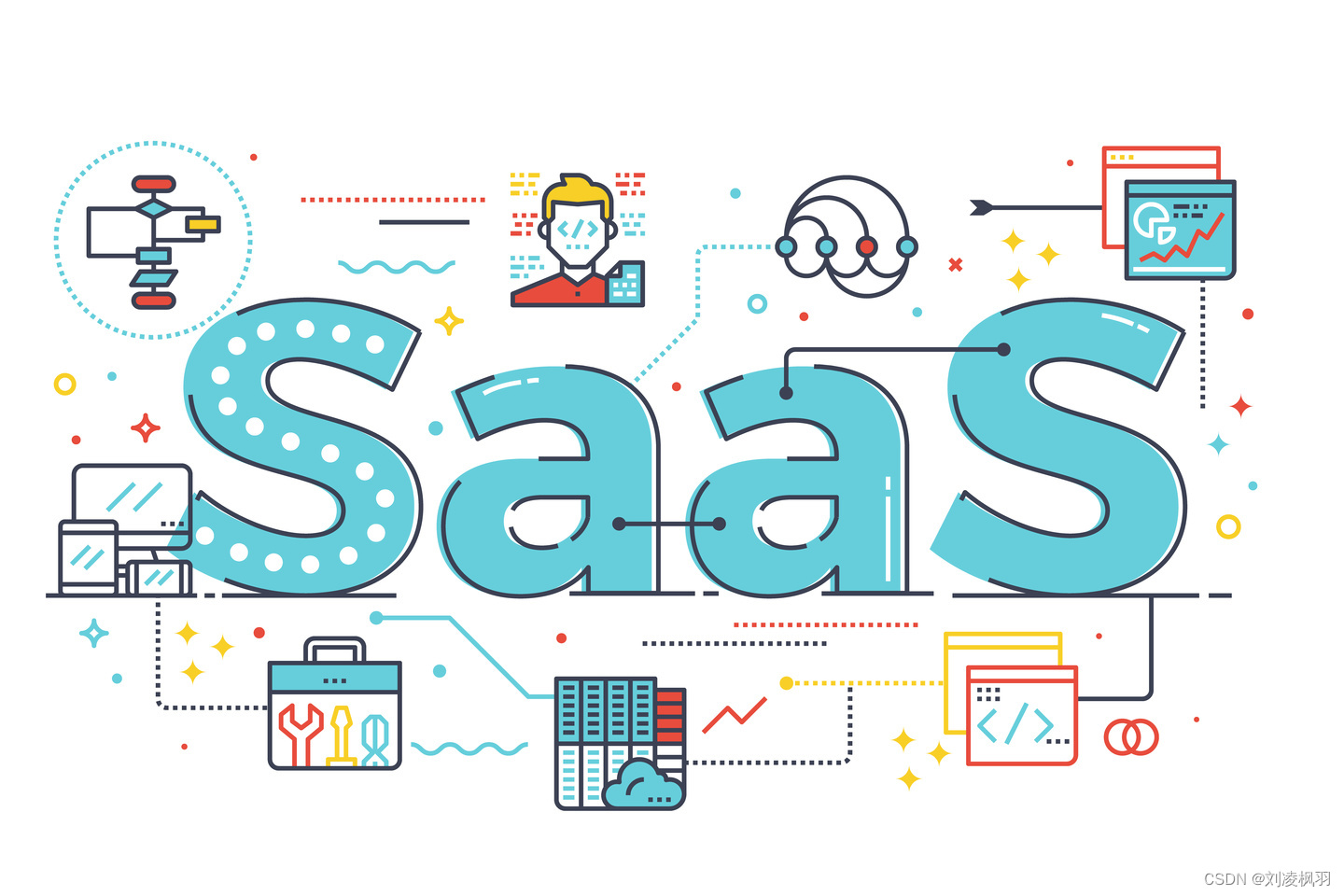
基于Vue+Element Plus实现表格组件
目录 前言分析实现例子效果图前言 表格对于管理类项目是很重要的,可以只管的展示和比比较数据。使用Element Plus能解决一部分问题,但是还存在一些缺点和不足。 分析 浏览器上表格数据展示空间不足。列显示太多不够直观。完全依赖官方表格组件代码过于臃肿不利于管理和优化…...
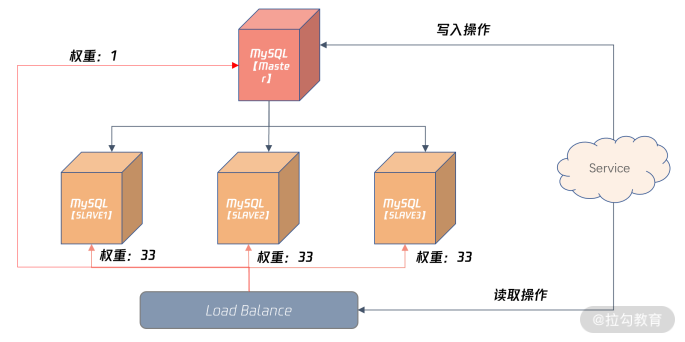
MYSQL 主从复制
在读多写少的网络环境下,MySQL 如何优化数据查询方案 假如说一个电商平台 到双十一了 大量的读写操作 如果不做点什么的话 平台就被冲烂了 那我们要怎么办呢? 你或许会想 林北直接一个redis缓存 帮数据库度过难关 这个操作实际上是不行的 因为应用缓存的原则之一…...
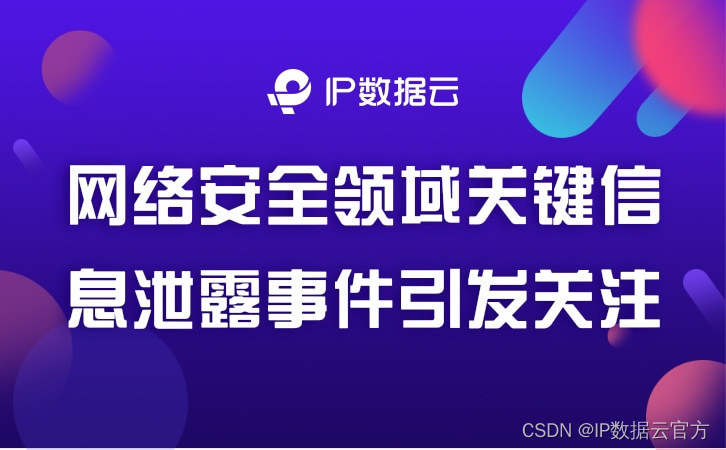
网络安全领域关键信息泄露事件引发关注
近日,一家知名网络安全公司发布了一份报告揭露了一起重大信息泄露事件。据称,该事件涉及大量敏感用户数据的泄露引发了全球网络安全领域的广泛关注。 根据报道,该事件发生在全球范围内涉及多个国家和组织。专家指出,此次泄露事件…...
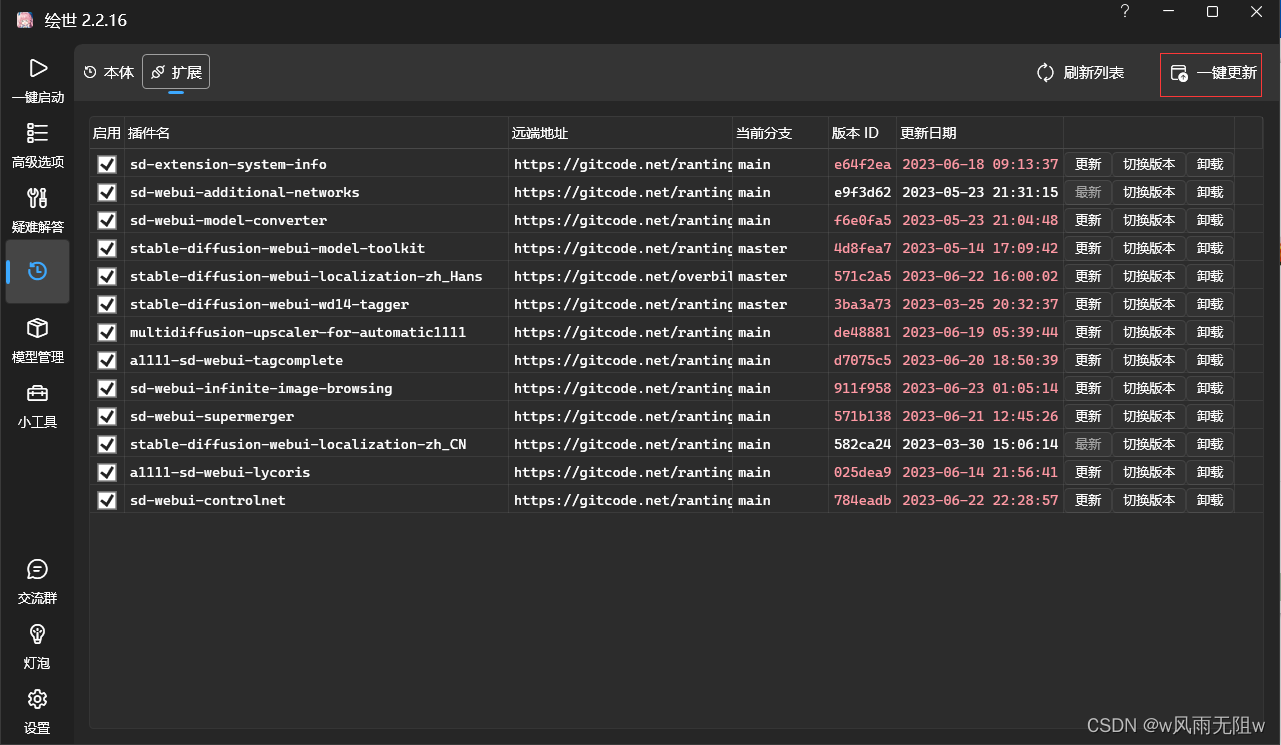
AI 绘画Stable Diffusion 研究(一)sd整合包v4.2 版本安装说明
部署包作者:秋葉aaaki 免责声明: 本安装包及启动器免费提供 无任何盈利目的 大家好,我是风雨无阻。众所周知,StableDiffusion 是非常强大的AI绘图工具,需要详细了解StableDiffusion的朋友,可查看我之前的这篇文章: 最…...
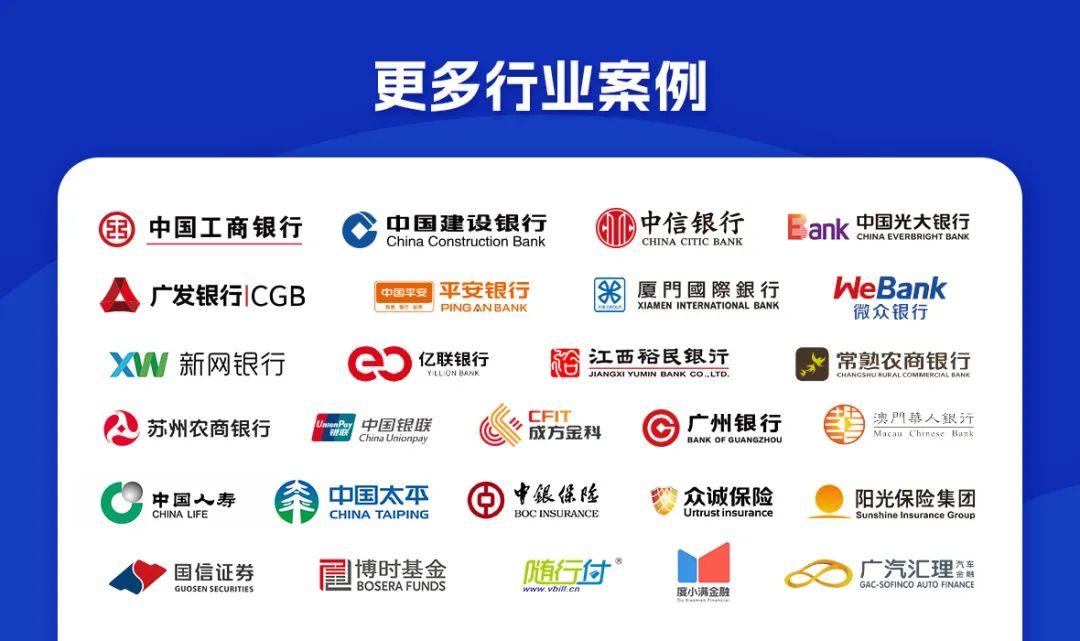
夯实数字化转型安全地基,华东某农商行开源安全治理经验
华东某农村商业银行是一家全国首批组建的股份制农村金融机构。近年来,该农商行坚持“科技强行”战略,进一步夯实数字化核心基础,积极推动金融科技与产品、服务的深度融合,努力拓展数字金融的包容性,让数字金融更有温度…...