计算机视觉基础——基于yolov5-face算法的车牌检测
文章目录
- 车牌检测算法检测实现
- 1.环境布置
- 2.数据处理
- 2.1 CCPD数据集介绍
- 2.1.1 ccpd2019及2020
- 2.1.2 文件名字解析
- 2.2数据集处理
- 2.2.1 CCPD数据处理
- 2.2.2 CPRD数据集处理
- 2.3 检测算法
- 2.3.1 数据配置car_plate.yaml
- 2.3.2 模型配置
- 2.3.3 train.py
- 2.3.4 训练结果
- 2.4 部署
- 2.4.1 pth推理(detect.py)
- 2.4.2 onnx推理
- 2.4.3 trt推理
- 2.4.4 numpy版本trt推理
- 2.4.5 推理结果展示
- 参考开源
车牌检测算法检测实现
1.环境布置
- torch==1.8.1
- torchvision==0.9.1
pip install -r requirements.txt
2.数据处理
2.1 CCPD数据集介绍
2.1.1 ccpd2019及2020
CCPD数据集主要采集于安徽某停车场一段时间内的数据,
所有图片尺寸固定为720×1160(w×h),
大约包含25w+的各种场景图片,如下图所示:
类别 | 描述 | 图片数 |
---|---|---|
CCPD-Base | 通用车牌图片 | 200k |
CCPD-FN | 车牌离摄像头拍摄位置相对较近或较远 | 20k |
CCPD-DB | 车牌区域亮度较亮、较暗或者不均匀 | 20k |
CCPD-Rotate | 车牌水平倾斜 20 到 50 度,竖直倾斜-10到 10 度 | 10k |
CCPD-Tilt | 车牌水平倾斜 15 到 45 度,竖直倾斜 15 到 45 度 | 10k |
CCPD-Weather | 车牌在雨雪雾天气拍摄得到 | 10k |
CCPD-Challenge | 在车牌检测识别任务中较有挑战性的图片 | 10k |
CCPD-Blur | 由于摄像机镜头抖动导致的模楜车牌图片 | 5k |
CCPD-NP | 没有安装车牌的新车图片 | 5k |
2.1.2 文件名字解析
1、01:车牌占整个界面比例;(一般没用,可忽略)
2、86_91: 车牌的水平角度和垂直角度
3、298\&341_449\&414: 车牌标注框左上角和右下角的坐标
4、458\&394_308\&410_304\&357_454\&341:车牌四个顶点的坐标,顺序为右下、左下、左上、右上
5、0_0_14_28_24_26_29: 这个代表着和省份 (第一位)、地市 (第二位)、车牌号 (剩余部分) 的映射关系
6、124: 亮度,值越大亮度越高(仅供参考)
7、24:模糊度,值越小越模糊(仅供参考)
2.2数据集处理
2.2.1 CCPD数据处理
1.解压ccpd,cprd数据集2019,ccpd文件太大,解压慢,利用程序进行解压
import tarfile
# 指定tar文件路径
tar_path = r".\CCPD2019\CCPD2019.tar"
# 打开tar文件
with tarfile.open(tar_path, "r") as tar:# 解压所有文件到指定目录tar.extractall(r".\ccpd")
# 解压完成
print("解压完成")
ccpd数据集处理方法
import os
import shutil
import cv2
import numpy as np
from tqdm import tqdmdef allFilePath(rootPath, allFIleList):'''获取指定目录下所有以.jpg结尾的文件的路径,并将这些路径存储在一个列表中。'''fileList = os.listdir(rootPath)for temp in fileList:if os.path.isfile(os.path.join(rootPath, temp)):if temp.endswith(".jpg"):allFIleList.append(os.path.join(rootPath, temp))else:allFilePath(os.path.join(rootPath, temp), allFIleList)def order_points(pts):'''对给定的坐标点进行排序,使得列表中的第一个点是左上角,第二个点是右上角,第三个点是右下角,第四个点是左下角。返回排序后的坐标点列表。'''# initialzie a list of coordinates that will be ordered# such that the first entry in the list is the top-left,# the second entry is the top-right, the third is the# bottom-right, and the fourth is the bottom-leftpts = pts[:4, :]rect = np.zeros((5, 2), dtype="float32")# the top-left point will have the smallest sum, whereas# the bottom-right point will have the largest sums = pts.sum(axis=1)rect[0] = pts[np.argmin(s)]rect[2] = pts[np.argmax(s)]# now, compute the difference between the points, the# top-right point will have the smallest difference,# whereas the bottom-left will have the largest differencediff = np.diff(pts, axis=1)rect[1] = pts[np.argmin(diff)]rect[3] = pts[np.argmax(diff)]# return the ordered coordinatesreturn rectdef get_rect_and_landmarks(img_path):'''该函数用于从图像文件路径中解析出矩形框和关键点的坐标,并返回解析后的结果。'''file_name = img_path.split("/")[-1].split("-")landmarks_np = np.zeros((5, 2))rect = file_name[2].split("_")landmarks = file_name[3].split("_")rect_str = "&".join(rect)landmarks_str = "&".join(landmarks)rect = rect_str.split("&")landmarks = landmarks_str.split("&")rect = [int(x) for x in rect]landmarks = [int(x) for x in landmarks]for i in range(4):landmarks_np[i][0] = landmarks[2 * i]landmarks_np[i][1] = landmarks[2 * i + 1]# middle_landmark_w =int((landmarks[4]+landmarks[6])/2)# middle_landmark_h =int((landmarks[5]+landmarks[7])/2)# landmarks.append(middle_landmark_w)# landmarks.append(middle_landmark_h)landmarks_np_new = order_points(landmarks_np)# landmarks_np_new[4]=np.array([middle_landmark_w,middle_landmark_h])return rect, landmarks, landmarks_np_newdef x1x2y1y2_yolo(rect, landmarks, img):h, w, c = img.shaperect[0] = max(0, rect[0])rect[1] = max(0, rect[1])rect[2] = min(w - 1, rect[2] - rect[0])rect[3] = min(h - 1, rect[3] - rect[1])annotation = np.zeros((1, 14))annotation[0, 0] = (rect[0] + rect[2] / 2) / w # cxannotation[0, 1] = (rect[1] + rect[3] / 2) / h # cyannotation[0, 2] = rect[2] / w # wannotation[0, 3] = rect[3] / h # hannotation[0, 4] = landmarks[0] / w # l0_xannotation[0, 5] = landmarks[1] / h # l0_yannotation[0, 6] = landmarks[2] / w # l1_xannotation[0, 7] = landmarks[3] / h # l1_yannotation[0, 8] = landmarks[4] / w # l2_xannotation[0, 9] = landmarks[5] / h # l2_yannotation[0, 10] = landmarks[6] / w # l3_xannotation[0, 11] = landmarks[7] / h # l3_y# annotation[0, 12] = landmarks[8] / w # l4_x# annotation[0, 13] = landmarks[9] / h # l4_yreturn annotationdef xywh2yolo(rect, landmarks_sort, img):h, w, c = img.shaperect[0] = max(0, rect[0])rect[1] = max(0, rect[1])rect[2] = min(w - 1, rect[2] - rect[0])rect[3] = min(h - 1, rect[3] - rect[1])annotation = np.zeros((1, 12))annotation[0, 0] = (rect[0] + rect[2] / 2) / w # cxannotation[0, 1] = (rect[1] + rect[3] / 2) / h # cyannotation[0, 2] = rect[2] / w # wannotation[0, 3] = rect[3] / h # hannotation[0, 4] = landmarks_sort[0][0] / w # l0_xannotation[0, 5] = landmarks_sort[0][1] / h # l0_yannotation[0, 6] = landmarks_sort[1][0] / w # l1_xannotation[0, 7] = landmarks_sort[1][1] / h # l1_yannotation[0, 8] = landmarks_sort[2][0] / w # l2_xannotation[0, 9] = landmarks_sort[2][1] / h # l2_yannotation[0, 10] = landmarks_sort[3][0] / w # l3_xannotation[0, 11] = landmarks_sort[3][1] / h # l3_y# annotation[0, 12] = landmarks_sort[4][0] / w # l4_x# annotation[0, 13] = landmarks_sort[4][1] / h # l4_yreturn annotation
def yolo2x1y1x2y2(annotation, img):h, w, c = img.shaperect = annotation[:, 0:4].squeeze().tolist()landmarks = annotation[:, 4:].squeeze().tolist()rect_w = w * rect[2]rect_h = h * rect[3]rect_x = int(rect[0] * w - rect_w / 2)rect_y = int(rect[1] * h - rect_h / 2)new_rect = [rect_x, rect_y, rect_x + rect_w, rect_y + rect_h]for i in range(5):landmarks[2 * i] = landmarks[2 * i] * wlandmarks[2 * i + 1] = landmarks[2 * i + 1] * hreturn new_rect, landmarksdef update_txt(file_root = r"I:/CCPD2019/ccpd",save_img_path=r"H:\data\images",save_txt_path="H:\data\labels"):print(file_root, "start!!!!!")file_list = []count = 0allFilePath(file_root, file_list)# print(file_list)# exit()for img_path in file_list:count += 1# img_path = r"ccpd_yolo_test/02-90_85-173&466_452&541-452&553_176&556_178&463_454&460-0_0_6_26_15_26_32-68-53.jpg"text_path = img_path.replace(".jpg", ".txt")# 读取图片img = cv2.imread(img_path)rect, landmarks, landmarks_sort = get_rect_and_landmarks(img_path)# annotation=x1x2y1y2_yolo(rect,landmarks,img)annotation = xywh2yolo(rect, landmarks_sort, img)str_label = "0 "for i in range(len(annotation[0])):str_label = str_label + " " + str(annotation[0][i])str_label = str_label.replace('[', '').replace(']', '')str_label = str_label.replace(',', '') + '\n'# if os.path.exists(text_path):# continue# else:shutil.move(img_path,os.path.join(os.path.join(save_img_path,os.path.basename(img_path))))text_path_save = os.path.join(save_txt_path,os.path.basename(text_path))# print(text_path_save)# exit()with open(text_path_save, "w") as f:f.write(str_label)print(text_path,"finished!")# print(count, img_path)print(os.getpid(),"end!!!")def delete_non_jpg_images(image_folder):for filename in os.listdir(image_folder):if not filename.endswith(".jpg"):file_path = os.path.join(image_folder, filename)os.remove(file_path)print("删除完毕")def move_files_to_folders(images_folder, folders_folder, labels_folder):for filename in os.listdir(images_folder):if filename.endswith(".jpg"):image_path = os.path.join(images_folder, filename)label_path = os.path.join(images_folder, os.path.splitext(filename)[0] + ".txt")folder_path = os.path.join(folders_folder, filename)labels_folder_path = os.path.join(labels_folder, os.path.splitext(filename)[0] + ".txt")if not os.path.exists(folder_path) and not os.path.exists(labels_folder_path) and os.path.exists(label_path):# 不存在同名shutil.move(image_path, folder_path)shutil.move(label_path, labels_folder_path)if __name__ == '__main__':# 1. 处理ccpd文件夹import multiprocessingpool = multiprocessing.Pool(processes=14) # 这里使用4个进程files = []for dir in os.listdir(r"I:/CCPD2019/ccpd"):files.append(os.path.join(r"I:/CCPD2019/ccpd",dir))# 使用进程池执行任务results = pool.map(update_txt,files)# 关闭进程池,防止新任务被提交pool.close()# 等待所有任务完成pool.join()# 2. 清理异常文件夹# 调用删除非jpg图像的函数image_folder = r"H:\data\images"# 删除文件delete_non_jpg_images(image_folder)# 3.加入一些新增文件夹文件# 指定文件夹路径# images_folder = r"single_yellow_val"# folders_folder = r"H:\data\images"# labels_folder = r"H:\data\labels"# # 调用移动文件的函数# move_files_to_folders(images_folder, folders_folder, labels_folder)
处理后文件,共计329499个文件
处理后为类别+左上角+右下角+4个角点
2.2.2 CPRD数据集处理
Unified Chinese License Plate Detection and Recognition with High Efficiency(Arxiv 2022)
这段话描述了标签文件夹中的txt文件的注释格式。每个txt文件的文件名与相应图像的名称相同。
在txt文件中,每一行表示图像中的一个车牌(LP)的注释。
一个LP的注释格式如下:
x1,y1,x2,y2,x3,y3,x4,y4,type,content
前八个数字表示边界四边形的坐标。
"type"注释表示LP的类型,0表示蓝色车牌,1表示黄色单线车牌,2表示黄色双线车牌,3表示白色车牌。
"content"注释表示LP的内容。
2.3 检测算法
YOLOv5-Face是YOLOv5的一个改进版本,特别针对人脸检测任务。它添加了一个5-Point Landmark Regression Head(关键点回归),并对Landmark Regression Head使用了Wing loss进行约束。此外,YOLOv5-Face还设计了不同模型尺寸的检测器,从大模型到超小模型,以实现在嵌入式或移动设备上的实时检测。在WiderFace数据集上的实验结果表明,YOLOv5-Face在几乎所有的Easy、Medium和Hard子集上都能达到最先进的性能,超过了特定设计的人脸检测器。与许多其他的人脸检测器不同,YOLOv5-Face把人脸检测作为一个一般的目标检测任务来看待。
这里将yolov5-face 修改为四个关键点,做目标检测任务
2.3.1 数据配置car_plate.yaml
# PASCAL VOC dataset http://host.robots.ox.ac.uk/pascal/VOC/
# Train command: python train.py --data voc.yaml
# Default dataset location is next to /yolov5:
# /parent_folder
# /VOC
# /yolov5# download command/URL (optional)
download: bash data/scripts/get_voc.sh# train and val data as 1) directory: path/images/, 2) file: path/images.txt, or 3) list: [path1/images/, path2/images/]
train: E:/data/train/images
val: E:/data/val_detect/val
# number of classes
nc: 2# class names
names: [ 'single_plate','double_plate']
2.3.2 模型配置
# parameters
nc: 2 # number of classes
depth_multiple: 1.0 # model depth multiple
width_multiple: 0.5 # layer channel multiple# anchors
anchors:- [4,5, 8,10, 13,16] # P3/8- [23,29, 43,55, 73,105] # P4/16- [146,217, 231,300, 335,433] # P5/32# YOLOv5 backbone
backbone:# [from, number, module, args][[-1, 1, StemBlock, [32, 3, 2]], # 0-P2/4[-1, 1, ShuffleV2Block, [128, 2]], # 1-P3/8[-1, 3, ShuffleV2Block, [128, 1]], # 2[-1, 1, ShuffleV2Block, [256, 2]], # 3-P4/16[-1, 7, ShuffleV2Block, [256, 1]], # 4[-1, 1, ShuffleV2Block, [512, 2]], # 5-P5/32[-1, 3, ShuffleV2Block, [512, 1]], # 6]# YOLOv5 head
head:[[-1, 1, Conv, [128, 1, 1]],[-1, 1, nn.Upsample, [None, 2, 'nearest']],[[-1, 4], 1, Concat, [1]], # cat backbone P4[-1, 1, C3, [128, False]], # 10[-1, 1, Conv, [128, 1, 1]],[-1, 1, nn.Upsample, [None, 2, 'nearest']],[[-1, 2], 1, Concat, [1]], # cat backbone P3[-1, 1, C3, [128, False]], # 14 (P3/8-small)[-1, 1, Conv, [128, 3, 2]],[[-1, 11], 1, Concat, [1]], # cat head P4[-1, 1, C3, [128, False]], # 17 (P4/16-medium)[-1, 1, Conv, [128, 3, 2]],[[-1, 7], 1, Concat, [1]], # cat head P5[-1, 1, C3, [128, False]], # 20 (P5/32-large)[[14, 17, 20], 1, Detect, [nc, anchors]], # Detect(P3, P4, P5)]
2.3.3 train.py
parser = argparse.ArgumentParser()# 权重parser.add_argument('--weights', type=str, default='best.pt', help='initial weights path')# 默认配置文件parser.add_argument('--cfg', type=str, default='models/car_plate.yaml', help='model.yaml path')parser.add_argument('--data', type=str, default='data/car_plate.yaml', help='data.yaml path')parser.add_argument('--hyp', type=str, default='data/hyp.scratch.yaml', help='hyperparameters path')parser.add_argument('--epochs', type=int, default=10)parser.add_argument('--batch-size', type=int, default=64, help='total batch size for all GPUs')parser.add_argument('--img-size', nargs='+', type=int, default=[640, 640], help='[train, test] image sizes')parser.add_argument('--rect', action='store_true', help='rectangular training')parser.add_argument('--resume', nargs='?', const=True, default=False, help='resume most recent training')parser.add_argument('--nosave', action='store_true', help='only save final checkpoint')parser.add_argument('--notest', action='store_true', help='only test final epoch')parser.add_argument('--noautoanchor', action='store_true', help='disable autoanchor check')parser.add_argument('--evolve', action='store_true', help='evolve hyperparameters')parser.add_argument('--bucket', type=str, default='', help='gsutil bucket')parser.add_argument('--cache-images', action='store_true', help='cache images for faster training')parser.add_argument('--image-weights', action='store_true', help='use weighted image selection for training')parser.add_argument('--device', default='', help='cuda device, i.e. 0 or 0,1,2,3 or cpu')parser.add_argument('--multi-scale', action='store_true', default=True, help='vary img-size +/- 50%%')parser.add_argument('--single-cls', action='store_true', help='train multi-class data as single-class')parser.add_argument('--adam', action='store_true', help='use torch.optim.Adam() optimizer')parser.add_argument('--sync-bn', action='store_true', help='use SyncBatchNorm, only available in DDP mode')parser.add_argument('--local_rank', type=int, default=-1, help='DDP parameter, do not modify')parser.add_argument('--log-imgs', type=int, default=2, help='number of images for W&B logging, max 100')parser.add_argument('--log-artifacts', action='store_true', help='log artifacts, i.e. final trained model')parser.add_argument('--workers', type=int, default=4, help='maximum number of dataloader workers')parser.add_argument('--project', default='runs/train', help='save to project/name')parser.add_argument('--name', default='exp', help='save to project/name')parser.add_argument('--exist-ok', action='store_true', help='existing project/name ok, do not increment')
2.3.4 训练结果
2.4 部署
2.4.1 pth推理(detect.py)
#!/usr/bin/env python
import warnings
warnings.filterwarnings("ignore")
import os
import cv2
import numpy as np
import time
import torch
import copy
from models.experimental import attempt_load
from utils.datasets import letterbox
from utils.general import check_img_size, non_max_suppression_face, scale_coords
from utils.torch_utils import time_synchronizedclors = [(255, 0, 0), (0, 255, 0), (0, 0, 255), (255, 255, 0), (0, 255, 255)]def load_model(weights, device):model = attempt_load(weights, map_location=device) # load FP32 modelreturn model
def scale_coords_landmarks(img1_shape, coords, img0_shape, ratio_pad=None):# Rescale coords (xyxy) from img1_shape to img0_shapeif ratio_pad is None: # calculate from img0_shapegain = min(img1_shape[0] / img0_shape[0], img1_shape[1] / img0_shape[1]) # gain = old / newpad = (img1_shape[1] - img0_shape[1] * gain) / 2, (img1_shape[0] - img0_shape[0] * gain) / 2 # wh paddingelse:gain = ratio_pad[0][0]pad = ratio_pad[1]coords[:, [0, 2, 4, 6]] -= pad[0] # x paddingcoords[:, [1, 3, 5, 7]] -= pad[1] # y paddingcoords[:, :10] /= gain# clip_coords(coords, img0_shape)coords[:, 0].clamp_(0, img0_shape[1]) # x1coords[:, 1].clamp_(0, img0_shape[0]) # y1coords[:, 2].clamp_(0, img0_shape[1]) # x2coords[:, 3].clamp_(0, img0_shape[0]) # y2coords[:, 4].clamp_(0, img0_shape[1]) # x3coords[:, 5].clamp_(0, img0_shape[0]) # y3coords[:, 6].clamp_(0, img0_shape[1]) # x4coords[:, 7].clamp_(0, img0_shape[0]) # y4# coords[:, 8].clamp_(0, img0_shape[1]) # x5# coords[:, 9].clamp_(0, img0_shape[0]) # y5return coords
def get_plate_rec_landmark(img, xyxy, conf, landmarks, class_num, device):h, w, c = img.shaperesult_dict = {}tl = 1 or round(0.002 * (h + w) / 2) + 1 # line/font thicknessx1 = int(xyxy[0])y1 = int(xyxy[1])x2 = int(xyxy[2])y2 = int(xyxy[3])landmarks_np = np.zeros((4, 2))rect = [x1, y1, x2, y2]for i in range(4):point_x = int(landmarks[2 * i])point_y = int(landmarks[2 * i + 1])landmarks_np[i] = np.array([point_x, point_y])class_label = int(class_num) # 车牌的的类型0代表单牌,1代表双层车牌result_dict['box'] = rectresult_dict['landmarks'] = landmarks_np.tolist()result_dict['class'] = class_labelreturn result_dict
class Detect:def __init__(self, conf_thres=0.6, iou_thres=0.5, img_size=640):self.conf_thres = conf_thresself.iou_thres = iou_thresself.img_size = img_sizeself.device = "cuda" if torch.cuda.is_available() else "cpu"self.detect_model = load_model("weights/best.pt", self.device)def detect(self, orgimg):dict_list = []if orgimg is None:return []if orgimg.shape[-1] == 4:orgimg = cv2.cvtColor(orgimg, cv2.COLOR_BGRA2BGR)h0, w0 = orgimg.shape[:2] # orig hwimg0 = copy.deepcopy(orgimg)r = self.img_size / max(h0, w0) # resize image to img_sizeif r != 1: # always resize down, only resize up if training with augmentationinterp = cv2.INTER_AREA if r < 1 else cv2.INTER_LINEARimg0 = cv2.resize(img0, (int(w0 * r), int(h0 * r)), interpolation=interp)imgsz = check_img_size(self.img_size, s=self.detect_model.stride.max()) # check img_sizeimg = letterbox(img0, new_shape=imgsz,auto=False)[0]# Convertimg = img[:, :, ::-1].transpose(2, 0, 1).copy() # BGR to RGB, to 3x416x416# Run inferencet0 = time.time()img = torch.from_numpy(img).to(self.device)img = img.float() # uint8 to fp16/32img /= 255.0 # 0 - 255 to 0.0 - 1.0if img.ndimension() == 3:img = img.unsqueeze(0)# Inferencet1 = time_synchronized()pred = self.detect_model(img)[0]print(pred.shape)t2 = time_synchronized()print(f"infer time is {(t2-t1)*1000} ms")# Apply NMSpred = non_max_suppression_face(pred, self.conf_thres, self.iou_thres)# print(pred.shape)# Process detectionsfor i, det in enumerate(pred): # detections per imageif len(det):# Rescale boxes from img_size to im0 sizedet[:, :4] = scale_coords(img.shape[2:], det[:, :4], orgimg.shape).round()# Print resultsfor c in det[:, -1].unique():n = (det[:, -1] == c).sum() # detections per classdet[:, 5:13] = scale_coords_landmarks(img.shape[2:], det[:, 5:13], orgimg.shape).round()for j in range(det.size()[0]):xyxy = det[j, :4].view(-1).tolist()conf = det[j, 4].cpu().numpy()landmarks = det[j, 5:13].view(-1).tolist()class_num = det[j, 13].cpu().numpy()result_dict = get_plate_rec_landmark(orgimg, xyxy, conf, landmarks, class_num, self.device)dict_list.append(result_dict)return dict_listdef draw_result(self, orgimg, dict_list):'''返回绘制之后的原图'''for result in dict_list:rect_area = result['box']x, y, w, h = rect_area[0], rect_area[1], rect_area[2] - rect_area[0], rect_area[3] - rect_area[1]padding_w = 0.05 * wpadding_h = 0.11 * hrect_area[0] = max(0, int(x - padding_w))rect_area[1] = max(0, int(y - padding_h))rect_area[2] = min(orgimg.shape[1], int(rect_area[2] + padding_w))rect_area[3] = min(orgimg.shape[0], int(rect_area[3] + padding_h))landmarks = result['landmarks']label = result['class']# result_str+=result+" "for i in range(4): # 关键点cv2.circle(orgimg, (int(landmarks[i][0]), int(landmarks[i][1])), 5, clors[i], -1)cv2.rectangle(orgimg, (rect_area[0], rect_area[1]), (rect_area[2], rect_area[3]), clors[label], 2) # 画框cv2.putText(img, str(label), (rect_area[0], rect_area[1]), cv2.FONT_HERSHEY_SIMPLEX, 0.5, clors[label], 2)return orgimg
if __name__ == '__main__':det = Detect()img_dir = "images"save_dir = "result"if not os.path.exists(save_dir):os.makedirs(save_dir)for file in os.listdir(img_dir):img_path = os.path.join(img_dir,file)img = cv2.imdecode(np.fromfile(img_path, dtype=np.uint8), -1)dict_list = det.detect(img)# print(dict_list)result_img = det.draw_result(img, dict_list)save_dir_path = os.path.join(save_dir,file)cv2.imwrite(save_dir_path,result_img)
2.4.2 onnx推理
import argparse
import time, os
import torch
from detect import scale_coords_landmarks, get_plate_rec_landmark
from torch2trt.trt_model import TrtModel
from utils.general import non_max_suppression_face, scale_coords, check_img_size
import cv2
import copy
from utils.torch_utils import time_synchronized
import numpy as npclors = [(255, 0, 0), (0, 255, 0), (0, 0, 255), (255, 255, 0), (0, 255, 255)]def letterbox(img, size=(640, 640)):h, w, c = img.shape# 缩放因子ratio = min(size[0] / h, size[1] / w)new_h, new_w = int(h * ratio), int(w * ratio)top = int((size[0] - new_h) / 2)left = int((size[1] - new_w) / 2)bottom = size[0] - new_h - topright = size[1] - new_w - leftimg_resize = cv2.resize(img, (new_w, new_h))img = cv2.copyMakeBorder(img_resize, top, bottom, left, right, borderType=cv2.BORDER_CONSTANT,value=(114, 114, 114))return img, ratio, left, topdef nms(boxes, iou_thresh):# numpy nmsindex = np.argsort(boxes[:, 4])[::-1]keep = []while index.size > 0:i = index[0]keep.append(i)x1 = np.maximum(boxes[i, 0], boxes[index[1:], 0])y1 = np.maximum(boxes[i, 1], boxes[index[1:], 1])x2 = np.minimum(boxes[i, 2], boxes[index[1:], 2])y2 = np.minimum(boxes[i, 3], boxes[index[1:], 3])w = np.maximum(0, x2 - x1)h = np.maximum(0, y2 - y1)inter_area = w * hunion_area = (boxes[i, 2] - boxes[i, 0]) * (boxes[i, 3] - boxes[i, 1]) + (boxes[index[1:], 2] - boxes[index[1:], 0]) * (boxes[index[1:], 3] - boxes[index[1:], 1])iou = inter_area / (union_area - inter_area)idx = np.where(iou <= iou_thresh)[0]index = index[idx + 1]return keepdef restore_box(boxes, r, left, top): # 返回原图上面的坐标boxes[:, [0, 2, 5, 7, 9, 11]] -= leftboxes[:, [1, 3, 6, 8, 10, 12]] -= topboxes[:, [0, 2, 5, 7, 9, 11]] /= rboxes[:, [1, 3, 6, 8, 10, 12]] /= rreturn boxesdef post_precessing(dets, ratio, left, top, conf_thresh=0.3, iou_thresh=0.5): # 检测后处理# 选取大于置信度的choice = dets[:, :, 4] > conf_threshdets = dets[choice]dets[:, 13:15] *= dets[:, 4:5]box = dets[:, :4]boxes = xywh2xyxy(box)score = np.max(dets[:, 13:15], axis=-1, keepdims=True)index = np.argmax(dets[:, 13:15], axis=-1).reshape(-1, 1)output = np.concatenate((boxes, score, dets[:, 5:13], index), axis=1)reserve_ = nms(output, iou_thresh)output = output[reserve_]output = restore_box(output, ratio, left, top)return outputdef xywh2xyxy(boxes): # xywh坐标变为 左上 ,右下坐标 x1,y1 x2,y2xywh = copy.deepcopy(boxes)xywh[:, 0] = boxes[:, 0] - boxes[:, 2] / 2xywh[:, 1] = boxes[:, 1] - boxes[:, 3] / 2xywh[:, 2] = boxes[:, 0] + boxes[:, 2] / 2xywh[:, 3] = boxes[:, 1] + boxes[:, 3] / 2return xywhclass Detect:def __init__(self, conf_thres=0.6, iou_thres=0.5, img_size=640, trt_path="weights/best.trt"):self.conf_thres = conf_thresself.iou_thres = iou_thresself.img_size = img_sizeself.device = "cuda" if torch.cuda.is_available() else "cpu"self.detect_model = TrtModel(trt_path)def detect_processing(self, img, img_size=(640, 640)):img, ratio, left, top = letterbox(img, size=img_size)img = img[:, :, ::-1].transpose(2, 0, 1).copy().astype(np.float32)img = img / 255img = img.reshape(1, *img.shape)return img, ratio, left, topdef detect(self, orgimg):dict_list = []if orgimg is None:return []if orgimg.shape[-1] == 4:orgimg = cv2.cvtColor(orgimg, cv2.COLOR_BGRA2BGR)h0, w0 = orgimg.shape[:2] # orig hwimg0 = copy.deepcopy(orgimg)# imgsz = check_img_size(self.img_size, s=32) # check img_sizeimg, ratio, left, top = self.detect_processing(img0)if img.ndim == 3:img = img[None, ...]# Inferencet1 = time_synchronized()pred = self.detect_model(img).reshape([1, 25200, 15])output = post_precessing(pred, ratio, left, top, conf_thresh=self.conf_thres, iou_thresh=self.iou_thres)for output in output:result_dict = {}rect = output[:4].astype(int).tolist()land_marks = output[5:13].astype(int).reshape(4, 2)conf = output[4].astype(int).tolist()result_dict['box'] = rectresult_dict['class'] = confresult_dict['landmarks'] = land_marks.tolist()dict_list.append(result_dict)return dict_listdef draw_result(self, orgimg, dict_list):'''返回绘制之后的原图'''for result in dict_list:rect_area = result['box']x, y, w, h = rect_area[0], rect_area[1], rect_area[2] - rect_area[0], rect_area[3] - rect_area[1]padding_w = 0.05 * wpadding_h = 0.11 * hrect_area[0] = max(0, int(x - padding_w))rect_area[1] = max(0, int(y - padding_h))rect_area[2] = min(orgimg.shape[1], int(rect_area[2] + padding_w))rect_area[3] = min(orgimg.shape[0], int(rect_area[3] + padding_h))landmarks = result['landmarks']label = result['class']# result_str+=result+" "for i in range(4): # 关键点cv2.circle(orgimg, (int(landmarks[i][0]), int(landmarks[i][1])), 5, clors[i], -1)cv2.rectangle(orgimg, (rect_area[0], rect_area[1]), (rect_area[2], rect_area[3]), clors[label], 2) # 画框cv2.putText(img, str(label), (rect_area[0], rect_area[1]), cv2.FONT_HERSHEY_SIMPLEX, 0.5, clors[label], 2)return orgimgdef __del__(self):self.detect_model.destroy()if __name__ == '__main__':# ============可视化================# img_vis(img, orgimg, pred)det = Detect()img_dir = "images"save_dir = "result"if not os.path.exists(save_dir):os.makedirs(save_dir)for file in os.listdir(img_dir):img_path = os.path.join(img_dir, file)img = cv2.imdecode(np.fromfile(img_path, dtype=np.uint8), -1)dict_list = det.detect(img)print(dict_list)result_img = det.draw_result(img, dict_list)save_dir_path = os.path.join(save_dir, file)cv2.imwrite(save_dir_path, result_img)
2.4.3 trt推理
import argparse
import time,os
import torch
from detect import scale_coords_landmarks, get_plate_rec_landmark
from torch2trt.trt_model import TrtModel
from utils.datasets import letterbox
from utils.general import non_max_suppression_face, scale_coords, check_img_size
import cv2
import copy
from utils.torch_utils import time_synchronized
import numpy as np
clors = [(255, 0, 0), (0, 255, 0), (0, 0, 255), (255, 255, 0), (0, 255, 255)]
def img_process(img_path,long_side=640,stride_max=32):'''图像预处理'''orgimg=cv2.imread(img_path)img0 = copy.deepcopy(orgimg)h0, w0 = orgimg.shape[:2] # orig hwr = long_side/ max(h0, w0) # resize image to img_sizeif r != 1: # always resize down, only resize up if training with augmentationinterp = cv2.INTER_AREA if r < 1 else cv2.INTER_LINEARimg0 = cv2.resize(img0, (int(w0 * r), int(h0 * r)), interpolation=interp)imgsz = check_img_size(long_side, s=stride_max) # check img_sizeimg = letterbox(img0, new_shape=imgsz,auto=False)[0] # auto True最小矩形 False固定尺度# Convertimg = img[:, :, ::-1].transpose(2, 0, 1).copy() # BGR to RGB, to 3x416x416img = torch.from_numpy(img)img = img.float() # uint8 to fp16/32img /= 255.0 # 0 - 255 to 0.0 - 1.0if img.ndimension() == 3:img = img.unsqueeze(0)return img,orgimgclass Detect:def __init__(self, conf_thres=0.6, iou_thres=0.5, img_size=640,trt_path="weights/best.trt"):self.conf_thres = conf_thresself.iou_thres = iou_thresself.img_size = img_sizeself.device = "cuda" if torch.cuda.is_available() else "cpu"self.detect_model = TrtModel(trt_path)def detect(self, orgimg):dict_list = []if orgimg is None:return []if orgimg.shape[-1] == 4:orgimg = cv2.cvtColor(orgimg, cv2.COLOR_BGRA2BGR)h0, w0 = orgimg.shape[:2] # orig hwimg0 = copy.deepcopy(orgimg)r = self.img_size / max(h0, w0) # resize image to img_sizeif r != 1: # always resize down, only resize up if training with augmentationinterp = cv2.INTER_AREA if r < 1 else cv2.INTER_LINEARimg0 = cv2.resize(img0, (int(w0 * r), int(h0 * r)), interpolation=interp)imgsz = check_img_size(self.img_size, s=32) # check img_sizeimg = letterbox(img0, new_shape=imgsz,auto=False)[0]# Convertimg = img[:, :, ::-1].transpose(2, 0, 1).copy() # BGR to RGB, to 3x416x416# Run inferencet0 = time.time()# img = torch.from_numpy(img).to(self.device)img = img.astype(float) # uint8 to fp16/32img /= 255.0 # 0 - 255 to 0.0 - 1.0if img.ndim == 3:img = img[None,...]# Inferencet1 = time_synchronized()pred = self.detect_model(img).reshape([1, 25200, 15])t2 = time_synchronized()print(f"infer time is {(t2-t1)*1000} ms")# Apply NMS# pred = torch.tensor(pred)pred = non_max_suppression_face(torch.tensor(pred), self.conf_thres, self.iou_thres)# print(type(pred))# Process detectionsfor i, det in enumerate(pred): # detections per imageif len(det):# Rescale boxes from img_size to im0 size# print(type(det))det[:, :4] = scale_coords(img.shape[2:], det[:, :4], orgimg.shape).round()# Print resultsfor c in det[:, -1].unique():n = (det[:, -1] == c).sum() # detections per classdet[:, 5:13] = scale_coords_landmarks(img.shape[2:], det[:, 5:13], orgimg.shape).round()for j in range(det.size()[0]):xyxy = det[j, :4].view(-1).tolist()conf = det[j, 4].cpu().numpy()landmarks = det[j, 5:13].view(-1).tolist()class_num = det[j, 13].cpu().numpy()result_dict = get_plate_rec_landmark(orgimg, xyxy, conf, landmarks, class_num, self.device)dict_list.append(result_dict)return dict_listdef draw_result(self, orgimg, dict_list):'''返回绘制之后的原图'''for result in dict_list:rect_area = result['box']x, y, w, h = rect_area[0], rect_area[1], rect_area[2] - rect_area[0], rect_area[3] - rect_area[1]padding_w = 0.05 * wpadding_h = 0.11 * hrect_area[0] = max(0, int(x - padding_w))rect_area[1] = max(0, int(y - padding_h))rect_area[2] = min(orgimg.shape[1], int(rect_area[2] + padding_w))rect_area[3] = min(orgimg.shape[0], int(rect_area[3] + padding_h))landmarks = result['landmarks']label = result['class']# result_str+=result+" "for i in range(4): # 关键点cv2.circle(orgimg, (int(landmarks[i][0]), int(landmarks[i][1])), 5, clors[i], -1)cv2.rectangle(orgimg, (rect_area[0], rect_area[1]), (rect_area[2], rect_area[3]), clors[label], 2) # 画框cv2.putText(img, str(label), (rect_area[0], rect_area[1]), cv2.FONT_HERSHEY_SIMPLEX, 0.5, clors[label], 2)return orgimgdef __del__(self):self.detect_model.destroy()if __name__ == '__main__':# ============可视化================# img_vis(img, orgimg, pred)det = Detect()img_dir = "images"save_dir = "result"if not os.path.exists(save_dir):os.makedirs(save_dir)for file in os.listdir(img_dir):img_path = os.path.join(img_dir, file)img = cv2.imdecode(np.fromfile(img_path, dtype=np.uint8), -1)dict_list = det.detect(img)print(dict_list)result_img = det.draw_result(img, dict_list)save_dir_path = os.path.join(save_dir, file)cv2.imwrite(save_dir_path, result_img)
2.4.4 numpy版本trt推理
import argparse
import time, os
import torch
from detect import scale_coords_landmarks, get_plate_rec_landmark
from torch2trt.trt_model import TrtModel
from utils.general import non_max_suppression_face, scale_coords, check_img_size
import cv2
import copy
from utils.torch_utils import time_synchronized
import numpy as np
clors = [(255, 0, 0), (0, 255, 0), (0, 0, 255), (255, 255, 0), (0, 255, 255)]
def letterbox(img, size=(640, 640)):h, w, c = img.shape# 缩放因子ratio = min(size[0] / h, size[1] / w)new_h, new_w = int(h * ratio), int(w * ratio)top = int((size[0] - new_h) / 2)left = int((size[1] - new_w) / 2)bottom = size[0] - new_h - topright = size[1] - new_w - leftimg_resize = cv2.resize(img, (new_w, new_h))img = cv2.copyMakeBorder(img_resize, top, bottom, left, right, borderType=cv2.BORDER_CONSTANT,value=(114, 114, 114))return img, ratio, left, topdef nms(boxes, iou_thresh):# numpy nmsindex = np.argsort(boxes[:, 4])[::-1]keep = []while index.size > 0:i = index[0]keep.append(i)x1 = np.maximum(boxes[i, 0], boxes[index[1:], 0])y1 = np.maximum(boxes[i, 1], boxes[index[1:], 1])x2 = np.minimum(boxes[i, 2], boxes[index[1:], 2])y2 = np.minimum(boxes[i, 3], boxes[index[1:], 3])w = np.maximum(0, x2 - x1)h = np.maximum(0, y2 - y1)inter_area = w * hunion_area = (boxes[i, 2] - boxes[i, 0]) * (boxes[i, 3] - boxes[i, 1]) + (boxes[index[1:], 2] - boxes[index[1:], 0]) * (boxes[index[1:], 3] - boxes[index[1:], 1])iou = inter_area / (union_area - inter_area)idx = np.where(iou <= iou_thresh)[0]index = index[idx + 1]return keepdef restore_box(boxes, r, left, top): # 返回原图上面的坐标boxes[:, [0, 2, 5, 7, 9, 11]] -= leftboxes[:, [1, 3, 6, 8, 10, 12]] -= topboxes[:, [0, 2, 5, 7, 9, 11]] /= rboxes[:, [1, 3, 6, 8, 10, 12]] /= rreturn boxesdef post_precessing(dets, ratio, left, top, conf_thresh=0.3, iou_thresh=0.5): # 检测后处理# 选取大于置信度的choice = dets[:, :, 4] > conf_threshdets = dets[choice]dets[:, 13:15] *= dets[:, 4:5]box = dets[:, :4]boxes = xywh2xyxy(box)score = np.max(dets[:, 13:15], axis=-1, keepdims=True)index = np.argmax(dets[:, 13:15], axis=-1).reshape(-1, 1)output = np.concatenate((boxes, score, dets[:, 5:13], index), axis=1)reserve_ = nms(output, iou_thresh)output = output[reserve_]output = restore_box(output, ratio, left, top)return outputdef xywh2xyxy(boxes): # xywh坐标变为 左上 ,右下坐标 x1,y1 x2,y2xywh = copy.deepcopy(boxes)xywh[:, 0] = boxes[:, 0] - boxes[:, 2] / 2xywh[:, 1] = boxes[:, 1] - boxes[:, 3] / 2xywh[:, 2] = boxes[:, 0] + boxes[:, 2] / 2xywh[:, 3] = boxes[:, 1] + boxes[:, 3] / 2return xywhclass Detect:def __init__(self, conf_thres=0.6, iou_thres=0.5, img_size=640, trt_path="weights/best.trt"):self.conf_thres = conf_thresself.iou_thres = iou_thresself.img_size = img_sizeself.device = "cuda" if torch.cuda.is_available() else "cpu"self.detect_model = TrtModel(trt_path)def detect_processing(self, img, img_size=(640, 640)):img, ratio, left, top = letterbox(img, size=img_size)img = img[:, :, ::-1].transpose(2, 0, 1).copy().astype(np.float32)img = img / 255img = img.reshape(1, *img.shape)return img, ratio, left, topdef detect(self, orgimg):dict_list = []if orgimg is None:return []if orgimg.shape[-1] == 4:orgimg = cv2.cvtColor(orgimg, cv2.COLOR_BGRA2BGR)h0, w0 = orgimg.shape[:2] # orig hwimg0 = copy.deepcopy(orgimg)# imgsz = check_img_size(self.img_size, s=32) # check img_sizeimg, ratio, left, top = self.detect_processing(img0)if img.ndim == 3:img = img[None, ...]# Inferencet1 = time_synchronized()pred = self.detect_model(img).reshape([1, 25200, 15])output = post_precessing(pred, ratio, left, top, conf_thresh=self.conf_thres, iou_thresh=self.iou_thres)for output in output:result_dict = {}rect = output[:4].astype(int).tolist()land_marks = output[5:13].astype(int).reshape(4, 2)conf = output[4].astype(int).tolist()result_dict['box'] = rectresult_dict['class'] = confresult_dict['landmarks'] = land_marks.tolist()dict_list.append(result_dict)return dict_listdef draw_result(self, orgimg, dict_list):'''返回绘制之后的原图'''for result in dict_list:rect_area = result['box']x, y, w, h = rect_area[0], rect_area[1], rect_area[2] - rect_area[0], rect_area[3] - rect_area[1]padding_w = 0.05 * wpadding_h = 0.11 * hrect_area[0] = max(0, int(x - padding_w))rect_area[1] = max(0, int(y - padding_h))rect_area[2] = min(orgimg.shape[1], int(rect_area[2] + padding_w))rect_area[3] = min(orgimg.shape[0], int(rect_area[3] + padding_h))landmarks = result['landmarks']label = result['class']# result_str+=result+" "for i in range(4): # 关键点cv2.circle(orgimg, (int(landmarks[i][0]), int(landmarks[i][1])), 5, clors[i], -1)cv2.rectangle(orgimg, (rect_area[0], rect_area[1]), (rect_area[2], rect_area[3]), clors[label], 2) # 画框cv2.putText(img, str(label), (rect_area[0], rect_area[1]), cv2.FONT_HERSHEY_SIMPLEX, 0.5, clors[label], 2)return orgimgdef __del__(self):self.detect_model.destroy()if __name__ == '__main__':# ============可视化================# img_vis(img, orgimg, pred)det = Detect()img_dir = "images"save_dir = "result"if not os.path.exists(save_dir):os.makedirs(save_dir)for file in os.listdir(img_dir):img_path = os.path.join(img_dir, file)img = cv2.imdecode(np.fromfile(img_path, dtype=np.uint8), -1)dict_list = det.detect(img)print(dict_list)result_img = det.draw_result(img, dict_list)save_dir_path = os.path.join(save_dir, file)cv2.imwrite(save_dir_path, result_img)
2.4.5 推理结果展示
参考开源
Chinese_license_plate_detection_recognition
相关文章:
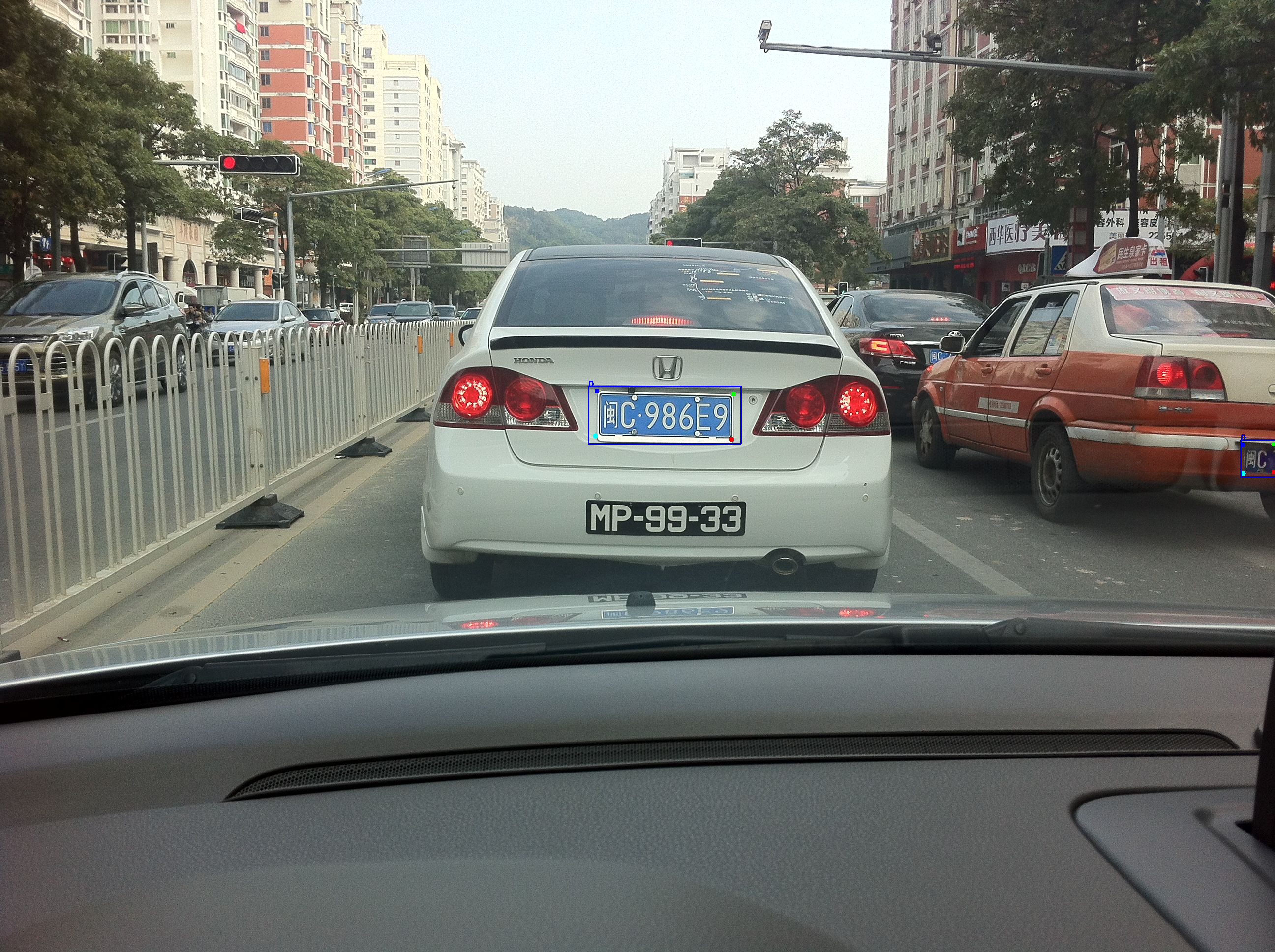
计算机视觉基础——基于yolov5-face算法的车牌检测
文章目录 车牌检测算法检测实现1.环境布置2.数据处理2.1 CCPD数据集介绍2.1.1 ccpd2019及20202.1.2 文件名字解析 2.2数据集处理2.2.1 CCPD数据处理2.2.2 CPRD数据集处理 2.3 检测算法2.3.1 数据配置car_plate.yaml2.3.2 模型配置2.3.3 train.py2.3.4 训练结果 2.4 部署2.4.1 p…...

【好书推荐】AI时代架构师修炼之道:ChatGPT让架构师插上翅膀
目录 前言 ChatGPT对架构师工作的帮助 快速理解和分析需求 提供代码建议和解决方案 辅助系统设计和优化 提高团队协作效率 如何使用ChatGPT提高架构师工作效率 了解用户需求和分析问题 编码实践和问题解决 系统设计和优化建议 团队协作和沟通效率提升 知识管理和文…...
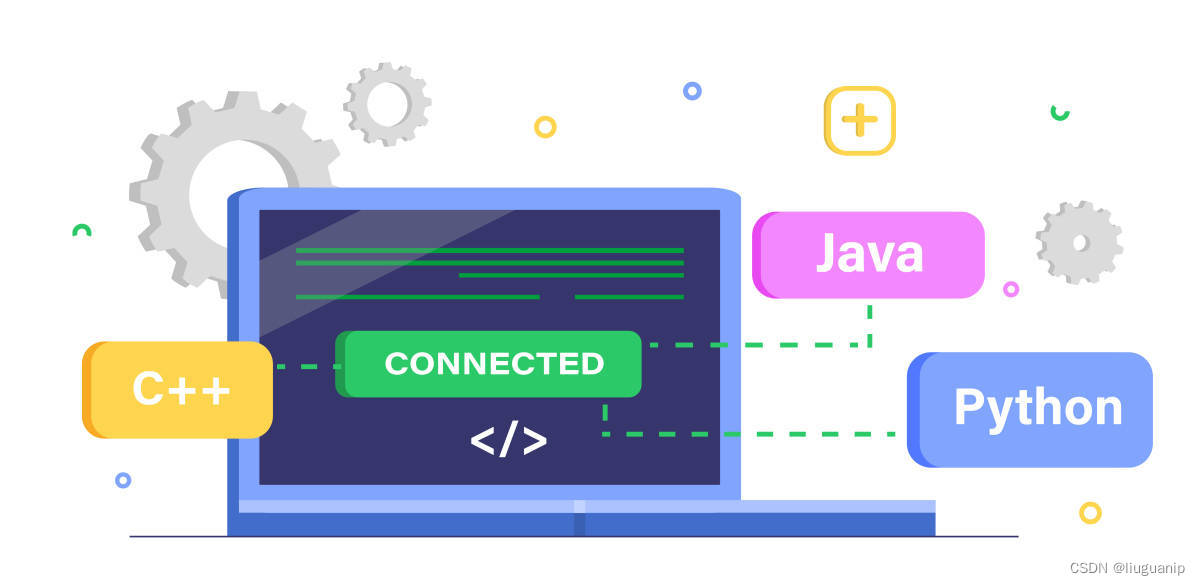
全局代理和局部代理的区别
在计算机领域中,代理是一种常见的网络技术,它可以帮助用户更好地控制网络访问和数据传输。代理可以分为全局代理和局部代理两种,它们有着不同的作用和适用场景。 一、全局代理 全局代理指的是在系统级别设置的代理,它可以代理所…...
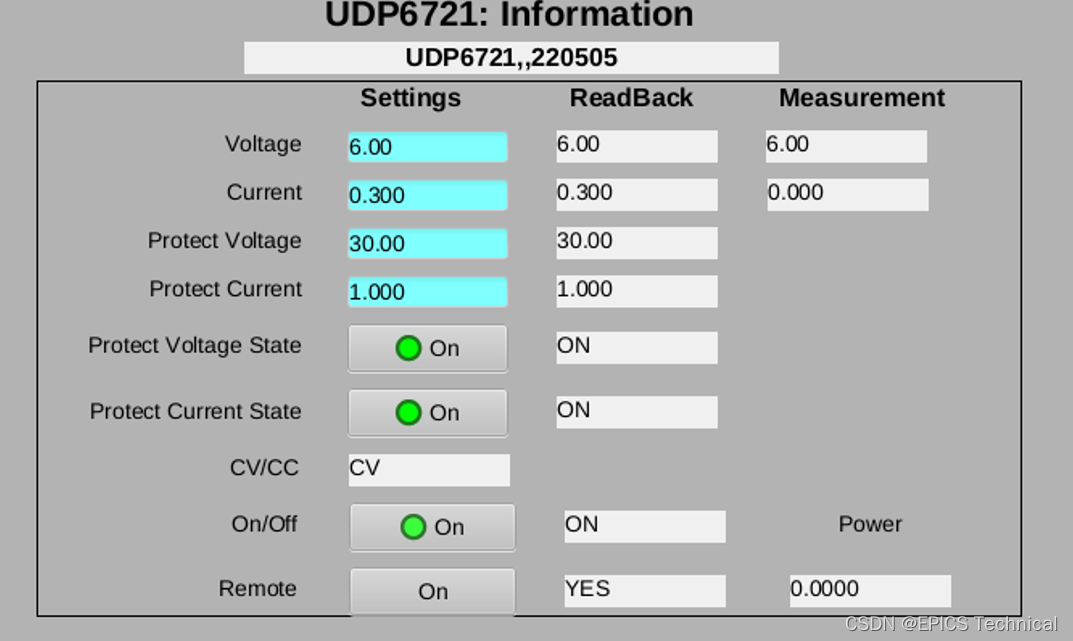
基于EPICS stream模块的直流电源的IOC控制程序实例
本实例程序实现了对优利德UDP6720系列直流电源的网络控制和访问,先在此介绍这个项目中使用的硬件: 1、UDP6721直流电源:受控设备 2、moxa串口服务器5150:将UDP6721直流电源设备串口连接转成网络连接 3、香橙派Zero3:运…...
Unity3D ECS架构适合作为主架构还是局部架构
前言 前言 Unity3D是一款广泛应用于游戏开发的跨平台游戏引擎,提供了丰富的功能和工具来简化游戏开发的过程。而Entity-Component-System(ECS)架构则是一种面向数据的设计模式,它将游戏对象(Entity)分解为…...
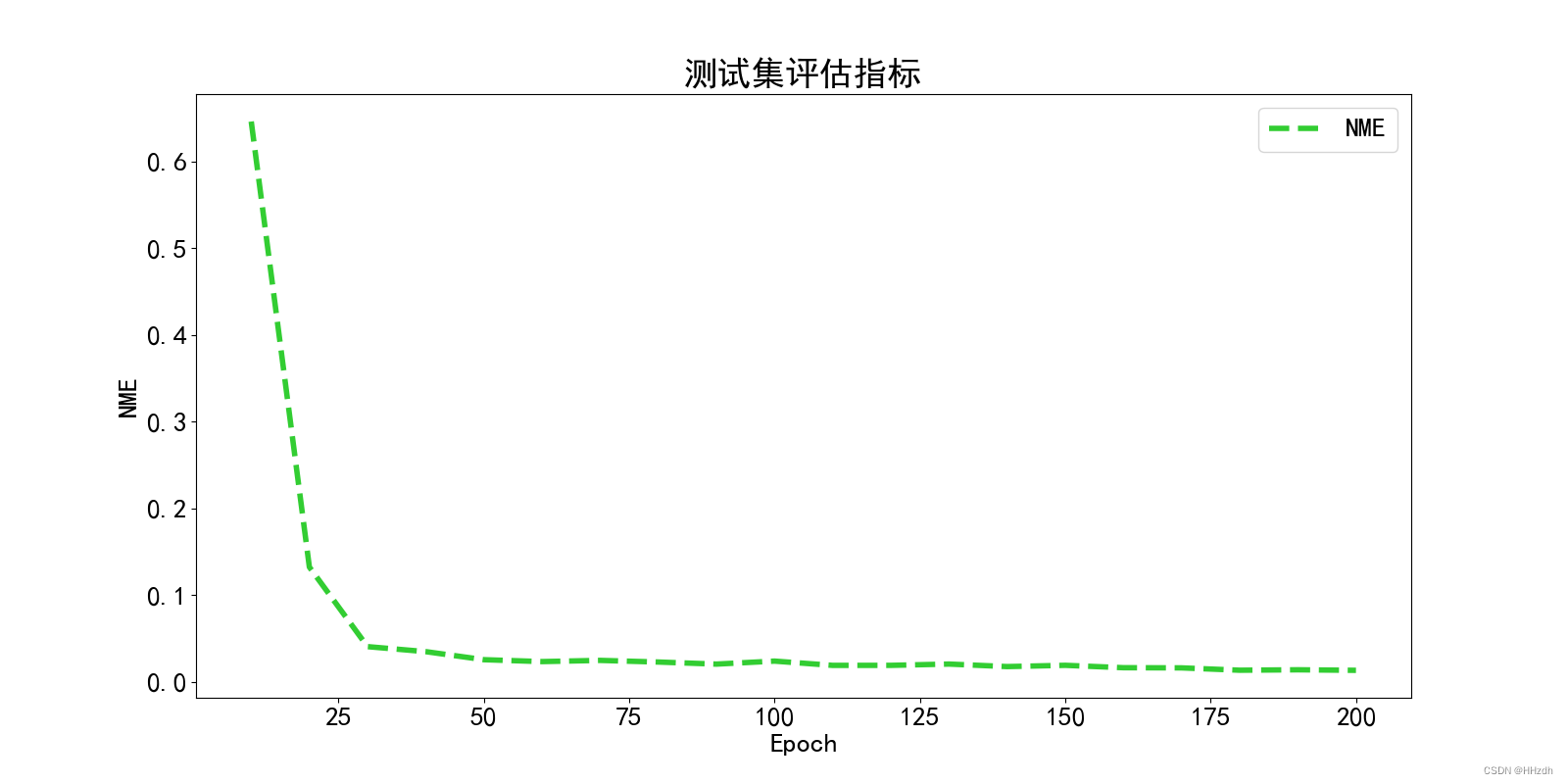
从零开始的目标检测和关键点检测(三):训练一个Glue的RTMPose模型
从零开始的目标检测和关键点检测(三):训练一个Glue的RTMPose模型 一、重写config文件二、开始训练三、ncnn部署 从零开始的目标检测和关键点检测(一):用labelme标注数据集 从零开始的目标检测和关键点检测…...
Qt6 中弹出消息框,一段时间后自动退出
以下代码功能,弹出模态消息框,然后,等待 3 秒,消息框自动退出 QMessageBox msgbox;msgbox.setText("sleep 3s");QTimer::singleShot(3000, &msgbox, &QMessageBox::close);msgbox.exec();...
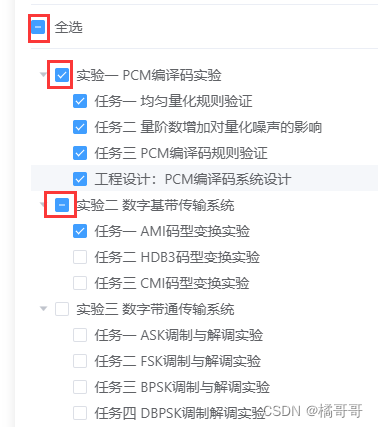
elementUI树节点全选,反选,半选状态
// <template>部分 <div class"check-block"><el-divider></el-divider><el-checkbox :indeterminate"indeterminate" v-model"checkAll" change"handleCheckAllChange">全选</el-checkbox><e…...
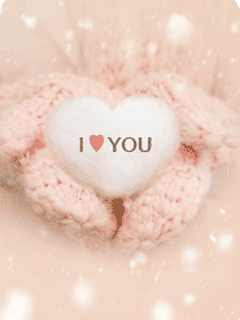
Kafka、RabbitMQ、RocketMQ中间件的对比
消息中间件现在有不少,网上很多文章都对其做过对比,在这我对其做进一步总结与整理。 RocketMQ 淘宝内部的交易系统使用了淘宝自主研发的Notify消息中间件,使用Mysql作为消息存储媒介,可完全水平扩容,为了进一步降低成…...
Mac 创建并使用 .zshrc 文件
1,打开终端输入指令 touch .zshrc 2,你可能希望将 .bash_profile 文件中的内容复制到 .zshrc 文件中,那建议复制过来。 3,使用 .zshrc 文件 执行以下指令: source .zshrc 注:以后希望使用 .bash_prof…...
Unity3D移动开发如何依据性能选择Shader
前言 在Unity3D移动开发中,选择合适的Shader是非常重要的,它直接影响到游戏的性能和画面效果。本文将介绍如何依据性能选择Shader,并给出相应的技术详解以及代码实现。 对惹,这里有一个游戏开发交流小组,希望大家可以…...
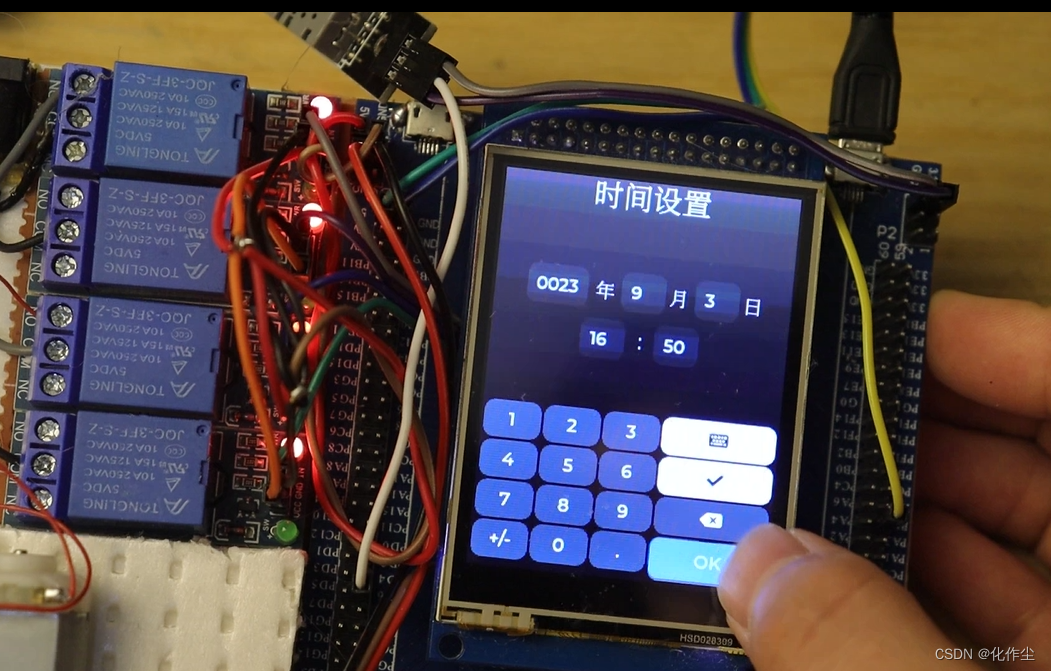
基于stm32F4的智能宠物喂食器的设计:LVGL界面、定时喂食喂水通风
宠物喂食器 一、功能设计二、元器件选型三、UI设计四、原理图设计五、源代码设计六、成品展示 实物链接:https://m.tb.cn/h.5iCUX6H?tkPL65WXCEipQ CZ3457 一、功能设计 1、设计一个触摸屏作为人机交互 2、通过触摸屏设置时间定时喂食喂水通风 3、获取当前水槽的…...
jumpserver堡垒机docker方式安装部署
1、环境要求 请先自行创建 数据库 和 Redis, 版本要求参考上面环境要求说明 mysql>5.7 redis >5.0 2、创建数据库 mysql: create database jumpserver default charset utf8; GRANT ALL PRIVILEGES ON jumpserver.* TO jumpserver% IDENTIFIED BY nu4x599…...
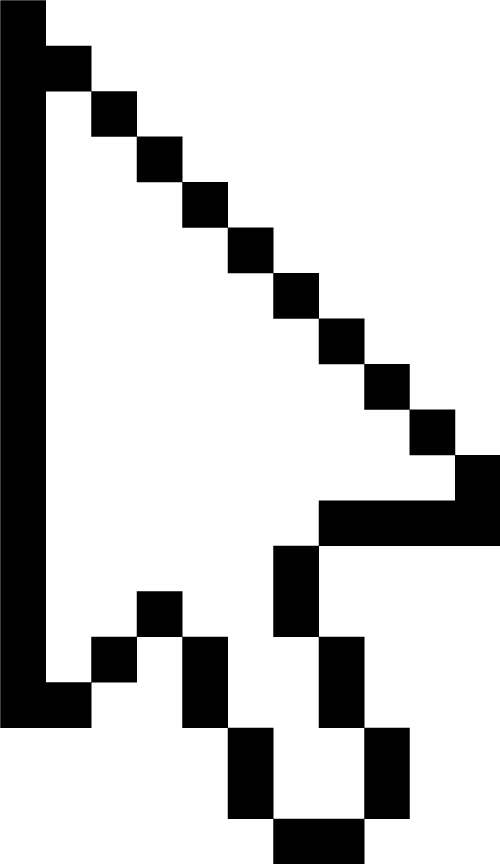
在基于亚马逊云科技的湖仓一体架构上构建数据血缘的探索和实践
背景介绍 随着大数据技术的进步,企业和组织越来越依赖数据驱动的决策。数据的质量、来源及其流动性因此显得非常关键。数据血缘分析为我们提供了一种追踪数据从起点到终点的方法,有助于理解数据如何被转换和消费,同时对数据治理和合规性起到关…...
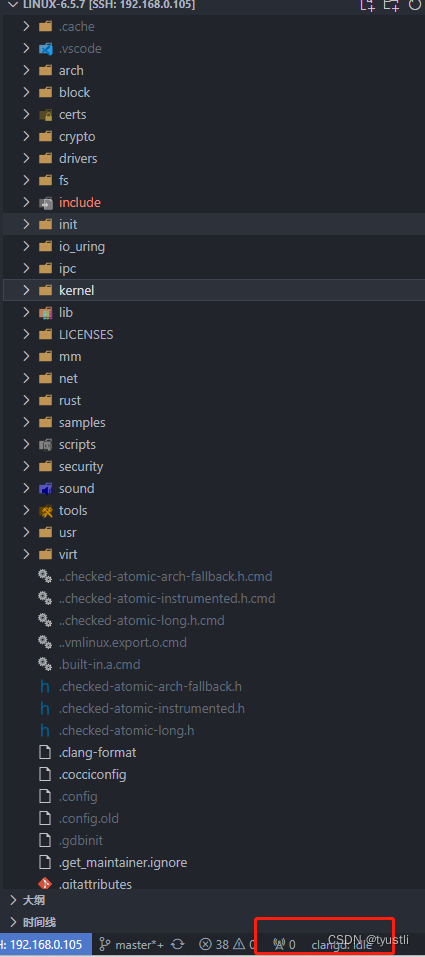
VScode clangd 插件浏览 linux 源码
文章目录 VScode clangd 插件浏览 linux 源码clangd 安装与配置VScode 插件安装clangd 安装方法一方法二 clangd 配置 cmake 生成bear 生成 compile_commands.json触发 clangd linux 内核脚本生成 compile_commands.json 文件三种方式对比 VScode clangd 插件浏览 linux 源码 …...
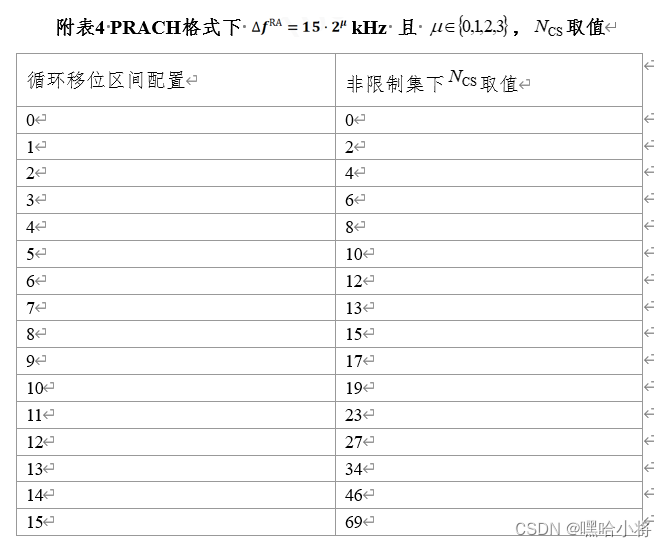
GZ035 5G组网与运维赛题第8套
2023年全国职业院校技能大赛 GZ035 5G组网与运维赛项(高职组) 赛题第8套 一、竞赛须知 1.竞赛内容分布 竞赛模块1--5G公共网络规划部署与开通(35分) 子任务1:5G公共网络部署与调试(15分) 子…...
《golang设计模式》第三部分·行为型模式-02-命令模式(Command)
文章目录 1. 概述1.1 角色1.2 类图 2. 代码示例2.1 设计2.2 代码2.3 类图 1. 概述 命令模式(Command)将类的业务行为以对象的方式封装,以便实现行为的参数化、撤销或重做等需求。 非命令模式的困惑: 类的行为在运行时是以实例方法…...

【linux进程控制(一)】进程终止--如何干掉一个进程?
💓博主CSDN主页:杭电码农-NEO💓 ⏩专栏分类:Linux从入门到精通⏪ 🚚代码仓库:NEO的学习日记🚚 🌹关注我🫵带你学更多操作系统知识 🔝🔝 进程终止 1. 前言2. 文章整体…...
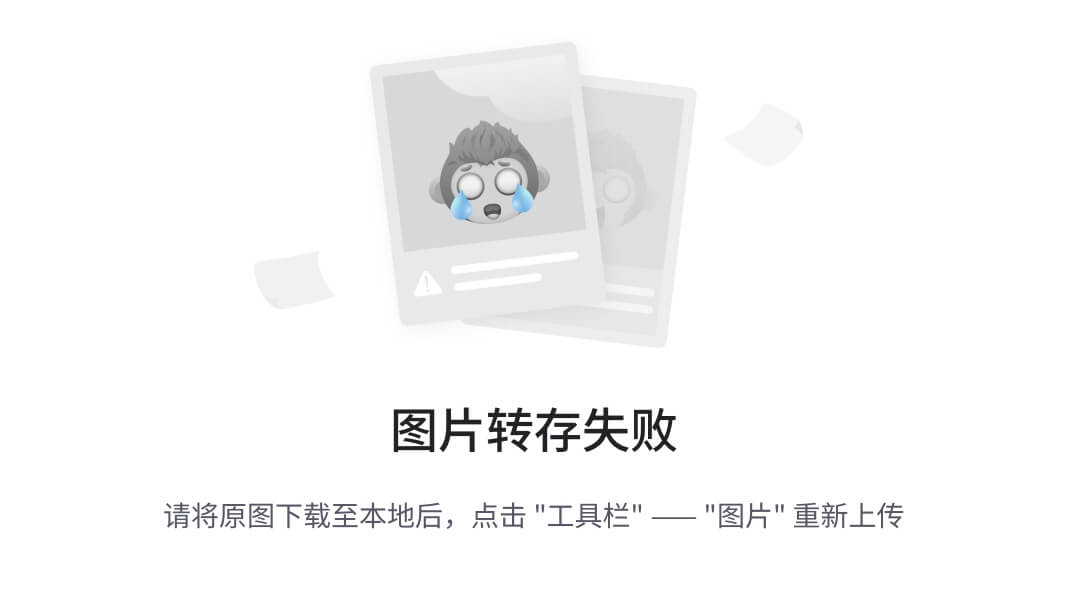
言情小说怎么推广?如何推广网络小说?
网络小说是一种文学形式,它的受众群体相当广泛,其实也面临着很强的竞争,因此,网络推广是小说宣传的一项重要工作,这里小马识途营销顾问就分享一下小说推广的渠道和方法。 1、软文推广 在推广小说的过程中,…...
TensorFlow 的应用场景有哪些
TensorFlow是一个开源的人工智能框架,由Google公司开发。它是一个强大的工具,可以用于数值计算、机器学习和深度学习等领域,具有灵活性、可扩展性、可移植性等特点。 TensorFlow的基本概念包括: Tensor:Tensor是Tens…...
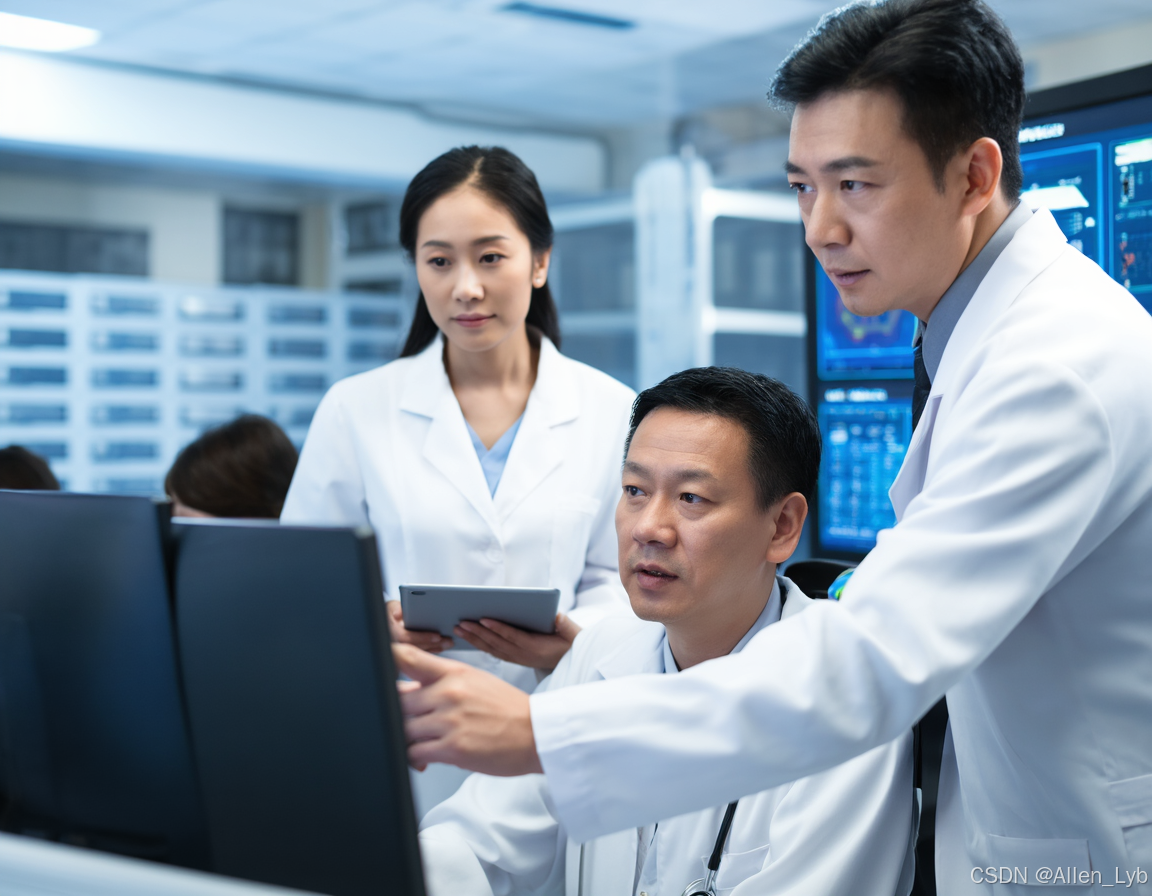
智慧医疗能源事业线深度画像分析(上)
引言 医疗行业作为现代社会的关键基础设施,其能源消耗与环境影响正日益受到关注。随着全球"双碳"目标的推进和可持续发展理念的深入,智慧医疗能源事业线应运而生,致力于通过创新技术与管理方案,重构医疗领域的能源使用模式。这一事业线融合了能源管理、可持续发…...
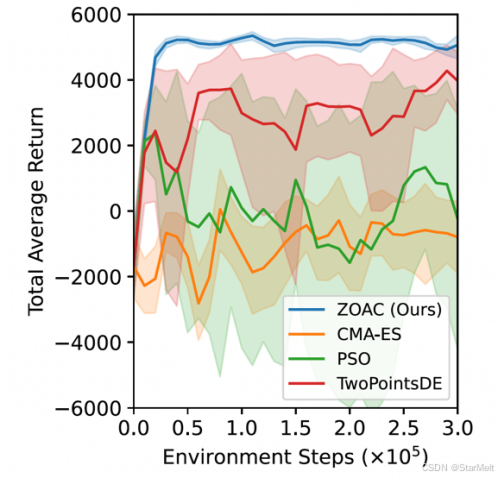
突破不可导策略的训练难题:零阶优化与强化学习的深度嵌合
强化学习(Reinforcement Learning, RL)是工业领域智能控制的重要方法。它的基本原理是将最优控制问题建模为马尔可夫决策过程,然后使用强化学习的Actor-Critic机制(中文译作“知行互动”机制),逐步迭代求解…...
Admin.Net中的消息通信SignalR解释
定义集线器接口 IOnlineUserHub public interface IOnlineUserHub {/// 在线用户列表Task OnlineUserList(OnlineUserList context);/// 强制下线Task ForceOffline(object context);/// 发布站内消息Task PublicNotice(SysNotice context);/// 接收消息Task ReceiveMessage(…...
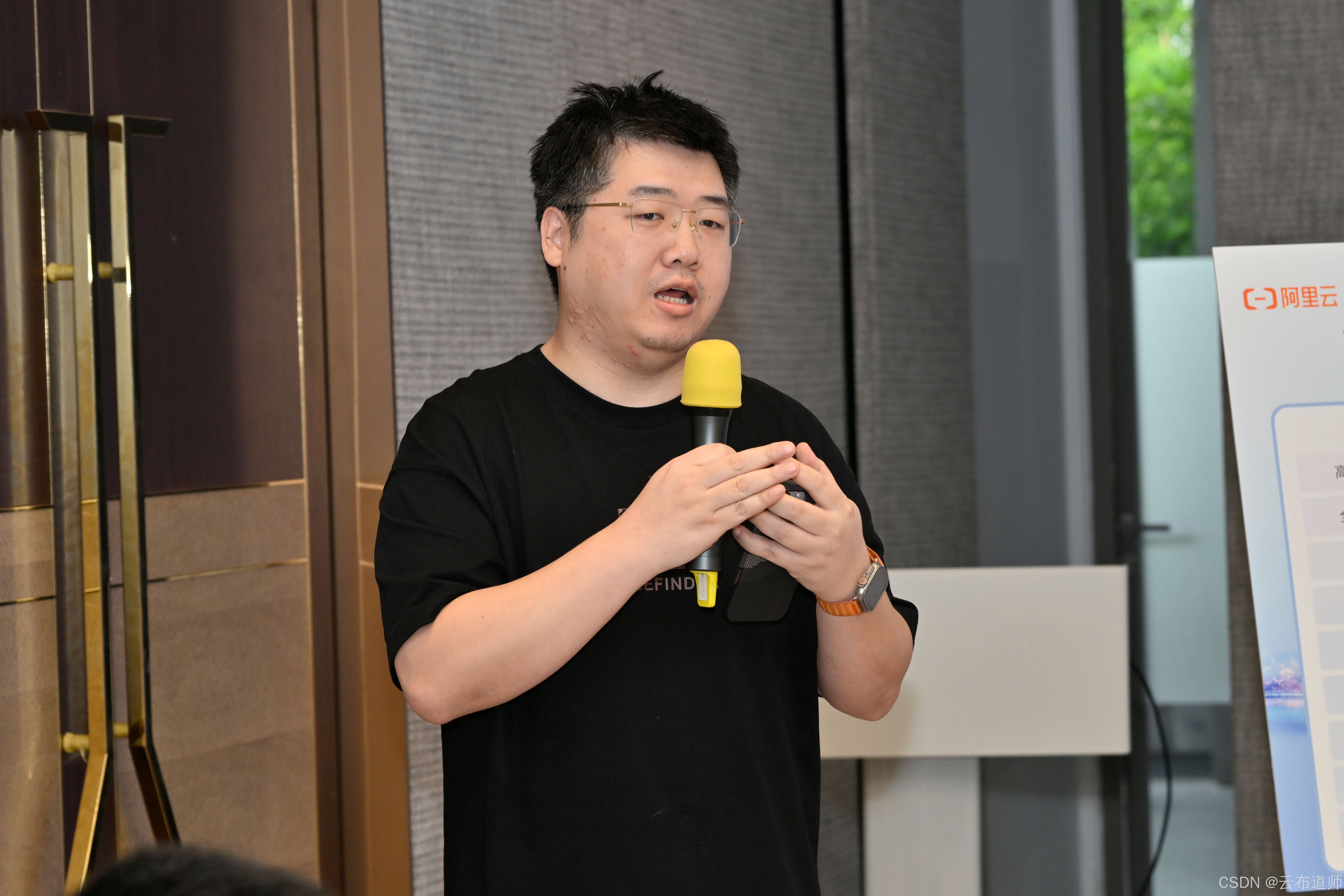
云启出海,智联未来|阿里云网络「企业出海」系列客户沙龙上海站圆满落地
借阿里云中企出海大会的东风,以**「云启出海,智联未来|打造安全可靠的出海云网络引擎」为主题的阿里云企业出海客户沙龙云网络&安全专场于5.28日下午在上海顺利举办,现场吸引了来自携程、小红书、米哈游、哔哩哔哩、波克城市、…...
IGP(Interior Gateway Protocol,内部网关协议)
IGP(Interior Gateway Protocol,内部网关协议) 是一种用于在一个自治系统(AS)内部传递路由信息的路由协议,主要用于在一个组织或机构的内部网络中决定数据包的最佳路径。与用于自治系统之间通信的 EGP&…...
MVC 数据库
MVC 数据库 引言 在软件开发领域,Model-View-Controller(MVC)是一种流行的软件架构模式,它将应用程序分为三个核心组件:模型(Model)、视图(View)和控制器(Controller)。这种模式有助于提高代码的可维护性和可扩展性。本文将深入探讨MVC架构与数据库之间的关系,以…...
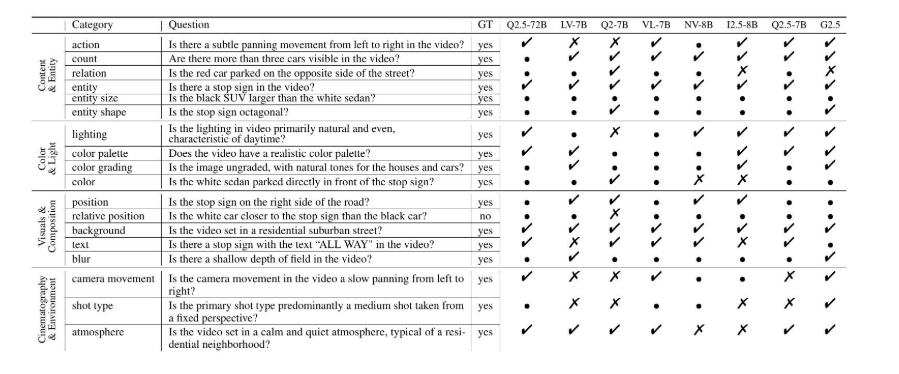
视频字幕质量评估的大规模细粒度基准
大家读完觉得有帮助记得关注和点赞!!! 摘要 视频字幕在文本到视频生成任务中起着至关重要的作用,因为它们的质量直接影响所生成视频的语义连贯性和视觉保真度。尽管大型视觉-语言模型(VLMs)在字幕生成方面…...
LLM基础1_语言模型如何处理文本
基于GitHub项目:https://github.com/datawhalechina/llms-from-scratch-cn 工具介绍 tiktoken:OpenAI开发的专业"分词器" torch:Facebook开发的强力计算引擎,相当于超级计算器 理解词嵌入:给词语画"…...

Ascend NPU上适配Step-Audio模型
1 概述 1.1 简述 Step-Audio 是业界首个集语音理解与生成控制一体化的产品级开源实时语音对话系统,支持多语言对话(如 中文,英文,日语),语音情感(如 开心,悲伤)&#x…...
Pydantic + Function Calling的结合
1、Pydantic Pydantic 是一个 Python 库,用于数据验证和设置管理,通过 Python 类型注解强制执行数据类型。它广泛用于 API 开发(如 FastAPI)、配置管理和数据解析,核心功能包括: 数据验证:通过…...