resnet网络特征提取过程可视化
我们在训练图片时,是不是要看看具体提取时的每个特征图提取的样子,找了很多,终于功夫不负有心人,找到了,通过修改的代码:
resnet代码:
import torch
import torch.nn as nn
from torchvision.models.utils import load_state_dict_from_url
import math
model_urls = {'resnet18': 'https://download.pytorch.org/models/resnet18-5c106cde.pth','resnet34': 'https://download.pytorch.org/models/resnet34-333f7ec4.pth','resnet50': 'https://download.pytorch.org/models/resnet50-19c8e357.pth','resnet101': 'https://download.pytorch.org/models/resnet101-5d3b4d8f.pth','resnet152': 'https://download.pytorch.org/models/resnet152-b121ed2d.pth',
}class GhostModule(nn.Module):def __init__(self, inp, oup, kernel_size=1, ratio=2, dw_size=3, stride=1, relu=True):super(GhostModule, self).__init__()self.oup = oupinit_channels = math.ceil(oup / ratio)new_channels = init_channels*(ratio-1)self.primary_conv = nn.Sequential(nn.Conv2d(inp, init_channels, kernel_size, stride, kernel_size//2, bias=False),nn.BatchNorm2d(init_channels),nn.ReLU(inplace=True) if relu else nn.Sequential(),)self.cheap_operation = nn.Sequential(nn.Conv2d(init_channels, new_channels, dw_size, 1, dw_size//2, groups=init_channels, bias=False),nn.BatchNorm2d(new_channels),nn.ReLU(inplace=True) if relu else nn.Sequential(),)def forward(self, x):x1 = self.primary_conv(x)x2 = self.cheap_operation(x1)out = torch.cat([x1,x2], dim=1)return out[:,:self.oup,:,:]def conv3x3(in_planes, out_planes, stride=1, groups=1, dilation=1):return nn.Conv2d(in_planes, out_planes, kernel_size=3, stride=stride,padding=dilation, groups=groups, bias=False, dilation=dilation)def conv1x1(in_planes, out_planes, stride=1):return nn.Conv2d(in_planes, out_planes, kernel_size=1, stride=stride, bias=False)class BasicBlock(nn.Module):expansion = 1def __init__(self, inplanes, planes, stride=1, downsample=None, groups=1,base_width=64, dilation=1, norm_layer=None):super(BasicBlock, self).__init__()if norm_layer is None:norm_layer = nn.BatchNorm2dif groups != 1 or base_width != 64:raise ValueError('BasicBlock only supports groups=1 and base_width=64')if dilation > 1:raise NotImplementedError("Dilation > 1 not supported in BasicBlock")# Both self.conv1 and self.downsample layers downsample the input when stride != 1self.conv1 = conv3x3(inplanes, planes, stride)self.bn1 = norm_layer(planes)self.relu = nn.ReLU(inplace=True)self.conv2 = conv3x3(planes, planes)self.bn2 = norm_layer(planes)self.downsample = downsampleself.stride = stridedef forward(self, x):identity = xout = self.conv1(x)out = self.bn1(out)out = self.relu(out)out = self.conv2(out)out = self.bn2(out)if self.downsample is not None:identity = self.downsample(x)out += identityout = self.relu(out)return outclass Bottleneck(nn.Module):expansion = 4def __init__(self, inplanes, planes, stride=1, downsample=None, groups=1,base_width=64, dilation=1, norm_layer=None):super(Bottleneck, self).__init__()if norm_layer is None:norm_layer = nn.BatchNorm2dwidth = int(planes * (base_width / 64.)) * groups# Both self.conv2 and self.downsample layers downsample the input when stride != 1self.conv1 = conv1x1(inplanes, width)self.bn1 = norm_layer(width)self.conv2 = conv3x3(width, width, stride, groups, dilation)self.bn2 = norm_layer(width)self.conv3 = conv1x1(width, planes * self.expansion)self.bn3 = norm_layer(planes * self.expansion)self.relu = nn.ReLU(inplace=True)self.downsample = downsampleself.stride = stridedef forward(self, x):identity = xout = self.conv1(x)out = self.bn1(out)out = self.relu(out)out = self.conv2(out)out = self.bn2(out)out = self.relu(out)out = self.conv3(out)out = self.bn3(out)if self.downsample is not None:identity = self.downsample(x)out += identityout = self.relu(out)return outclass ResNet(nn.Module):def __init__(self, block, layers, num_classes=1000, zero_init_residual=False,groups=1, width_per_group=64, replace_stride_with_dilation=None,norm_layer=None):super(ResNet, self).__init__()if norm_layer is None:norm_layer = nn.BatchNorm2dself._norm_layer = norm_layerself.inplanes = 64self.dilation = 1if replace_stride_with_dilation is None:replace_stride_with_dilation = [False, False, False]if len(replace_stride_with_dilation) != 3:raise ValueError("replace_stride_with_dilation should be None ""or a 3-element tuple, got {}".format(replace_stride_with_dilation))self.block = blockself.groups = groupsself.base_width = width_per_group# 224,224,3 -> 112,112,64self.conv1 = nn.Conv2d(3, self.inplanes, kernel_size=7, stride=2, padding=3,bias=False)self.bn1 = norm_layer(self.inplanes)self.relu = nn.ReLU(inplace=True)# 112,112,64 -> 56,56,64self.maxpool = nn.MaxPool2d(kernel_size=3, stride=2, padding=1)# 56,56,64 -> 56,56,256self.layer1 = self._make_layer(block, 64, layers[0])# 56,56,256 -> 28,28,512self.layer2 = self._make_layer(block, 128, layers[1], stride=2,dilate=replace_stride_with_dilation[0])# 28,28,512 -> 14,14,1024self.layer3 = self._make_layer(block, 256, layers[2], stride=2,dilate=replace_stride_with_dilation[1])# 14,14,1024 -> 7,7,2048self.layer4 = self._make_layer(block, 512, layers[3], stride=2,dilate=replace_stride_with_dilation[2])# 7,7,2048 -> 2048self.avgpool = nn.AdaptiveAvgPool2d((1, 1))# 2048 -> num_classesself.fc = nn.Linear(512 * block.expansion, num_classes)for m in self.modules():if isinstance(m, nn.Conv2d):nn.init.kaiming_normal_(m.weight, mode='fan_out', nonlinearity='relu')elif isinstance(m, (nn.BatchNorm2d, nn.GroupNorm)):nn.init.constant_(m.weight, 1)nn.init.constant_(m.bias, 0)if zero_init_residual:for m in self.modules():if isinstance(m, Bottleneck):nn.init.constant_(m.bn3.weight, 0)def _make_layer(self, block, planes, blocks, stride=1, dilate=False):norm_layer = self._norm_layerdownsample = Noneprevious_dilation = self.dilationif dilate:self.dilation *= stridestride = 1if stride != 1 or self.inplanes != planes * block.expansion:downsample = nn.Sequential(conv1x1(self.inplanes, planes * block.expansion, stride),norm_layer(planes * block.expansion),)layers = []# Conv_blocklayers.append(block(self.inplanes, planes, stride, downsample, self.groups,self.base_width, previous_dilation, norm_layer))self.inplanes = planes * block.expansionfor _ in range(1, blocks):# identity_blocklayers.append(block(self.inplanes, planes, groups=self.groups,base_width=self.base_width, dilation=self.dilation,norm_layer=norm_layer))return nn.Sequential(*layers)def forward(self, x):x = self.conv1(x)x = self.bn1(x)x = self.relu(x)x = self.maxpool(x)x = self.layer1(x)x = self.layer2(x)x = self.layer3(x)x = self.layer4(x)x = self.avgpool(x)x = torch.flatten(x, 1)x = self.fc(x)return xdef freeze_backbone(self):backbone = [self.conv1, self.bn1, self.layer1, self.layer2, self.layer3, self.layer4]for module in backbone:for param in module.parameters():param.requires_grad = Falsedef Unfreeze_backbone(self):backbone = [self.conv1, self.bn1, self.layer1, self.layer2, self.layer3, self.layer4]for module in backbone:for param in module.parameters():param.requires_grad = Truedef resnet18(pretrained=False, progress=True, num_classes=1000):model = ResNet(BasicBlock, [2, 2, 2, 2])if pretrained:state_dict = load_state_dict_from_url(model_urls['resnet18'], model_dir='./model_data',progress=progress)model.load_state_dict(state_dict)if num_classes!=1000:model.fc = nn.Linear(512 * model.block.expansion, num_classes)return modeldef resnet34(pretrained=False, progress=True, num_classes=1000):model = ResNet(BasicBlock, [3, 4, 6, 3])if pretrained:state_dict = load_state_dict_from_url(model_urls['resnet34'], model_dir='./model_data',progress=progress)model.load_state_dict(state_dict)if num_classes!=1000:model.fc = nn.Linear(512 * model.block.expansion, num_classes)return modeldef resnet50(pretrained=False, progress=True, num_classes=1000):model = ResNet(Bottleneck, [3, 4, 6, 3])if pretrained:state_dict = load_state_dict_from_url(model_urls['resnet50'], model_dir='./model_data',progress=progress)model.load_state_dict(state_dict)if num_classes!=1000:model.fc = nn.Linear(512 * model.block.expansion, num_classes)return modeldef resnet101(pretrained=False, progress=True, num_classes=1000):model = ResNet(Bottleneck, [3, 4, 23, 3])if pretrained:state_dict = load_state_dict_from_url(model_urls['resnet101'], model_dir='./model_data',progress=progress)model.load_state_dict(state_dict)if num_classes!=1000:model.fc = nn.Linear(512 * model.block.expansion, num_classes)return modeldef resnet152(pretrained=False, progress=True, num_classes=1000):model = ResNet(Bottleneck, [3, 8, 36, 3])if pretrained:state_dict = load_state_dict_from_url(model_urls['resnet152'], model_dir='./model_data',progress=progress)model.load_state_dict(state_dict)if num_classes!=1000:model.fc = nn.Linear(512 * model.block.expansion, num_classes)return model
可以查看网络的结构:
#--------------------------------------------#
# 该部分代码只用于看网络结构,并非测试代码
#--------------------------------------------#
import torch
from thop import clever_format, profile
from torchsummary import summaryfrom nets import get_model_from_name
# from nets import resnet_cbam # 使用哪个引入哪个即可
if __name__ == "__main__":input_shape = [224, 224]num_classes = 3 #写自己的分类个数,如果是训练图像分割,要多分一个背景,比如猫狗两种,num_classes=2+1# backbone = "mobilenetv2"backbone = "resnet50"device = torch.device("cuda" if torch.cuda.is_available() else "cpu")model = get_model_from_name[backbone](num_classes=num_classes, pretrained=False).to(device)summary(model, (3, input_shape[0], input_shape[1]))dummy_input = torch.randn(1, 3, input_shape[0], input_shape[1]).to(device)flops, params = profile(model.to(device), (dummy_input, ), verbose=False)#--------------------------------------------------------## flops * 2是因为profile没有将卷积作为两个operations# 有些论文将卷积算乘法、加法两个operations。此时乘2# 有些论文只考虑乘法的运算次数,忽略加法。此时不乘2# 本代码选择乘2,参考YOLOX。#--------------------------------------------------------#flops = flops * 2flops, params = clever_format([flops, params], "%.3f")print('Total GFLOPS: %s' % (flops))print('Total params: %s' % (params))
结构如下:(resnet50的)
其中两个残差模块Conv Block用于改变通道数,Identity Block用于加深网络
第一个残差块4个卷积,第二个3个
(4+3+3 ) + ( 4+3+3+3) + ( 4+3+3+3+3+3+3) + ( 4+3+3)==52
再加上一上来有个7*7卷积==53个
----------------------------------------------------------------
list Layer (type) Output Shape Param #
================================================================
0 Conv2d-1 [-1, 64, 112, 112] 9,408
BatchNorm2d-2 [-1, 64, 112, 112] 128
ReLU-3 [-1, 64, 112, 112] 0
MaxPool2d-4 [-1, 64, 56, 56] 0
1 Conv2d-5 [-1, 64, 56, 56] 4,096
BatchNorm2d-6 [-1, 64, 56, 56] 128
ReLU-7 [-1, 64, 56, 56] 0
2 Conv2d-8 [-1, 64, 56, 56] 36,864
BatchNorm2d-9 [-1, 64, 56, 56] 128
ReLU-10 [-1, 64, 56, 56] 0
3 Conv2d-11 [-1, 256, 56, 56] 16,384
BatchNorm2d-12 [-1, 256, 56, 56] 512
4 Conv2d-13 [-1, 256, 56, 56] 16,384
BatchNorm2d-14 [-1, 256, 56, 56] 512
ReLU-15 [-1, 256, 56, 56] 0
Bottleneck-16 [-1, 256, 56, 56] 0
5 Conv2d-17 [-1, 64, 56, 56] 16,384
BatchNorm2d-18 [-1, 64, 56, 56] 128
ReLU-19 [-1, 64, 56, 56] 0
6 Conv2d-20 [-1, 64, 56, 56] 36,864
BatchNorm2d-21 [-1, 64, 56, 56] 128
ReLU-22 [-1, 64, 56, 56] 0
7 Conv2d-23 [-1, 256, 56, 56] 16,384
BatchNorm2d-24 [-1, 256, 56, 56] 512
ReLU-25 [-1, 256, 56, 56] 0
Bottleneck-26 [-1, 256, 56, 56] 0
8 Conv2d-27 [-1, 64, 56, 56] 16,384
BatchNorm2d-28 [-1, 64, 56, 56] 128
ReLU-29 [-1, 64, 56, 56] 0
9 Conv2d-30 [-1, 64, 56, 56] 36,864
BatchNorm2d-31 [-1, 64, 56, 56] 128
ReLU-32 [-1, 64, 56, 56] 0
10 Conv2d-33 [-1, 256, 56, 56] 16,384
BatchNorm2d-34 [-1, 256, 56, 56] 512
ReLU-35 [-1, 256, 56, 56] 0
Bottleneck-36 [-1, 256, 56, 56] 0
11 Conv2d-37 [-1, 128, 56, 56] 32,768
BatchNorm2d-38 [-1, 128, 56, 56] 256
ReLU-39 [-1, 128, 56, 56] 0
12 Conv2d-40 [-1, 128, 28, 28] 147,456
BatchNorm2d-41 [-1, 128, 28, 28] 256
ReLU-42 [-1, 128, 28, 28] 0
13 Conv2d-43 [-1, 512, 28, 28] 65,536
BatchNorm2d-44 [-1, 512, 28, 28] 1,024
14 Conv2d-45 [-1, 512, 28, 28] 131,072
BatchNorm2d-46 [-1, 512, 28, 28] 1,024
ReLU-47 [-1, 512, 28, 28] 0
Bottleneck-48 [-1, 512, 28, 28] 0
15 Conv2d-49 [-1, 128, 28, 28] 65,536
BatchNorm2d-50 [-1, 128, 28, 28] 256
ReLU-51 [-1, 128, 28, 28] 0
16 Conv2d-52 [-1, 128, 28, 28] 147,456
BatchNorm2d-53 [-1, 128, 28, 28] 256
ReLU-54 [-1, 128, 28, 28] 0
17 Conv2d-55 [-1, 512, 28, 28] 65,536
BatchNorm2d-56 [-1, 512, 28, 28] 1,024
ReLU-57 [-1, 512, 28, 28] 0
Bottleneck-58 [-1, 512, 28, 28] 0
18 Conv2d-59 [-1, 128, 28, 28] 65,536
BatchNorm2d-60 [-1, 128, 28, 28] 256
ReLU-61 [-1, 128, 28, 28] 0
19 Conv2d-62 [-1, 128, 28, 28] 147,456
BatchNorm2d-63 [-1, 128, 28, 28] 256
ReLU-64 [-1, 128, 28, 28] 0
20 Conv2d-65 [-1, 512, 28, 28] 65,536
BatchNorm2d-66 [-1, 512, 28, 28] 1,024
ReLU-67 [-1, 512, 28, 28] 0
Bottleneck-68 [-1, 512, 28, 28] 0
21 Conv2d-69 [-1, 128, 28, 28] 65,536
BatchNorm2d-70 [-1, 128, 28, 28] 256
ReLU-71 [-1, 128, 28, 28] 0
22 Conv2d-72 [-1, 128, 28, 28] 147,456
BatchNorm2d-73 [-1, 128, 28, 28] 256
ReLU-74 [-1, 128, 28, 28] 0
23 Conv2d-75 [-1, 512, 28, 28] 65,536
BatchNorm2d-76 [-1, 512, 28, 28] 1,024
ReLU-77 [-1, 512, 28, 28] 0
Bottleneck-78 [-1, 512, 28, 28] 0
24 Conv2d-79 [-1, 256, 28, 28] 131,072
BatchNorm2d-80 [-1, 256, 28, 28] 512
ReLU-81 [-1, 256, 28, 28] 0
25 Conv2d-82 [-1, 256, 14, 14] 589,824
BatchNorm2d-83 [-1, 256, 14, 14] 512
ReLU-84 [-1, 256, 14, 14] 0
26 Conv2d-85 [-1, 1024, 14, 14] 262,144
BatchNorm2d-86 [-1, 1024, 14, 14] 2,048
27 Conv2d-87 [-1, 1024, 14, 14] 524,288
BatchNorm2d-88 [-1, 1024, 14, 14] 2,048
ReLU-89 [-1, 1024, 14, 14] 0
Bottleneck-90 [-1, 1024, 14, 14] 0
28 Conv2d-91 [-1, 256, 14, 14] 262,144
BatchNorm2d-92 [-1, 256, 14, 14] 512
ReLU-93 [-1, 256, 14, 14] 0
29 Conv2d-94 [-1, 256, 14, 14] 589,824
BatchNorm2d-95 [-1, 256, 14, 14] 512
ReLU-96 [-1, 256, 14, 14] 0
30 Conv2d-97 [-1, 1024, 14, 14] 262,144
BatchNorm2d-98 [-1, 1024, 14, 14] 2,048
ReLU-99 [-1, 1024, 14, 14] 0
Bottleneck-100 [-1, 1024, 14, 14] 0
31 Conv2d-101 [-1, 256, 14, 14] 262,144
BatchNorm2d-102 [-1, 256, 14, 14] 512
ReLU-103 [-1, 256, 14, 14] 0
32 Conv2d-104 [-1, 256, 14, 14] 589,824
BatchNorm2d-105 [-1, 256, 14, 14] 512
ReLU-106 [-1, 256, 14, 14] 0
33 Conv2d-107 [-1, 1024, 14, 14] 262,144
BatchNorm2d-108 [-1, 1024, 14, 14] 2,048
ReLU-109 [-1, 1024, 14, 14] 0
Bottleneck-110 [-1, 1024, 14, 14] 0
34 Conv2d-111 [-1, 256, 14, 14] 262,144
BatchNorm2d-112 [-1, 256, 14, 14] 512
ReLU-113 [-1, 256, 14, 14] 0
35 Conv2d-114 [-1, 256, 14, 14] 589,824
BatchNorm2d-115 [-1, 256, 14, 14] 512
ReLU-116 [-1, 256, 14, 14] 0
36 Conv2d-117 [-1, 1024, 14, 14] 262,144
BatchNorm2d-118 [-1, 1024, 14, 14] 2,048
ReLU-119 [-1, 1024, 14, 14] 0
Bottleneck-120 [-1, 1024, 14, 14] 0
37 Conv2d-121 [-1, 256, 14, 14] 262,144
BatchNorm2d-122 [-1, 256, 14, 14] 512
ReLU-123 [-1, 256, 14, 14] 0
38 Conv2d-124 [-1, 256, 14, 14] 589,824
BatchNorm2d-125 [-1, 256, 14, 14] 512
ReLU-126 [-1, 256, 14, 14] 0
39 Conv2d-127 [-1, 1024, 14, 14] 262,144
BatchNorm2d-128 [-1, 1024, 14, 14] 2,048
ReLU-129 [-1, 1024, 14, 14] 0
Bottleneck-130 [-1, 1024, 14, 14] 0
40 Conv2d-131 [-1, 256, 14, 14] 262,144
BatchNorm2d-132 [-1, 256, 14, 14] 512
ReLU-133 [-1, 256, 14, 14] 0
41 Conv2d-134 [-1, 256, 14, 14] 589,824
BatchNorm2d-135 [-1, 256, 14, 14] 512
ReLU-136 [-1, 256, 14, 14] 0
42 Conv2d-137 [-1, 1024, 14, 14] 262,144
BatchNorm2d-138 [-1, 1024, 14, 14] 2,048
ReLU-139 [-1, 1024, 14, 14] 0
Bottleneck-140 [-1, 1024, 14, 14] 0
43 Conv2d-141 [-1, 512, 14, 14] 524,288
BatchNorm2d-142 [-1, 512, 14, 14] 1,024
ReLU-143 [-1, 512, 14, 14] 0
44 Conv2d-144 [-1, 512, 7, 7] 2,359,296
BatchNorm2d-145 [-1, 512, 7, 7] 1,024
ReLU-146 [-1, 512, 7, 7] 0
45 Conv2d-147 [-1, 2048, 7, 7] 1,048,576
BatchNorm2d-148 [-1, 2048, 7, 7] 4,096
46 Conv2d-149 [-1, 2048, 7, 7] 2,097,152
BatchNorm2d-150 [-1, 2048, 7, 7] 4,096
ReLU-151 [-1, 2048, 7, 7] 0
Bottleneck-152 [-1, 2048, 7, 7] 0
47 Conv2d-153 [-1, 512, 7, 7] 1,048,576
BatchNorm2d-154 [-1, 512, 7, 7] 1,024
ReLU-155 [-1, 512, 7, 7] 0
48 Conv2d-156 [-1, 512, 7, 7] 2,359,296
BatchNorm2d-157 [-1, 512, 7, 7] 1,024
ReLU-158 [-1, 512, 7, 7] 0
49 Conv2d-159 [-1, 2048, 7, 7] 1,048,576
BatchNorm2d-160 [-1, 2048, 7, 7] 4,096
ReLU-161 [-1, 2048, 7, 7] 0
Bottleneck-162 [-1, 2048, 7, 7] 0
50 Conv2d-163 [-1, 512, 7, 7] 1,048,576
BatchNorm2d-164 [-1, 512, 7, 7] 1,024
ReLU-165 [-1, 512, 7, 7] 0
51 Conv2d-166 [-1, 512, 7, 7] 2,359,296
BatchNorm2d-167 [-1, 512, 7, 7] 1,024
ReLU-168 [-1, 512, 7, 7] 0
52 Conv2d-169 [-1, 2048, 7, 7] 1,048,576
BatchNorm2d-170 [-1, 2048, 7, 7] 4,096
ReLU-171 [-1, 2048, 7, 7] 0
Bottleneck-172 [-1, 2048, 7, 7] 0
AdaptiveAvgPool2d-173 [-1, 2048, 1, 1] 0
Linear-174 [-1, 3] 6,147
================================================================
Total params: 23,514,179
Trainable params: 23,514,179
Non-trainable params: 0
----------------------------------------------------------------
Input size (MB): 0.57
Forward/backward pass size (MB): 286.55
Params size (MB): 89.70
Estimated Total Size (MB): 376.82
----------------------------------------------------------------
Total GFLOPS: 8.263G
Total params: 23.514M
可视化代码:
此段代码参考:https://blog.csdn.net/qq_34769162/article/details/115567093
# https://blog.csdn.net/qq_34769162/article/details/115567093
import numpy as npimport torch
import torchvision
from PIL import Image
from torchvision import transforms as Timport matplotlib.pyplot as plt
import pylabimport torch
import torchvisionfeature_extractor = torchvision.models.resnet50(pretrained=True)
if torch.cuda.is_available():feature_extractor.cuda()device = torch.device('cuda') if torch.cuda.is_available() else torch.device('cpu')class SaveOutput:def __init__(self):self.outputs = []def __call__(self, module, module_in, module_out):self.outputs.append(module_out)def clear(self):self.outputs = []save_output = SaveOutput()hook_handles = []for layer in feature_extractor.modules():if isinstance(layer, torch.nn.Conv2d):handle = layer.register_forward_hook(save_output)hook_handles.append(handle)from PIL import Image
from torchvision import transforms as Timage = Image.open('img/rot.jpg')
transform = T.Compose([T.Resize((224, 224)), T.ToTensor()])
X = transform(image).unsqueeze(dim=0).to(device)out = feature_extractor(X)print(len(save_output.outputs))
# 选择看的层数
# a_list = [0, 1, 6, 15, 28, 35]
a_list = [0, 1, 5, 11, 15, 24,28,43,47]# 43:512,14,14
#47:512, 7, 7
for i in a_list:print(save_output.outputs[i].cpu().detach().squeeze(0).shape)def grid_gray_image(imgs, each_row: int):'''imgs shape: batch * size (e.g., 64x32x32, 64 is the number of the gray images, and (32, 32) is the size of each gray image)'''row_num = imgs.shape[0]//each_rowfor i in range(row_num):img = imgs[i*each_row]img = (img - img.min()) / (img.max() - img.min())for j in range(1, each_row):tmp_img = imgs[i*each_row+j]tmp_img = (tmp_img - tmp_img.min()) / (tmp_img.max() - tmp_img.min())img = np.hstack((img, tmp_img))if i == 0:ans = imgelse:ans = np.vstack((ans, img))return ans# a_list = [0, 1, 5, 11, 15, 24,28,43,47]
img0 = save_output.outputs[0].cpu().detach().squeeze(0)
img0 = grid_gray_image(img0.numpy(), 8)
img1 = save_output.outputs[1].cpu().detach().squeeze(0)
img1 = grid_gray_image(img1.numpy(), 8)
img5 = save_output.outputs[5].cpu().detach().squeeze(0)
img5 = grid_gray_image(img5.numpy(), 8)
img11 = save_output.outputs[11].cpu().detach().squeeze(0)
img11 = grid_gray_image(img11.numpy(), 16)
img15 = save_output.outputs[15].cpu().detach().squeeze(0)
img15 = grid_gray_image(img15.numpy(), 16)
img24 = save_output.outputs[24].cpu().detach().squeeze(0)
img24 = grid_gray_image(img24.numpy(), 16)
img28 = save_output.outputs[28].cpu().detach().squeeze(0)
img28 = grid_gray_image(img28.numpy(), 16)
img43 = save_output.outputs[43].cpu().detach().squeeze(0)
img43 = grid_gray_image(img43.numpy(), 16)
img47 = save_output.outputs[47].cpu().detach().squeeze(0)
img47 = grid_gray_image(img47.numpy(), 16)# 64,112,112
plt.figure(figsize=(15, 15))
plt.imshow(img0, cmap='gray')#64,56,56
plt.figure(figsize=(15, 15))
plt.imshow(img1, cmap='gray')#64,56,56
plt.figure(figsize=(15, 15))
plt.imshow(img5, cmap='gray')#128,56,56
plt.figure(figsize=(30, 15))
plt.imshow(img11, cmap='gray')#128,28,28
plt.figure(figsize=(30, 15))
plt.imshow(img15, cmap='gray')#256,28,28
plt.figure(figsize=(30, 30))
plt.imshow(img24, cmap='gray')#256,14,14
plt.figure(figsize=(30, 30))
plt.imshow(img28, cmap='gray')#512,14,14
plt.figure(figsize=(45, 45))
plt.imshow(img43, cmap='gray')#512,7,7
plt.figure(figsize=(45, 45))
plt.imshow(img47, cmap='gray')pylab.show()
原图:
resnet50来说,首个卷积层的卷积核为7*7,将输入的三通道彩色图像通道增加至64,尺寸从224*224对折为112*112.
从输入图片3,224,224——>64, 112, 112
我们对首个卷积层的提取结果进行可视化:
最后一层太抽象看不清了,512,7,7
相关文章:
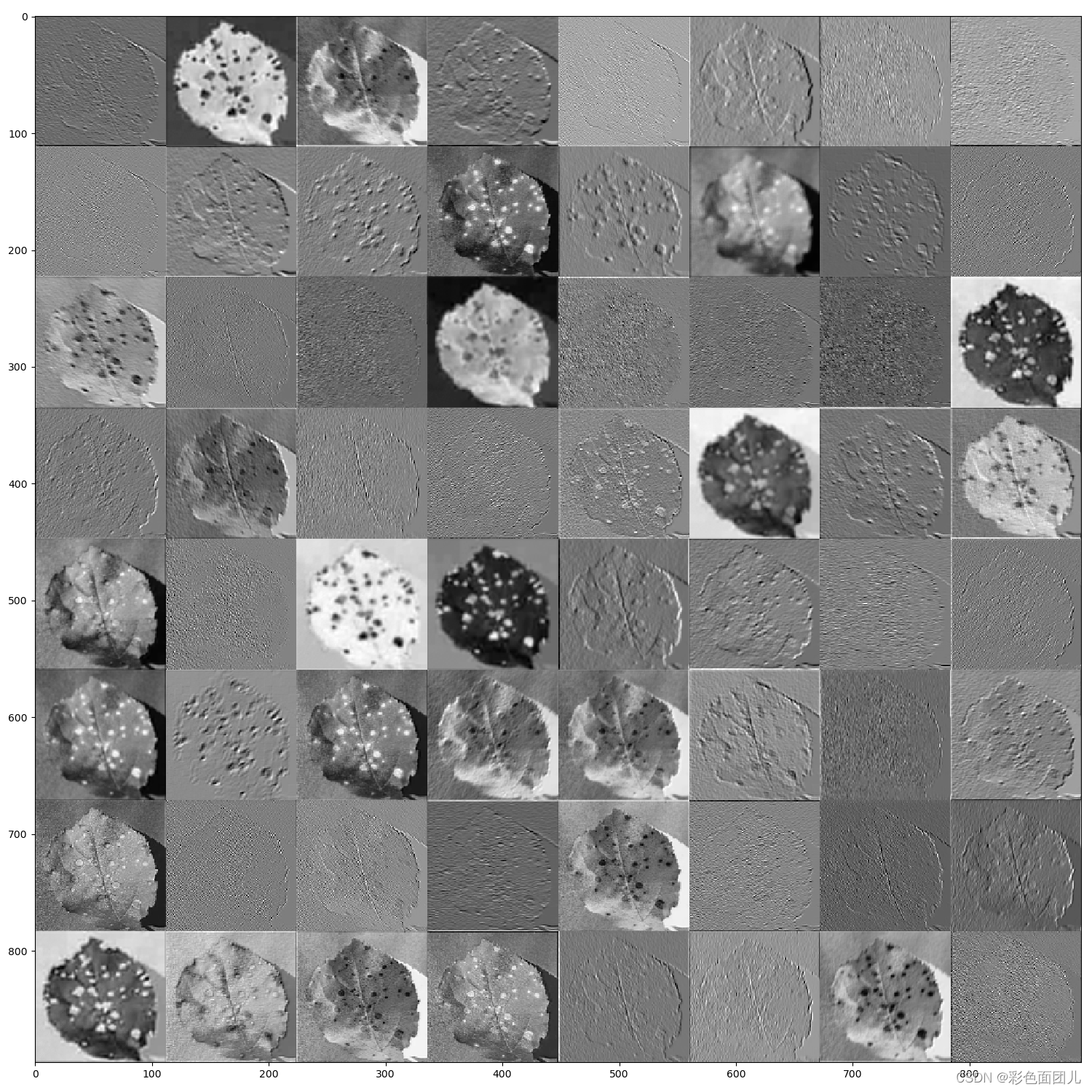
resnet网络特征提取过程可视化
我们在训练图片时,是不是要看看具体提取时的每个特征图提取的样子,找了很多,终于功夫不负有心人,找到了,通过修改的代码: resnet代码: import torch import torch.nn as nn from torchvision…...
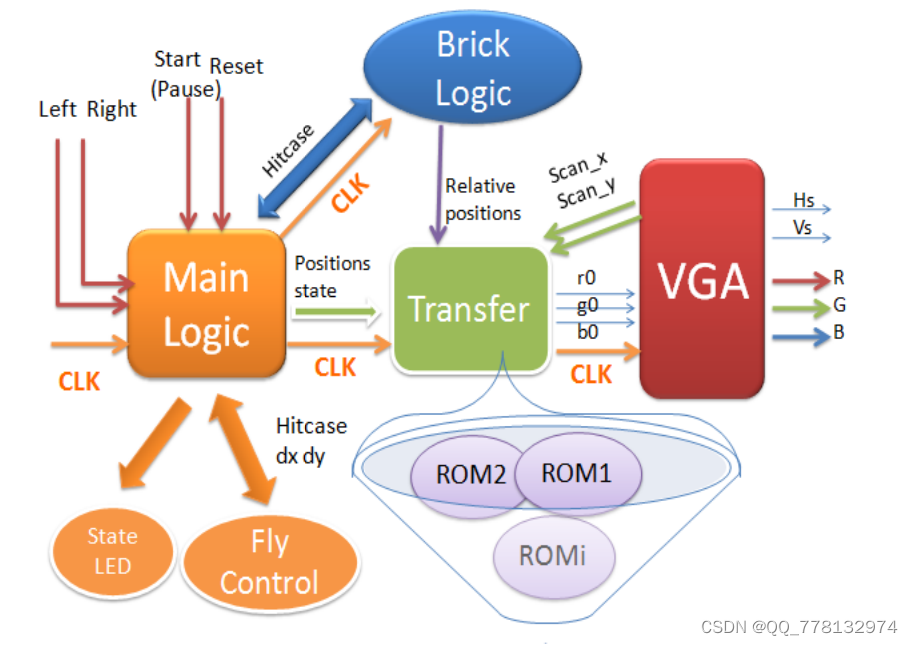
FPGA打砖块游戏设计(有上板照片)VHDL
这是一款经典打砖块游戏,我们的努力让它更精致更好玩,我们将它取名为打砖块游戏(Flyball),以下是该系统的一些基本功能: 画面简约而经典,色彩绚丽而活泼,动画流畅 玩家顺序挑战3个不同难度的级别,趣味十足 计分功能,卡通字母数字 4条生命值,由生命条显示…...
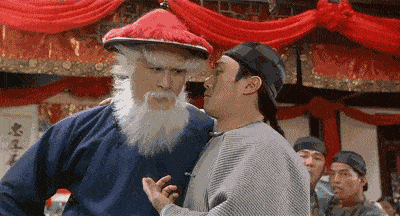
【Unity入门】3D物体
【Unity入门】3D物体 大家好,我是Lampard~~ 欢迎来到Unity入门系列博客,所学知识来自B站阿发老师~感谢 (一)物体移动旋转缩放 (1)物体移动 在上一篇文章【Unity入门】场景视图操作我们学会了在场景中创建3…...
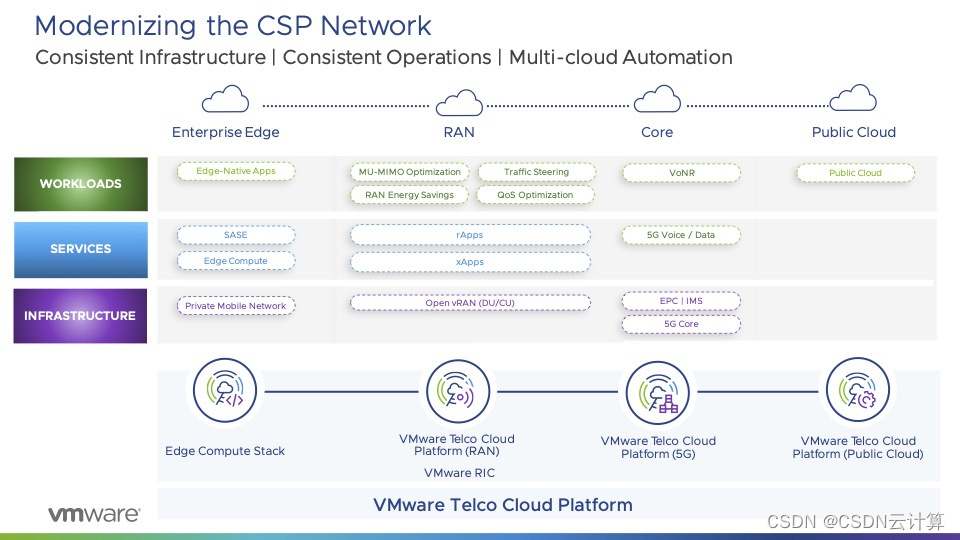
网络现代化势在必行,VMware 发布软件定义网络 SD-WAN 全新方案
出品 | CSDN云计算 作为计算存储网络基础设施三大件之一,网络一直是 IT 核心技术,并不断向前发展。 数字化转型浪潮下,各行业都在探索创新应用,而数字化创新,也是对 5G 和云边端等网络基础设施提出更高需求,…...
java学习笔记——抽象类
2.1 概述 由来 父类中的方法,被他的子类们重写,子类各自的实现都不尽相同。那么父类的方法声明和方法主体,只有声明还有意义,而方法主体则没有存在的意义了。我们把没有主体的方法称为抽象方法。java语法规定,包含抽象…...
Redis删除策略
删除策略就是针对已过期数据的处理策略。 针对过期数据要进行删除的时候都有哪些删除策略呢? 1.定时删除2.惰性删除3.定期删除1、立即删除 当key设置有过期时间,且过期时间到达时,由定时器任务立即执行对键的删除操作。 优点:节…...
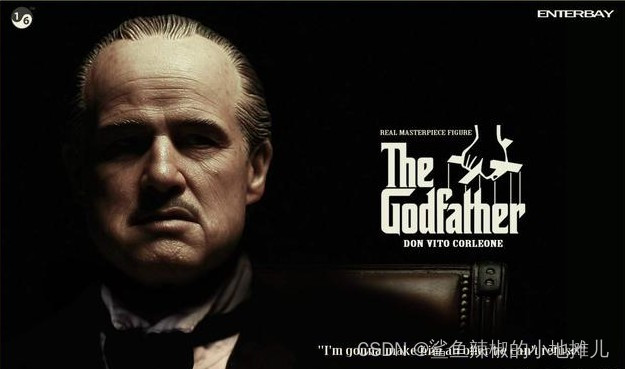
【新星计划2023】SQL SERVER (01) -- 基础知识
【新星计划2023】SQL SERVER -- 基础知识1. Introduction1.1 Official Website1.2 Conn Tool2. 基础命令2.1 建库建表2.2 Alter2.3 Drop2.3 Big Data -- Postgres3.Awakening1. Introduction 1.1 Official Website 官方文档(小技巧) Officail Website: …...
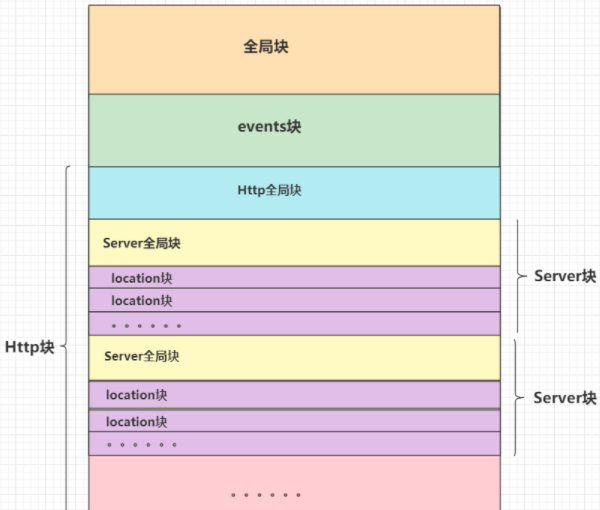
nginx配置详解
一.nginx常用命令1.Windows(1).查看nginx的版本号nginx -v(2).启动nginxstart nginx(3).快速停止或关闭nginxnginx -s stop(4).正常停止或关闭nginxnginx -s quit(5).配置文件nginx.conf修改重装载命令nginx -s reload2.Linux(1).进入 nginx 目录中cd /usr/local/nginx/sbin(2)…...
关于Java中堆和栈的学习
文章目录1.概述1.1 堆1.2 栈2.堆内存2.1 什么是堆内存?2.2堆内存的特点是什么?2.3new对象在堆中如何分配?3.栈内存3.1什么是栈内存?3.2栈内存的特点3.3栈内存的内存分配机制3.4数据共享4.栈与堆的区别4.1差异4.2相同5. 面试题: java堆和栈的区别**申请方式****申请后系统的…...
ORBSLAM3 --- 闭环及地图融合线程
目录 1.闭环及地图合并线程的目的和意义 2.闭环及地图合并流程 3.ORBSLAM3中的闭环与地图融合线程解...
libvirt零知识学习6 —— libvirt源码编译安装(4)
接前一篇文章libvirt零知识学习5 —— libvirt源码编译安装(3) 上一篇文章中解决了YAJL包的编译时依赖问题。但是在解决后再次执行meson build时又遇到了新的错误“ERROR: Program rst2html5 rst2html5.py rst2html5-3 not found or not executable”。本…...
数据仓库相关面试题
1.请介绍一下星型模型和雪花模型的区别及适用场景。 星型模型和雪花模型是数据仓库中常见的两种数据建模方式。 星型模型是由一个中心事实表和多个与之相关的维度表构成的,维度表通常只有一层,每个维度表只关联一个事实表。在星型模型中,事实…...
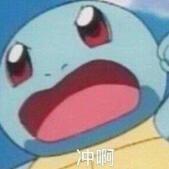
2023年PMP考试前两个月开始备考时间足够吗?
够了,PMP真的不难,目前的考试都只有选择题,往后可能会增加别的题型, PMP新版大纲加入了ACP敏捷管理的内容,而且还不少,敏捷混合题型占到了 50%,2023年8月将启用第七版《PMBOK》,大家…...

56 | fstab开机挂载
1 fstab的参数解析 【file system】【mount point】【type】【options】【dump】【pass】 其中: file systems:要挂载的分区或存储设备。 mount point:file systems 的挂载位置。 type:要挂载设备或是分区的文件系统类型&…...
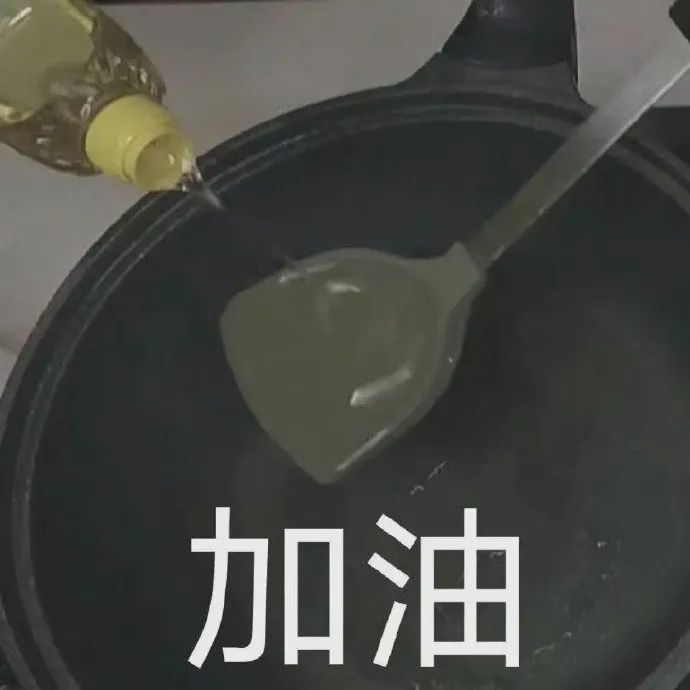
看齐iOS砍掉祖传功能,Android 16G内存也危险了
手机内存发展是真的迅速,12GB 没保持几年现在又朝着 16GB 普及。 相比 iOS 的墓碑机制,Android 后台就主打一个真实,只是可惜 APP 不那么老实。 如果你较早接触 Android 机,各种系统管理、优化 APP 的一键加速、清理应该还历历在…...
LeetCode 1012. Numbers With Repeated Digits【数位DP,数学】困难
本文属于「征服LeetCode」系列文章之一,这一系列正式开始于2021/08/12。由于LeetCode上部分题目有锁,本系列将至少持续到刷完所有无锁题之日为止;由于LeetCode还在不断地创建新题,本系列的终止日期可能是永远。在这一系列刷题文章…...
信息系统项目管理师 第4章 信息系统管理
1.管理方法 1.管理基础 1.层次结构 信息系统是对信息进行采集、处理、存储、管理和检索,形成组织中的信息流动和处理,必要时能向有关人员提供有用信息的系统。 信息系统之上是管理,它监督系统的设计和结构,并监控其整体性能。 …...
JVM系统优化实践(11):GC如何搞垮线上系统
您好,我是湘王,这是我的CSDN博客,欢迎您来,欢迎您再来~看了那么多G1 GC的传说,再来看看怎么预防GC把工程师精心设计的系统给搞垮。在JVM的运行过程中,既有创建对象,又有GC࿰…...
统计软件与数据分析—Lesson2
jupyter Note环境配置,安装及使用以及python数据的读取操作统计软件与数据分析—Lesson21.Jupyter Note环境配置,安装及使用1.1 Jupyter Note 基本操作1.2 Notebook中的Magic开关1.2.1 Magic开关总览1.2.2 Line Magic 全局1.2.3 Cell Magic 当前cell1.3 …...
ISO体系认证全方位解析让!
ISO体系认证全方位解析让! 常常有人问小编, 某某体系是什么意思? 某某证书的有效期是多久? 新版标准的转换要求有哪些? 小编尽量一一解答, 但难免会错过部分朋友的问题。 为了更全面地解决大家关于认证的疑…...
Linux简单的操作
ls ls 查看当前目录 ll 查看详细内容 ls -a 查看所有的内容 ls --help 查看方法文档 pwd pwd 查看当前路径 cd cd 转路径 cd .. 转上一级路径 cd 名 转换路径 …...
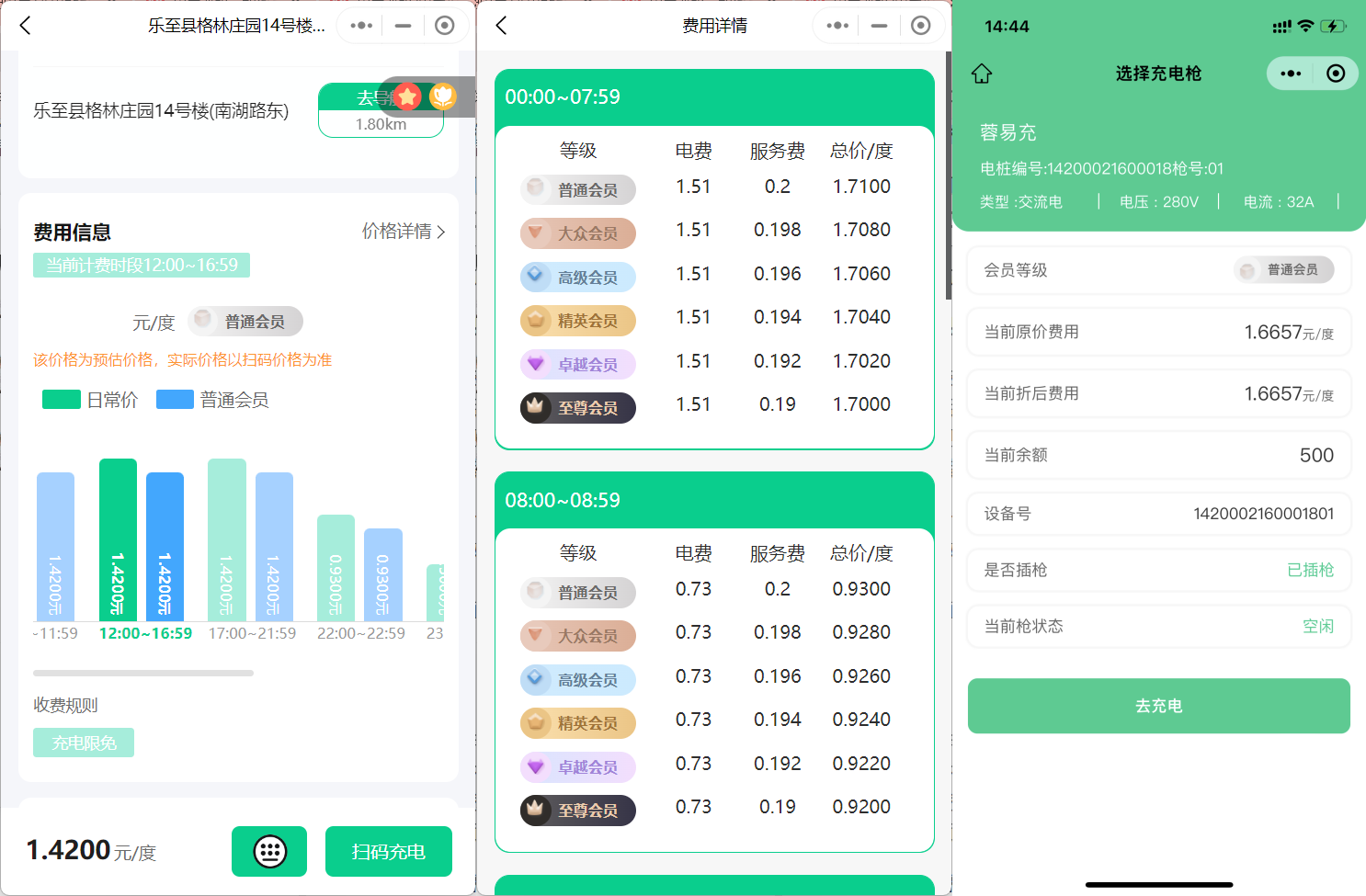
新能源汽车智慧充电桩管理方案:新能源充电桩散热问题及消防安全监管方案
随着新能源汽车的快速普及,充电桩作为核心配套设施,其安全性与可靠性备受关注。然而,在高温、高负荷运行环境下,充电桩的散热问题与消防安全隐患日益凸显,成为制约行业发展的关键瓶颈。 如何通过智慧化管理手段优化散…...
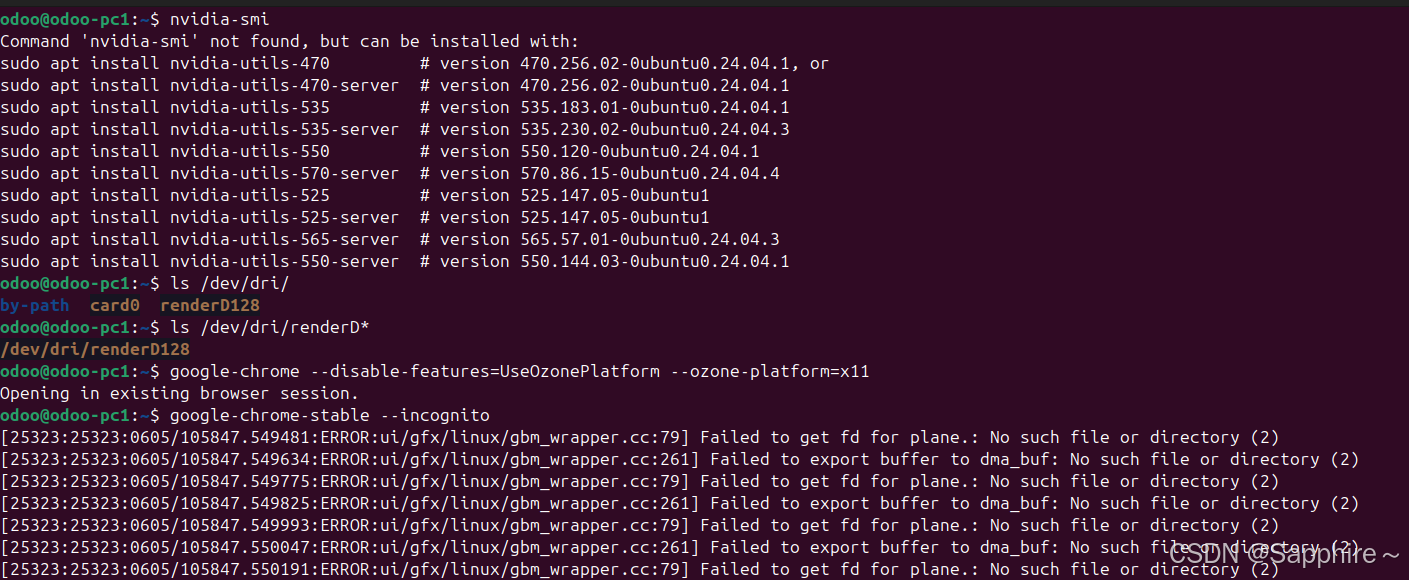
Linux-07 ubuntu 的 chrome 启动不了
文章目录 问题原因解决步骤一、卸载旧版chrome二、重新安装chorme三、启动不了,报错如下四、启动不了,解决如下 总结 问题原因 在应用中可以看到chrome,但是打不开(说明:原来的ubuntu系统出问题了,这个是备用的硬盘&a…...
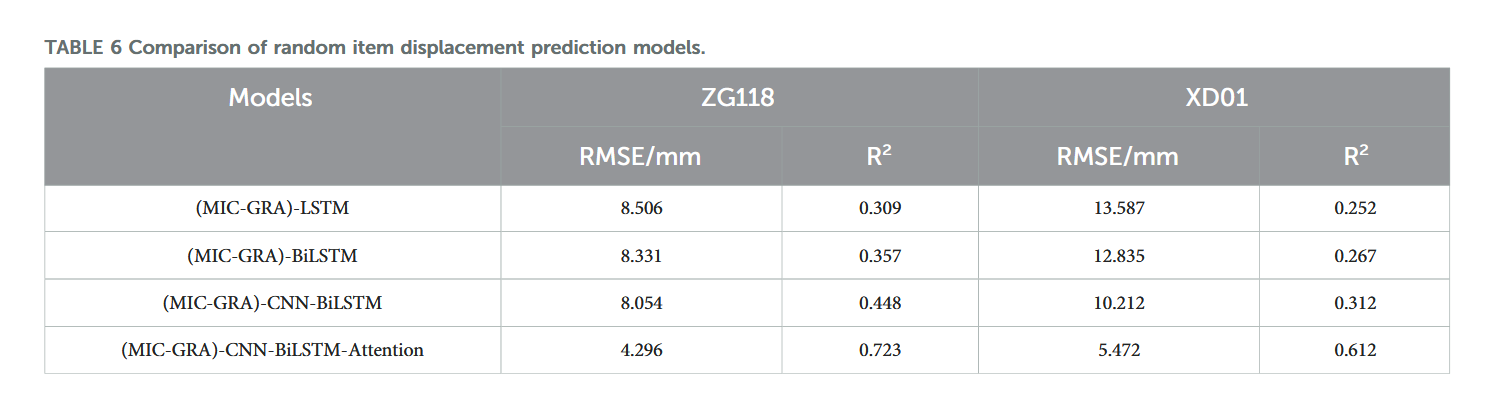
【论文阅读28】-CNN-BiLSTM-Attention-(2024)
本文把滑坡位移序列拆开、筛优质因子,再用 CNN-BiLSTM-Attention 来动态预测每个子序列,最后重构出总位移,预测效果超越传统模型。 文章目录 1 引言2 方法2.1 位移时间序列加性模型2.2 变分模态分解 (VMD) 具体步骤2.3.1 样本熵(S…...
laravel8+vue3.0+element-plus搭建方法
创建 laravel8 项目 composer create-project --prefer-dist laravel/laravel laravel8 8.* 安装 laravel/ui composer require laravel/ui 修改 package.json 文件 "devDependencies": {"vue/compiler-sfc": "^3.0.7","axios": …...
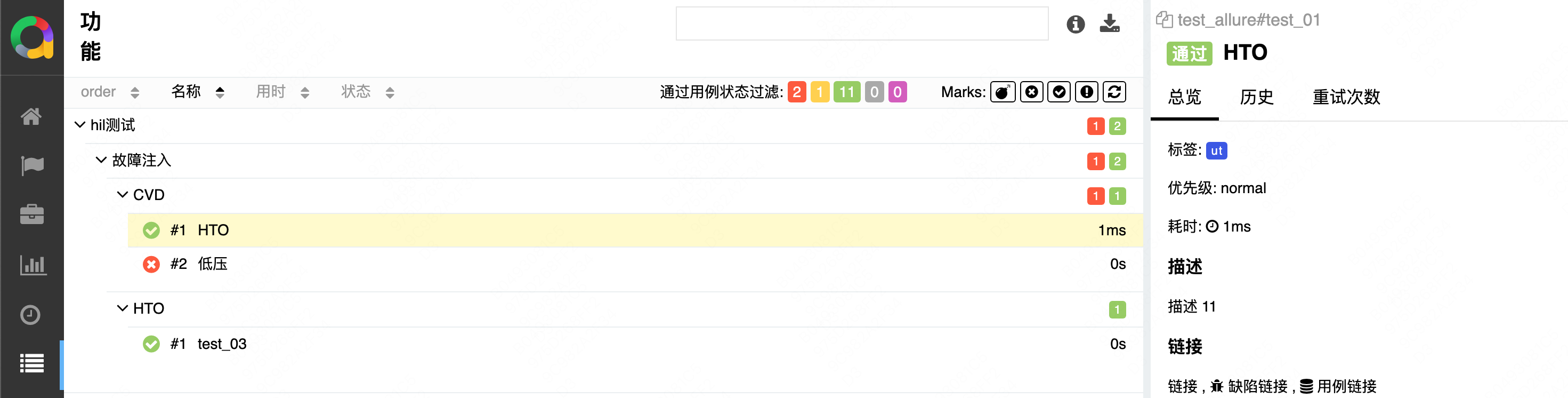
初学 pytest 记录
安装 pip install pytest用例可以是函数也可以是类中的方法 def test_func():print()class TestAdd: # def __init__(self): 在 pytest 中不可以使用__init__方法 # self.cc 12345 pytest.mark.api def test_str(self):res add(1, 2)assert res 12def test_int(self):r…...
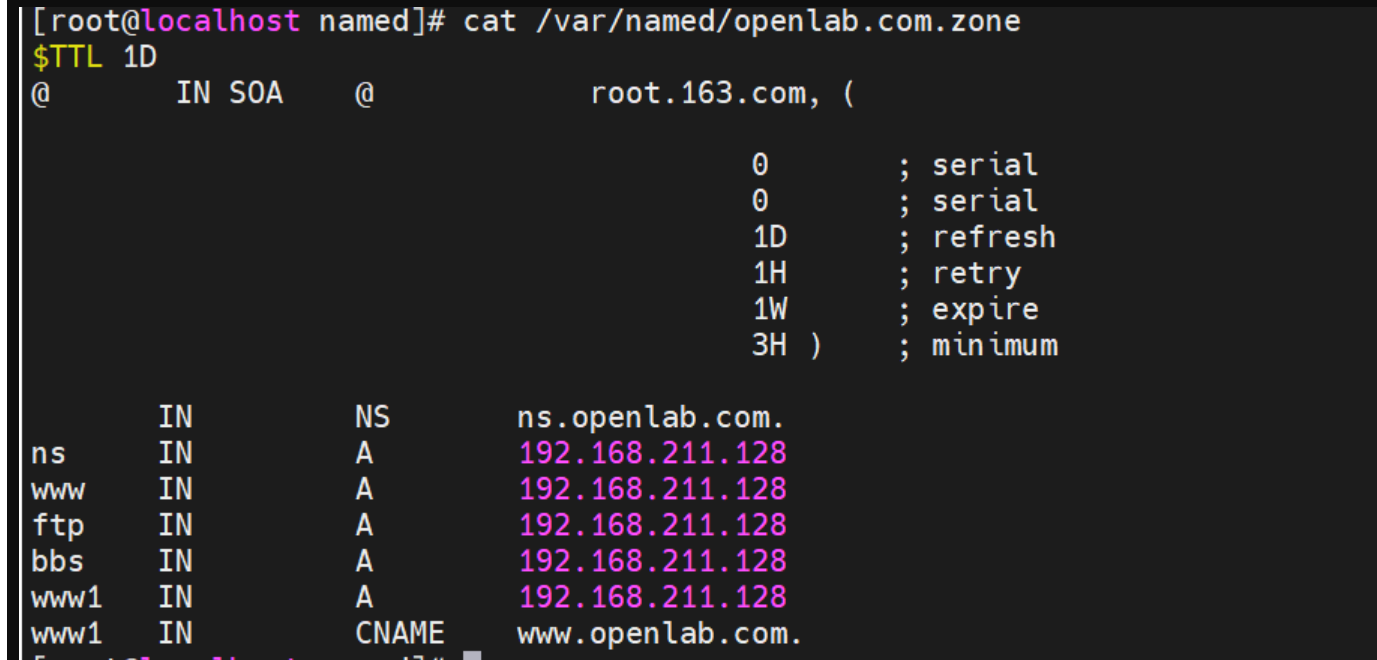
搭建DNS域名解析服务器(正向解析资源文件)
正向解析资源文件 1)准备工作 服务端及客户端都关闭安全软件 [rootlocalhost ~]# systemctl stop firewalld [rootlocalhost ~]# setenforce 0 2)服务端安装软件:bind 1.配置yum源 [rootlocalhost ~]# cat /etc/yum.repos.d/base.repo [Base…...
NPOI Excel用OLE对象的形式插入文件附件以及插入图片
static void Main(string[] args) {XlsWithObjData();Console.WriteLine("输出完成"); }static void XlsWithObjData() {// 创建工作簿和单元格,只有HSSFWorkbook,XSSFWorkbook不可以HSSFWorkbook workbook new HSSFWorkbook();HSSFSheet sheet (HSSFSheet)workboo…...
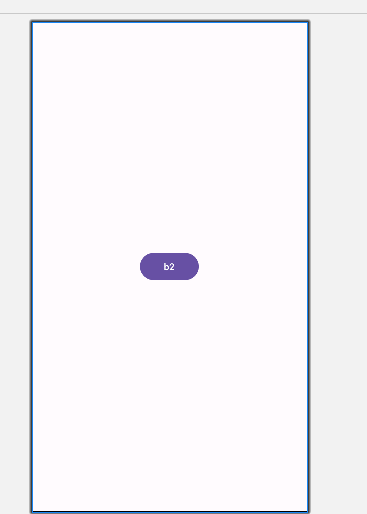
android RelativeLayout布局
<?xml version"1.0" encoding"utf-8"?> <RelativeLayout xmlns:android"http://schemas.android.com/apk/res/android"android:layout_width"match_parent"android:layout_height"match_parent"android:gravity&…...
【WebSocket】SpringBoot项目中使用WebSocket
1. 导入坐标 如果springboot父工程没有加入websocket的起步依赖,添加它的坐标的时候需要带上版本号。 <dependency><groupId>org.springframework.boot</groupId><artifactId>spring-boot-starter-websocket</artifactId> </dep…...