深度学习之目标检测篇——残差网络与FPN结合
- 特征金字塔
- 多尺度融合
- 特征金字塔的网络原理
- 这里是基于resnet网络与Fpn做的结合,主要把resnet中的特征层利用FPN的思想一起结合,实现resnet_fpn。增强目标检测backone的有效性。
- 代码实现如下:
import torch
from torch import Tensor
from collections import OrderedDict
import torch.nn.functional as F
from torch import nn
from torch.jit.annotations import Tuple, List, Dictclass Bottleneck(nn.Module):expansion = 4def __init__(self, in_channel, out_channel, stride=1, downsample=None, norm_layer=None):super(Bottleneck, self).__init__()if norm_layer is None:norm_layer = nn.BatchNorm2dself.conv1 = nn.Conv2d(in_channels=in_channel, out_channels=out_channel,kernel_size=(1,1), stride=(1,1), bias=False) # squeeze channelsself.bn1 = norm_layer(out_channel)# -----------------------------------------self.conv2 = nn.Conv2d(in_channels=out_channel, out_channels=out_channel,kernel_size=(3,3), stride=(stride,stride), bias=False, padding=(1,1))self.bn2 = norm_layer(out_channel)# -----------------------------------------self.conv3 = nn.Conv2d(in_channels=out_channel, out_channels=out_channel * self.expansion,kernel_size=(1,1), stride=(1,1), bias=False) # unsqueeze channelsself.bn3 = norm_layer(out_channel * self.expansion)self.relu = nn.ReLU(inplace=True)self.downsample = downsampledef forward(self, x):identity = xif self.downsample is not None:identity = self.downsample(x)out = self.conv1(x)out = self.bn1(out)out = self.relu(out)out = self.conv2(out)out = self.bn2(out)out = self.relu(out)out = self.conv3(out)out = self.bn3(out)out += identityout = self.relu(out)return outclass ResNet(nn.Module):def __init__(self, block, blocks_num, num_classes=1000, include_top=True, norm_layer=None):''':param block:块:param blocks_num:块数:param num_classes: 分类数:param include_top::param norm_layer: BN'''super(ResNet, self).__init__()if norm_layer is None:norm_layer = nn.BatchNorm2dself._norm_layer = norm_layerself.include_top = include_topself.in_channel = 64self.conv1 = nn.Conv2d(in_channels=3, out_channels=self.in_channel, kernel_size=(7,7), stride=(2,2),padding=(3,3), bias=False)self.bn1 = norm_layer(self.in_channel)self.relu = nn.ReLU(inplace=True)self.maxpool = nn.MaxPool2d(kernel_size=3, stride=2, padding=1)self.layer1 = self._make_layer(block, 64, blocks_num[0])self.layer2 = self._make_layer(block, 128, blocks_num[1], stride=2)self.layer3 = self._make_layer(block, 256, blocks_num[2], stride=2)self.layer4 = self._make_layer(block, 512, blocks_num[3], stride=2)if self.include_top:self.avgpool = nn.AdaptiveAvgPool2d((1, 1)) # output size = (1, 1)self.fc = nn.Linear(512 * block.expansion, num_classes)'''初始化'''for m in self.modules():if isinstance(m, nn.Conv2d):nn.init.kaiming_normal_(m.weight, mode='fan_out', nonlinearity='relu')def _make_layer(self, block, channel, block_num, stride=1):norm_layer = self._norm_layerdownsample = Noneif stride != 1 or self.in_channel != channel * block.expansion:downsample = nn.Sequential(nn.Conv2d(self.in_channel, channel * block.expansion, kernel_size=(1,1), stride=(stride,stride), bias=False),norm_layer(channel * block.expansion))layers = []layers.append(block(self.in_channel, channel, downsample=downsample,stride=stride, norm_layer=norm_layer))self.in_channel = channel * block.expansionfor _ in range(1, block_num):layers.append(block(self.in_channel, channel, norm_layer=norm_layer))return nn.Sequential(*layers)def forward(self, x):x = self.conv1(x)x = self.bn1(x)x = self.relu(x)x = self.maxpool(x)x = self.layer1(x)x = self.layer2(x)x = self.layer3(x)x = self.layer4(x)if self.include_top:x = self.avgpool(x)x = torch.flatten(x, 1)x = self.fc(x)return xclass IntermediateLayerGetter(nn.ModuleDict):"""Module wrapper that returns intermediate layers from a modelsIt has a strong assumption that the modules have been registeredinto the models in the same order as they are used.This means that one should **not** reuse the same nn.Moduletwice in the forward if you want this to work.Additionally, it is only able to query submodules that are directlyassigned to the models. So if `models` is passed, `models.feature1` canbe returned, but not `models.feature1.layer2`.Arguments:model (nn.Module): models on which we will extract the featuresreturn_layers (Dict[name, new_name]): a dict containing the namesof the modules for which the activations will be returned asthe key of the dict, and the value of the dict is the nameof the returned activation (which the user can specify)."""__annotations__ = {"return_layers": Dict[str, str],}def __init__(self, model, return_layers):if not set(return_layers).issubset([name for name, _ in model.named_children()]):raise ValueError("return_layers are not present in models")# {'layer1': '0', 'layer2': '1', 'layer3': '2', 'layer4': '3'}orig_return_layers = return_layersreturn_layers = {k: v for k, v in return_layers.items()}layers = OrderedDict()# 遍历模型子模块按顺序存入有序字典# 只保存layer4及其之前的结构,舍去之后不用的结构for name, module in model.named_children():layers[name] = moduleif name in return_layers:del return_layers[name]if not return_layers:breaksuper(IntermediateLayerGetter, self).__init__(layers)self.return_layers = orig_return_layersdef forward(self, x):out = OrderedDict()# 依次遍历模型的所有子模块,并进行正向传播,# 收集layer1, layer2, layer3, layer4的输出for name, module in self.named_children():x = module(x)if name in self.return_layers:out_name = self.return_layers[name]out[out_name] = xreturn outclass FeaturePyramidNetwork(nn.Module):"""Module that adds a FPN from on top of a set of feature maps. This is based on`"Feature Pyramid Network for Object Detection" <https://arxiv.org/abs/1612.03144>`_.The feature maps are currently supposed to be in increasing depthorder.The input to the models is expected to be an OrderedDict[Tensor], containingthe feature maps on top of which the FPN will be added.Arguments:in_channels_list (list[int]): number of channels for each feature map thatis passed to the moduleout_channels (int): number of channels of the FPN representationextra_blocks (ExtraFPNBlock or None): if provided, extra operations willbe performed. It is expected to take the fpn features, the originalfeatures and the names of the original features as input, and returnsa new list of feature maps and their corresponding names"""def __init__(self, in_channels_list, out_channels, extra_blocks=None):super(FeaturePyramidNetwork, self).__init__()# 用来调整resnet特征矩阵(layer1,2,3,4)的channel(kernel_size=1)self.inner_blocks = nn.ModuleList()# 对调整后的特征矩阵使用3x3的卷积核来得到对应的预测特征矩阵self.layer_blocks = nn.ModuleList()for in_channels in in_channels_list:if in_channels == 0:continueinner_block_module = nn.Conv2d(in_channels, out_channels, (1,1))layer_block_module = nn.Conv2d(out_channels, out_channels, (3,3), padding=(1,1))self.inner_blocks.append(inner_block_module)self.layer_blocks.append(layer_block_module)# initialize parameters now to avoid modifying the initialization of top_blocksfor m in self.children():if isinstance(m, nn.Conv2d):nn.init.kaiming_uniform_(m.weight, a=1)nn.init.constant_(m.bias, 0)self.extra_blocks = extra_blocksdef get_result_from_inner_blocks(self, x, idx):# type: (Tensor, int) -> Tensor"""This is equivalent to self.inner_blocks[idx](x),but torchscript doesn't support this yet"""num_blocks = len(self.inner_blocks)if idx < 0:idx += num_blocksi = 0out = xfor module in self.inner_blocks:if i == idx:out = module(x)i += 1return outdef get_result_from_layer_blocks(self, x, idx):# type: (Tensor, int) -> Tensor"""This is equivalent to self.layer_blocks[idx](x),but torchscript doesn't support this yet"""num_blocks = len(self.layer_blocks)if idx < 0:idx += num_blocksi = 0out = xfor module in self.layer_blocks:if i == idx:out = module(x)i += 1return outdef forward(self, x):# type: (Dict[str, Tensor]) -> Dict[str, Tensor]"""Computes the FPN for a set of feature maps.Arguments:x (OrderedDict[Tensor]): feature maps for each feature level.Returns:results (OrderedDict[Tensor]): feature maps after FPN layers.They are ordered from highest resolution first."""# unpack OrderedDict into two lists for easier handlingnames = list(x.keys())x = list(x.values())# 将resnet layer4的channel调整到指定的out_channels# last_inner = self.inner_blocks[-1](x[-1])last_inner = self.get_result_from_inner_blocks(x[-1], -1)# result中保存着每个预测特征层results = []# 将layer4调整channel后的特征矩阵,通过3x3卷积后得到对应的预测特征矩阵# results.append(self.layer_blocks[-1](last_inner))results.append(self.get_result_from_layer_blocks(last_inner, -1))# 倒序遍历resenet输出特征层,以及对应inner_block和layer_block# layer3 -> layer2 -> layer1 (layer4已经处理过了)# for feature, inner_block, layer_block in zip(# x[:-1][::-1], self.inner_blocks[:-1][::-1], self.layer_blocks[:-1][::-1]# ):# if not inner_block:# continue# inner_lateral = inner_block(feature)# feat_shape = inner_lateral.shape[-2:]# inner_top_down = F.interpolate(last_inner, size=feat_shape, mode="nearest")# last_inner = inner_lateral + inner_top_down# results.insert(0, layer_block(last_inner))for idx in range(len(x) - 2, -1, -1):inner_lateral = self.get_result_from_inner_blocks(x[idx], idx)feat_shape = inner_lateral.shape[-2:]inner_top_down = F.interpolate(last_inner, size=feat_shape, mode="nearest")last_inner = inner_lateral + inner_top_downresults.insert(0, self.get_result_from_layer_blocks(last_inner, idx))# 在layer4对应的预测特征层基础上生成预测特征矩阵5if self.extra_blocks is not None:results, names = self.extra_blocks(results, names)# make it back an OrderedDictout = OrderedDict([(k, v) for k, v in zip(names, results)])return outclass LastLevelMaxPool(torch.nn.Module):"""Applies a max_pool2d on top of the last feature map"""def forward(self, x, names):# type: (List[Tensor], List[str]) -> Tuple[List[Tensor], List[str]]names.append("pool")x.append(F.max_pool2d(x[-1], 1, 2, 0))return x, namesclass BackboneWithFPN(nn.Module):"""Adds a FPN on top of a models.Internally, it uses torchvision.models._utils.IntermediateLayerGetter toextract a submodel that returns the feature maps specified in return_layers.The same limitations of IntermediatLayerGetter apply here.Arguments:backbone (nn.Module)return_layers (Dict[name, new_name]): a dict containing the namesof the modules for which the activations will be returned asthe key of the dict, and the value of the dict is the nameof the returned activation (which the user can specify).in_channels_list (List[int]): number of channels for each feature mapthat is returned, in the order they are present in the OrderedDictout_channels (int): number of channels in the FPN.Attributes:out_channels (int): the number of channels in the FPN"""def __init__(self, backbone, return_layers, in_channels_list, out_channels):''':param backbone: 特征层:param return_layers: 返回的层数:param in_channels_list: 输入通道数:param out_channels: 输出通道数'''super(BackboneWithFPN, self).__init__()'返回有序字典模型'self.body = IntermediateLayerGetter(backbone, return_layers=return_layers)self.fpn = FeaturePyramidNetwork(in_channels_list=in_channels_list,out_channels=out_channels,extra_blocks=LastLevelMaxPool(),)# super(BackboneWithFPN, self).__init__(OrderedDict(# [("body", body), ("fpn", fpn)]))self.out_channels = out_channelsdef forward(self, x):x = self.body(x)x = self.fpn(x)return xdef resnet50_fpn_backbone():# FrozenBatchNorm2d的功能与BatchNorm2d类似,但参数无法更新# norm_layer=misc.FrozenBatchNorm2dresnet_backbone = ResNet(Bottleneck, [3, 4, 6, 3],include_top=False)# freeze layers# 冻结layer1及其之前的所有底层权重(基础通用特征)for name, parameter in resnet_backbone.named_parameters():if 'layer2' not in name and 'layer3' not in name and 'layer4' not in name:'''冻结权重,不参与训练'''parameter.requires_grad_(False)# 字典名字return_layers = {'layer1': '0', 'layer2': '1', 'layer3': '2', 'layer4': '3'}# in_channel 为layer4的输出特征矩阵channel = 2048in_channels_stage2 = resnet_backbone.in_channel // 8in_channels_list = [in_channels_stage2, # layer1 out_channel=256in_channels_stage2 * 2, # layer2 out_channel=512in_channels_stage2 * 4, # layer3 out_channel=1024in_channels_stage2 * 8, # layer4 out_channel=2048]out_channels = 256return BackboneWithFPN(resnet_backbone, return_layers, in_channels_list, out_channels)if __name__ == '__main__':net = resnet50_fpn_backbone()x = torch.randn(1,3,224,224)for key,value in net(x).items():print(key,value.shape)
- 测试结果
相关文章:

深度学习之目标检测篇——残差网络与FPN结合
特征金字塔多尺度融合特征金字塔的网络原理 这里是基于resnet网络与Fpn做的结合,主要把resnet中的特征层利用FPN的思想一起结合,实现resnet_fpn。增强目标检测backone的有效性。代码实现如下: import torch from torch import Tensor from c…...
2024-2030全球及中国埋线针行业研究及十五五规划分析报告
2023年全球埋线针市场规模大约为0.73亿美元,预计2030年将达到1.37亿美元,2024-2030期间年复合增长率(CAGR)为9.5%。未来几年,本行业具有很大不确定性,本文的2024-2030年的预测数据是基于过去几年的历史发展…...
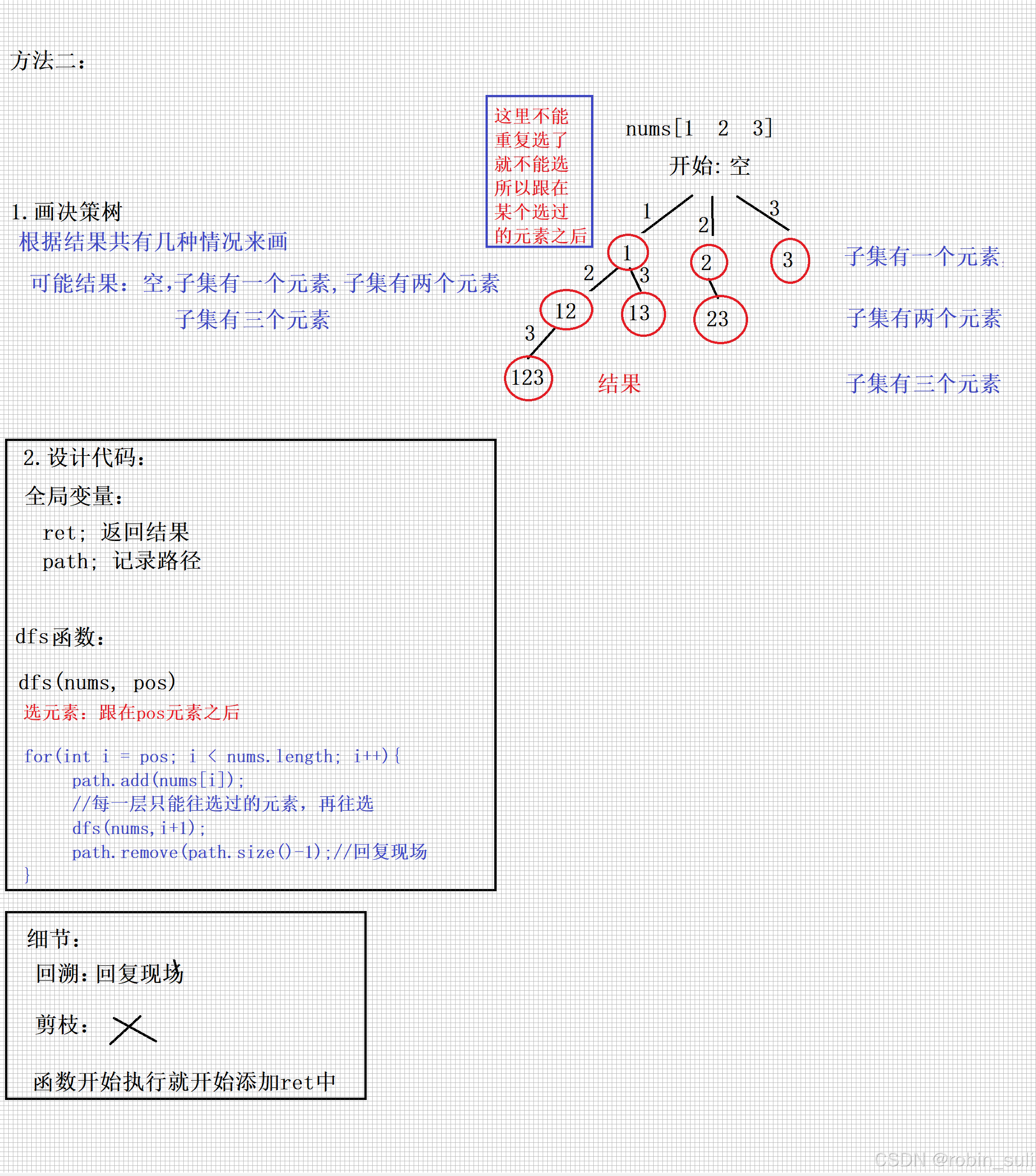
穷举vs暴搜vs深搜vs回溯vs剪枝专题一>子集
题目: 两个方法本质就是决策树的画法不同 方法一解析: 代码: class Solution {private List<List<Integer>> ret;//返回结果private List<Integer> path;//记录路径,注意返回现场public List<List<Int…...
MES系统工作流的单元测试方案
MES系统工作流的单元测试方案 在基于Java实现的MES系统中,若算子组成工作流并通过JSON传递数据,后端解析JSON后执行业务逻辑的流程,单元测试的核心是确保以下内容的正确性: 算子功能的正确性(每个算子单独的逻辑&…...
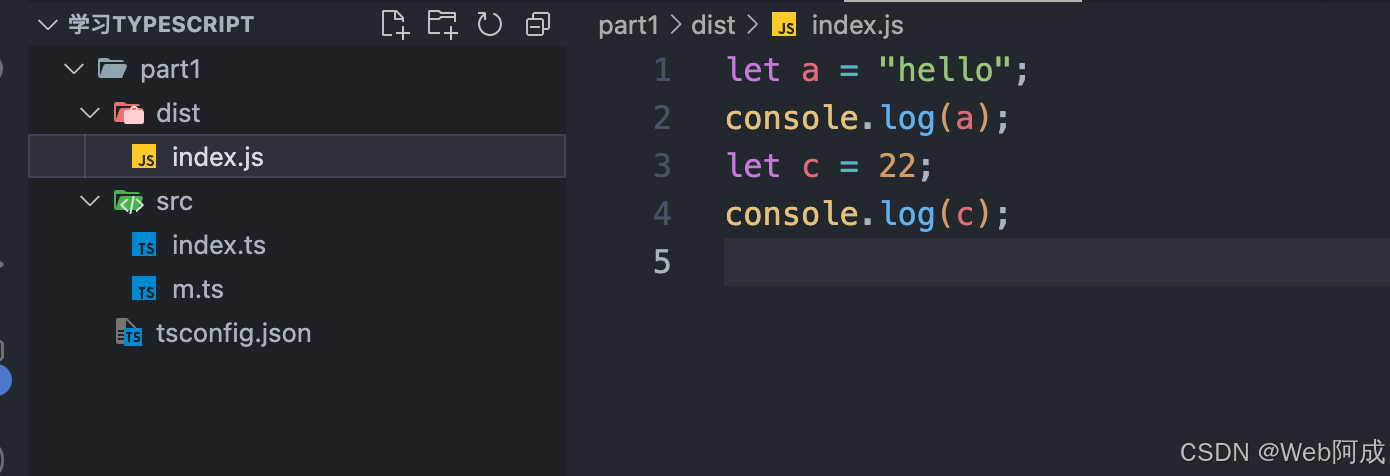
2.学习TypeScript 编译选项配置
自动编译 我们可以使用 tsc ...../.ts -w 命令进行ts文件的自动编译 执行后 编译会持续侦听 自动编译 这种方式只能侦听一个文件 对做项目肯定是不现实的,为了解决这个问题,我们需要添加一个tsconfig.json文件,写入一个基础对象 再有tsconfi…...

计算机网络之王道考研读书笔记-2
第 2 章 物理层 2.1 通信基础 2.1.1 基本概念 1.数据、信号与码元 通信的目的是传输信息。数据是指传送信息的实体。信号则是数据的电气或电磁表现,是数据在传输过程中的存在形式。码元是数字通信中数字信号的计量单位,这个时长内的信号称为 k 进制码…...
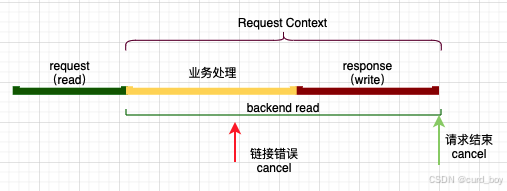
【BUG】记一次context canceled的报错
文章目录 案例分析gorm源码解读gin context 生命周期context什么时候cancel的什么时候context会被动cancel掉呢? 野生协程如何处理 案例分析 报错信息 {"L":"ERROR","T":"2024-12-17T11:11:33.0050800","file"…...
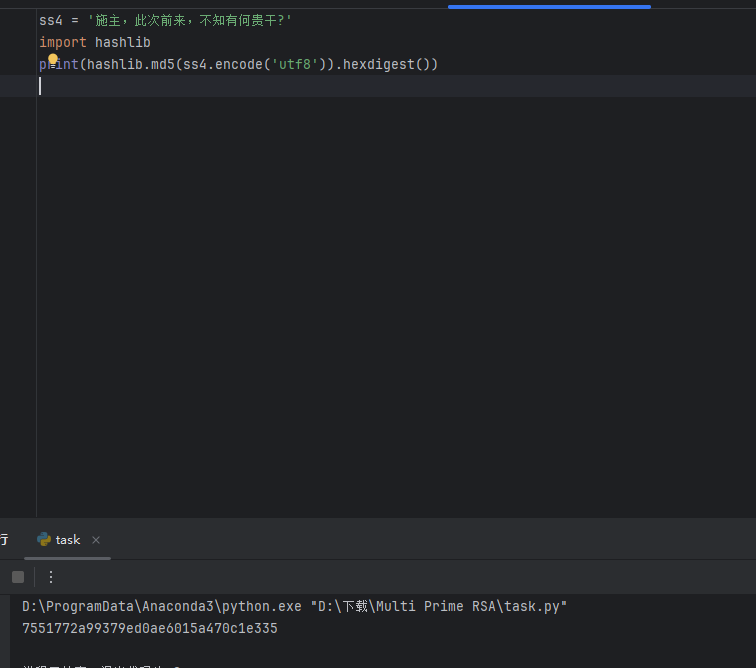
[SWPUCTF 2022 新生赛]善哉善哉
右击查看属性 然后放在010查看一下 摩斯密码解码 用佛曰解码 用md5加密看一下 最后一步md5,没有说明编码,尝试utf8和gbk ss4 施主,此次前来,不知有何贵干? import hashlib print(hashlib.md5(ss4.encode(utf8)).hexdigest())f…...
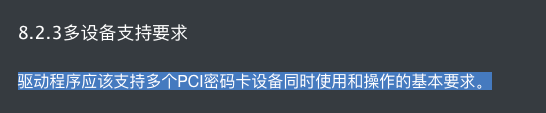
《PCI密码卡技术规范》题目
单选1 在《PCI密码卡技术规范》中,下列哪项不属于PCI密码卡的功能()。 A.密码运算功能 B.密钥管理功能 C.物理随机数产生功能 D.随主计算机可信检测功能 正确答案:D. <font style"color:#DF2A3F;">解析&…...
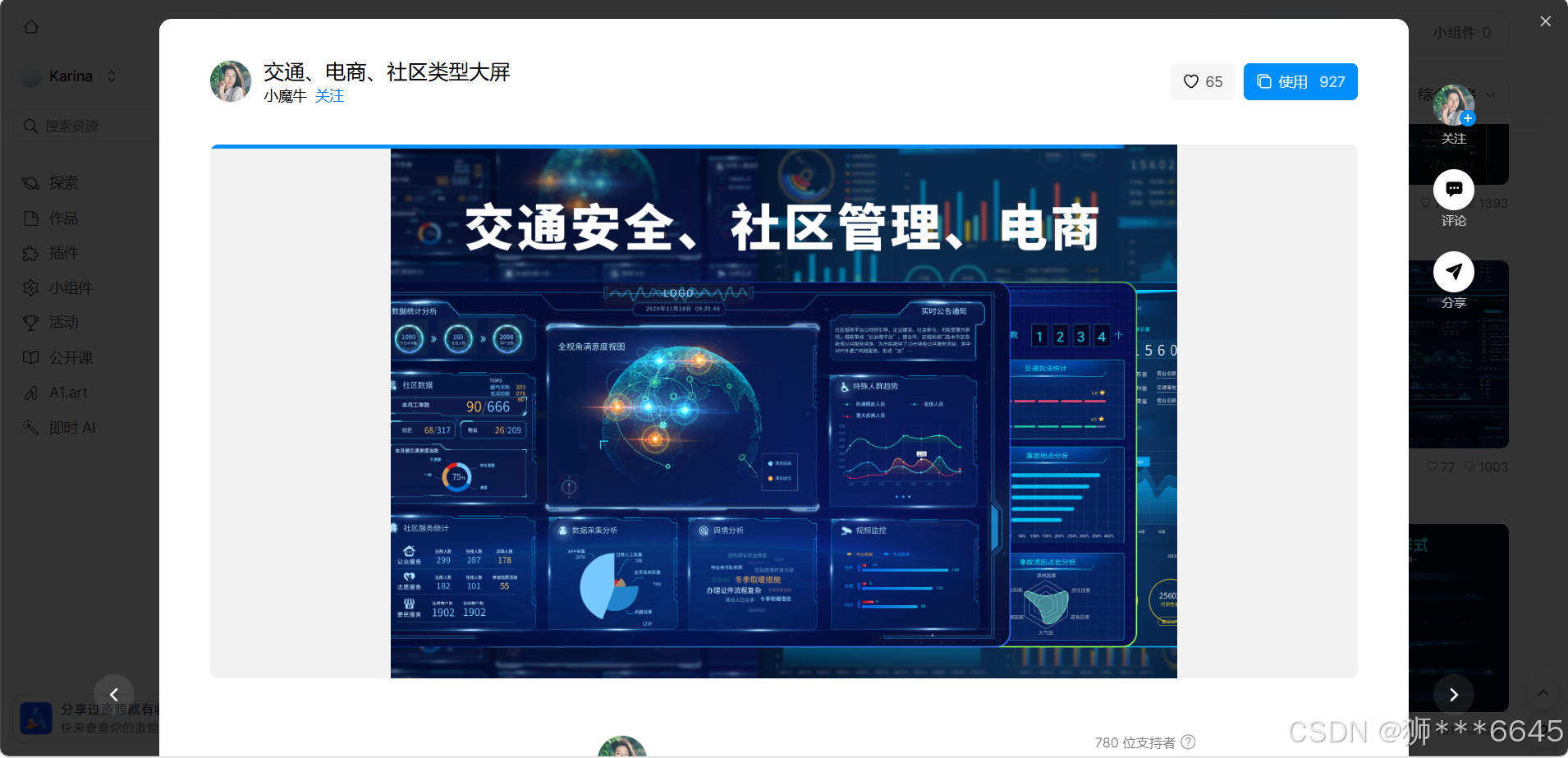
城市大屏设计素材宝库:助力设计师高效创作
城市大屏设计工作要求设计师在有限的时间内打造出令人惊叹的视觉效果,而拥有一套必备的素材集无疑是如虎添翼。这些素材犹如设计师的得力助手,无论是构建整体布局的设计模板,还是点缀细节的图标图形,都能在关键时刻发挥重要作用&a…...
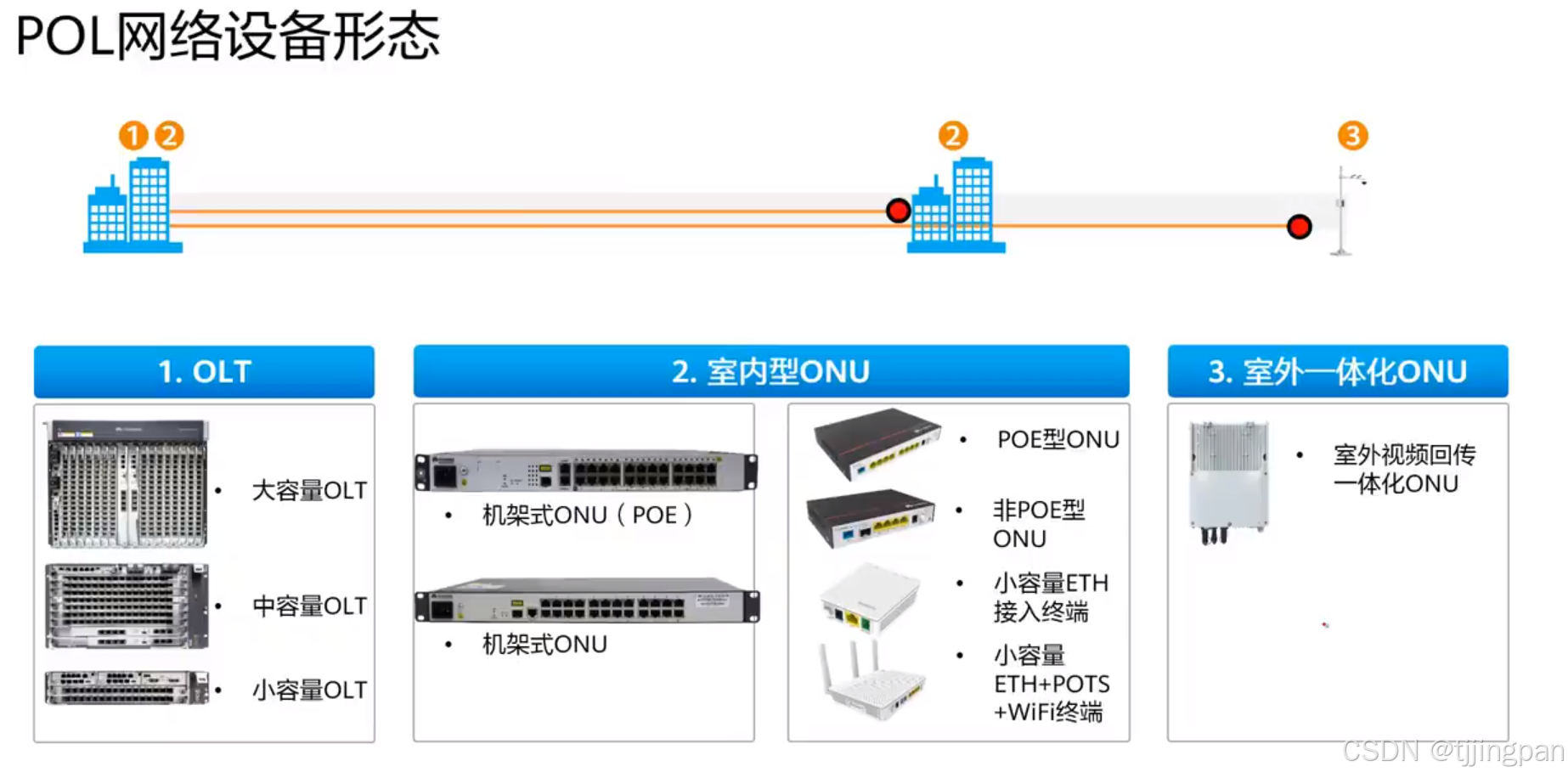
HCIA-Access V2.5_5_1PON系统概述_PON网络概述
PON网络设备有很多各类,可应用于不同的业务场景,从而实现不同的业务,本章介绍PON系统应用组成,分析PON系统的硬件结构和模块功能,描述PON系统的应用场景,帮助你对接入网中设备形态有更深刻的印象。 你可以…...

群落生态学研究进展】Hmsc包开展单物种和多物种分析的技术细节及Hmsc包的实际应用
联合物种分布模型(Joint Species Distribution Modelling,JSDM)在生态学领域,特别是群落生态学中发展最为迅速,它在分析和解读群落生态数据的革命性和独特视角使其受到广大国内外学者的关注。在学界不同研究团队研发出…...
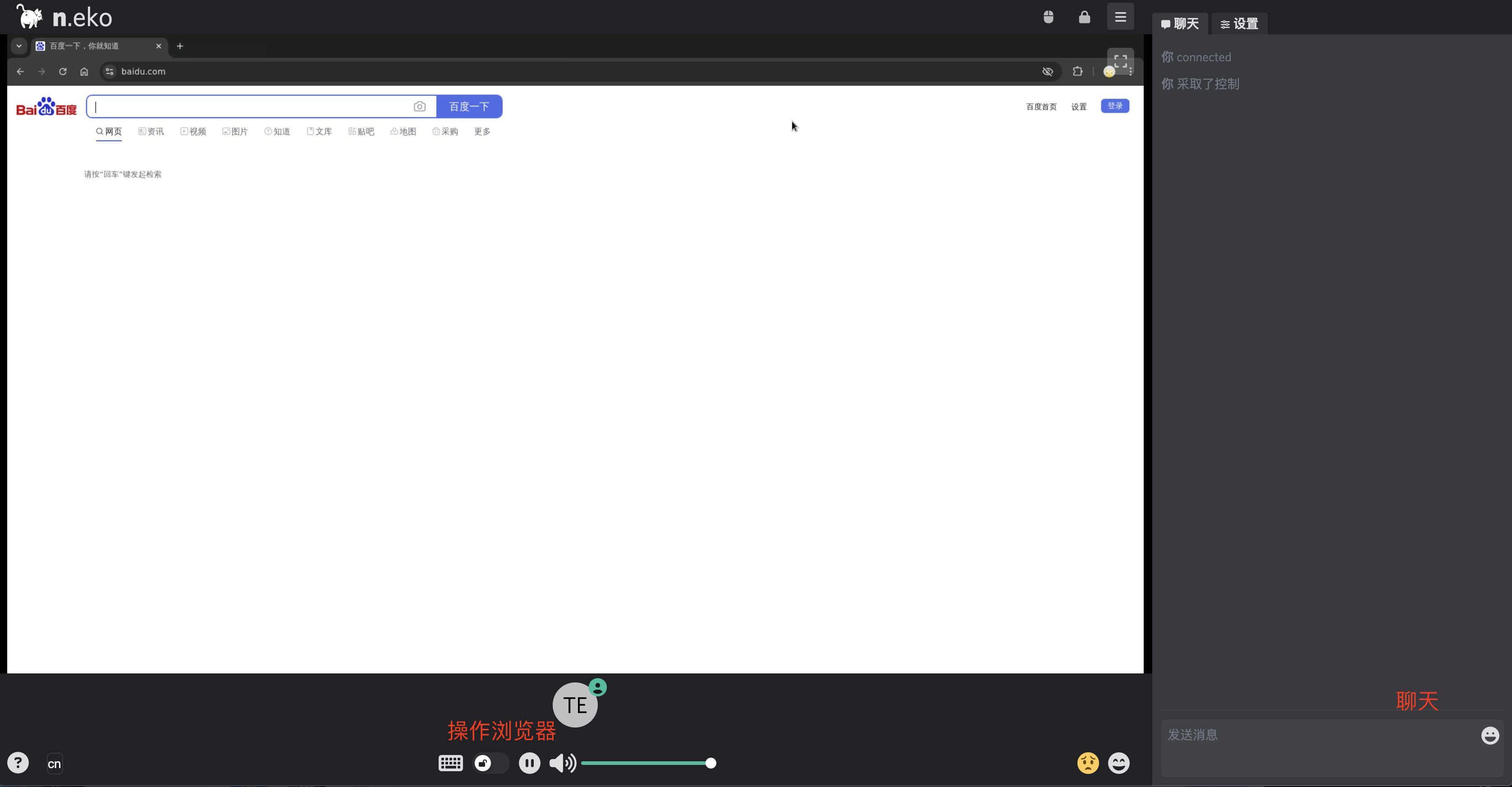
一个开源的自托管虚拟浏览器项目,支持在安全、私密的环境中使用浏览器
大家好,今天给大家分享一个开源的自托管虚拟浏览器项目Neko,旨在利用 WebRTC 技术在 Docker 容器中运行虚拟浏览器,为用户提供安全、私密且多功能的浏览体验。 项目介绍 Neko利用 WebRTC 技术在 Docker 容器中运行虚拟浏览器,提供…...
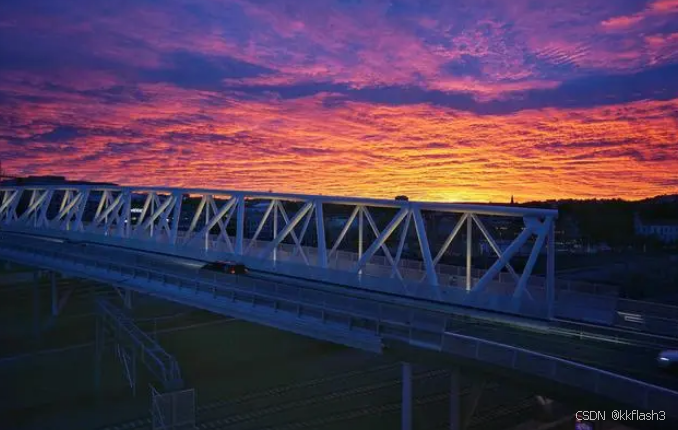
职场上,如何做好自我保护?
今天我们讨论一个话题:在职场上,如何保护好自己?废话不多说,我们直接上干货。 (一) 1.时刻准备一点零食或代餐,如果遇到长时间的会议,就补充点能量。代餐最好选流体,这…...
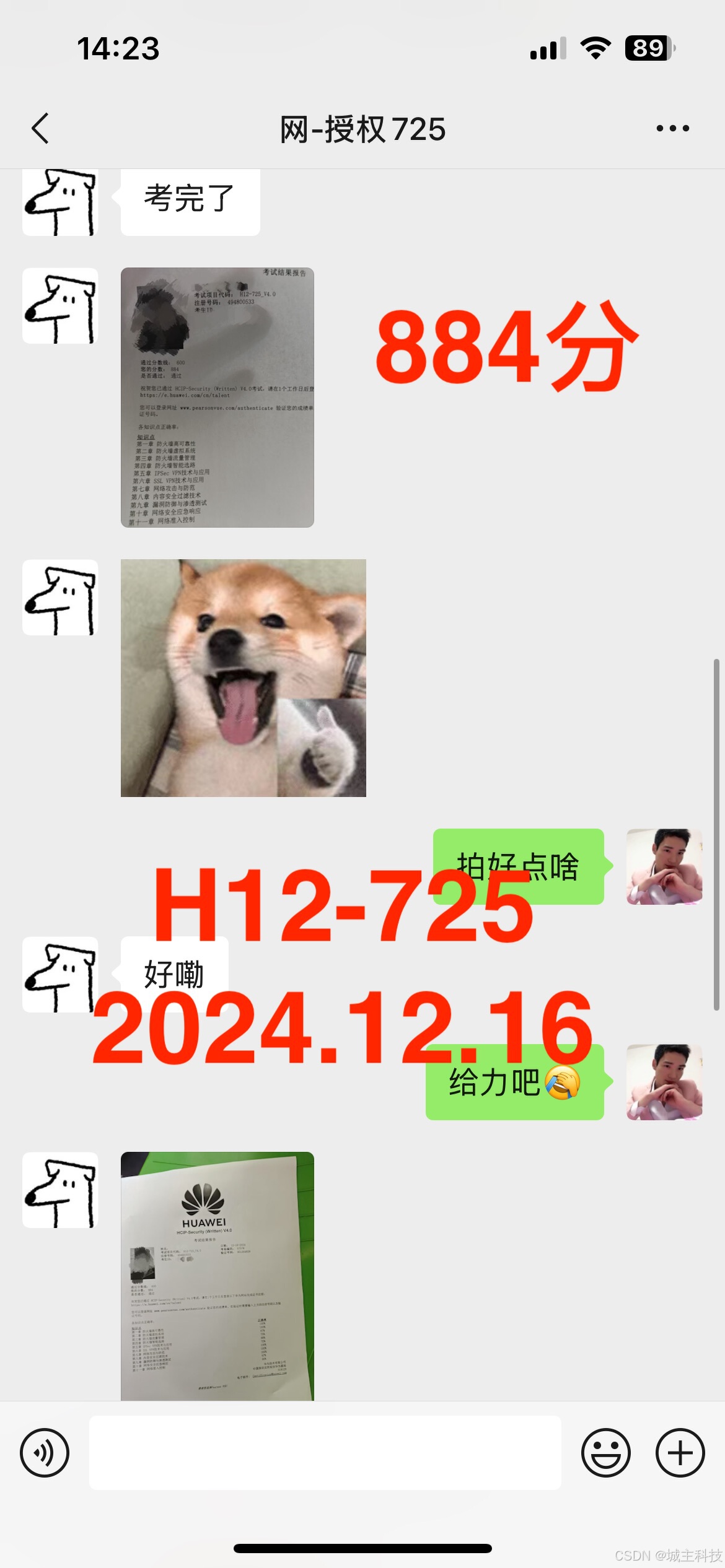
华为数通最新题库 H12-821 HCIP稳定过人中
以下是成绩单和考试人员 HCIP H12-831 HCIP H12-725 安全中级...
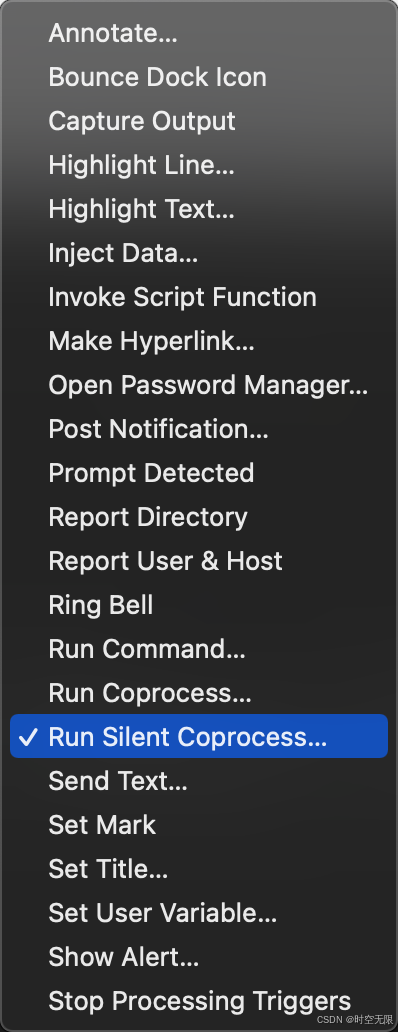
mac iterm2 使用 lrzsz
前言 mac os 终端不支持使用 rz sz 上传下载文件,本文提供解决方法。 mac 上安装 brew install lrzsz两个脚本 注意:/usr/local/bin/iterm2-send-zmodem.sh 中的 sz命令路径要和你mac 上 sz 命令路径一致。 /usr/local/bin/iterm2-recv-zmodem.sh 中…...
PostgreSql-学习06-libpq之同步命令处理
目录 一、环境 二、介绍 三、函数 1、PQsetdbLogin (1)作用 (2)声明 (3)参数介绍 (4)检测成功与否 2、PQfinish (1)作用 (2࿰…...
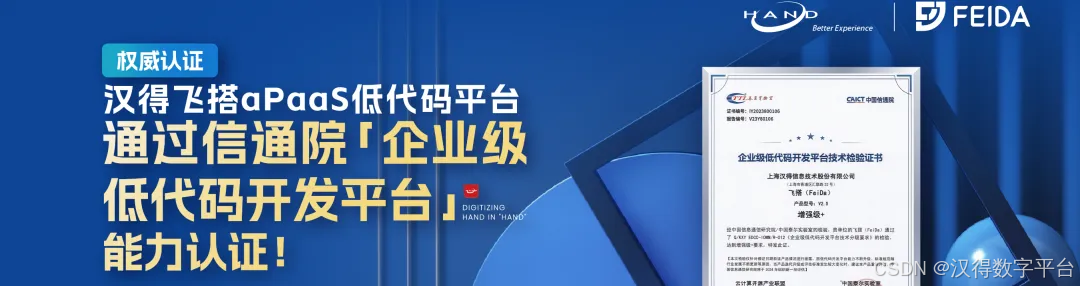
简单配置,全面保护:HZERO审计服务让安全触手可及
HZERO技术平台,凭借多年企业资源管理实施经验,深入理解企业痛点,为您提供了一套高效易用的审计解决方案。这套方案旨在帮助您轻松应对企业开发中的审计挑战,确保业务流程的合规性和透明度。 接下来,我将为大家详细介绍…...
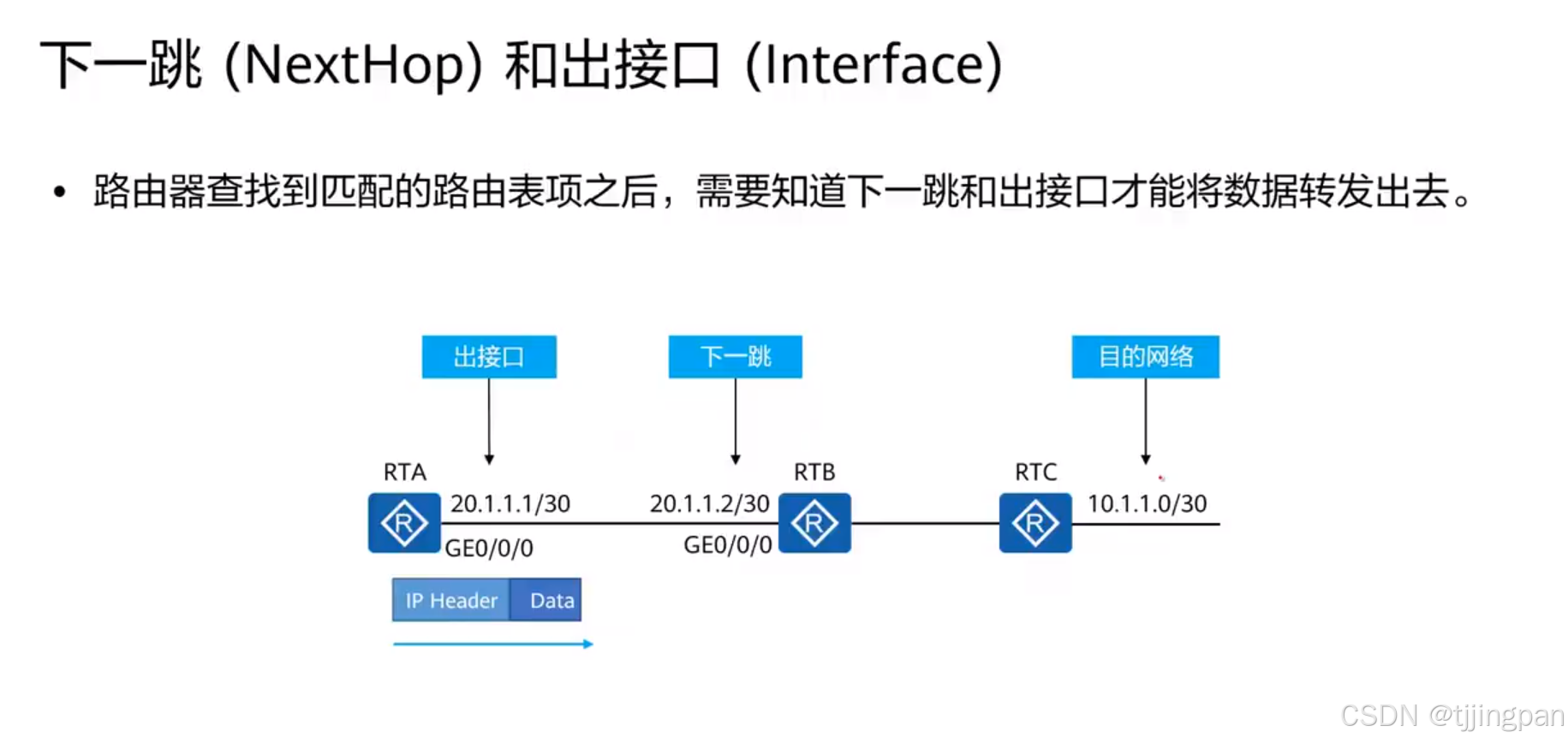
HCIA-Access V2.5_4_1_1路由协议基础_IP路由表
大型网络的拓扑结构一般会比较复杂,不同的部门,或者总部和分支可能处在不同的网络中,此时就需要使用路由器来连接不同的网络,实现网络之间的数据转发。 本章将介绍路由协议的基础知识、路由表的分类、静态路由基础与配置、VLAN间…...
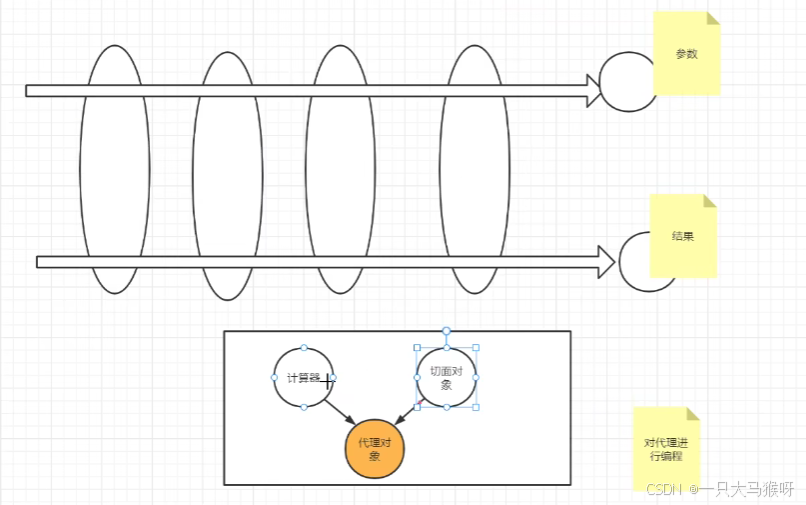
Spring IOC 和 AOP的学习笔记
Spring框架是java开发行业的标准 Spring全家桶 Web:Spring Web MVC/Spring MVC、Spring Web Flux 持久层:Spring Data / Spring Data JPA 、Spring Data Redis 、Spring Data MongoDB 安全校验:Spring Security 构建工程脚手架ÿ…...
uniapp 对接腾讯云IM群组成员管理(增删改查)
UniApp 实战:腾讯云IM群组成员管理(增删改查) 一、前言 在社交类App开发中,群组成员管理是核心功能之一。本文将基于UniApp框架,结合腾讯云IM SDK,详细讲解如何实现群组成员的增删改查全流程。 权限校验…...

使用VSCode开发Django指南
使用VSCode开发Django指南 一、概述 Django 是一个高级 Python 框架,专为快速、安全和可扩展的 Web 开发而设计。Django 包含对 URL 路由、页面模板和数据处理的丰富支持。 本文将创建一个简单的 Django 应用,其中包含三个使用通用基本模板的页面。在此…...
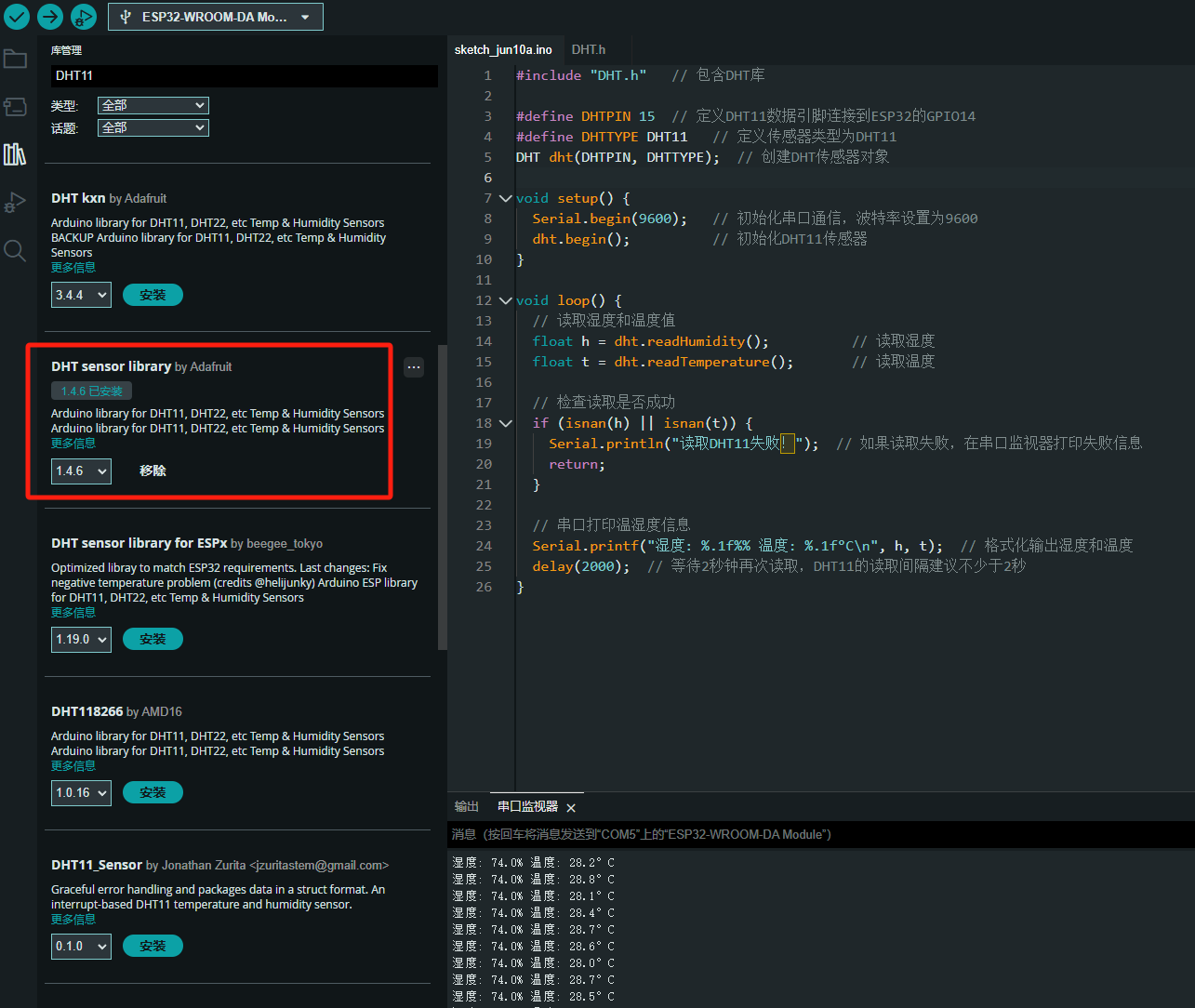
ESP32读取DHT11温湿度数据
芯片:ESP32 环境:Arduino 一、安装DHT11传感器库 红框的库,别安装错了 二、代码 注意,DATA口要连接在D15上 #include "DHT.h" // 包含DHT库#define DHTPIN 15 // 定义DHT11数据引脚连接到ESP32的GPIO15 #define D…...
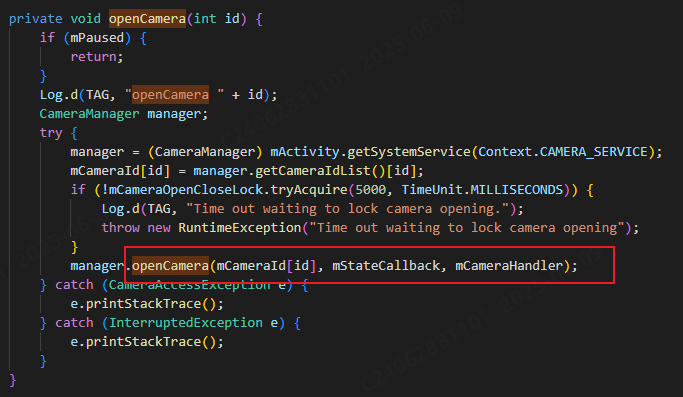
相机从app启动流程
一、流程框架图 二、具体流程分析 1、得到cameralist和对应的静态信息 目录如下: 重点代码分析: 启动相机前,先要通过getCameraIdList获取camera的个数以及id,然后可以通过getCameraCharacteristics获取对应id camera的capabilities(静态信息)进行一些openCamera前的…...
【决胜公务员考试】求职OMG——见面课测验1
2025最新版!!!6.8截至答题,大家注意呀! 博主码字不易点个关注吧,祝期末顺利~~ 1.单选题(2分) 下列说法错误的是:( B ) A.选调生属于公务员系统 B.公务员属于事业编 C.选调生有基层锻炼的要求 D…...
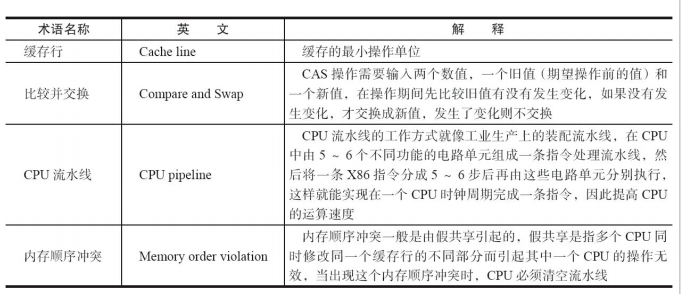
JUC笔记(上)-复习 涉及死锁 volatile synchronized CAS 原子操作
一、上下文切换 即使单核CPU也可以进行多线程执行代码,CPU会给每个线程分配CPU时间片来实现这个机制。时间片非常短,所以CPU会不断地切换线程执行,从而让我们感觉多个线程是同时执行的。时间片一般是十几毫秒(ms)。通过时间片分配算法执行。…...
【碎碎念】宝可梦 Mesh GO : 基于MESH网络的口袋妖怪 宝可梦GO游戏自组网系统
目录 游戏说明《宝可梦 Mesh GO》 —— 局域宝可梦探索Pokmon GO 类游戏核心理念应用场景Mesh 特性 宝可梦玩法融合设计游戏构想要素1. 地图探索(基于物理空间 广播范围)2. 野生宝可梦生成与广播3. 对战系统4. 道具与通信5. 延伸玩法 安全性设计 技术选…...
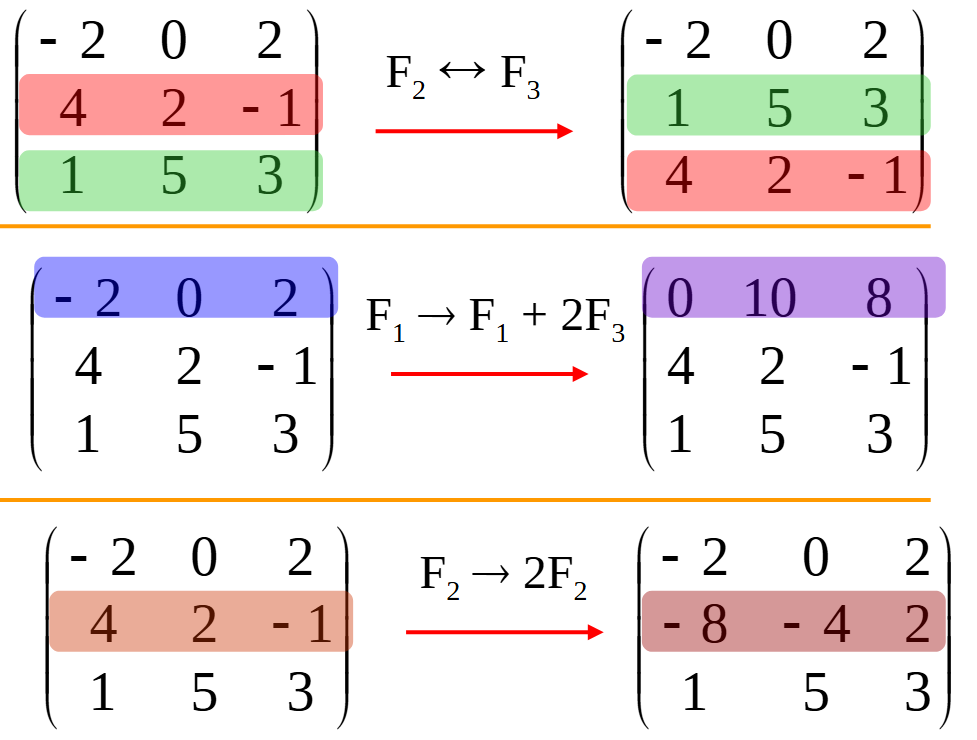
使用 SymPy 进行向量和矩阵的高级操作
在科学计算和工程领域,向量和矩阵操作是解决问题的核心技能之一。Python 的 SymPy 库提供了强大的符号计算功能,能够高效地处理向量和矩阵的各种操作。本文将深入探讨如何使用 SymPy 进行向量和矩阵的创建、合并以及维度拓展等操作,并通过具体…...
【生成模型】视频生成论文调研
工作清单 上游应用方向:控制、速度、时长、高动态、多主体驱动 类型工作基础模型WAN / WAN-VACE / HunyuanVideo控制条件轨迹控制ATI~镜头控制ReCamMaster~多主体驱动Phantom~音频驱动Let Them Talk: Audio-Driven Multi-Person Conversational Video Generation速…...
在QWebEngineView上实现鼠标、触摸等事件捕获的解决方案
这个问题我看其他博主也写了,要么要会员、要么写的乱七八糟。这里我整理一下,把问题说清楚并且给出代码,拿去用就行,照着葫芦画瓢。 问题 在继承QWebEngineView后,重写mousePressEvent或event函数无法捕获鼠标按下事…...