Seurat 中的数据可视化方法
本文[1]将使用从 2,700 PBMC 教程计算的 Seurat 对象来演示 Seurat 中的可视化技术。您可以从 SeuratData[2] 下载此数据集。
SeuratData::InstallData("pbmc3k")
library(Seurat)
library(SeuratData)
library(ggplot2)
library(patchwork)
pbmc3k.final <- LoadData("pbmc3k", type = "pbmc3k.final")
pbmc3k.final$groups <- sample(c("group1", "group2"), size = ncol(pbmc3k.final), replace = TRUE)
features <- c("LYZ", "CCL5", "IL32", "PTPRCAP", "FCGR3A", "PF4")
pbmc3k.final
## An object of class Seurat
## 13714 features across 2638 samples within 1 assay
## Active assay: RNA (13714 features, 2000 variable features)
## 3 layers present: data, counts, scale.data
## 2 dimensional reductions calculated: pca, umap
marker 特征表达的五种可视化
1. RidgePlot
# Ridge plots - from ggridges. Visualize single cell expression distributions in each cluster
RidgePlot(pbmc3k.final, features = features, ncol = 2)
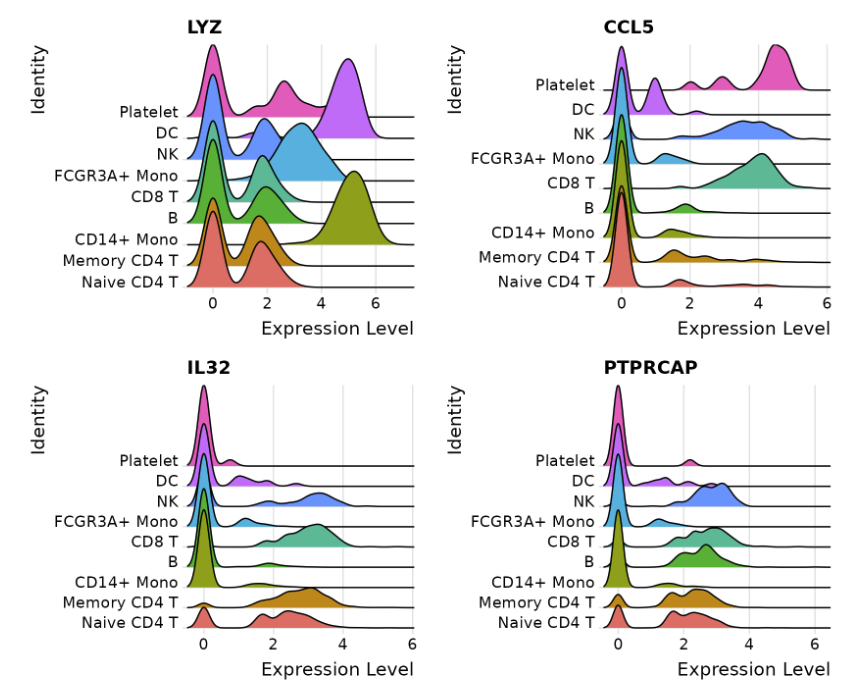
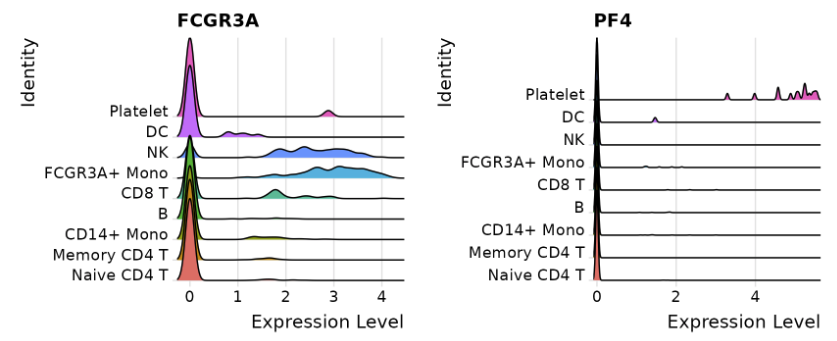
2. VlnPlot
# Violin plot - Visualize single cell expression distributions in each cluster
VlnPlot(pbmc3k.final, features = features)
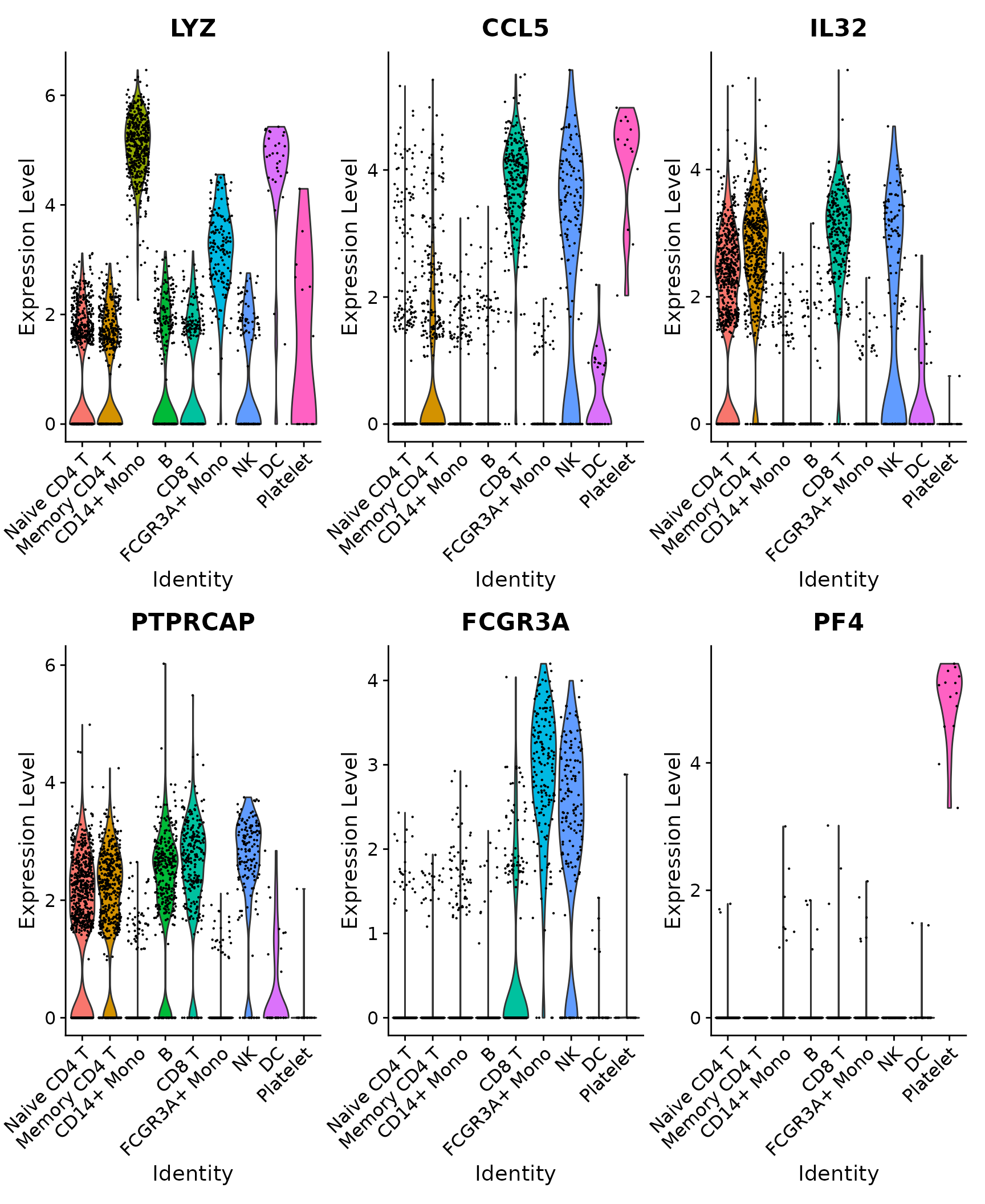
# Violin plots can also be split on some variable. Simply add the splitting variable to object
# metadata and pass it to the split.by argument
VlnPlot(pbmc3k.final, features = "percent.mt", split.by = "groups")
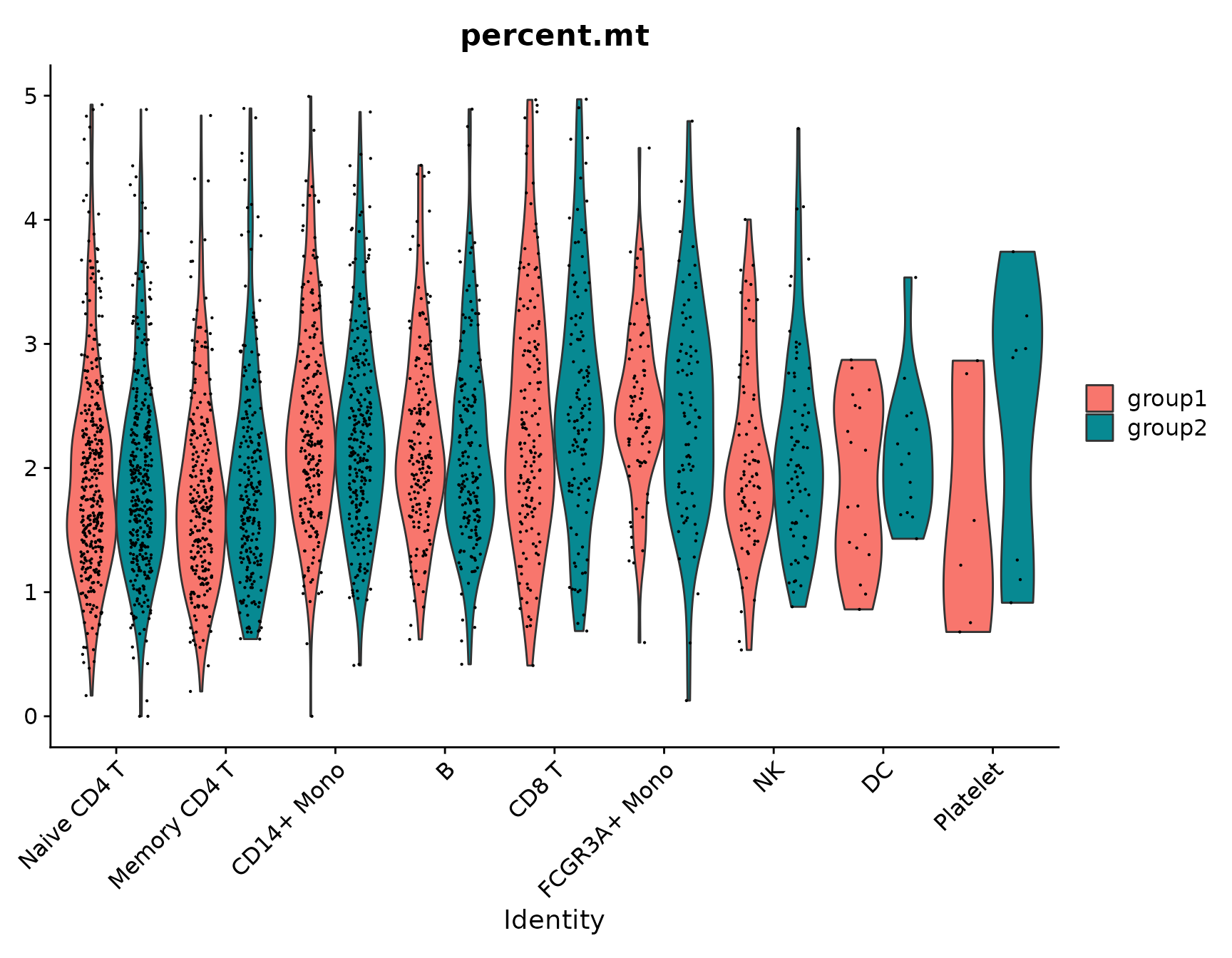
3. FeaturePlot
# Feature plot - visualize feature expression in low-dimensional space
FeaturePlot(pbmc3k.final, features = features)
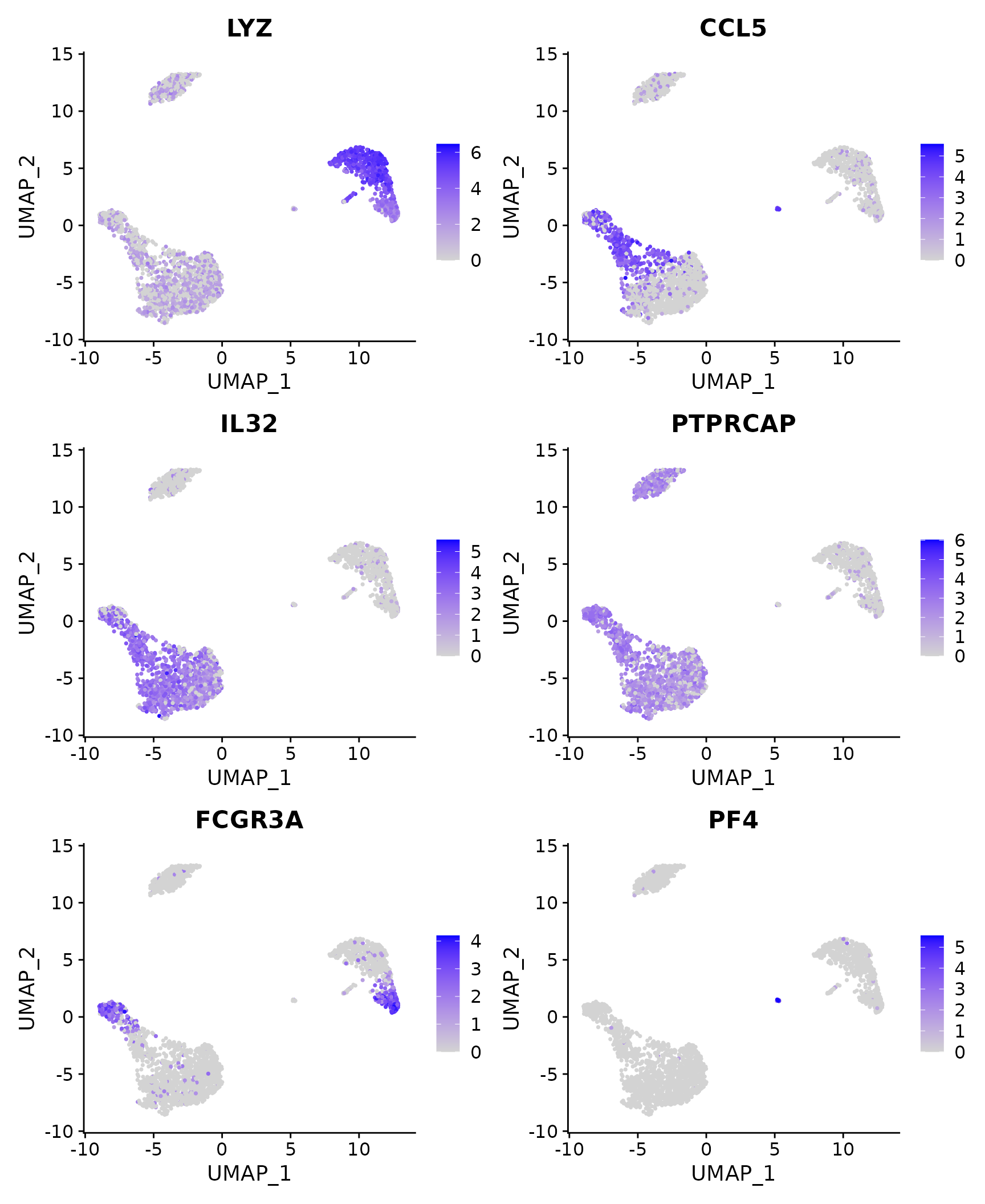
# Plot a legend to map colors to expression levels
FeaturePlot(pbmc3k.final, features = "MS4A1")
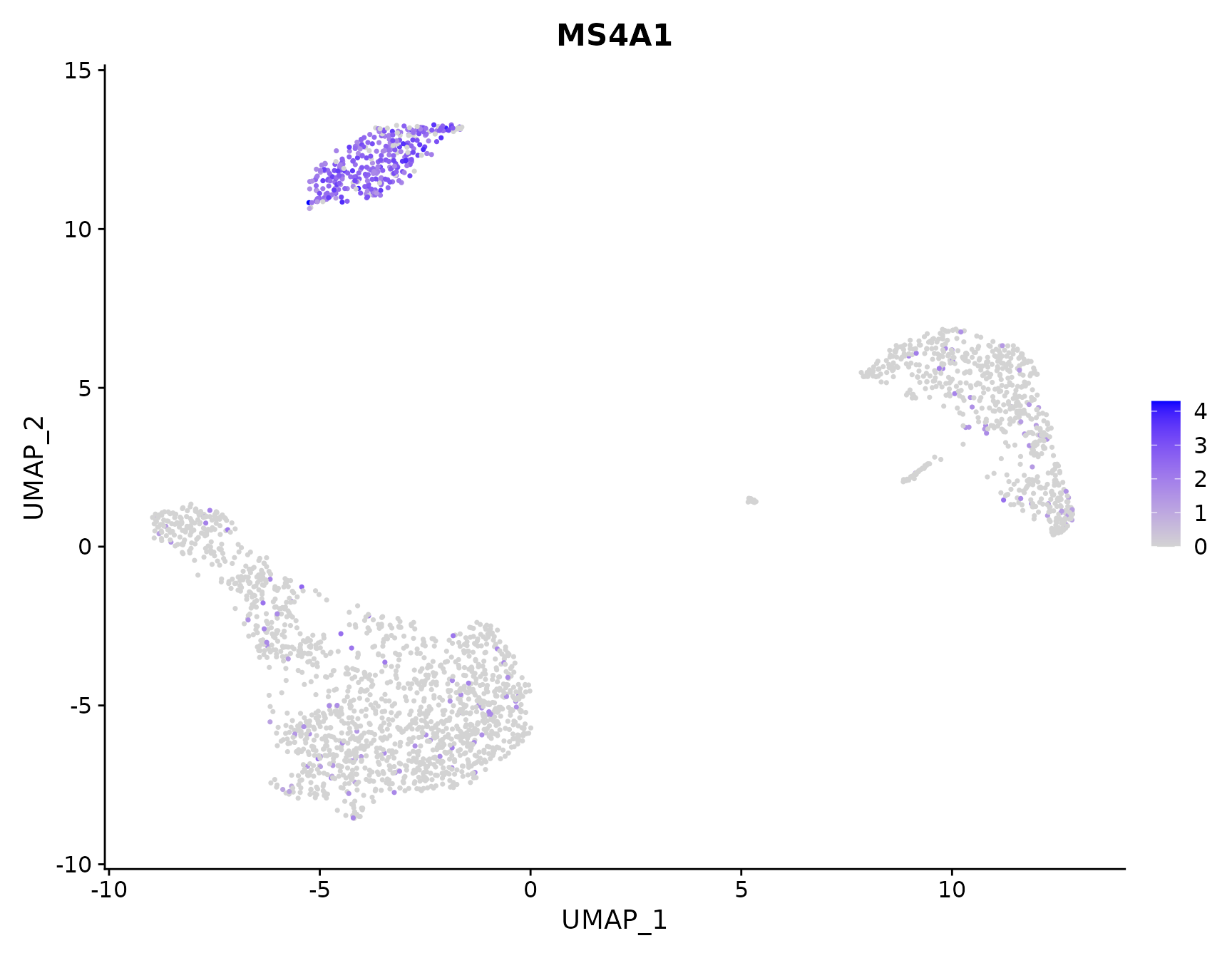
# Adjust the contrast in the plot
FeaturePlot(pbmc3k.final, features = "MS4A1", min.cutoff = 1, max.cutoff = 3)
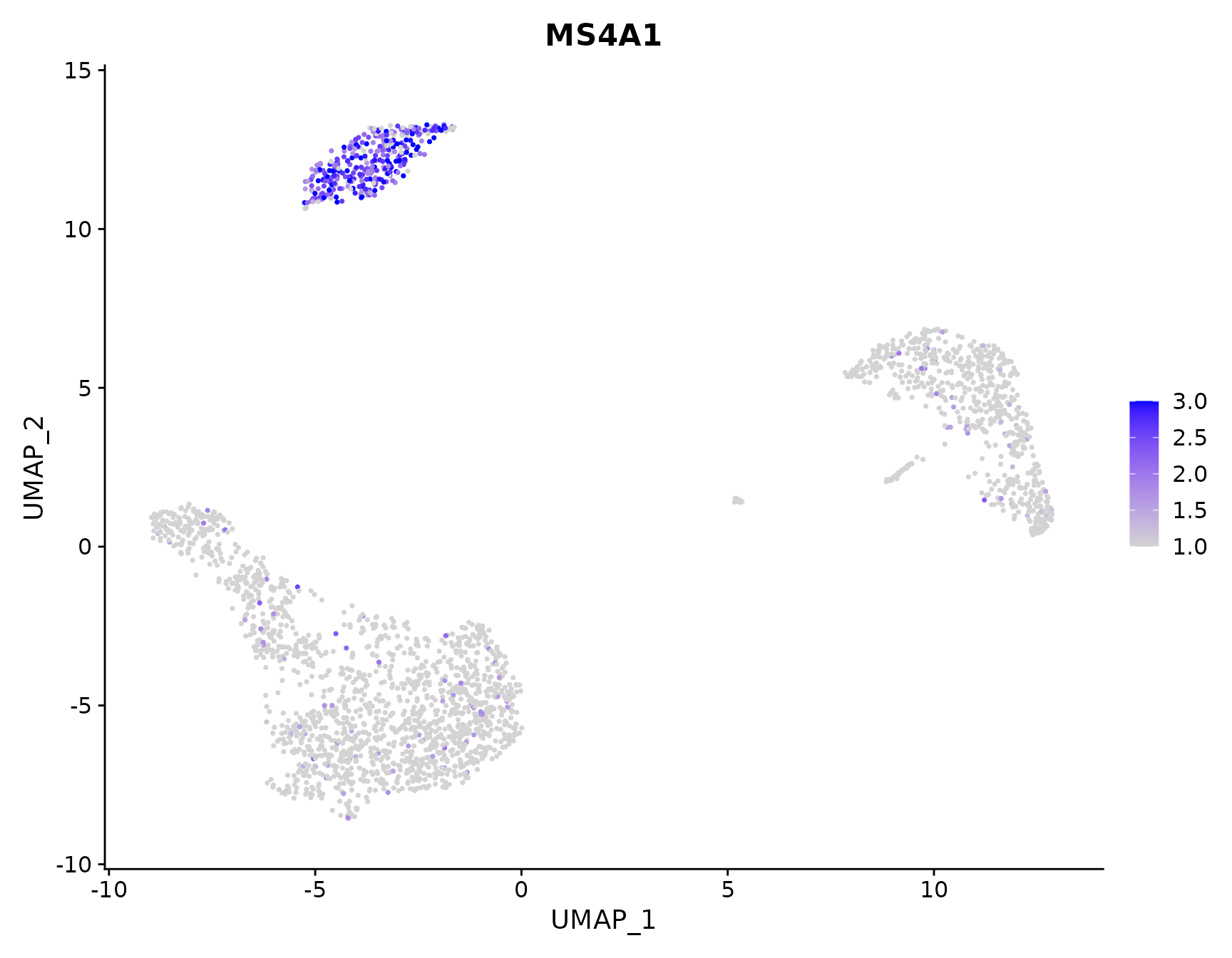
# Calculate feature-specific contrast levels based on quantiles of non-zero expression.
# Particularly useful when plotting multiple markers
FeaturePlot(pbmc3k.final, features = c("MS4A1", "PTPRCAP"), min.cutoff = "q10", max.cutoff = "q90")
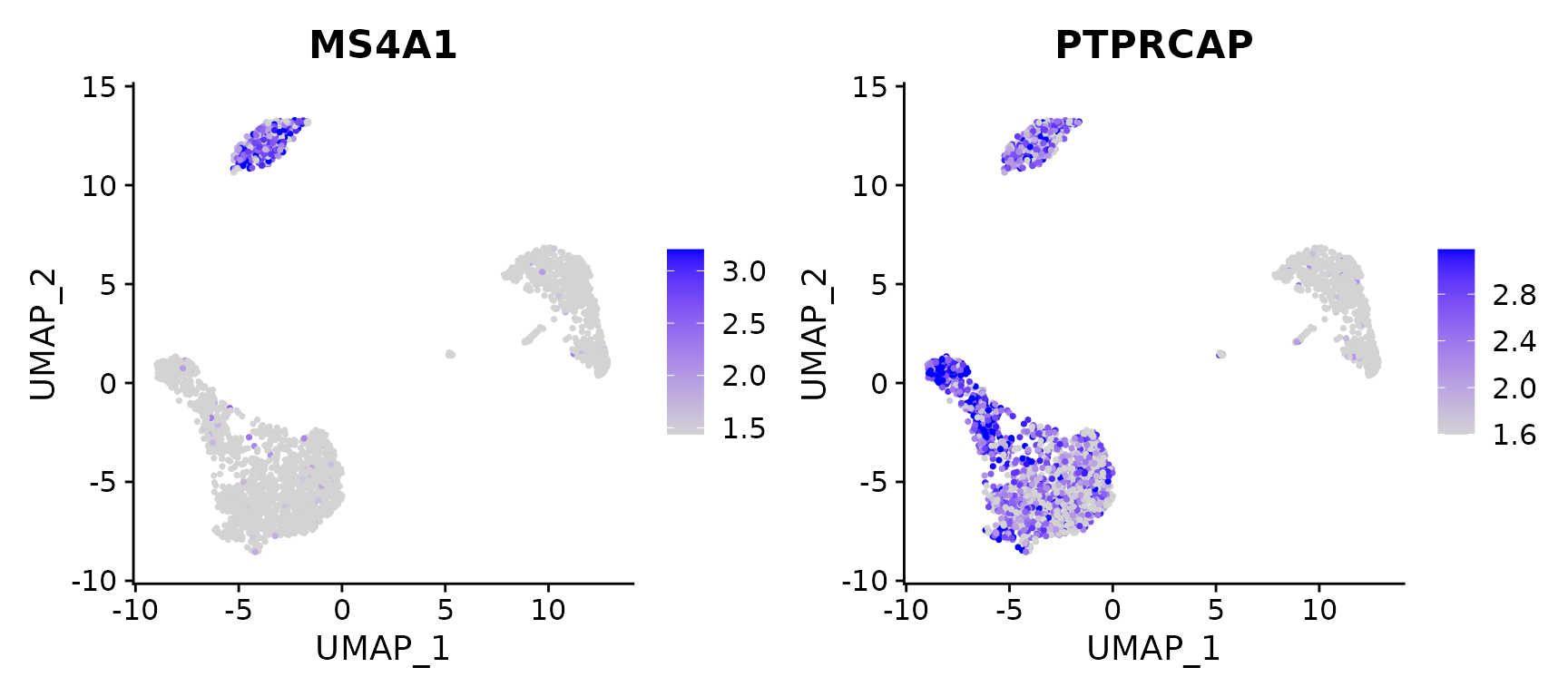
# Visualize co-expression of two features simultaneously
FeaturePlot(pbmc3k.final, features = c("MS4A1", "CD79A"), blend = TRUE)
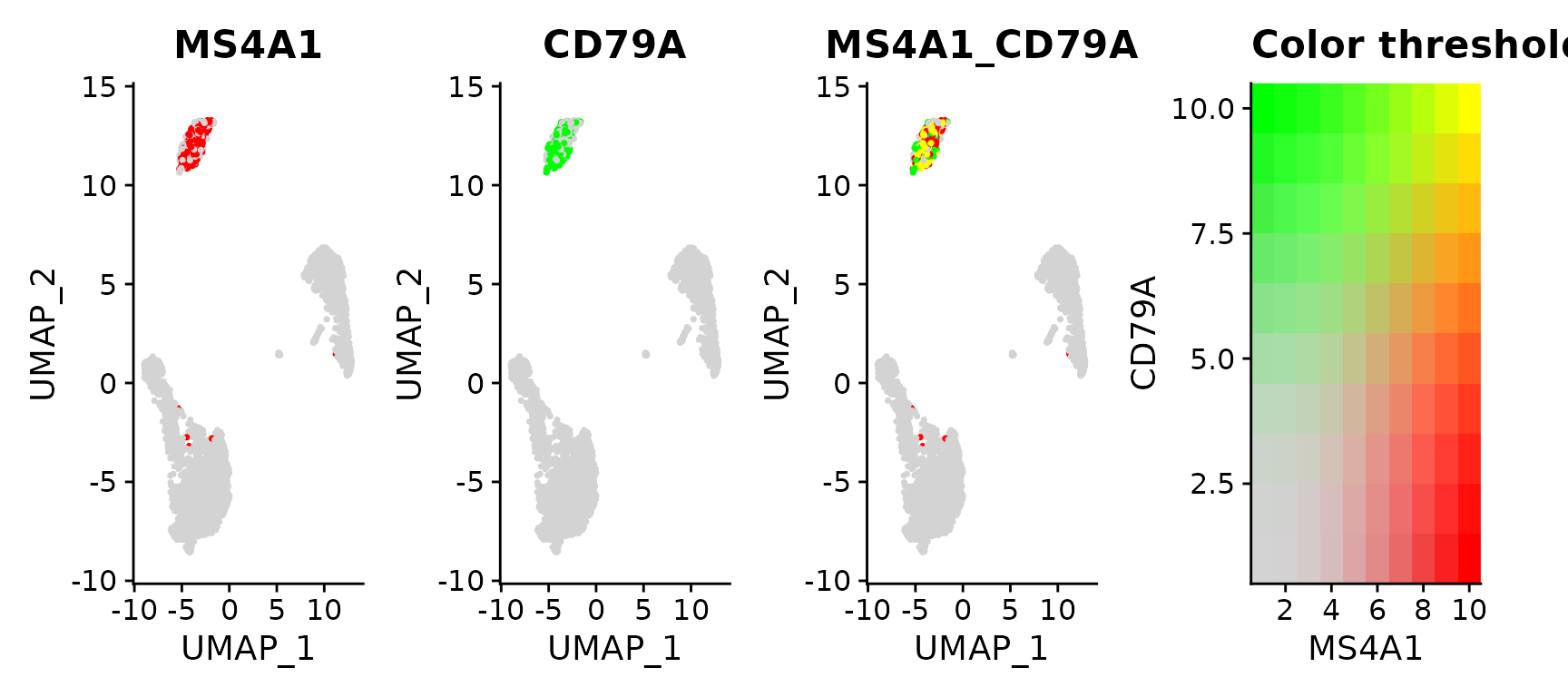
# Split visualization to view expression by groups (replaces FeatureHeatmap)
FeaturePlot(pbmc3k.final, features = c("MS4A1", "CD79A"), split.by = "groups")
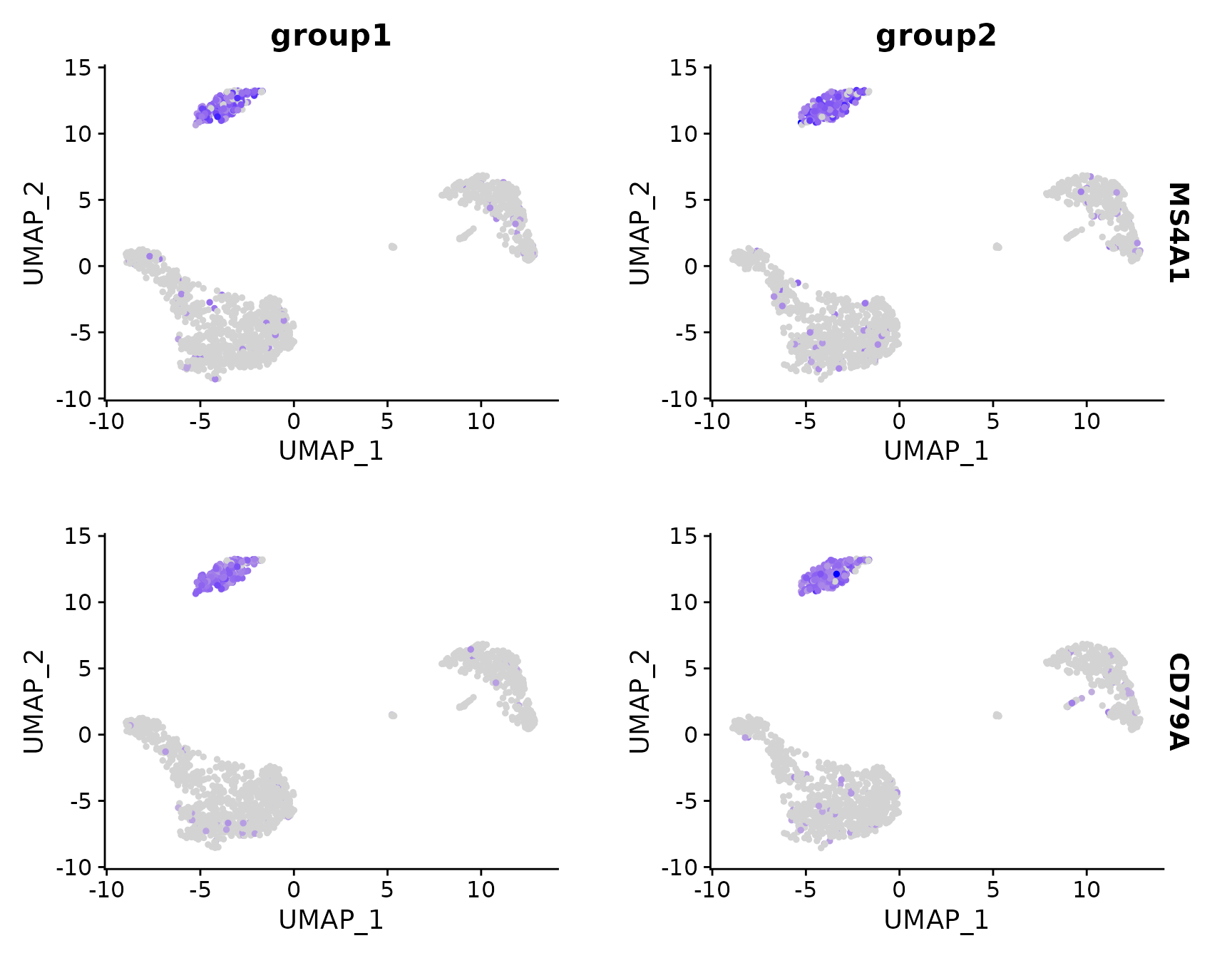
4. DotPlot
# Dot plots - the size of the dot corresponds to the percentage of cells expressing the
# feature in each cluster. The color represents the average expression level
DotPlot(pbmc3k.final, features = features) + RotatedAxis()
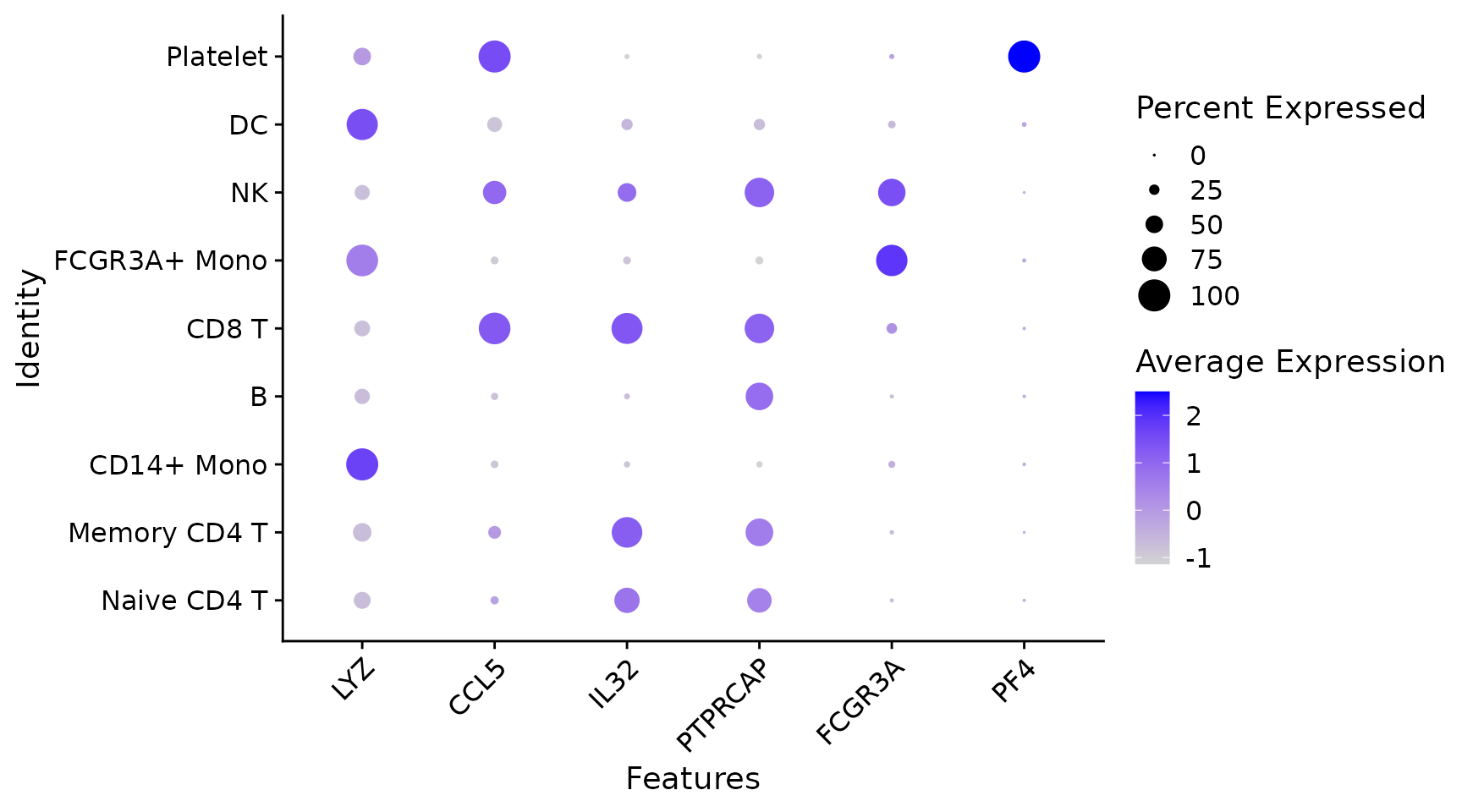
# SplitDotPlotGG has been replaced with the `split.by` parameter for DotPlot
DotPlot(pbmc3k.final, features = features, split.by = "groups") + RotatedAxis()
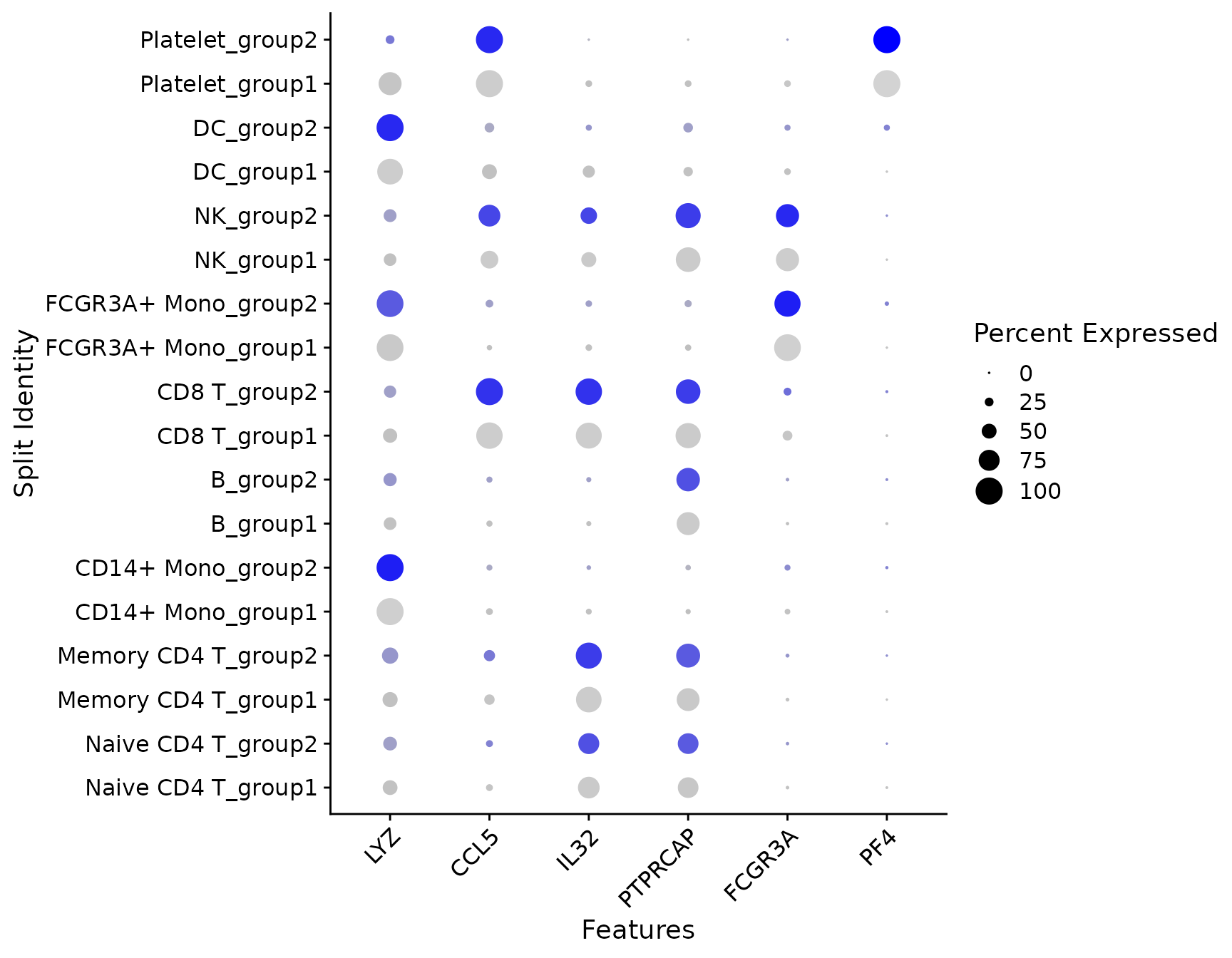
5. DoHeatmap
## Single cell heatmap of feature expression
DoHeatmap(subset(pbmc3k.final, downsample = 100), features = features, size = 3)
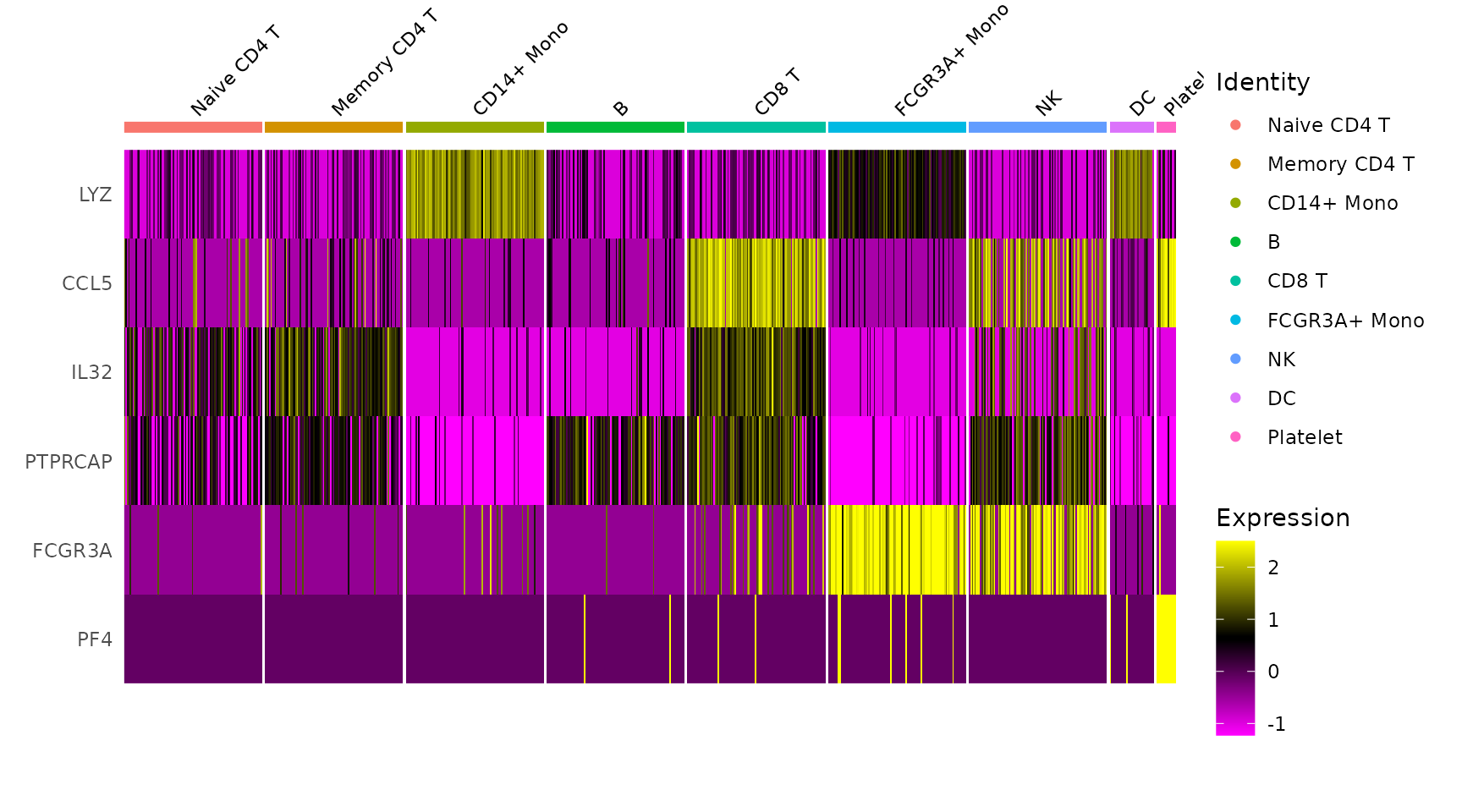
新绘图函数
DimPlot
# DimPlot replaces TSNEPlot, PCAPlot, etc. In addition, it will plot either 'umap', 'tsne', or
# 'pca' by default, in that order
DimPlot(pbmc3k.final)
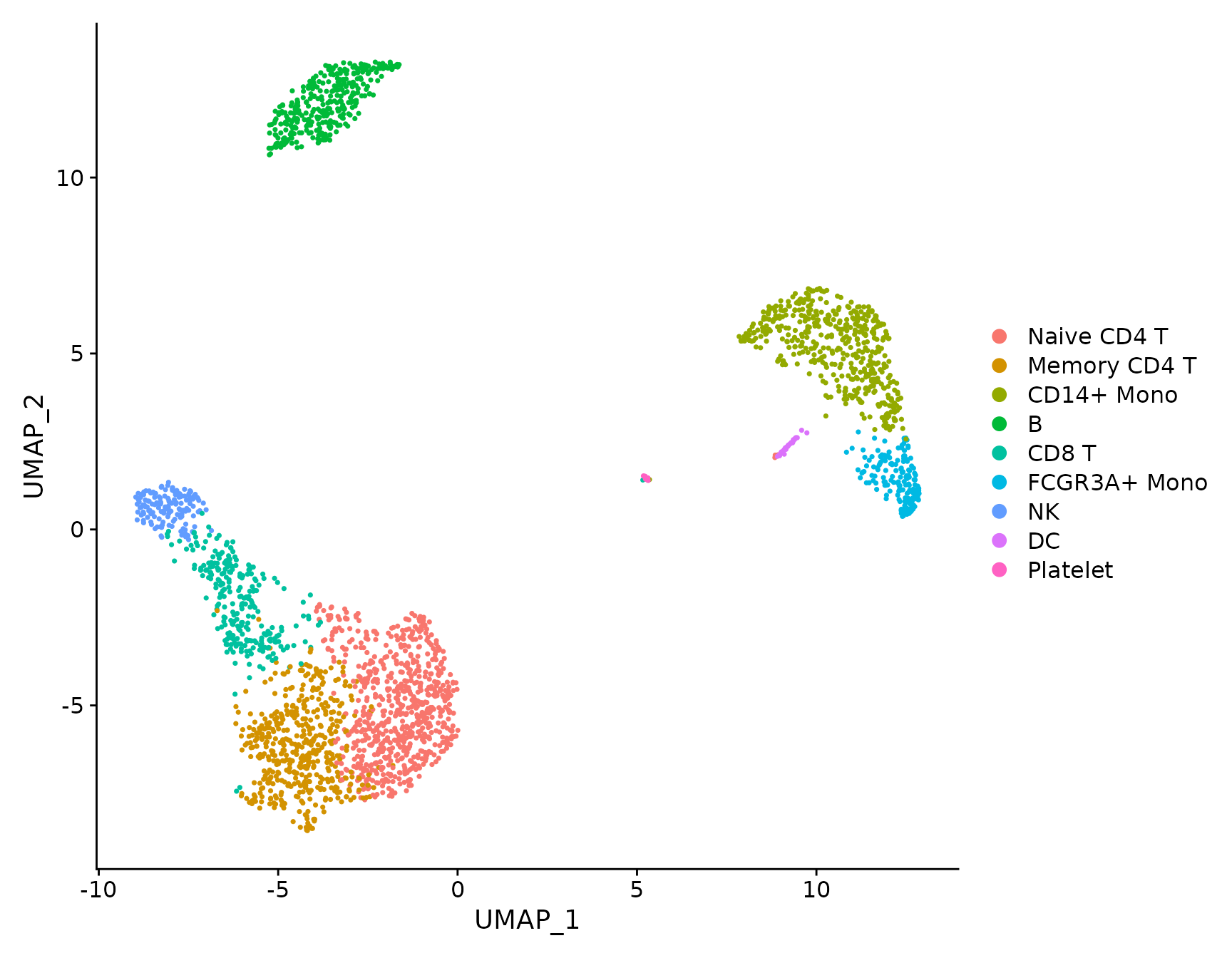
pbmc3k.final.no.umap <- pbmc3k.final
pbmc3k.final.no.umap[["umap"]] <- NULL
DimPlot(pbmc3k.final.no.umap) + RotatedAxis()
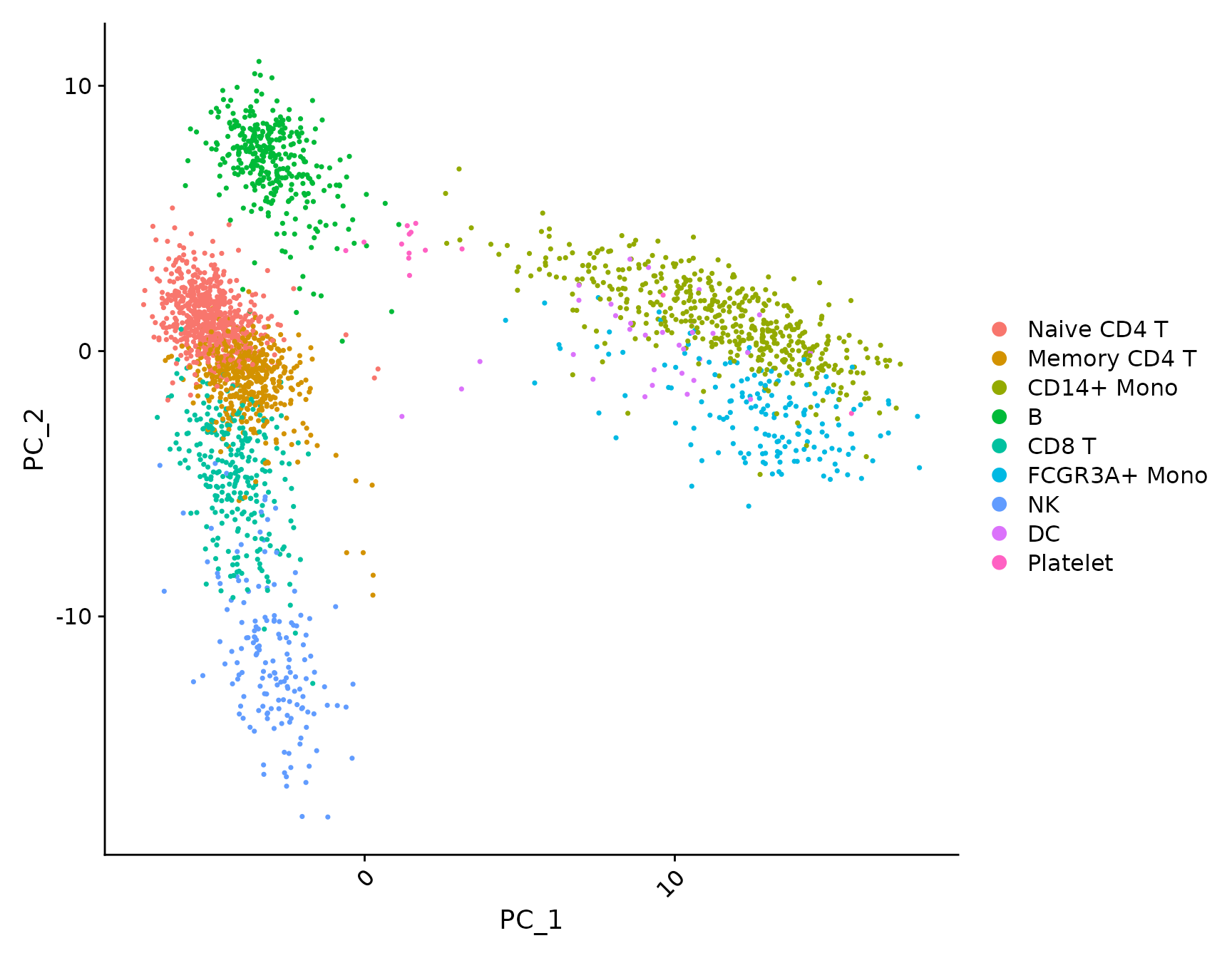
DoHeatmap
# DoHeatmap now shows a grouping bar, splitting the heatmap into groups or clusters. This can
# be changed with the `group.by` parameter
DoHeatmap(pbmc3k.final, features = VariableFeatures(pbmc3k.final)[1:100], cells = 1:500, size = 4,
angle = 90) + NoLegend()
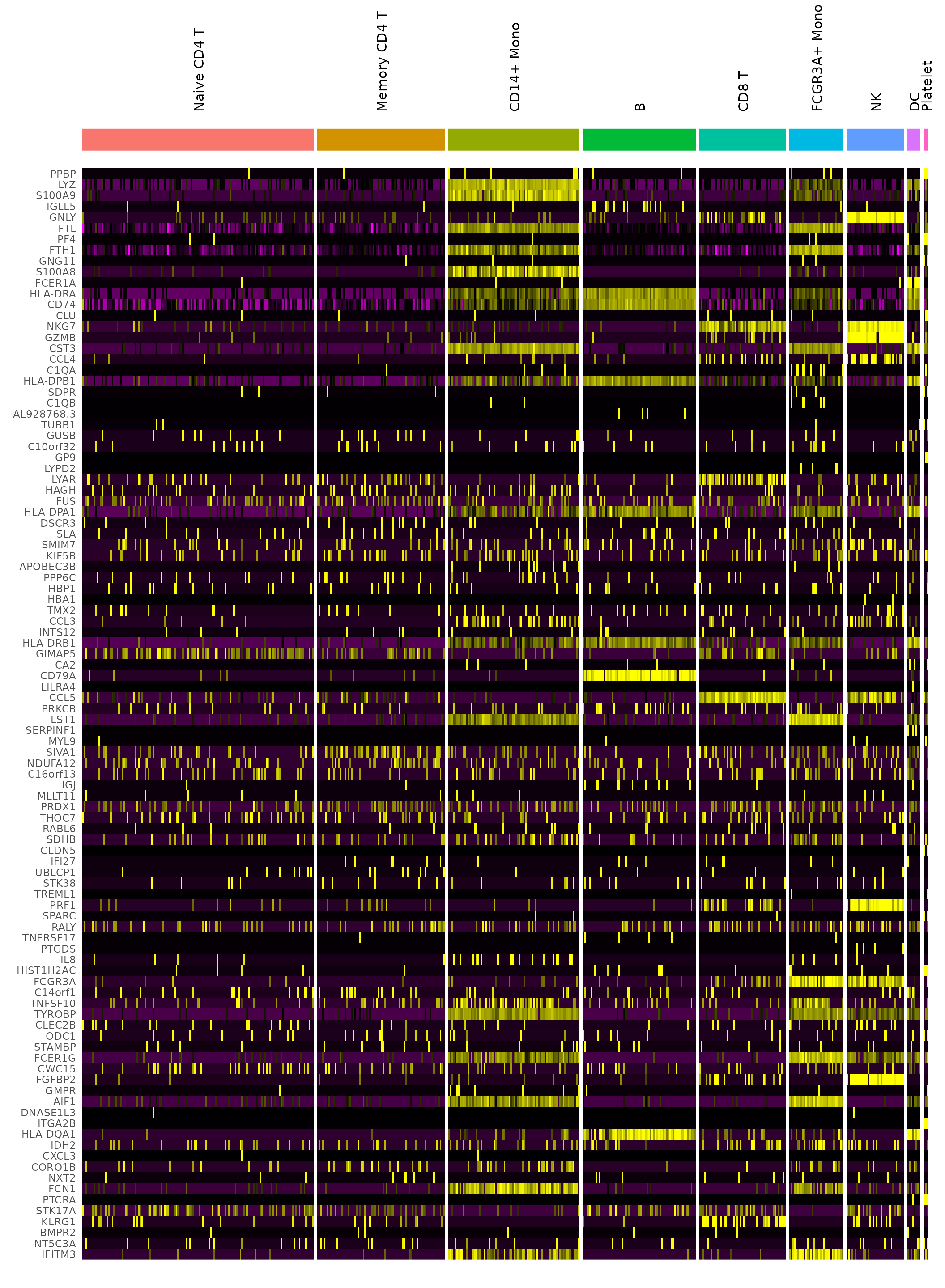
将主题应用于绘图
使用 Seurat,所有绘图函数默认返回基于 ggplot2 的绘图,允许人们像任何其他基于 ggplot2 的绘图一样轻松捕获和操作绘图。
baseplot <- DimPlot(pbmc3k.final, reduction = "umap")
# Add custom labels and titles
baseplot + labs(title = "Clustering of 2,700 PBMCs")
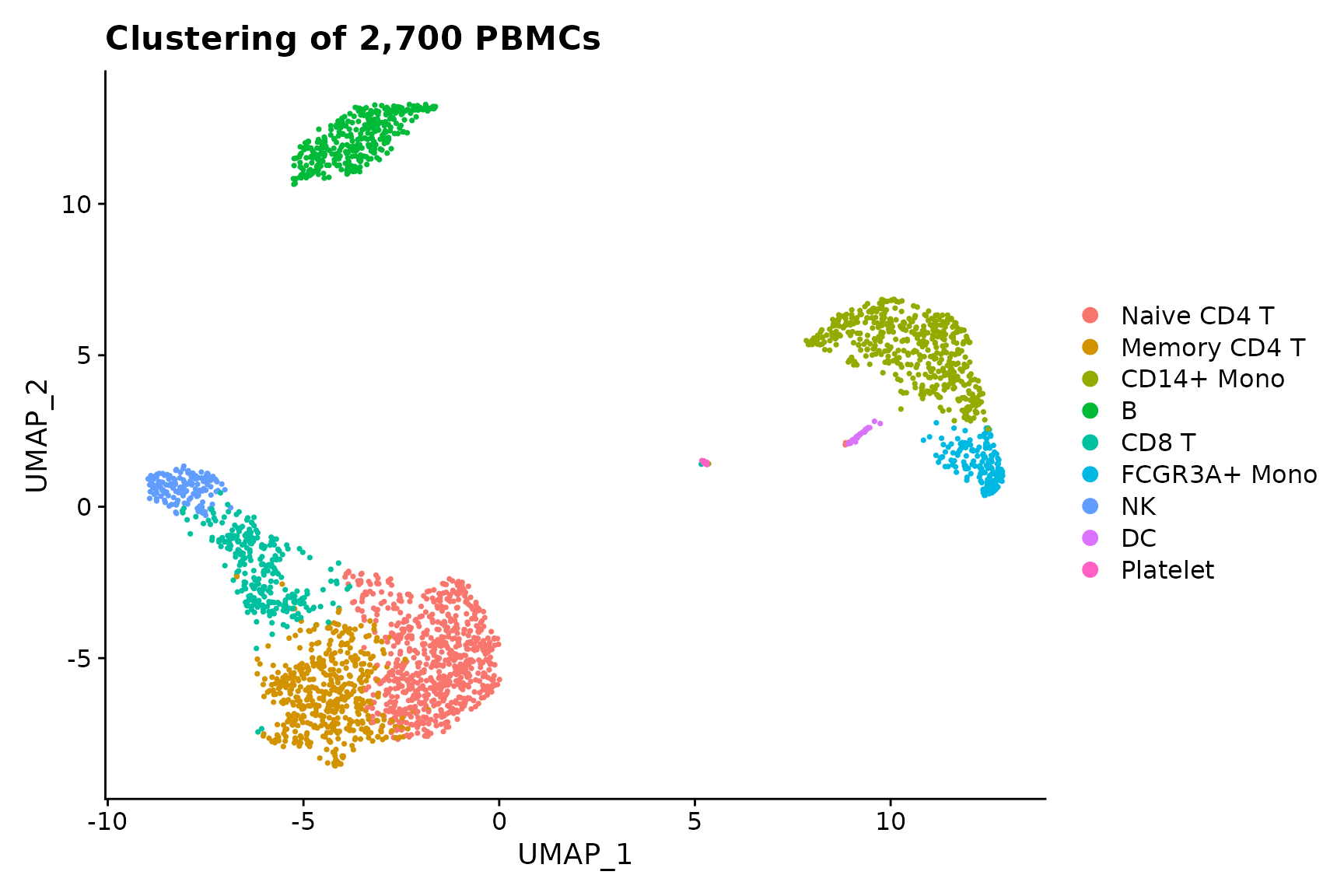
# Use community-created themes, overwriting the default Seurat-applied theme Install ggmin
# with remotes::install_github('sjessa/ggmin')
baseplot + ggmin::theme_powerpoint()
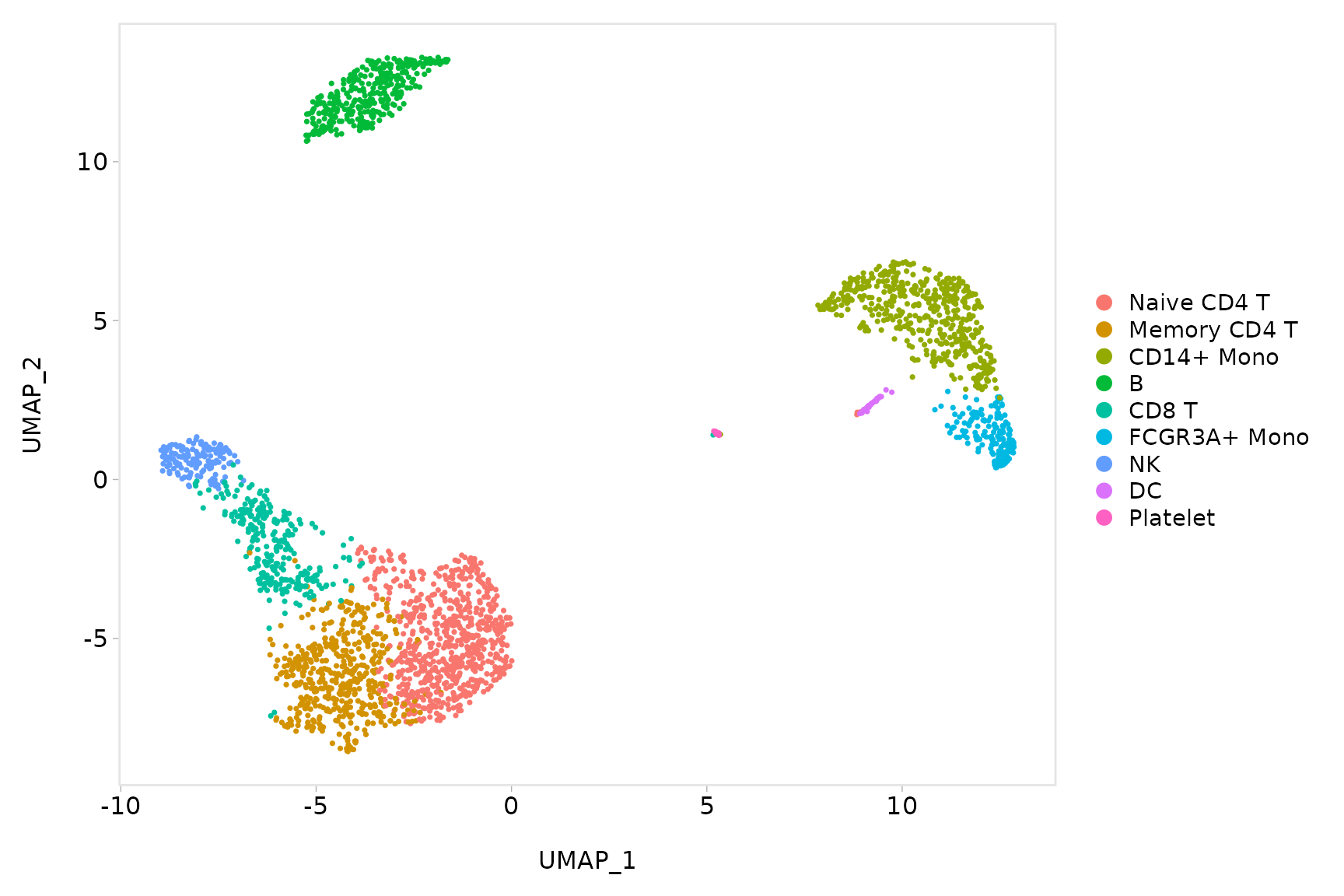
# Seurat also provides several built-in themes, such as DarkTheme; for more details see
# ?SeuratTheme
baseplot + DarkTheme()
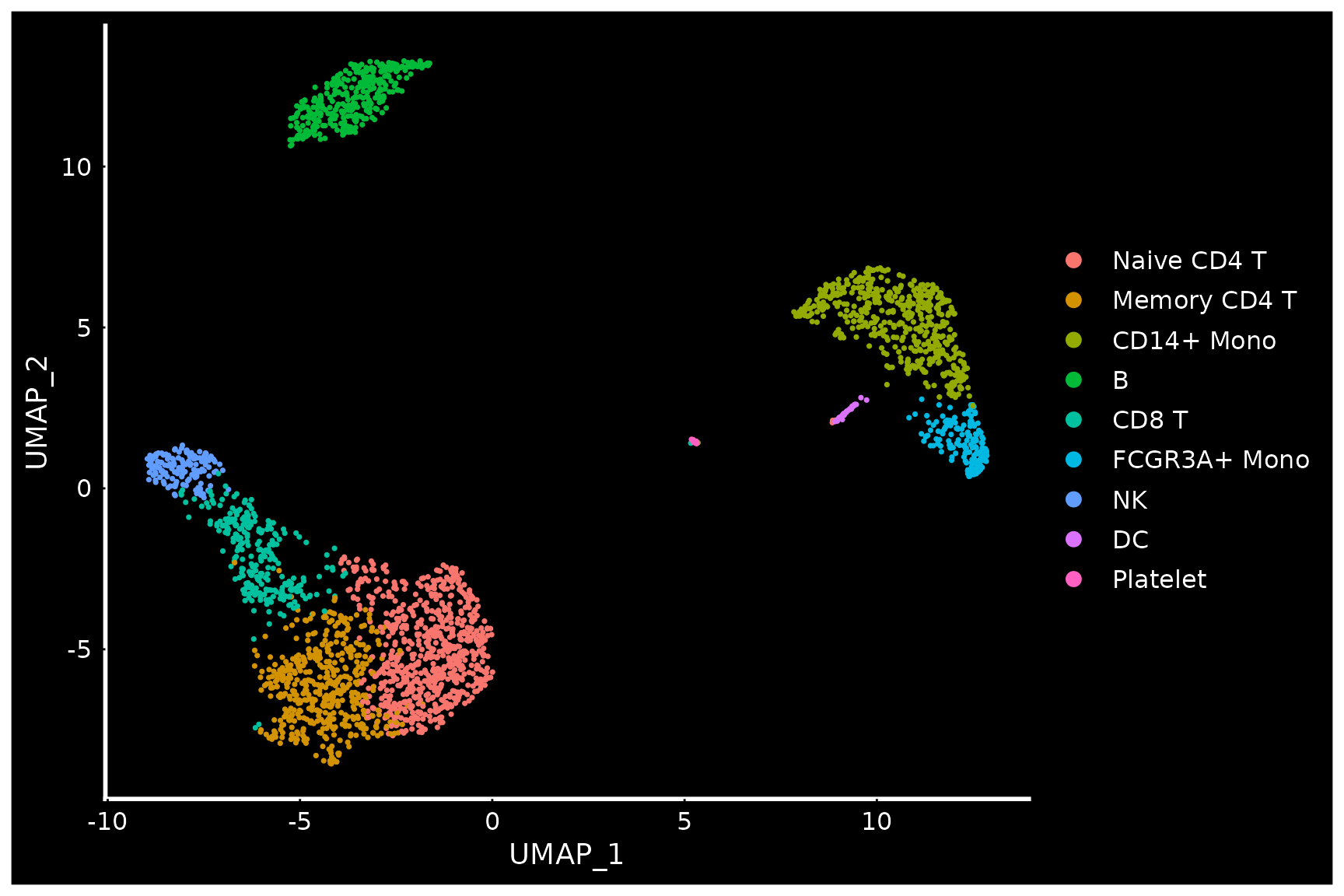
# Chain themes together
baseplot + FontSize(x.title = 20, y.title = 20) + NoLegend()
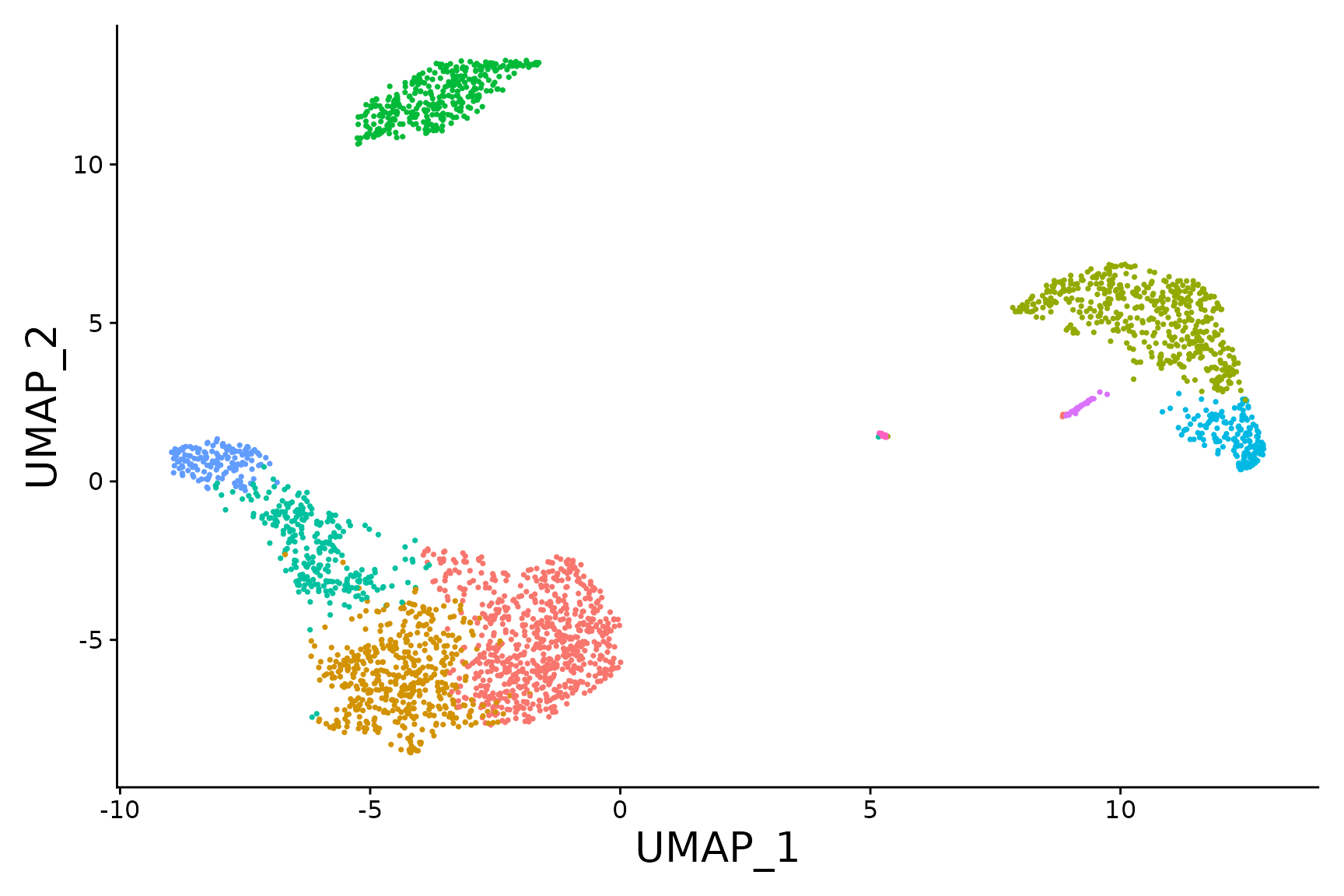
交互式绘图功能
Seurat 利用 R 的绘图库来创建交互式绘图。此交互式绘图功能适用于任何基于 ggplot2 的散点图(需要 geom_point 图层)。使用时,只需制作一个基于 ggplot2 的散点图(例如 DimPlot() 或 FeaturePlot())并将结果图传递给 HoverLocator()
# Include additional data to display alongside cell names by passing in a data frame of
# information. Works well when using FetchData
plot <- FeaturePlot(pbmc3k.final, features = "MS4A1")
HoverLocator(plot = plot, information = FetchData(pbmc3k.final, vars = c("ident", "PC_1", "nFeature_RNA")))
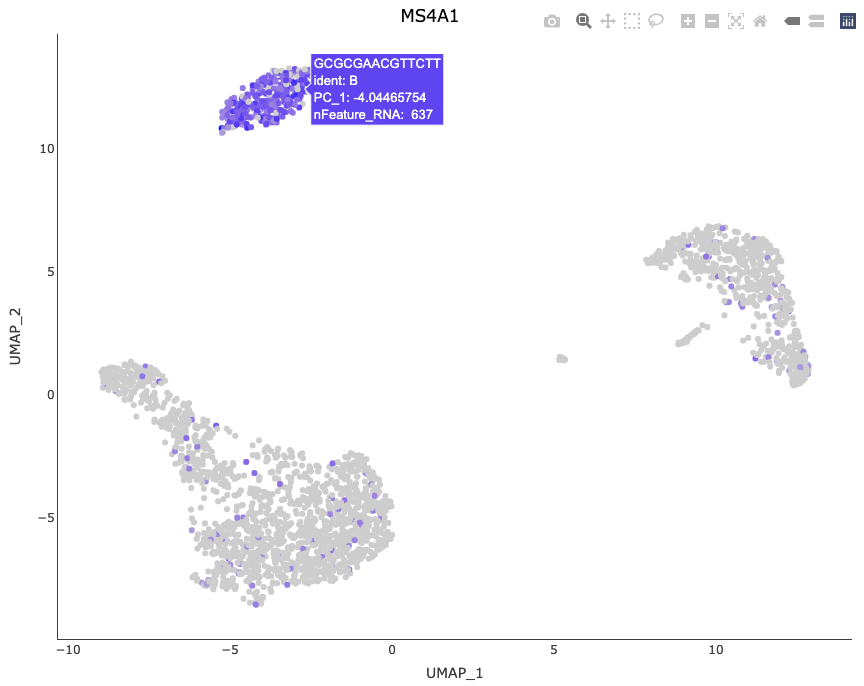
Seurat 提供的另一个交互功能是能够手动选择细胞以进行进一步研究。我们发现这对于小簇特别有用,这些小簇并不总是使用无偏聚类来分离,但看起来却截然不同。现在,您可以通过创建基于 ggplot2 的散点图(例如使用 DimPlot() 或 FeaturePlot(),并将返回的图传递给 CellSelector() 来选择这些单元格。CellSelector() 将返回一个包含所选点名称的向量,这样您就可以将它们设置为新的身份类并执行微分表达式。
例如,假设 DC 在聚类中与单核细胞合并,但我们想根据它们在 tSNE 图中的位置来了解它们的独特之处。
pbmc3k.final <- RenameIdents(pbmc3k.final, DC = "CD14+ Mono")
plot <- DimPlot(pbmc3k.final, reduction = "umap")
select.cells <- CellSelector(plot = plot)
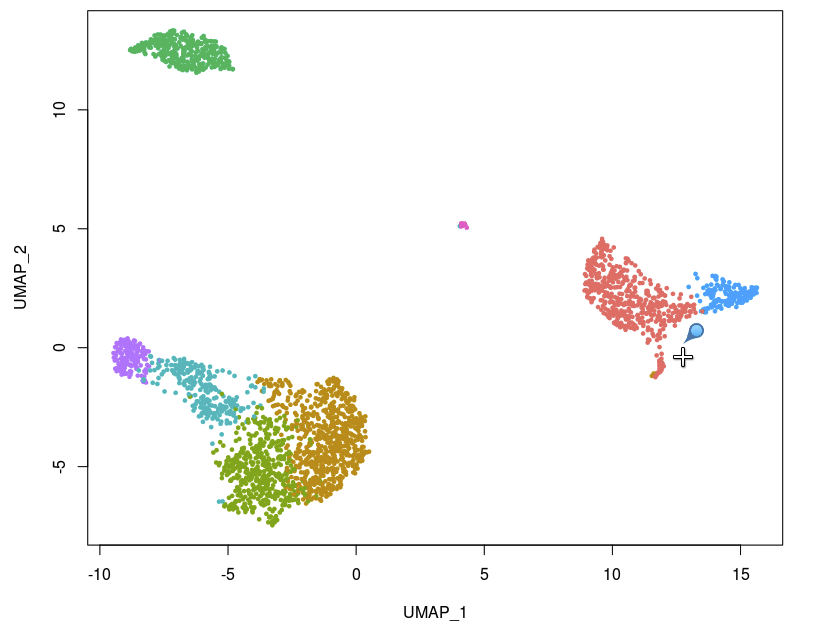
绘图配件
除了为绘图添加交互功能的新函数之外,Seurat 还提供了用于操作和组合绘图的新辅助功能。
# LabelClusters and LabelPoints will label clusters (a coloring variable) or individual points
# on a ggplot2-based scatter plot
plot <- DimPlot(pbmc3k.final, reduction = "pca") + NoLegend()
LabelClusters(plot = plot, id = "ident")
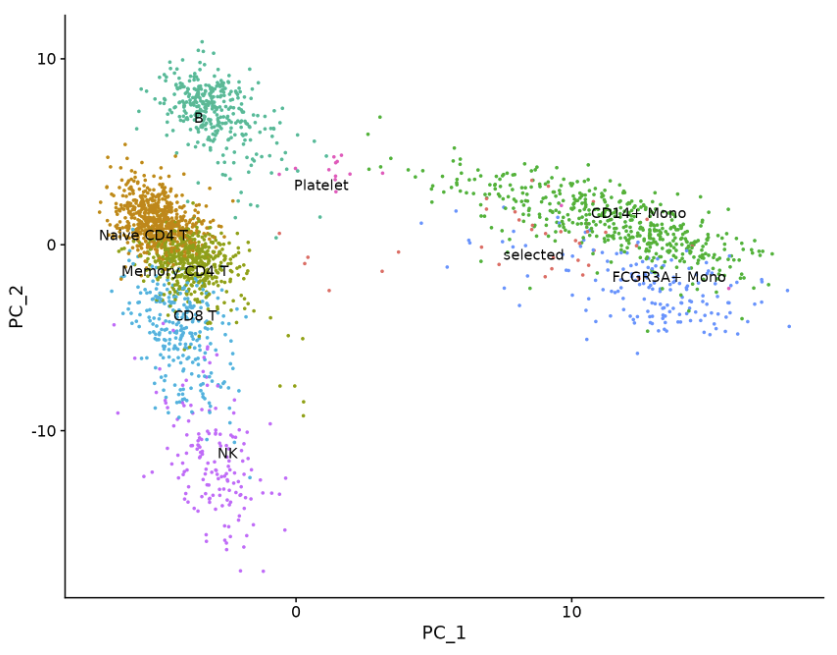
# Both functions support `repel`, which will intelligently stagger labels and draw connecting
# lines from the labels to the points or clusters
LabelPoints(plot = plot, points = TopCells(object = pbmc3k.final[["pca"]]), repel = TRUE)
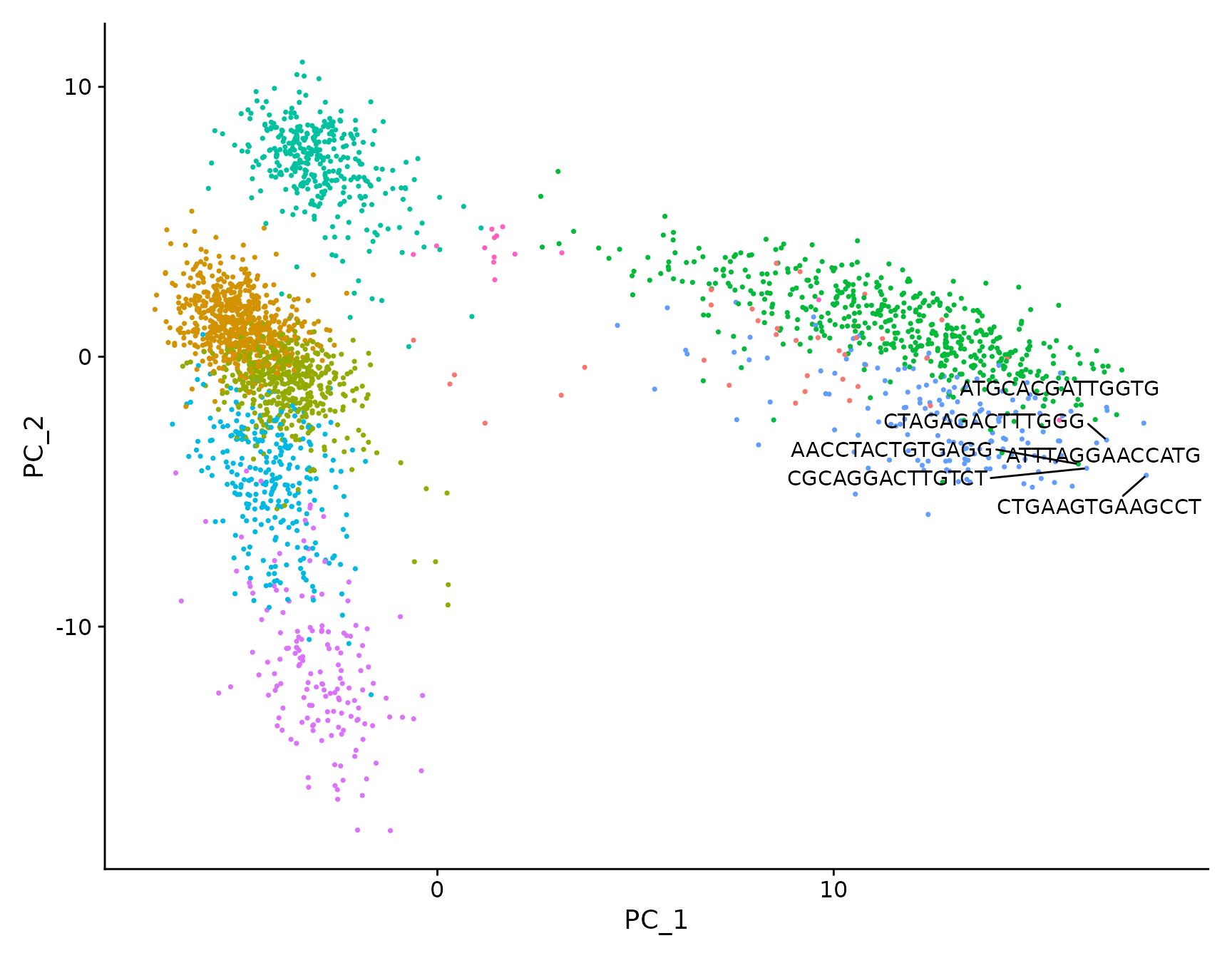
绘制多个图之前是通过CombinePlot() 函数实现的。我们不赞成使用此功能,转而使用拼凑系统。下面是一个简短的演示,但请参阅此处的 patchwork[3] 包网站以获取更多详细信息和示例。
plot1 <- DimPlot(pbmc3k.final)
# Create scatter plot with the Pearson correlation value as the title
plot2 <- FeatureScatter(pbmc3k.final, feature1 = "LYZ", feature2 = "CCL5")
# Combine two plots
plot1 + plot2
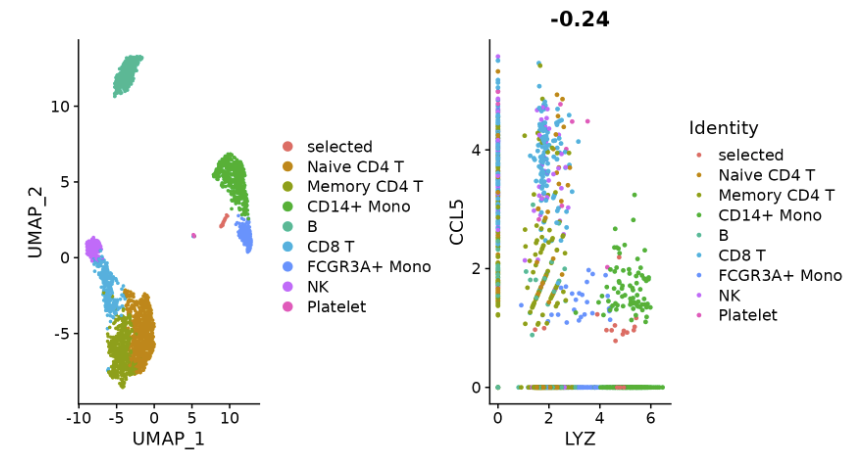
# Remove the legend from all plots
(plot1 + plot2) & NoLegend()
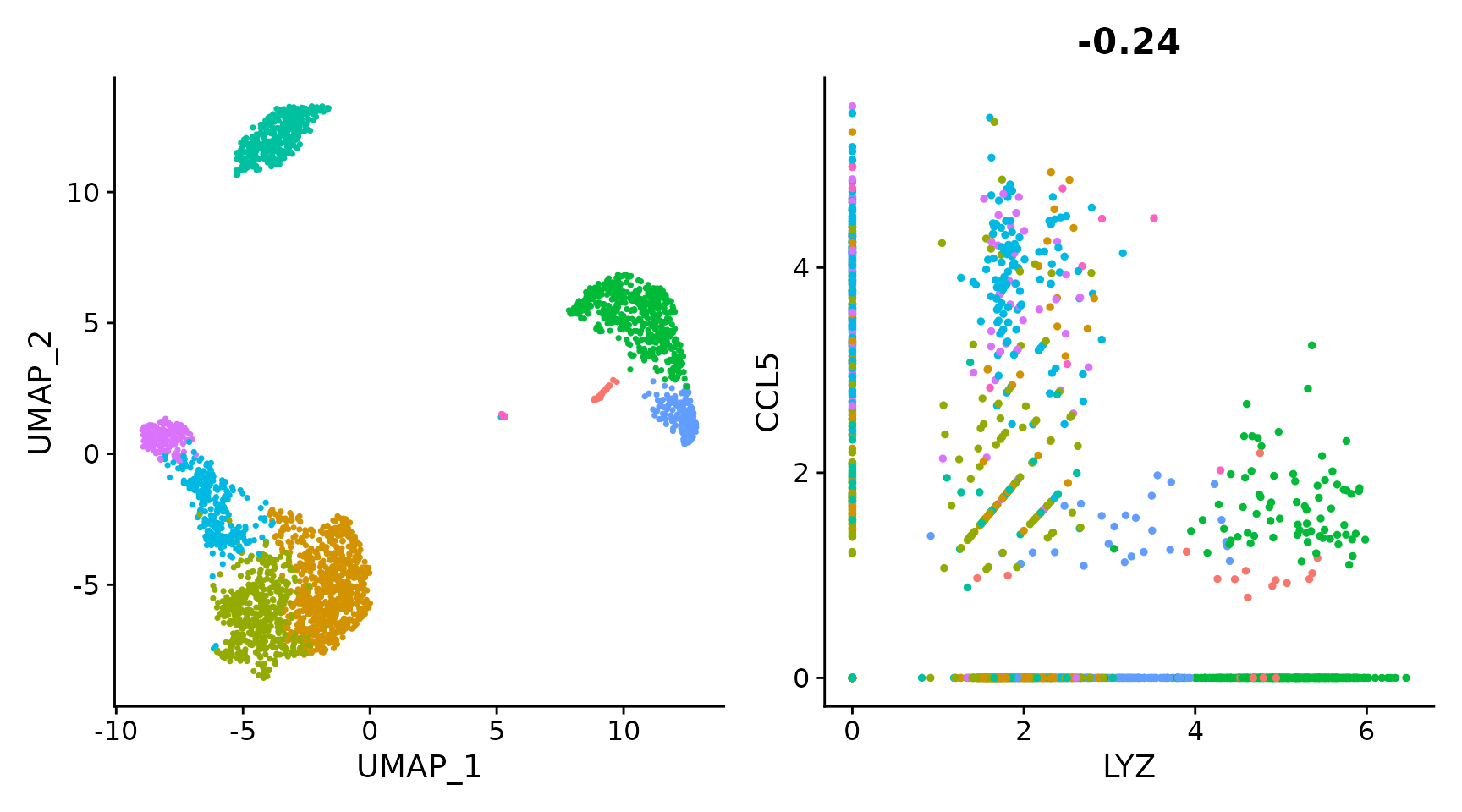
Source: https://satijalab.org/seurat/articles/visualization_vignette
[2]Data: https://github.com/satijalab/seurat-data
[3]patchwork: https://patchwork.data-imaginist.com/
本文由 mdnice 多平台发布
相关文章:
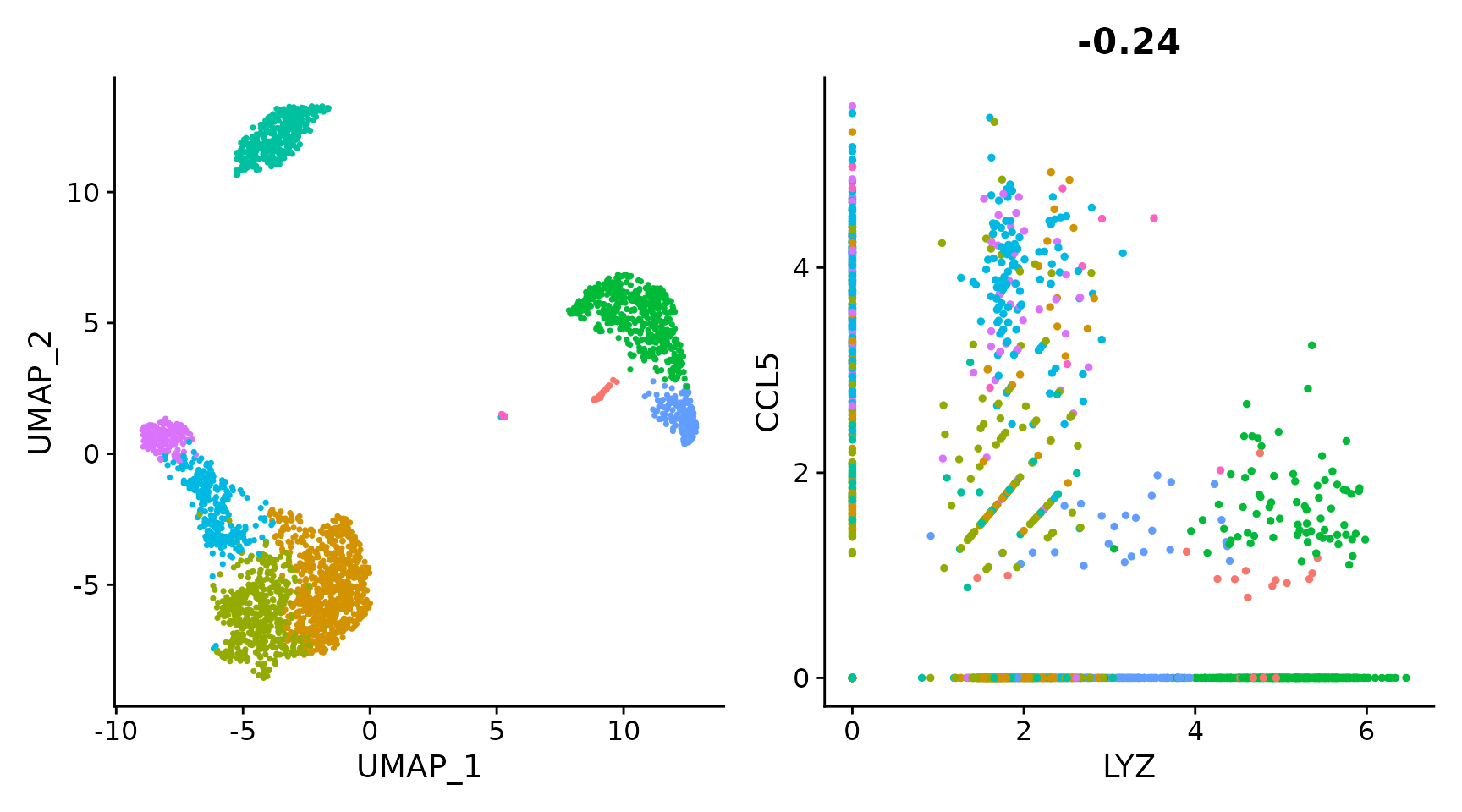
Seurat 中的数据可视化方法
本文[1]将使用从 2,700 PBMC 教程计算的 Seurat 对象来演示 Seurat 中的可视化技术。您可以从 SeuratData[2] 下载此数据集。 SeuratData::InstallData("pbmc3k")library(Seurat)library(SeuratData)library(ggplot2)library(patchwork)pbmc3k.final <- LoadData(…...
ImportError: cannot import name ‘InterpolationMode‘
InterpolationMode 在图像处理库中通常用于指定图像缩放时的插值方法。插值是一种数学方法,在图像大小变化时用于估算新像素位置的像素值。不同的插值方法会影响缩放后图像的质量和外观。 在你提供的 image_transform 函数中,InterpolationMode.BICUBIC…...
HSRP和VRRP
VRRP(Virtual Router Redundancy Protocol,虚拟路由器冗余协议) 是一种网络层的容错协议,主要用于在多台路由器之间提供默认网关冗余。在IP网络中,当一个子网有多个路由器时,VRRP可以确保在主用路由器失效…...
C及C++每日练习(1)
一.选择: 1.以下for循环的执行次数是() for(int x 0, y 0; (y 123) && (x < 4); x); A.是无限循环 B.循环次数不定 C.4次 D.3次 对于循环,其组成部分可以四个部分: for(初始化;循环进行条件;调整) …...
Oracle 12c dataguard查看主备库同步情况的新变化
导读 本文介绍Oracle 12c dataguard在维护方面的新变化 前提:主库备库的同步是正常的。 1、主库上查看archive Log list SYScdb1> archive log list; Database log mode Archive Mode Automatic archival Enabled Archive destination…...
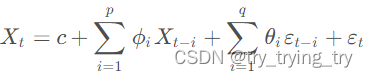
时间序列-AR MA ARIMA
一、AR模型(自回归) AR探索趋势和周期性 预测依赖于过去的观测值和模型中的参数。模型的阶数 p pp 决定了需要考虑多少个过去时间点的观测值。 求AR模型的阶数 p和参数 ϕ i \phi_i ϕi ,常常会使用统计方法如最小二乘法、信息准则(如AIC、BIC…...
Spring Boot(六十六):集成Alibaba Druid 连接池
1 Alibaba Druid介绍 在现代的Java应用中,使用一个高效可靠的数据源是至关重要的。Druid连接池作为一款强大的数据库连接池,提供了丰富的监控和管理功能,成为很多Java项目的首选。本文将详细介绍如何在Spring Boot项目中配置数据源,集成Druid连接池,以实现更高效的数据库…...
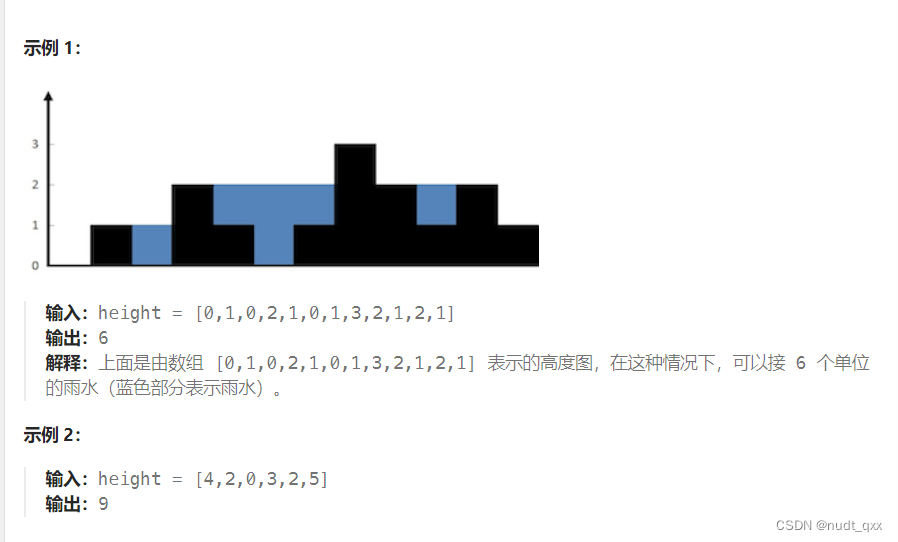
leetcode 经典题目42.接雨水
链接:https://leetcode.cn/problems/trapping-rain-water 题目描述 给定 n 个非负整数表示每个宽度为 1 的柱子的高度图,计算按此排列的柱子,下雨之后能接多少雨水。 思路分析 首先,我们需要遍历数组,对于每个元素&am…...
高防服务器的主要作用有哪些?
高防服务器是属于服务器的一种,主要是为了解决流量攻击而设计的,高防服务器能够维护服务器的稳定性和安全性,具备很高的防御能力和更加优质的网络带宽,能够提供更加可靠的服务保障,那么高防服务器主要都有哪些作用呢&a…...
【30 天 JavaScript 挑战】学习笔记
30 天 JavaScript 挑战 专为 JavaScript 初学者设计 掌握必备 JavaScript 技能 前端人,前端魂,刷完 JS 即入门! 题目地址:https://leetcode.cn/studyplan/30-days-of-javascript/ 个人学习笔记:https://github.com/kaimo313/…...
生成 Linux/ubuntu/Debian 上已安装软件包的列表
你可以在终端中使用以下命令生成已安装软件包的列表: 列出所有已安装的软件包: dpkg --get-selections要将列表保存到文件中: dpkg -l > installed_packages_detailed.txt这将在当前目录中创建一个名为“installed_packages_detailed.txt”…...
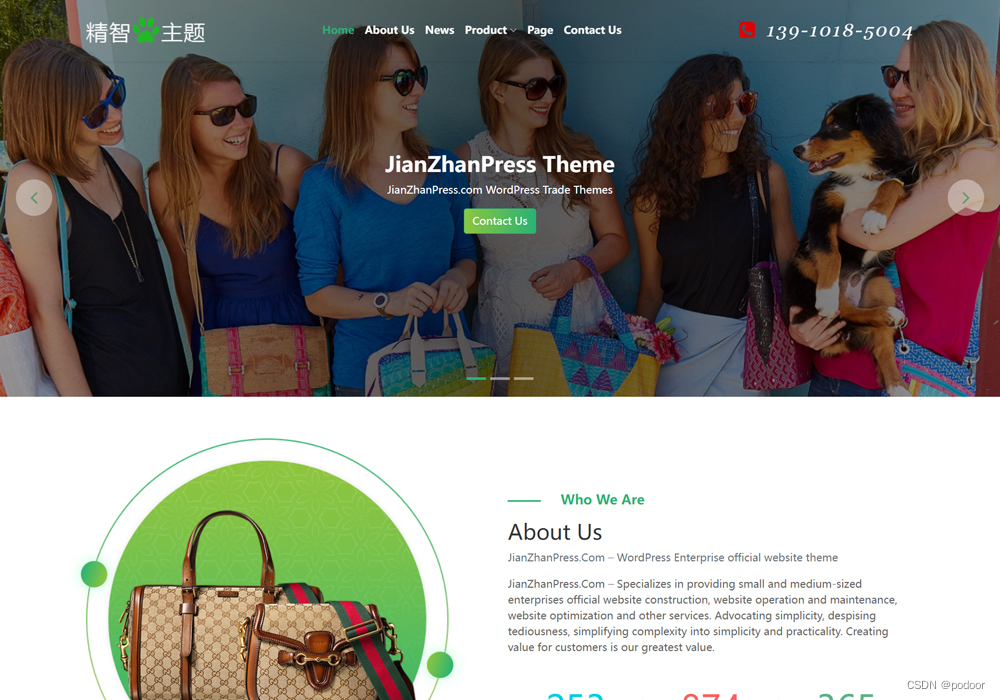
精品中国货出海wordpress外贸独立站建站模板
旗袍唐装wordpress外贸网站模板 旗袍、唐装、华服wordpress外贸网站模板,适合做衣服生意的外贸公司官网使用。 https://www.jianzhanpress.com/?p3695 劳动防护wordpress外贸独立站模板 劳动防护wordpress外贸独立站模板,劳动保护、劳动防护用品外贸…...
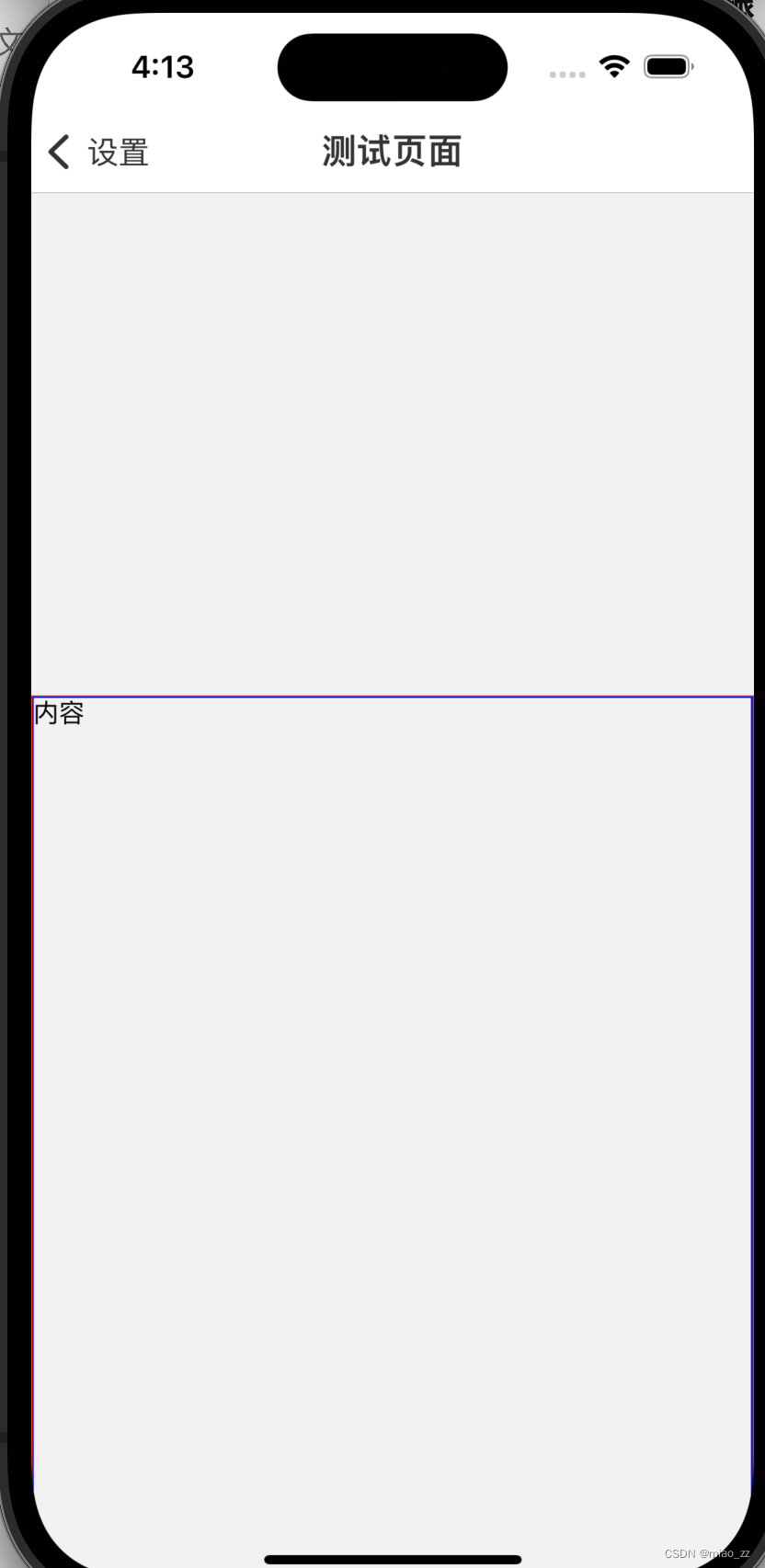
使用Animated.View实现全屏页面可以向下拖动,松开手指页面返回原处的效果
使用Animated.View实现全屏页面可以向下拖动,松开手指页面返回原处的效果 效果示例图代码示例 效果示例图 代码示例 import React, {useRef, useState} from react; import {View,Text,Animated,Easing,PanResponder,StyleSheet, } from react-native;const TestDragCard () …...
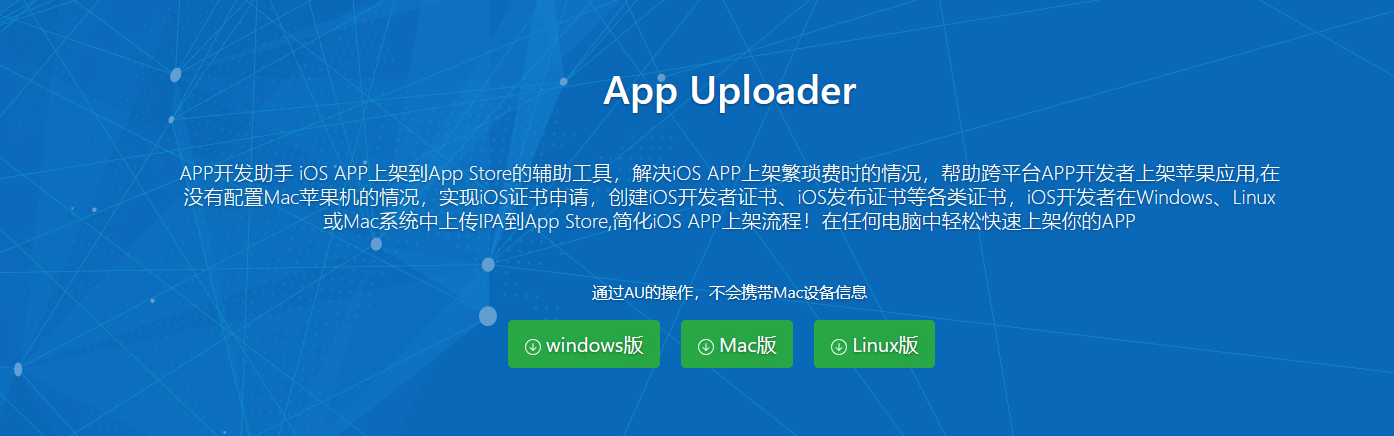
【教程】uni-app iOS打包解决profile文件与私钥证书不匹配问题
摘要 当在uni-app中进行iOS打包时,有时会遇到profile文件与私钥证书不匹配的问题。本文将介绍如何解决这一问题,以及相关的技术细节和操作步骤。 引言 在uni-app开发过程中,iOS打包是一个常见的操作。然而,有时会出现profile文…...
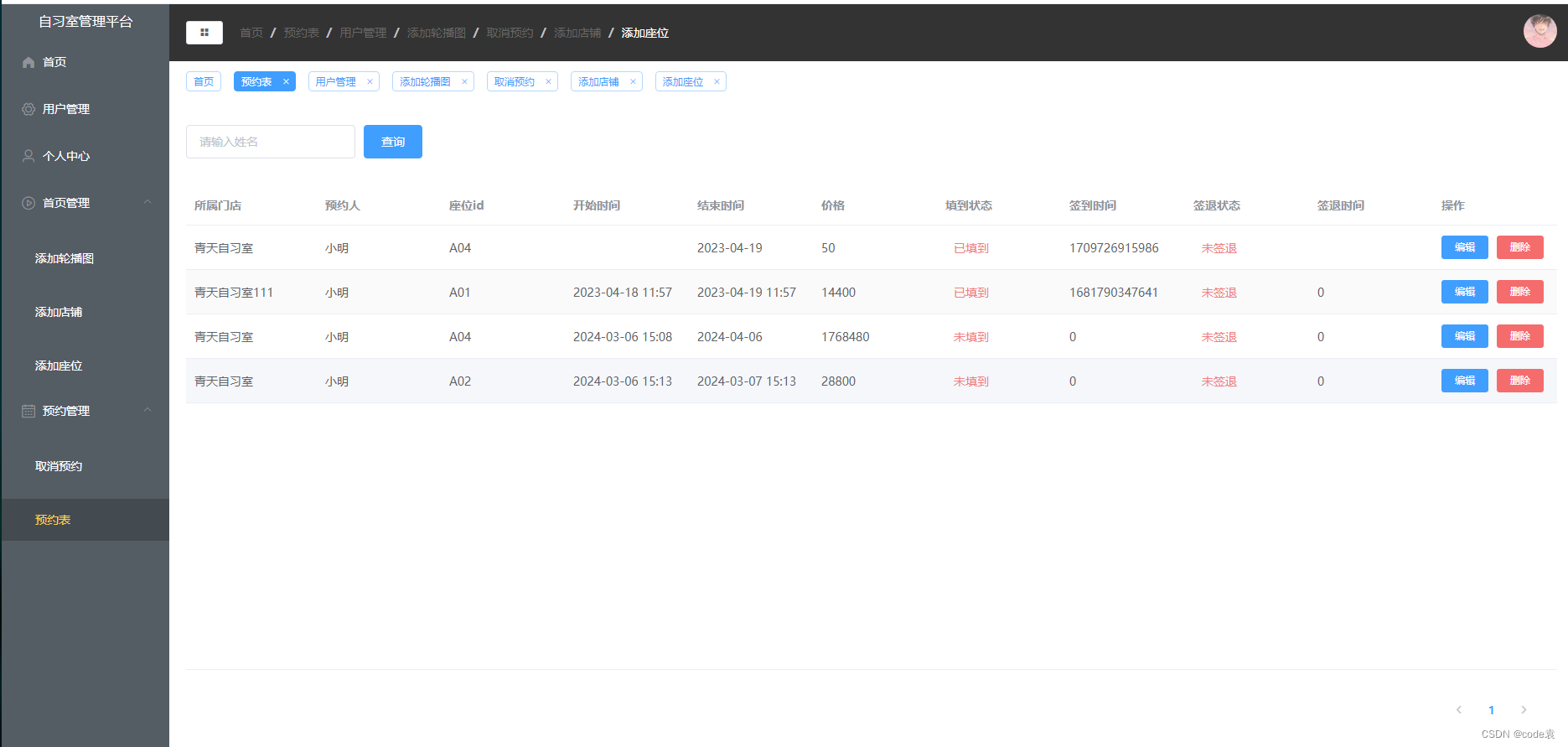
预约自习室
预约自习室 1、技术介绍 自习室预约系统的后端开发语言采用Node,后端开发框架采用Express,数据库采用的Node的最佳搭档MySQL。采用Vue作为前端开发框架,Element-UI作为开发的组件库,微信小程序。期间采用axios实现网页数据获取&a…...
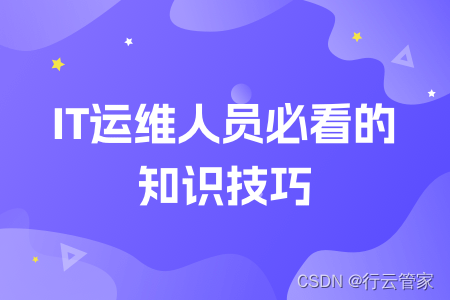
网络安全审计是什么意思?与等保测评有什么区别?
网络安全审计和等保测评在信息安全领域中都是非常重要的环节。但不少人对于这两者是傻傻分不清楚,今天我们就来简单聊聊网络安全审计是什么意思?与等保测评有什么区别? 网络安全审计是什么意思? 网络安全审计是通过对网络系统和网…...
HarmonyOS学习——HarmonyOS习题
harmonyOS开发学习课程 HarmonyOS第一课 1.【习题】运行Hello World工程 判断题 1. DevEco Studio是开发HarmonyOS应用的一站式集成开发环境。(√) 2. main_pages.json存放页面page路径配置信息。(√) 单选题 1. 在stage模…...
Python程序怎么让鼠标键盘在后台进行点击,不干扰用户其他鼠标键盘操作
在Python中实现鼠标和键盘在后台点击而不干扰用户的其他操作是一个比较复杂的任务。大多数库,如pyautogui或pynput,都是直接控制鼠标和键盘的,这意味着它们的操作会干扰用户的正常活动。 为了在不干扰用户的情况下实现这一点,你可…...
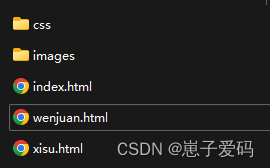
HTML静态网页成品作业(HTML+CSS)——新年春节介绍网页设计制作(3个页面)
🎉不定期分享源码,关注不丢失哦 文章目录 一、作品介绍二、作品演示1、首页2、子页13、子页2 三、代码目录四、网站代码HTML部分代码CSS部分代码 五、源码获取 一、作品介绍 🏷️本套采用HTMLCSS,未使用Javacsript代码࿰…...
vue实现base64格式转换为图片
找了很多,但是都不太好用,打算自己总结一个保姆级教学,无需动脑,电脑有电就能实现 在HTML部分,我们需要一个标签来放置图片 <template><div><img :src"imageSrc" alt"未获取到图片&qu…...
React Native 开发环境搭建(全平台详解)
React Native 开发环境搭建(全平台详解) 在开始使用 React Native 开发移动应用之前,正确设置开发环境是至关重要的一步。本文将为你提供一份全面的指南,涵盖 macOS 和 Windows 平台的配置步骤,如何在 Android 和 iOS…...
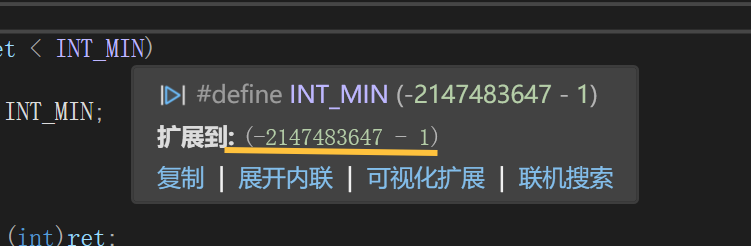
练习(含atoi的模拟实现,自定义类型等练习)
一、结构体大小的计算及位段 (结构体大小计算及位段 详解请看:自定义类型:结构体进阶-CSDN博客) 1.在32位系统环境,编译选项为4字节对齐,那么sizeof(A)和sizeof(B)是多少? #pragma pack(4)st…...
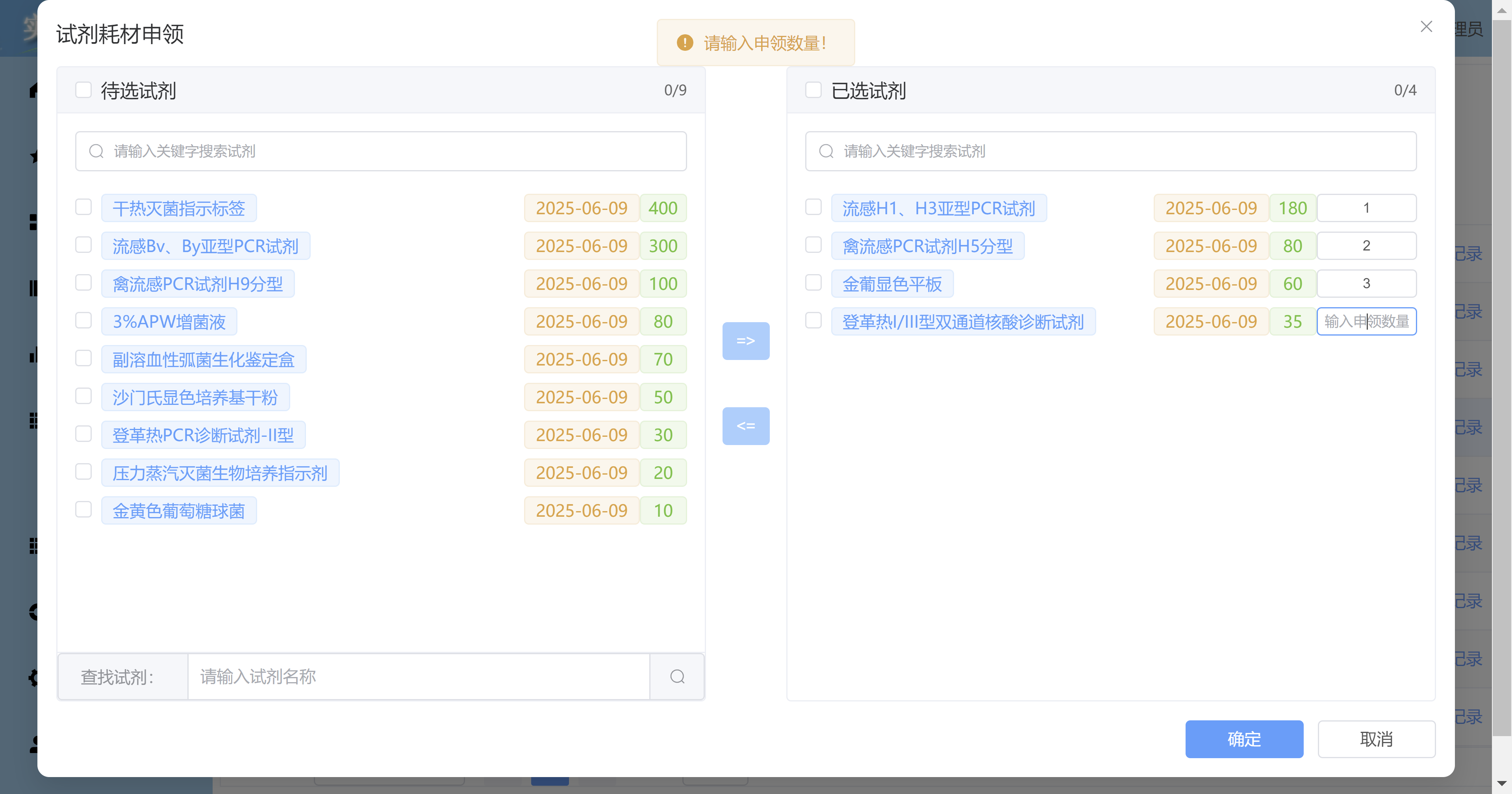
Vue3 + Element Plus + TypeScript中el-transfer穿梭框组件使用详解及示例
使用详解 Element Plus 的 el-transfer 组件是一个强大的穿梭框组件,常用于在两个集合之间进行数据转移,如权限分配、数据选择等场景。下面我将详细介绍其用法并提供一个完整示例。 核心特性与用法 基本属性 v-model:绑定右侧列表的值&…...
前端倒计时误差!
提示:记录工作中遇到的需求及解决办法 文章目录 前言一、误差从何而来?二、五大解决方案1. 动态校准法(基础版)2. Web Worker 计时3. 服务器时间同步4. Performance API 高精度计时5. 页面可见性API优化三、生产环境最佳实践四、终极解决方案架构前言 前几天听说公司某个项…...
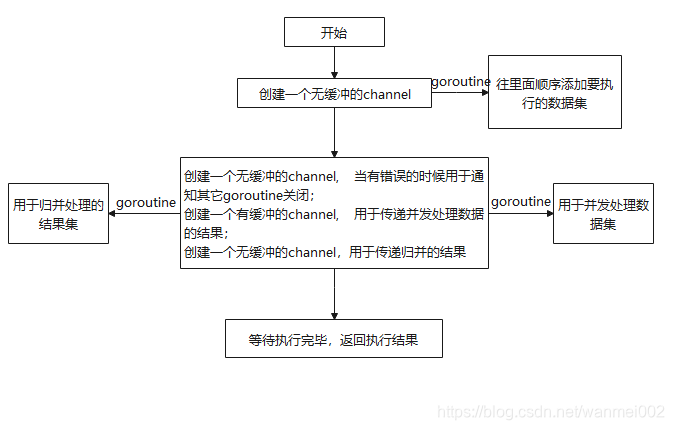
微服务商城-商品微服务
数据表 CREATE TABLE product (id bigint(20) UNSIGNED NOT NULL AUTO_INCREMENT COMMENT 商品id,cateid smallint(6) UNSIGNED NOT NULL DEFAULT 0 COMMENT 类别Id,name varchar(100) NOT NULL DEFAULT COMMENT 商品名称,subtitle varchar(200) NOT NULL DEFAULT COMMENT 商…...
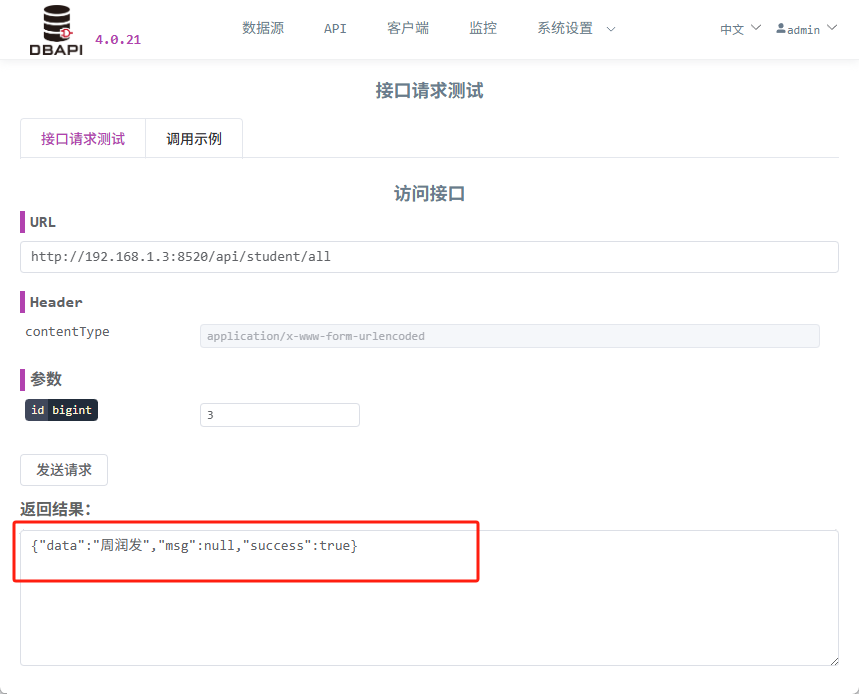
DBAPI如何优雅的获取单条数据
API如何优雅的获取单条数据 案例一 对于查询类API,查询的是单条数据,比如根据主键ID查询用户信息,sql如下: select id, name, age from user where id #{id}API默认返回的数据格式是多条的,如下: {&qu…...
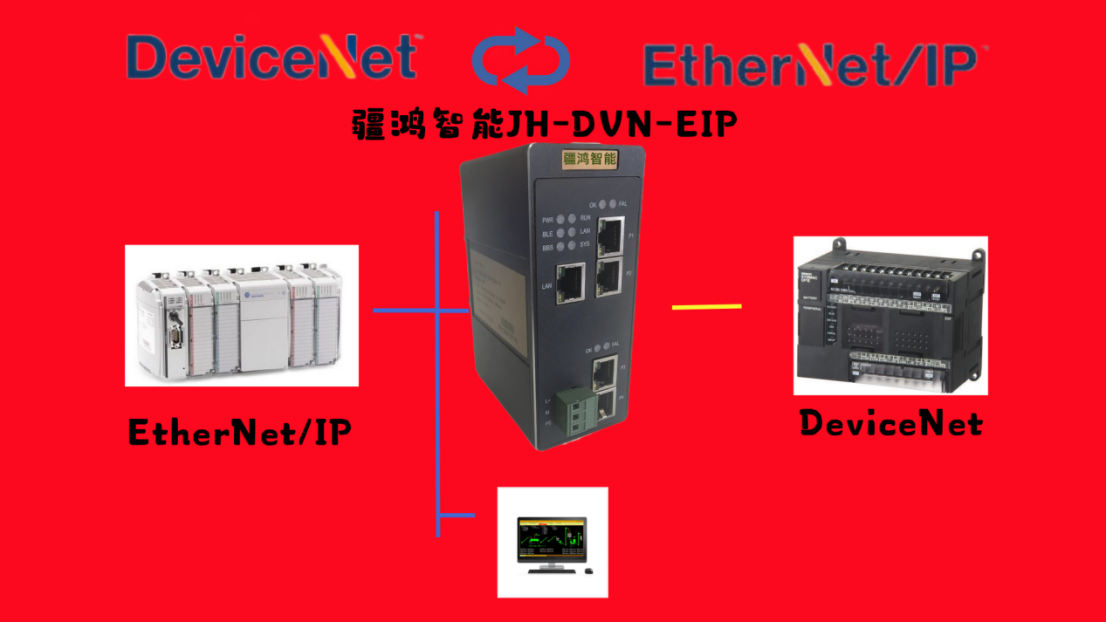
EtherNet/IP转DeviceNet协议网关详解
一,设备主要功能 疆鸿智能JH-DVN-EIP本产品是自主研发的一款EtherNet/IP从站功能的通讯网关。该产品主要功能是连接DeviceNet总线和EtherNet/IP网络,本网关连接到EtherNet/IP总线中做为从站使用,连接到DeviceNet总线中做为从站使用。 在自动…...
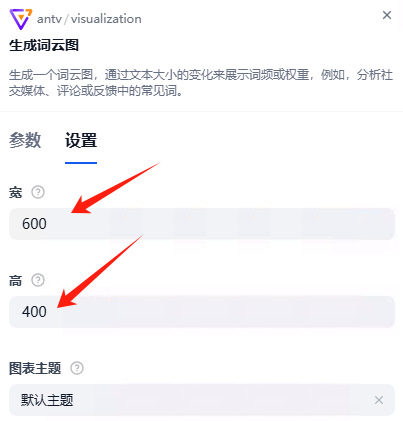
dify打造数据可视化图表
一、概述 在日常工作和学习中,我们经常需要和数据打交道。无论是分析报告、项目展示,还是简单的数据洞察,一个清晰直观的图表,往往能胜过千言万语。 一款能让数据可视化变得超级简单的 MCP Server,由蚂蚁集团 AntV 团队…...
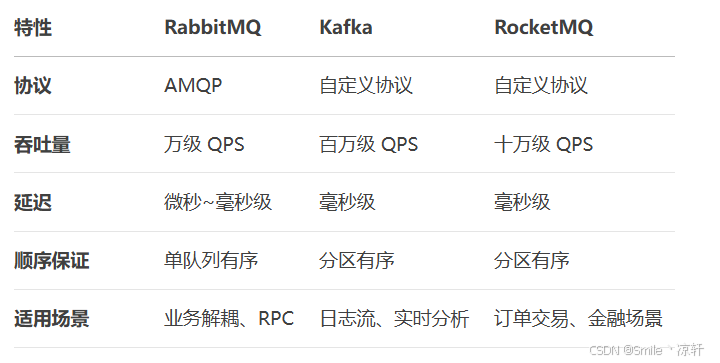
技术栈RabbitMq的介绍和使用
目录 1. 什么是消息队列?2. 消息队列的优点3. RabbitMQ 消息队列概述4. RabbitMQ 安装5. Exchange 四种类型5.1 direct 精准匹配5.2 fanout 广播5.3 topic 正则匹配 6. RabbitMQ 队列模式6.1 简单队列模式6.2 工作队列模式6.3 发布/订阅模式6.4 路由模式6.5 主题模式…...
【LeetCode】3309. 连接二进制表示可形成的最大数值(递归|回溯|位运算)
LeetCode 3309. 连接二进制表示可形成的最大数值(中等) 题目描述解题思路Java代码 题目描述 题目链接:LeetCode 3309. 连接二进制表示可形成的最大数值(中等) 给你一个长度为 3 的整数数组 nums。 现以某种顺序 连接…...