LangChain-15 Manage Prompt Size 管理上下文大小,用Agent的方式询问问题,并去百科检索内容,总结后返回
背景描述
这一节内容比较复杂:
- 涉及到使用工具进行百科的检索(有现成的插件)
- 有
AgentExecutor
来帮助我们执行 - 后续由于上下文过大, 我们通过计算
num_tokens
,来控制我们的上下文
安装依赖
pip install --upgrade --quiet langchain langchain-openai wikipedia
代码编写
GPT 3.5 Turbo 解决这个问题总是出错,偶尔可以正常解决,所以这里使用了 GPT-4-Turbo,准确率基本时100%
。
from operator import itemgetter
from langchain.agents import AgentExecutor, load_tools
from langchain.agents.format_scratchpad import format_to_openai_function_messages
from langchain.agents.output_parsers import OpenAIFunctionsAgentOutputParser
from langchain.tools import WikipediaQueryRun
from langchain_community.utilities import WikipediaAPIWrapper
from langchain_core.prompt_values import ChatPromptValue
from langchain_core.prompts import ChatPromptTemplate, MessagesPlaceholder
from langchain_openai import ChatOpenAI# Initialize Wikipedia tool with a wrapper for querying
wiki = WikipediaQueryRun(api_wrapper=WikipediaAPIWrapper(top_k_results=5, doc_content_chars_max=10_000)
)
tools = [wiki]prompt = ChatPromptTemplate.from_messages([("system", "You are a helpful assistant"),("user", "{input}"),MessagesPlaceholder(variable_name="agent_scratchpad"),]
)
llm = ChatOpenAI(model="gpt-4-0125-preview")agent = ({"input": itemgetter("input"),"agent_scratchpad": lambda x: format_to_openai_function_messages(x["intermediate_steps"]),}| prompt| llm.bind_functions(tools)| OpenAIFunctionsAgentOutputParser()
)agent_executor = AgentExecutor(agent=agent, tools=tools, verbose=True)
# agent_executor.invoke(
# {
# "input": "Who is the current US president? What's their home state? What's their home state's bird? What's that bird's scientific name?"
# }
# )agent_executor.invoke({"input": "大模型Grok是什么?作者是谁?他还干了什么?Grok是开源模型吗?如果是什么时候开源的?"}
)
运行结果
➜ python3 test15.py
/Users/wuzikang/Desktop/py/langchain_test/own_learn/env/lib/python3.12/site-packages/langchain/tools/__init__.py:63: LangChainDeprecationWarning: Importing tools from langchain is deprecated. Importing from langchain will no longer be supported as of langchain==0.2.0. Please import from langchain-community instead:`from langchain_community.tools import WikipediaQueryRun`.To install langchain-community run `pip install -U langchain-community`.warnings.warn(> Entering new AgentExecutor chain...Invoking: `wikipedia` with `Grok large model`Page: Grok (chatbot)
Summary: Grok is a generative artificial intelligence chatbot developed by xAI, based on a large language model (LLM). It was developed as an initiative by Elon Musk as a direct response to the rise of OpenAI's ChatGPT which Musk co-founded. The chatbot is advertised as "having a sense of humor" and direct access to Twitter (X). It is currently under beta testing for those with the premium version of X.Page: Large language model
Summary: A large language model (LLM) is a language model notable for its ability to achieve general-purpose language generation and other natural language processing tasks such as classification. LLMs acquire these abilities by learning statistical relationships from text documents during a computationally intensive self-supervised and semi-supervised training process. LLMs can be used for text generation, a form of generative AI, by taking an input text and repeatedly predicting the next token or word.LLMs are artificial neural networks. The largest and most capable are built with a decoder-only transformer-based architecture while some recent implementations are based on other architectures, such as recurrent neural network variants and Mamba (a state space model).Up to 2020, fine tuning was the only way a model could be adapted to be able to accomplish specific tasks. Larger sized models, such as GPT-3, however, can be prompt-engineered to achieve similar results. They are thought to acquire knowledge about syntax, semantics and "ontology" inherent in human language corpora, but also inaccuracies and biases present in the corpora.Some notable LLMs are OpenAI's GPT series of models (e.g., GPT-3.5 and GPT-4, used in ChatGPT and Microsoft Copilot), Google's PaLM and Gemini (the latter of which is currently used in the chatbot of the same name), xAI's Grok, Meta's LLaMA family of open-source models, Anthropic's Claude models, and Mistral AI's open source models.Page: Gemini (language model)
Summary: Gemini is a family of multimodal large language models developed by Google DeepMind, serving as the successor to LaMDA and PaLM 2. Comprising Gemini Ultra, Gemini Pro, and Gemini Nano, it was announced on December 6, 2023, positioned as a competitor to OpenAI's GPT-4. It powers the generative artificial intelligence chatbot of the same name.Page: Language model
Summary: A language model is a probabilistic model of a natural language. In 1980, the first significant statistical language model was proposed, and during the decade IBM performed ‘Shannon-style’ experiments, in which potential sources for language modeling improvement were identified by observing and analyzing the performance of human subjects in predicting or correcting text.Language models are useful for a variety of tasks, including speech recognition (helping prevent predictions of low-probability (e.g. nonsense) sequences), machine translation, natural language generation (generating more human-like text), optical character recognition, handwriting recognition, grammar induction, and information retrieval.Large language models, currently their most advanced form, are a combination of larger datasets (frequently using scraped words from the public internet), feedforward neural networks, and transformers. They have superseded recurrent neural network-based models, which had previously superseded the pure statistical models, such as word n-gram language model.Page: ChatGPT
Summary: ChatGPT (Chat Generative Pre-trained Transformer) is a chatbot developed by OpenAI and launched on November 30, 2022. Based on a large language model, it enables users to refine and steer a conversation towards a desired length, format, style, level of detail, and language. Successive prompts and replies, known as prompt engineering, are considered at each conversation stage as a context.By January 2023, it had become what was then the fastest-growing consumer software application in history, gaining over 100 million users and contributing to the growth of OpenAI's valuation to $29 billion. ChatGPT's release spurred the release of competing products, including Gemini, Ernie Bot, LLaMA, Claude, and Grok. Microsoft launched Copilot, based on OpenAI's GPT-4. Some observers raised concern about the potential of ChatGPT and similar programs to displace or atrophy human intelligence, enable plagiarism, or fuel misinformation.ChatGPT is available for use online in two versions, one built on GPT-3.5 and the other on GPT-4, both of which are members of OpenAI's proprietary series of generative pre-trained transformer (GPT) models, based on the transformer architecture developed by Google—and is fine-tuned for conversational applications using a combination of supervised learning and reinforcement learning from human feedback. ChatGPT was released as a freely available research preview, but due to its popularity, OpenAI now operates the service on a freemium model. It allows users on its free tier to access the GPT-3.5-based version, while the more advanced GPT-4-based version and priority access to newer features are provided to paid subscribers under the commercial name "ChatGPT Plus".
ChatGPT is credited with starting the AI boom, which has led to ongoing rapid and unprecedented investment in and public attention to the field of artificial intelligence.Grok是一个基于大型语言模型(LLM)的生成式人工智能聊天机器人,由xAI开发。Grok的开发是由Elon Musk作为对OpenAI推出的ChatGPT崛起的直接回应而启动的项目,其中Elon Musk是OpenAI的共同创始人。Grok的一个特点是它被宣传为“具有幽默感”,并且可以直接访问Twitter(现X)。目前,Grok仍处于Beta测试阶段,仅对X的高级版用户开放。至于Grok是否是一个开源模型,从目前的信息来看,并没有提及Grok是一个开源项目。通常,是否开源以及开源的时间点是由开发该模型的组织或团队决定的,关于Grok的开源状态,可能需要进一步从xAI或相关的官方消息中获取确切信息。Elon Musk是一位知名的企业家和工程师,他创办或领导了多个著名的技术和航天公司,包括SpaceX、Tesla Inc.、Neuralink和The Boring Company。他在推动太空探索、电动汽车发展和人工智能领域都有显著的贡献。> Finished chain.
可以看到 Agent
帮助我们执行总结出了结果:
Grok是一个基于大型语言模型(LLM)的生成式人工智能聊天机器人,由xAI开发。Grok的开发是由Elon Musk作为对OpenAI推出的ChatGPT崛起的直接回应而启动的项目,其中Elon Musk是OpenAI的共同创始人。Grok的一个特点是它被宣传为“具有幽默感”,并且可以直接访问Twitter(现X)。目前,Grok仍处于Beta测试阶段,仅对X的高级版用户开放。至于Grok是否是一个开源模型,从目前的信息来看,并没有提及Grok是一个开源项目。通常,是否开源以及开源的时间点是由开发该模型的组织或团队决定的,关于Grok的开源状态,可能需要进一步从xAI或相关的官方消息中获取确切信息。Elon Musk是一位知名的企业家和工程师,他创办或领导了多个著名的技术和航天公司,包括SpaceX、Tesla Inc.、Neuralink和The Boring Company。他在推动太空探索、电动汽车发展和人工智能领域都有显著的贡献。
消耗情况
由于上下文过大,资费是非常恐怖的
优化代码
我们通过定义了一个condense_prompt函数来计算和控制上下文
# 控制上下文大小
def condense_prompt(prompt: ChatPromptValue) -> ChatPromptValue:messages = prompt.to_messages()num_tokens = llm.get_num_tokens_from_messages(messages)ai_function_messages = messages[2:]# 这里限制了while num_tokens > 4_000:ai_function_messages = ai_function_messages[2:]num_tokens = llm.get_num_tokens_from_messages(messages[:2] + ai_function_messages)messages = messages[:2] + ai_function_messagesreturn ChatPromptValue(messages=messages)
完整的代码如下
from operator import itemgetter
from langchain.agents import AgentExecutor, load_tools
from langchain.agents.format_scratchpad import format_to_openai_function_messages
from langchain.agents.output_parsers import OpenAIFunctionsAgentOutputParser
from langchain.tools import WikipediaQueryRun
from langchain_community.utilities import WikipediaAPIWrapper
from langchain_core.prompt_values import ChatPromptValue
from langchain_core.prompts import ChatPromptTemplate, MessagesPlaceholder
from langchain_openai import ChatOpenAI# Initialize Wikipedia tool with a wrapper for querying
wiki = WikipediaQueryRun(api_wrapper=WikipediaAPIWrapper(top_k_results=5, doc_content_chars_max=10_000)
)
tools = [wiki]prompt = ChatPromptTemplate.from_messages([("system", "You are a helpful assistant"),("user", "{input}"),MessagesPlaceholder(variable_name="agent_scratchpad"),]
)
llm = ChatOpenAI(model="gpt-4-0125-preview")# 控制上下文大小
def condense_prompt(prompt: ChatPromptValue) -> ChatPromptValue:messages = prompt.to_messages()num_tokens = llm.get_num_tokens_from_messages(messages)ai_function_messages = messages[2:]# 这里限制了while num_tokens > 4_000:ai_function_messages = ai_function_messages[2:]num_tokens = llm.get_num_tokens_from_messages(messages[:2] + ai_function_messages)messages = messages[:2] + ai_function_messagesreturn ChatPromptValue(messages=messages)# 注意在Chain中加入
agent = ({"input": itemgetter("input"),"agent_scratchpad": lambda x: format_to_openai_function_messages(x["intermediate_steps"]),}| prompt| condense_prompt| llm.bind_functions(tools)| OpenAIFunctionsAgentOutputParser()
)agent_executor = AgentExecutor(agent=agent, tools=tools, verbose=True)
# agent_executor.invoke(
# {
# "input": "Who is the current US president? What's their home state? What's their home state's bird? What's that bird's scientific name?"
# }
# )agent_executor.invoke({"input": "大模型Grok是什么?作者是谁?他还干了什么?Grok是开源模型吗?如果是什么时候开源的?"}
)
相关文章:
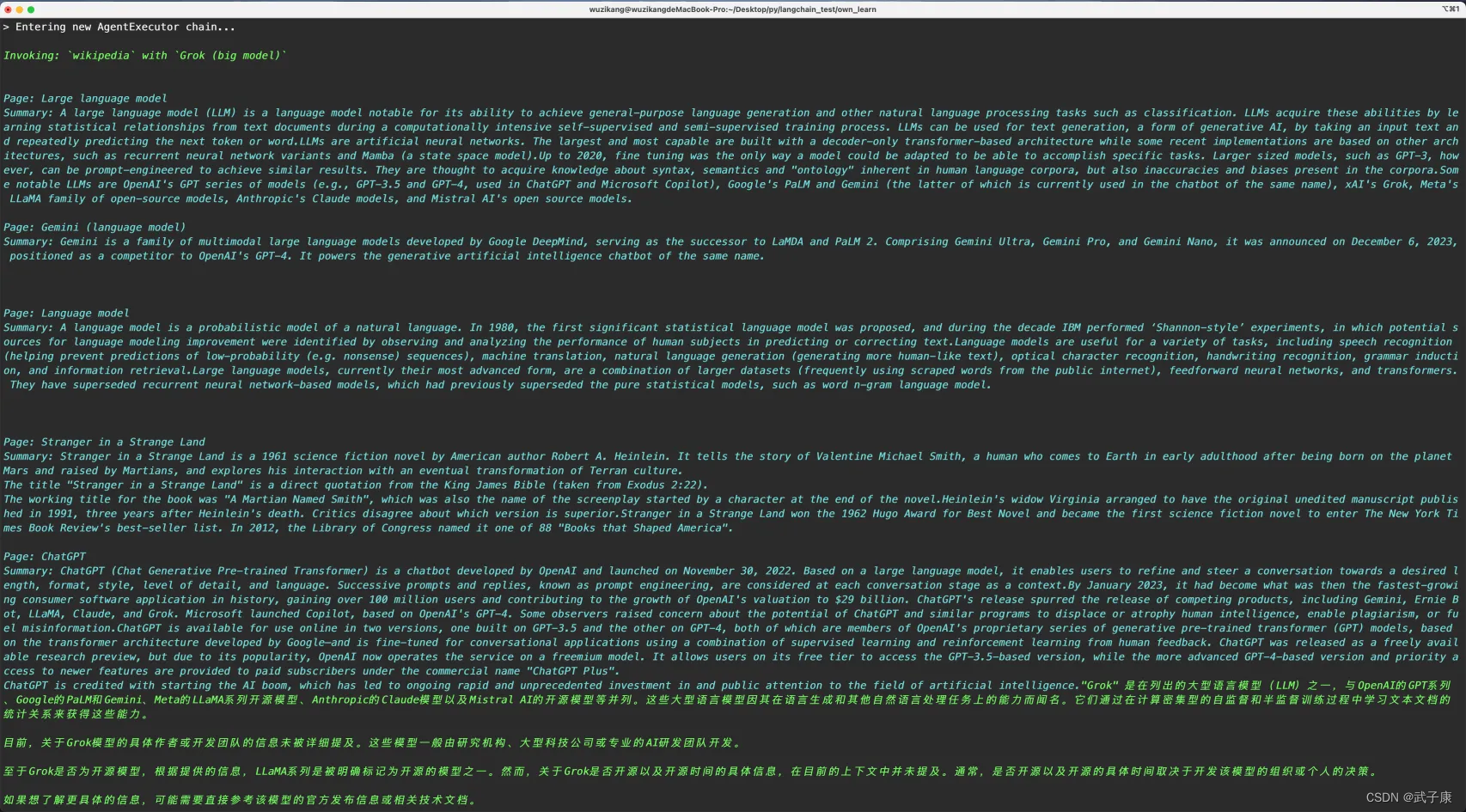
LangChain-15 Manage Prompt Size 管理上下文大小,用Agent的方式询问问题,并去百科检索内容,总结后返回
背景描述 这一节内容比较复杂: 涉及到使用工具进行百科的检索(有现成的插件)有AgentExecutor来帮助我们执行后续由于上下文过大, 我们通过计算num_tokens,来控制我们的上下文 安装依赖 pip install --upgrade --qu…...
OR-TOOL 背包算法
起因:最近公司要发票自动匹配, 比如财务输入10000W块,找到发票中能凑10000的。然后可以快速核销。 废话不多, 一 官方文档 https://developers.google.cn/optimization/pack/knapsack?hlzh-cn 二 POM文件 <!--google 算法包…...
前端h5录音
时隔差不多半个月, 现在才来写这编博客。由于某些原因,我一直没有写,请大家原谅。前段时间开发了一个小模块。模块的主要功能就是有一个录音的功能。也就是说,模仿微信发送语音的功能一样。不多说,直接来一段代码 //自…...
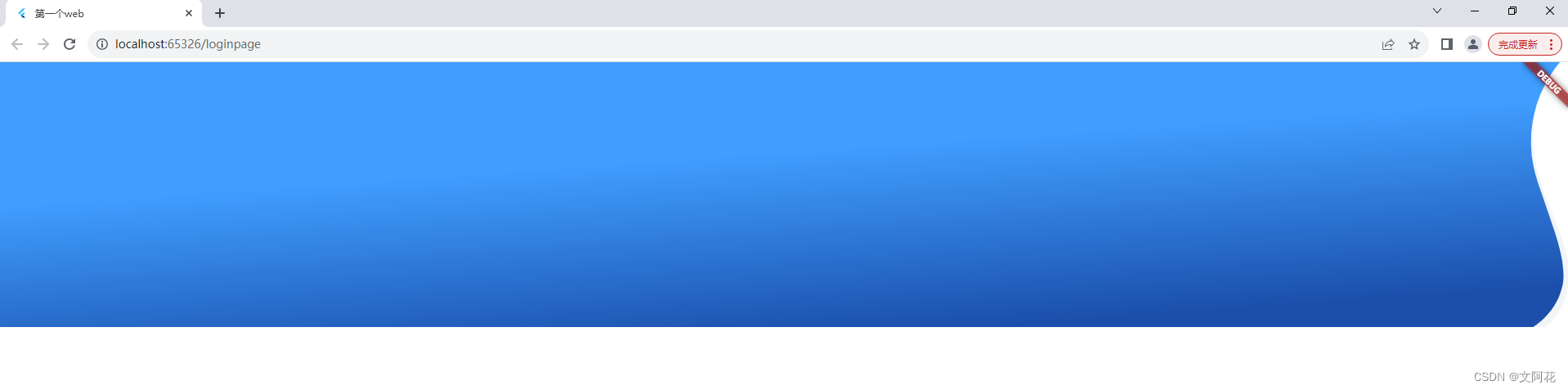
Android Studio 使用Flutter开发第一个Web页面(进行中)
附上Flutter官方文档 1、新建Flutter项目(需要勾选web选项) 新建项目构成为: 2、配置 Flutter 使用 path 策略 官方文档 在main.dart中,需要导入flutter_web_plugins/url_strategy.dart包,并在main(){}函数中usePath…...
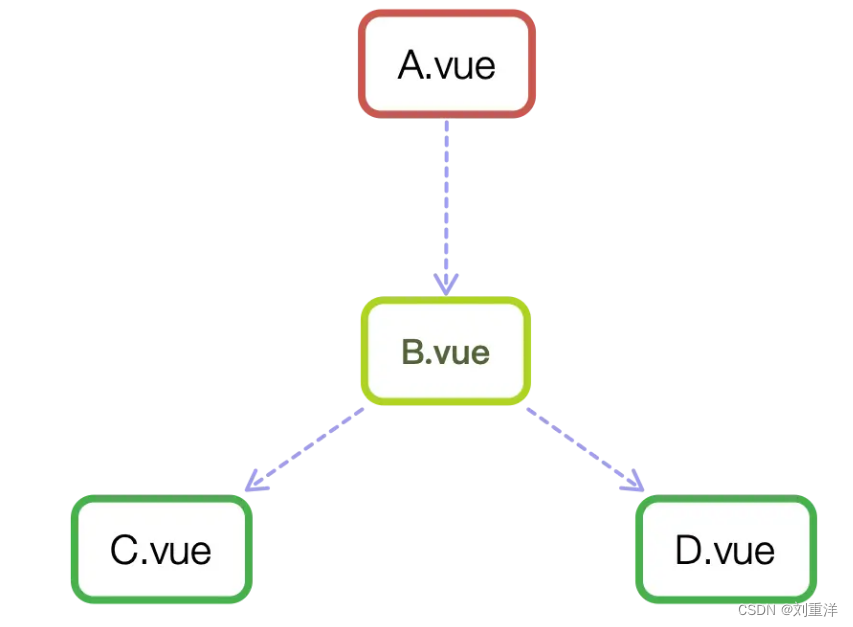
Vue.js组件精讲 第2章 基础:Vue.js组件的三个API:prop、event、slot
如果您已经对 Vue.js 组件的基础用法了如指掌,可以跳过本小节,不过当做复习稍读一下也无妨。 组件的构成 一个再复杂的组件,都是由三部分组成的:prop、event、slot,它们构成了 Vue.js 组件的 API。如果你开发的是一个…...
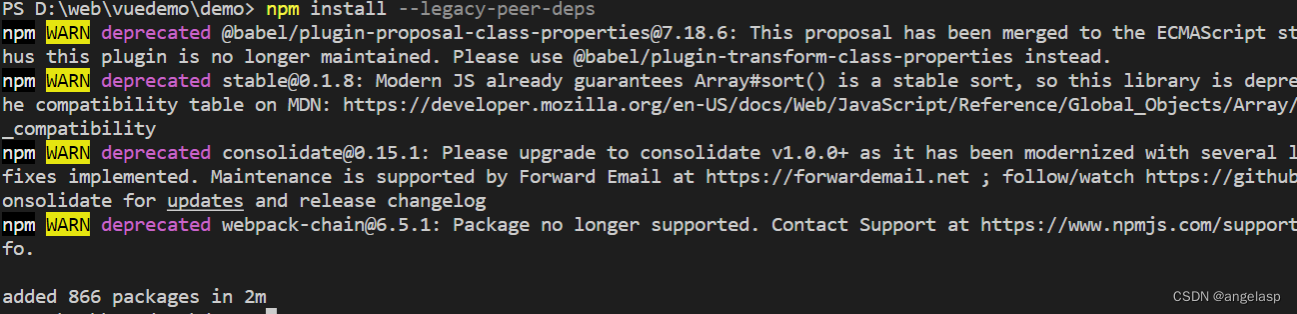
npm install 报 ERESOLVE unable to resolve dependency tree 异常解决方法
问题 在安装项目依赖时,很大可能会遇到安装不成功的问题,其中有一个很大的原因,可能就是因为你的npm版本导致的。 1.npm ERR! code ERESOLVE npm ERR! ERESOLVE unable to resolve dependency tree 2.ERESOLVE unable to resolve dependenc…...

RPC还是HTTP
RPC是一个远程调用的通讯协议 RPC要比HTTP快一些 1. HTTP体积大 原因是HTTP协议会带着一堆无用信息 HTTP由三部分组成 请求头 请求行 请求体 这三部分只有请求体是需要的 2. HTTP支持的序列化协议比较少 RPC支持更多轻量级的通讯协议 3. RPC协议支持定制...
Conda 常用命令总结
创建虚拟环境 conda create -n name python[your_version] 激活环境 conda activate name 退出环境 conda deactivate 查看虚拟环境 conda info --envs 删除虚拟环境 conda remove -n name --all 删除所有的安装包及cache(索引缓存、锁定文件、未使用过的包和tar包) …...
Spring MVC 文件上传和下载
文章目录 Spring MVC 中文件上传利用 commons-fileupload 文件上传使用 Servlet 3.1 内置的文件上传功能 Spring MVC 中文件下载 Spring MVC 中文件上传 为了能上传文件,必须将 from 表单的 method 设置为 POST,并将 enctype 设置为 multipart/form-data…...
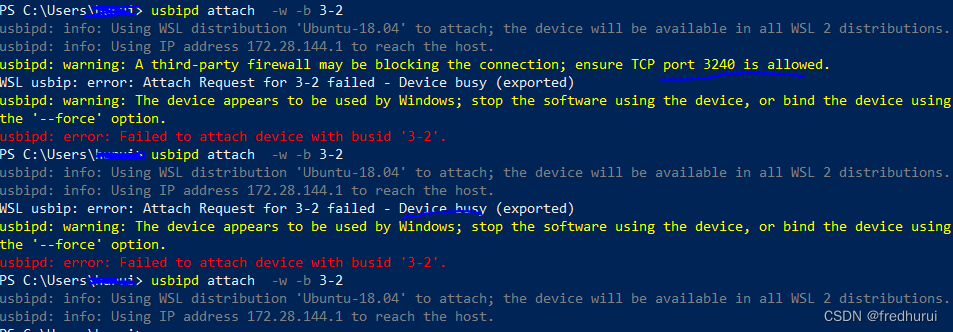
WSL访问adb usb device
1.Windows上用PowerShell运行: winget install --interactive --exact dorssel.usbipd-win 2.在WSLUbuntu上终端运行: sudo apt install linux-tools-generic hwdata sudo update-alternatives --install /usr/local/bin/usbip usbip /usr/lib/linux-too…...
CDF与PDF(描述随机变量的分布情况)
一、概念解释 CDF(Cumulative Distribution Function)和PDF(Probability Density Function)是概率论和统计学中常用的两个评价指标,用于描述随机变量的分布情况。 1. CDF(累积分布函数): - CDF是描述随机变量在某个取值及其之前所有可能取值的概率的函数。它表示了累…...
react项目中需要条形码功能,安装react-barcode使用时报错
react项目中需要条形码功能,用yarn add安装react-barcode后,在项目中使用import Barcode from ‘react-barcode’,页面中一直白屏,加载中 查看控制台报以下错误 load component failed Error: Module "./react-barcode"…...

ES6基础(JavaScript基础)
本文用于检验学习效果,忘记知识就去文末的链接复习 1. ECMAScript介绍 ECMAScript是一种由Ecma国际(前身为欧洲计算机制造商协会,英文名称是European Computer Manufacturers Association)通过ECMA-262标准化的脚本程序设计语言…...
[蓝桥杯] 纸张尺寸(C语言)
题目链接 蓝桥杯2022年第十三届省赛真题-纸张尺寸 - C语言网 题目理解 输入一行包含一个字符串表示纸张的名称,该名称一定是 A0、A1、A2、A3、A4、A5、A6、A7、A8、A9 之一,输出两行,每行包含一个整数,依次表示长边和短边的长度…...
AI推介-多模态视觉语言模型VLMs论文速览(arXiv方向):2024.04.05-2024.04.10
文章目录~ 1.BRAVE: Broadening the visual encoding of vision-language models2.ORacle: Large Vision-Language Models for Knowledge-Guided Holistic OR Domain Modeling3.MedRG: Medical Report Grounding with Multi-modal Large Language Model4.InternLM-XComposer2-4…...
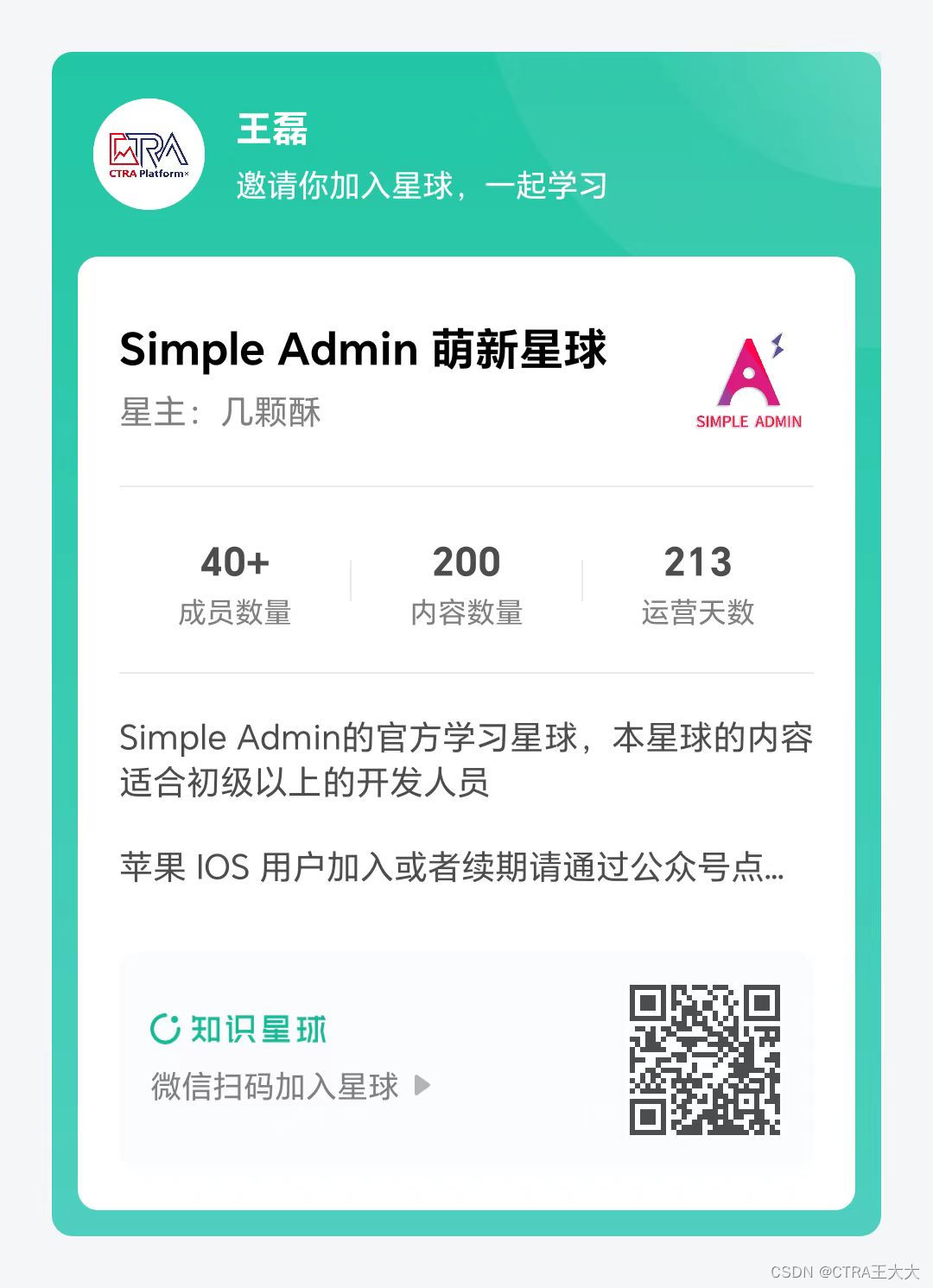
【golang】动态生成微信小程序二维码实战下:golang 生成 小程序二维码图片 并通过s3协议上传到对象存储桶 | 腾讯云 cos
项目背景 在自研的系统,需要实现类似草料二维码的功能 将我们自己的小程序,通过代码生成相想要的小程序二维码 代码已经上传到 Github 需要的朋友可以自取 https://github.com/ctra-wang/wechat-mini-qrcode 一、生成Qrcode并提交到对象存储 通过源生A…...
kubeadm k8s 1.24之后版本安装,带cri-dockerd
最后编辑时间:2024/3/26 适用于1.24之后的版本 单节点配置 检查是否已经安装kubectl, kubelet, kubeadm直接输入命令确定,如果提示没有该指令则正确 kubectl kubelet kubeadm如果之前安装,首先reset,然后使用apt remove和snap r…...
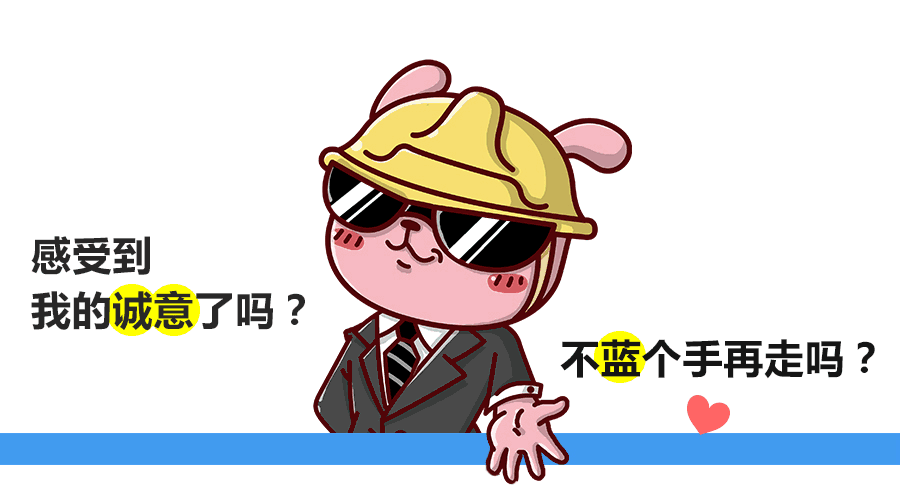
13-pyspark的共享变量用法总结
目录 前言广播变量广播变量的作用 广播变量的使用方式 累加器累加器的作用累加器的优缺点累加器的使用方式 PySpark实战笔记系列第四篇 10-用PySpark建立第一个Spark RDD(PySpark实战笔记系列第一篇)11-pyspark的RDD的变换与动作算子总结(PySpark实战笔记系列第二篇))12-pysp…...
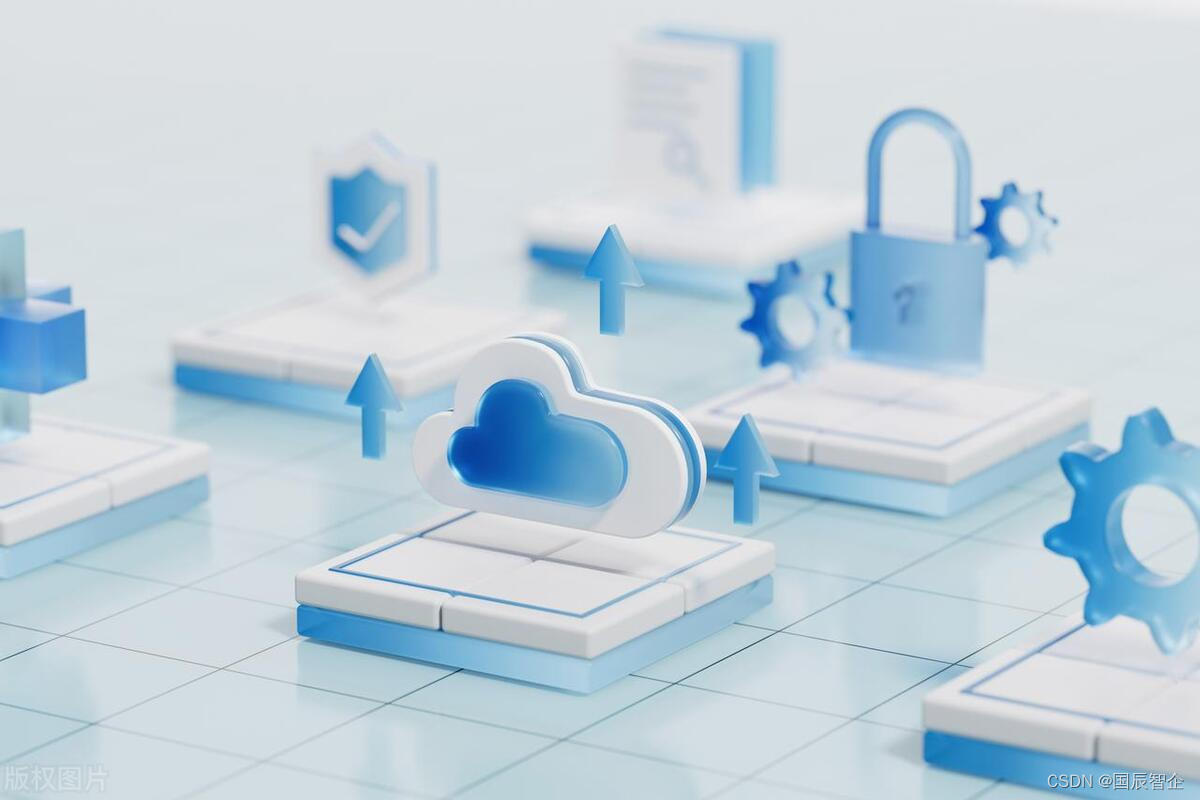
BI数据分析软件:行业趋势与功能特点剖析
随着数据量的爆炸性增长,企业对于数据的需求也日益迫切。BI数据分析软件作为帮助企业实现数据驱动决策的关键工具,在当前的商业环境中扮演着不可或缺的角色。本文将从行业趋势、功能特点以及适用场景等方面,深入剖析BI数据分析软件࿰…...
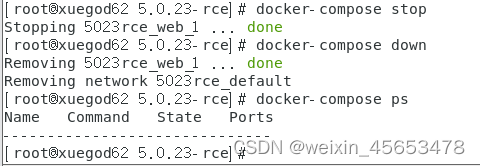
centos7上docker搭建vulhub靶场
1 vulhub靶场概述 VulHub是一个在线靶场平台,提供了丰富的漏洞环境供安全爱好者学习和实践。 该平台主要面向网络安全初学者和进阶者,通过模拟真实的漏洞环境,帮助用户深入了解漏洞的成因、利用方式以及防范措施。 此外,VulHub还…...
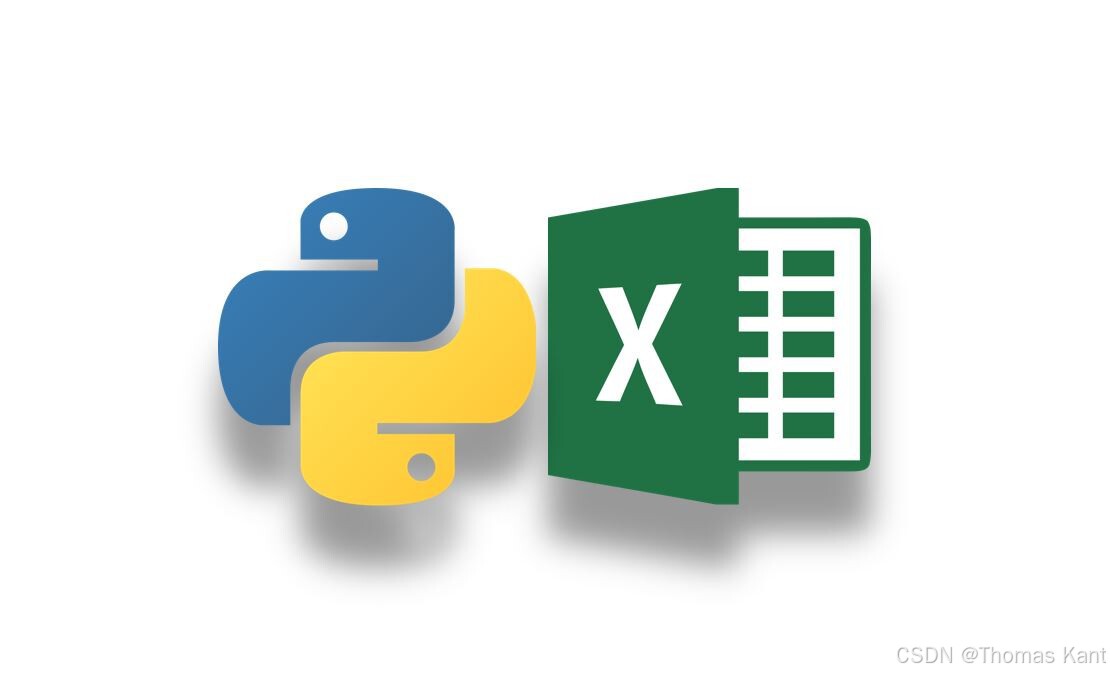
Python:操作 Excel 折叠
💖亲爱的技术爱好者们,热烈欢迎来到 Kant2048 的博客!我是 Thomas Kant,很开心能在CSDN上与你们相遇~💖 本博客的精华专栏: 【自动化测试】 【测试经验】 【人工智能】 【Python】 Python 操作 Excel 系列 读取单元格数据按行写入设置行高和列宽自动调整行高和列宽水平…...
【Java学习笔记】Arrays类
Arrays 类 1. 导入包:import java.util.Arrays 2. 常用方法一览表 方法描述Arrays.toString()返回数组的字符串形式Arrays.sort()排序(自然排序和定制排序)Arrays.binarySearch()通过二分搜索法进行查找(前提:数组是…...
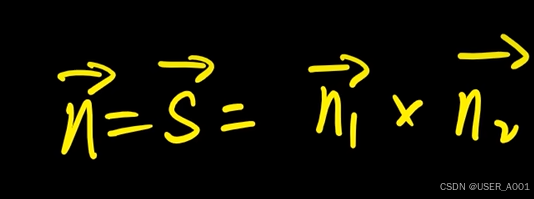
高等数学(下)题型笔记(八)空间解析几何与向量代数
目录 0 前言 1 向量的点乘 1.1 基本公式 1.2 例题 2 向量的叉乘 2.1 基础知识 2.2 例题 3 空间平面方程 3.1 基础知识 3.2 例题 4 空间直线方程 4.1 基础知识 4.2 例题 5 旋转曲面及其方程 5.1 基础知识 5.2 例题 6 空间曲面的法线与切平面 6.1 基础知识 6.2…...
拉力测试cuda pytorch 把 4070显卡拉满
import torch import timedef stress_test_gpu(matrix_size16384, duration300):"""对GPU进行压力测试,通过持续的矩阵乘法来最大化GPU利用率参数:matrix_size: 矩阵维度大小,增大可提高计算复杂度duration: 测试持续时间(秒&…...

Android15默认授权浮窗权限
我们经常有那种需求,客户需要定制的apk集成在ROM中,并且默认授予其【显示在其他应用的上层】权限,也就是我们常说的浮窗权限,那么我们就可以通过以下方法在wms、ams等系统服务的systemReady()方法中调用即可实现预置应用默认授权浮…...
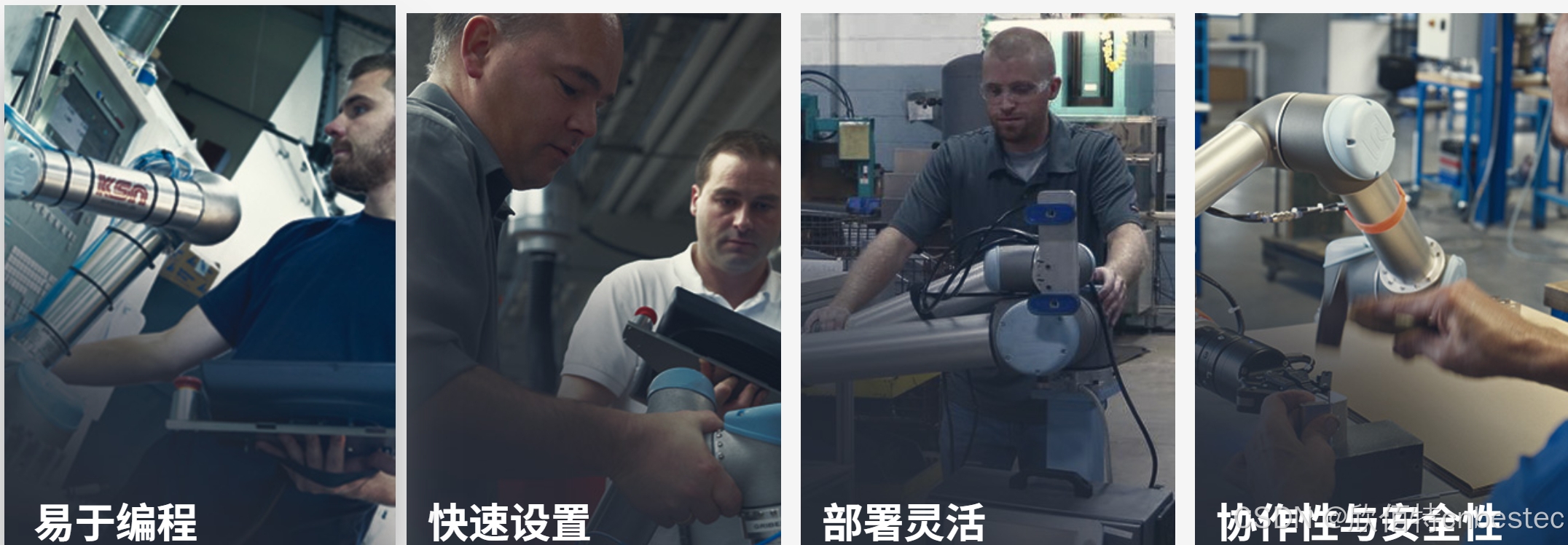
UR 协作机器人「三剑客」:精密轻量担当(UR7e)、全能协作主力(UR12e)、重型任务专家(UR15)
UR协作机器人正以其卓越性能在现代制造业自动化中扮演重要角色。UR7e、UR12e和UR15通过创新技术和精准设计满足了不同行业的多样化需求。其中,UR15以其速度、精度及人工智能准备能力成为自动化领域的重要突破。UR7e和UR12e则在负载规格和市场定位上不断优化…...
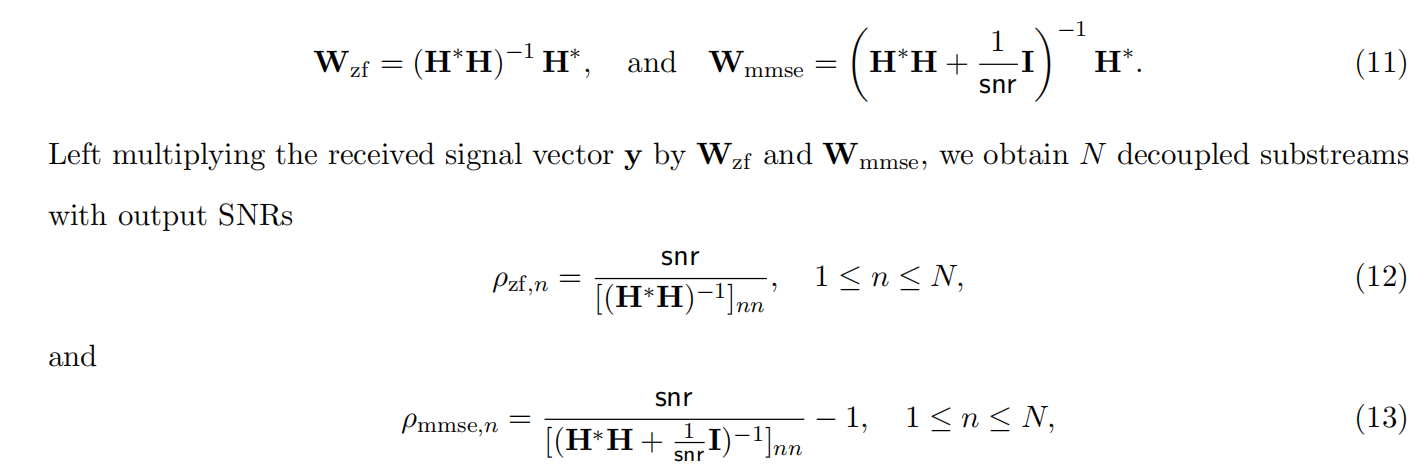
均衡后的SNRSINR
本文主要摘自参考文献中的前两篇,相关文献中经常会出现MIMO检测后的SINR不过一直没有找到相关数学推到过程,其中文献[1]中给出了相关原理在此仅做记录。 1. 系统模型 复信道模型 n t n_t nt 根发送天线, n r n_r nr 根接收天线的 MIMO 系…...
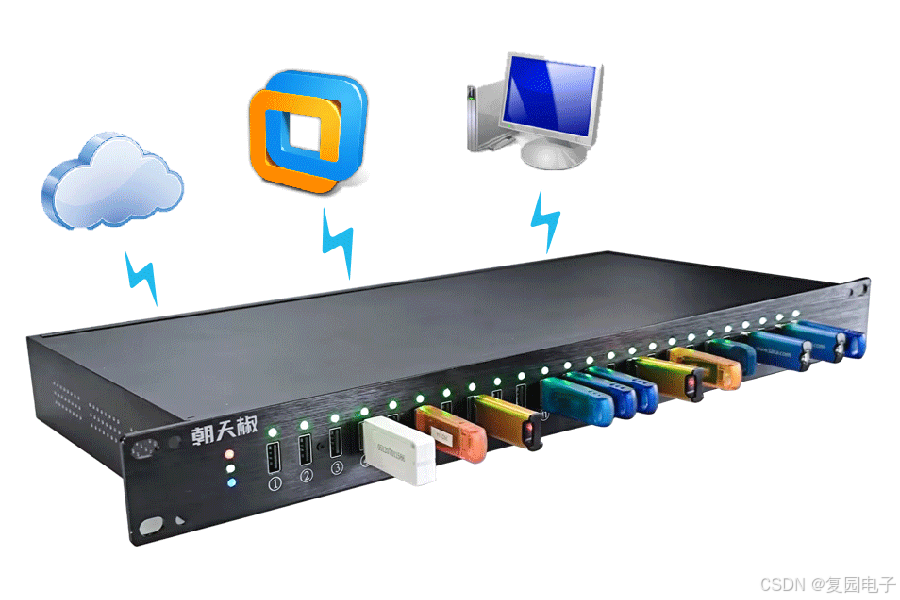
USB Over IP专用硬件的5个特点
USB over IP技术通过将USB协议数据封装在标准TCP/IP网络数据包中,从根本上改变了USB连接。这允许客户端通过局域网或广域网远程访问和控制物理连接到服务器的USB设备(如专用硬件设备),从而消除了直接物理连接的需要。USB over IP的…...
Pinocchio 库详解及其在足式机器人上的应用
Pinocchio 库详解及其在足式机器人上的应用 Pinocchio (Pinocchio is not only a nose) 是一个开源的 C 库,专门用于快速计算机器人模型的正向运动学、逆向运动学、雅可比矩阵、动力学和动力学导数。它主要关注效率和准确性,并提供了一个通用的框架&…...
CSS设置元素的宽度根据其内容自动调整
width: fit-content 是 CSS 中的一个属性值,用于设置元素的宽度根据其内容自动调整,确保宽度刚好容纳内容而不会超出。 效果对比 默认情况(width: auto): 块级元素(如 <div>)会占满父容器…...