Tensorflow2 中对模型进行编译,不同loss函数的选择下输入数据格式需求变化
一、tf2中常用的损失函数介绍
在 TensorFlow 2 中,编译模型时可以选择不同的损失函数来定义模型的目标函数。不同的损失函数适用于不同的问题类型和模型架构。下面是几种常见的损失函数以及它们的作用和适用场景:
1.均方误差(Mean Squared Error, MSE):MSE 是回归问题中常用的损失函数,用于衡量预测值与真实值之间的平均平方差。较大的误差会得到更大的惩罚,适用于回归任务。
model.compile(loss='mse', ...)
2.二进制交叉熵(Binary Cross Entropy):二进制交叉熵是二分类问题中常用的损失函数,用于衡量两个类别之间的差异性。适用于二分类问题,输出为一个概率值的 sigmoid 激活的模型。
model.compile(loss='binary_crossentropy', ...)
3.多类交叉熵(Categorical Cross Entropy):多类交叉熵是多分类问题中常用的损失函数,用于衡量多个类别之间的差异性。适用于多分类问题,输出为每个类别的概率分布的 softmax 激活的模型。
model.compile(loss='categorical_crossentropy', ...)
4.稀疏分类交叉熵(Sparse Categorical Cross Entropy):类似于多类交叉熵,但适用于标签以整数形式表示的多分类问题,而不是 one-hot 编码。
model.compile(loss='sparse_categorical_crossentropy', ...)
5.KL 散度损失(Kullback-Leibler Divergence):KL 散度用于衡量两个概率分布的差异性。在生成模型中,常与自动编码器等模型结合使用,促使模型输出接近于预定义的概率分布。
model.compile(loss='kullback_leibler_divergence', ...)
除了上述常见的损失函数之外,还有其他一些定制化的损失函数,可以根据具体任务和需求来自定义。通过 tf.keras.losses 模块,您可以查看更多可用的损失函数,并选择适合自己模型的损失函数。在选择损失函数时,需要根据任务类型、数据分布以及模型设计进行合理选择,以获得最佳的训练效果。
二、两种损失函数的比较分析
多类交叉熵(Categorical Cross Entropy)和稀疏分类交叉熵(Sparse Categorical Cross Entropy)
相同点:都可用于数据多分类任务。
不同点:对数据的输入要求不一样,多类交叉熵(Categorical Cross Entropy)要求数据为one-hot 编码,这个主要是针对数据的标签数据,比如我们的数据标签数据读取的时候,其类别是0-9,这个数据可以是一列数据,这个时候我们可以使用稀疏分类交叉熵(Sparse Categorical Cross Entropy)函数直接进行编译。
one_hot编码(独热编码)说明:
一种将每个元素表示为二进制向量的编码方式,其中只有一个元素为1,其余元素都为0。例如,如果我们有一个长度为N的列表,那么它的one-hot编码将是一个NxN的矩阵,其中第i行表示第i个元素的编码。例如,如果我们有一个包含3种颜色的列表["红","蓝","绿"],那么它们的one-hot编码将是:
红:[1,0,0] 蓝:[0,1,0] 绿:[0,0,1]
这种编码方式常用于机器学习中,可以将每个类别标签转换为one-hot向量以便进行训练。
如果是使用多类交叉熵(Categorical Cross Entropy)作为损失函数,那么我们对数据进行one-hot编码,代码有的地方使用:
y_train=tf.keras.utils.to_categorical(y_train) #报错y_test=tf.keras.utils.to_categorical(y_test)
在tensorflow2.5环境下报错:
tensorflow.python.framework.errors_impl.InvalidArgumentError: logits and labels must have the same first dimension, got logits shape [12,16] and labels shape [204][[node sparse_categorical_crossentropy/SparseSoftmaxCrossEntropyWithLogits/SparseSoftmaxCrossEntropyWithLogits (defined at /PycharmProjects/pythonProject/ML_New/MLP_Classifier_tf/MLP_Classifier_tf_imgVali.py:286) ]] [Op:__inference_train_function_762]Function call stack:
train_function
这里我们可以使用以下代码替代:
y_train_one_hot = tf.one_hot(y_train, depth=num_classes)
y_test_one_hot = tf.one_hot(y_test, depth=num_classes)
三、示例代码分析
Sparse Categorical Cross Entropy和Categorical Cross Entropy对应的损失函数围为:
loss='sparse_categorical_crossentropy' loss='categorical_crossentropy'
使用minist数据做一个简单的MLP模型分类,这里先使用Sparse Categorical Cross Entropy损失函数。代码如下:
import tensorflow as tf
from tensorflow.keras.models import Sequential
from tensorflow.keras.layers import Dense# 准备数据集
(x_train, y_train), (x_test, y_test) = tf.keras.datasets.mnist.load_data()
x_train = x_train.reshape(-1, 784) / 255.0
x_test = x_test.reshape(-1, 784) / 255.0# 构建模型
model = Sequential()
model.add(Dense(64, activation='relu', input_dim=784))
model.add(Dense(64, activation='relu'))
model.add(Dense(10, activation='softmax'))# 编译模型
model.compile(optimizer='adam',loss='sparse_categorical_crossentropy',metrics=['accuracy'])# 训练模型
model.fit(x_train, y_train, epochs=10, batch_size=32, validation_data=(x_test, y_test))# 评估模型
loss, accuracy = model.evaluate(x_test, y_test)
print('Test Loss:', loss)
print('Test Accuracy:', accuracy)# 使用模型进行预测
predictions = model.predict(x_test[:5])
print('Predictions:', tf.argmax(predictions, axis=1))
print('Labels:', y_test[:5])
运行结果如下:
D:\PycharmProjects\pythonProject\venv\Scripts\python.exe D:/PycharmProjects/pythonProject/ML_New/MLP_Classifier_tf/MLP_TEST_MINIST.py
2023-10-14 22:28:27.465600: I tensorflow/stream_executor/platform/default/dso_loader.cc:53] Successfully opened dynamic library cudart64_110.dll
2023-10-14 22:28:30.610122: I tensorflow/stream_executor/platform/default/dso_loader.cc:53] Successfully opened dynamic library nvcuda.dll
2023-10-14 22:28:30.637119: I tensorflow/core/common_runtime/gpu/gpu_device.cc:1733] Found device 0 with properties:
pciBusID: 0000:01:00.0 name: NVIDIA GeForce RTX 2070 computeCapability: 7.5
coreClock: 1.62GHz coreCount: 36 deviceMemorySize: 8.00GiB deviceMemoryBandwidth: 417.29GiB/s
2023-10-14 22:28:30.637445: I tensorflow/stream_executor/platform/default/dso_loader.cc:53] Successfully opened dynamic library cudart64_110.dll
2023-10-14 22:28:30.648571: I tensorflow/stream_executor/platform/default/dso_loader.cc:53] Successfully opened dynamic library cublas64_11.dll
2023-10-14 22:28:30.648748: I tensorflow/stream_executor/platform/default/dso_loader.cc:53] Successfully opened dynamic library cublasLt64_11.dll
2023-10-14 22:28:30.652682: I tensorflow/stream_executor/platform/default/dso_loader.cc:53] Successfully opened dynamic library cufft64_10.dll
2023-10-14 22:28:30.654729: I tensorflow/stream_executor/platform/default/dso_loader.cc:53] Successfully opened dynamic library curand64_10.dll
2023-10-14 22:28:30.657643: I tensorflow/stream_executor/platform/default/dso_loader.cc:53] Successfully opened dynamic library cusolver64_11.dll
2023-10-14 22:28:30.661178: I tensorflow/stream_executor/platform/default/dso_loader.cc:53] Successfully opened dynamic library cusparse64_11.dll
2023-10-14 22:28:30.662311: I tensorflow/stream_executor/platform/default/dso_loader.cc:53] Successfully opened dynamic library cudnn64_8.dll
2023-10-14 22:28:30.662510: I tensorflow/core/common_runtime/gpu/gpu_device.cc:1871] Adding visible gpu devices: 0
2023-10-14 22:28:30.662864: I tensorflow/core/platform/cpu_feature_guard.cc:142] This TensorFlow binary is optimized with oneAPI Deep Neural Network Library (oneDNN) to use the following CPU instructions in performance-critical operations: AVX AVX2
To enable them in other operations, rebuild TensorFlow with the appropriate compiler flags.
2023-10-14 22:28:30.663583: I tensorflow/core/common_runtime/gpu/gpu_device.cc:1733] Found device 0 with properties:
pciBusID: 0000:01:00.0 name: NVIDIA GeForce RTX 2070 computeCapability: 7.5
coreClock: 1.62GHz coreCount: 36 deviceMemorySize: 8.00GiB deviceMemoryBandwidth: 417.29GiB/s
2023-10-14 22:28:30.663941: I tensorflow/core/common_runtime/gpu/gpu_device.cc:1871] Adding visible gpu devices: 0
2023-10-14 22:28:31.130464: I tensorflow/core/common_runtime/gpu/gpu_device.cc:1258] Device interconnect StreamExecutor with strength 1 edge matrix:
2023-10-14 22:28:31.130645: I tensorflow/core/common_runtime/gpu/gpu_device.cc:1264] 0
2023-10-14 22:28:31.130748: I tensorflow/core/common_runtime/gpu/gpu_device.cc:1277] 0: N
2023-10-14 22:28:31.130967: I tensorflow/core/common_runtime/gpu/gpu_device.cc:1418] Created TensorFlow device (/job:localhost/replica:0/task:0/device:GPU:0 with 6001 MB memory) -> physical GPU (device: 0, name: NVIDIA GeForce RTX 2070, pci bus id: 0000:01:00.0, compute capability: 7.5)
2023-10-14 22:28:31.709522: I tensorflow/compiler/mlir/mlir_graph_optimization_pass.cc:176] None of the MLIR Optimization Passes are enabled (registered 2)
Epoch 1/10
2023-10-14 22:28:31.920032: I tensorflow/stream_executor/platform/default/dso_loader.cc:53] Successfully opened dynamic library cublas64_11.dll
2023-10-14 22:28:32.369951: I tensorflow/stream_executor/platform/default/dso_loader.cc:53] Successfully opened dynamic library cublasLt64_11.dll
1875/1875 [==============================] - 5s 2ms/step - loss: 0.2845 - accuracy: 0.9174 - val_loss: 0.1443 - val_accuracy: 0.9547
Epoch 2/10
1875/1875 [==============================] - 5s 3ms/step - loss: 0.1261 - accuracy: 0.9633 - val_loss: 0.1085 - val_accuracy: 0.9646
Epoch 3/10
1875/1875 [==============================] - 5s 3ms/step - loss: 0.0937 - accuracy: 0.9716 - val_loss: 0.1034 - val_accuracy: 0.9690
Epoch 4/10
1875/1875 [==============================] - 5s 3ms/step - loss: 0.0731 - accuracy: 0.9772 - val_loss: 0.0987 - val_accuracy: 0.9714
Epoch 5/10
1875/1875 [==============================] - 5s 3ms/step - loss: 0.0612 - accuracy: 0.9810 - val_loss: 0.0828 - val_accuracy: 0.9749
Epoch 6/10
1875/1875 [==============================] - 5s 3ms/step - loss: 0.0507 - accuracy: 0.9835 - val_loss: 0.0955 - val_accuracy: 0.9702
Epoch 7/10
1875/1875 [==============================] - 5s 3ms/step - loss: 0.0430 - accuracy: 0.9859 - val_loss: 0.0863 - val_accuracy: 0.9746
Epoch 8/10
1875/1875 [==============================] - 5s 3ms/step - loss: 0.0374 - accuracy: 0.9874 - val_loss: 0.0935 - val_accuracy: 0.9737
Epoch 9/10
1875/1875 [==============================] - 6s 3ms/step - loss: 0.0328 - accuracy: 0.9894 - val_loss: 0.0902 - val_accuracy: 0.9754
Epoch 10/10
1875/1875 [==============================] - 6s 3ms/step - loss: 0.0287 - accuracy: 0.9900 - val_loss: 0.0902 - val_accuracy: 0.9771
313/313 [==============================] - 1s 2ms/step - loss: 0.0902 - accuracy: 0.9771
Test Loss: 0.09022707492113113
Test Accuracy: 0.9771000146865845
Predictions: tf.Tensor([7 2 1 0 4], shape=(5,), dtype=int64)
Labels: [7 2 1 0 4]Process finished with exit code 0
我们将损失函数修改为Categorical Cross Entropy运行代码就会报错:
ValueError: Shapes (32, 1) and (32, 10) are incompatible
这是因为我们没有将标签数据转化为独热编码,我们转换一下,,在model.fit()函数前加上:
y_train = tf.one_hot(y_train, depth=10)
y_test= tf.one_hot(y_test, depth=10)
运行结果如下:
D:\PycharmProjects\pythonProject\venv\Scripts\python.exe D:/PycharmProjects/pythonProject/ML_New/MLP_Classifier_tf/MLP_TEST_MINIST.py
2023-10-14 23:20:04.708405: I tensorflow/stream_executor/platform/default/dso_loader.cc:53] Successfully opened dynamic library cudart64_110.dll
2023-10-14 23:20:07.803493: I tensorflow/stream_executor/platform/default/dso_loader.cc:53] Successfully opened dynamic library nvcuda.dll
2023-10-14 23:20:07.833164: I tensorflow/core/common_runtime/gpu/gpu_device.cc:1733] Found device 0 with properties:
pciBusID: 0000:01:00.0 name: NVIDIA GeForce RTX 2070 computeCapability: 7.5
coreClock: 1.62GHz coreCount: 36 deviceMemorySize: 8.00GiB deviceMemoryBandwidth: 417.29GiB/s
2023-10-14 23:20:07.833480: I tensorflow/stream_executor/platform/default/dso_loader.cc:53] Successfully opened dynamic library cudart64_110.dll
2023-10-14 23:20:07.840527: I tensorflow/stream_executor/platform/default/dso_loader.cc:53] Successfully opened dynamic library cublas64_11.dll
2023-10-14 23:20:07.840689: I tensorflow/stream_executor/platform/default/dso_loader.cc:53] Successfully opened dynamic library cublasLt64_11.dll
2023-10-14 23:20:07.844132: I tensorflow/stream_executor/platform/default/dso_loader.cc:53] Successfully opened dynamic library cufft64_10.dll
2023-10-14 23:20:07.845657: I tensorflow/stream_executor/platform/default/dso_loader.cc:53] Successfully opened dynamic library curand64_10.dll
2023-10-14 23:20:07.848488: I tensorflow/stream_executor/platform/default/dso_loader.cc:53] Successfully opened dynamic library cusolver64_11.dll
2023-10-14 23:20:07.852061: I tensorflow/stream_executor/platform/default/dso_loader.cc:53] Successfully opened dynamic library cusparse64_11.dll
2023-10-14 23:20:07.853130: I tensorflow/stream_executor/platform/default/dso_loader.cc:53] Successfully opened dynamic library cudnn64_8.dll
2023-10-14 23:20:07.853317: I tensorflow/core/common_runtime/gpu/gpu_device.cc:1871] Adding visible gpu devices: 0
2023-10-14 23:20:07.853652: I tensorflow/core/platform/cpu_feature_guard.cc:142] This TensorFlow binary is optimized with oneAPI Deep Neural Network Library (oneDNN) to use the following CPU instructions in performance-critical operations: AVX AVX2
To enable them in other operations, rebuild TensorFlow with the appropriate compiler flags.
2023-10-14 23:20:07.854467: I tensorflow/core/common_runtime/gpu/gpu_device.cc:1733] Found device 0 with properties:
pciBusID: 0000:01:00.0 name: NVIDIA GeForce RTX 2070 computeCapability: 7.5
coreClock: 1.62GHz coreCount: 36 deviceMemorySize: 8.00GiB deviceMemoryBandwidth: 417.29GiB/s
2023-10-14 23:20:07.854879: I tensorflow/core/common_runtime/gpu/gpu_device.cc:1871] Adding visible gpu devices: 0
2023-10-14 23:20:08.326771: I tensorflow/core/common_runtime/gpu/gpu_device.cc:1258] Device interconnect StreamExecutor with strength 1 edge matrix:
2023-10-14 23:20:08.326942: I tensorflow/core/common_runtime/gpu/gpu_device.cc:1264] 0
2023-10-14 23:20:08.327041: I tensorflow/core/common_runtime/gpu/gpu_device.cc:1277] 0: N
2023-10-14 23:20:08.327252: I tensorflow/core/common_runtime/gpu/gpu_device.cc:1418] Created TensorFlow device (/job:localhost/replica:0/task:0/device:GPU:0 with 6001 MB memory) -> physical GPU (device: 0, name: NVIDIA GeForce RTX 2070, pci bus id: 0000:01:00.0, compute capability: 7.5)
2023-10-14 23:20:08.914697: I tensorflow/compiler/mlir/mlir_graph_optimization_pass.cc:176] None of the MLIR Optimization Passes are enabled (registered 2)
Epoch 1/10
2023-10-14 23:20:09.138669: I tensorflow/stream_executor/platform/default/dso_loader.cc:53] Successfully opened dynamic library cublas64_11.dll1/1875 [..............................] - ETA: 21:57 - loss: 2.4066 - accuracy: 0.12502023-10-14 23:20:09.626287: I tensorflow/stream_executor/platform/default/dso_loader.cc:53] Successfully opened dynamic library cublasLt64_11.dll
1875/1875 [==============================] - 6s 3ms/step - loss: 0.2784 - accuracy: 0.9182 - val_loss: 0.1517 - val_accuracy: 0.9510
Epoch 2/10
1875/1875 [==============================] - 5s 3ms/step - loss: 0.1217 - accuracy: 0.9633 - val_loss: 0.1258 - val_accuracy: 0.9611
Epoch 3/10
1875/1875 [==============================] - 5s 3ms/step - loss: 0.0874 - accuracy: 0.9731 - val_loss: 0.1045 - val_accuracy: 0.9666
Epoch 4/10
1875/1875 [==============================] - 5s 3ms/step - loss: 0.0701 - accuracy: 0.9778 - val_loss: 0.0929 - val_accuracy: 0.9718
Epoch 5/10
1875/1875 [==============================] - 5s 3ms/step - loss: 0.0569 - accuracy: 0.9821 - val_loss: 0.0853 - val_accuracy: 0.9751
Epoch 6/10
1875/1875 [==============================] - 5s 3ms/step - loss: 0.0488 - accuracy: 0.9844 - val_loss: 0.0911 - val_accuracy: 0.9706
Epoch 7/10
1875/1875 [==============================] - 5s 3ms/step - loss: 0.0402 - accuracy: 0.9868 - val_loss: 0.0847 - val_accuracy: 0.9748
Epoch 8/10
1875/1875 [==============================] - 5s 3ms/step - loss: 0.0355 - accuracy: 0.9882 - val_loss: 0.0975 - val_accuracy: 0.9723
Epoch 9/10
1875/1875 [==============================] - 5s 3ms/step - loss: 0.0307 - accuracy: 0.9895 - val_loss: 0.1027 - val_accuracy: 0.9743
Epoch 10/10
1875/1875 [==============================] - 5s 3ms/step - loss: 0.0281 - accuracy: 0.9907 - val_loss: 0.1004 - val_accuracy: 0.9734
313/313 [==============================] - 1s 2ms/step - loss: 0.1004 - accuracy: 0.9734
Test Loss: 0.10037881135940552
Test Accuracy: 0.9733999967575073
Predictions: tf.Tensor([7 2 1 0 4], shape=(5,), dtype=int64)
Labels: tf.Tensor(
[[0. 0. 0. 0. 0. 0. 0. 1. 0. 0.][0. 0. 1. 0. 0. 0. 0. 0. 0. 0.][0. 1. 0. 0. 0. 0. 0. 0. 0. 0.][1. 0. 0. 0. 0. 0. 0. 0. 0. 0.][0. 0. 0. 0. 1. 0. 0. 0. 0. 0.]], shape=(5, 10), dtype=float32)Process finished with exit code 0
注意事项:
使用稀疏交叉熵损失函数编译的模型的预测结果标签从0开始。如果自己的数据是从1开始的,那么后面做验证分析的时候需要注意两者应该保持一致。
在使用稀疏交叉熵损失函数进行多分类问题训练时,标签通常使用整数表示,并且标签值的范围是从0到类别数量减1。模型的输出也应该是每个类别的概率分布。
例如,如果有3个类别,标签将被编码为0、1和2,并且模型的输出将是一个长度为3的概率分布向量,表示对每个类别的预测概率。
在预测时,模型会返回对每个类别的预测概率,通过取最大概率对应的索引,就可以得到预测的类别。这个索引范围是从0到类别数量减1。与稀疏交叉熵不同,使用普通的(非稀疏)交叉熵损失函数时,标签通常使用 one-hot 编码,其中每个类别都由一个向量表示,只有真实标签对应的位置为1,其余都为0。在这种情况下,预测结果的标签也是从0开始的。
相关文章:
Tensorflow2 中对模型进行编译,不同loss函数的选择下输入数据格式需求变化
一、tf2中常用的损失函数介绍 在 TensorFlow 2 中,编译模型时可以选择不同的损失函数来定义模型的目标函数。不同的损失函数适用于不同的问题类型和模型架构。下面是几种常见的损失函数以及它们的作用和适用场景: 1.均方误差(Mean Squared …...
【python】基础语法(三)--异常、模块、包
异常 代码中出现的报错问题,可能会导致整个代码的停止,为了避免这种情况,有了捕获异常操作; 捕获异常 提前预知可能出错的代码,做好准备,避免因bug导致整个项目停止; try:可能出…...
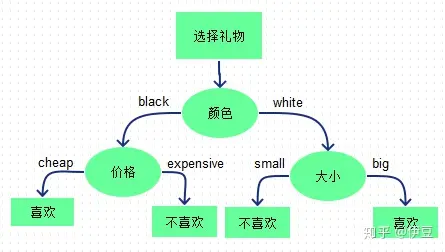
XGBoost+LR融合
1、背景简介 xgboostlr模型融合方法用于分类或者回归的思想最早由facebook在广告ctr预测中提出,其论文Practical Lessons from Predicting Clicks on Ads at Facebook有对其进行阐述。在这篇论文中他们提出了一种将xgboost作为feature transform的方法。大概的思想…...
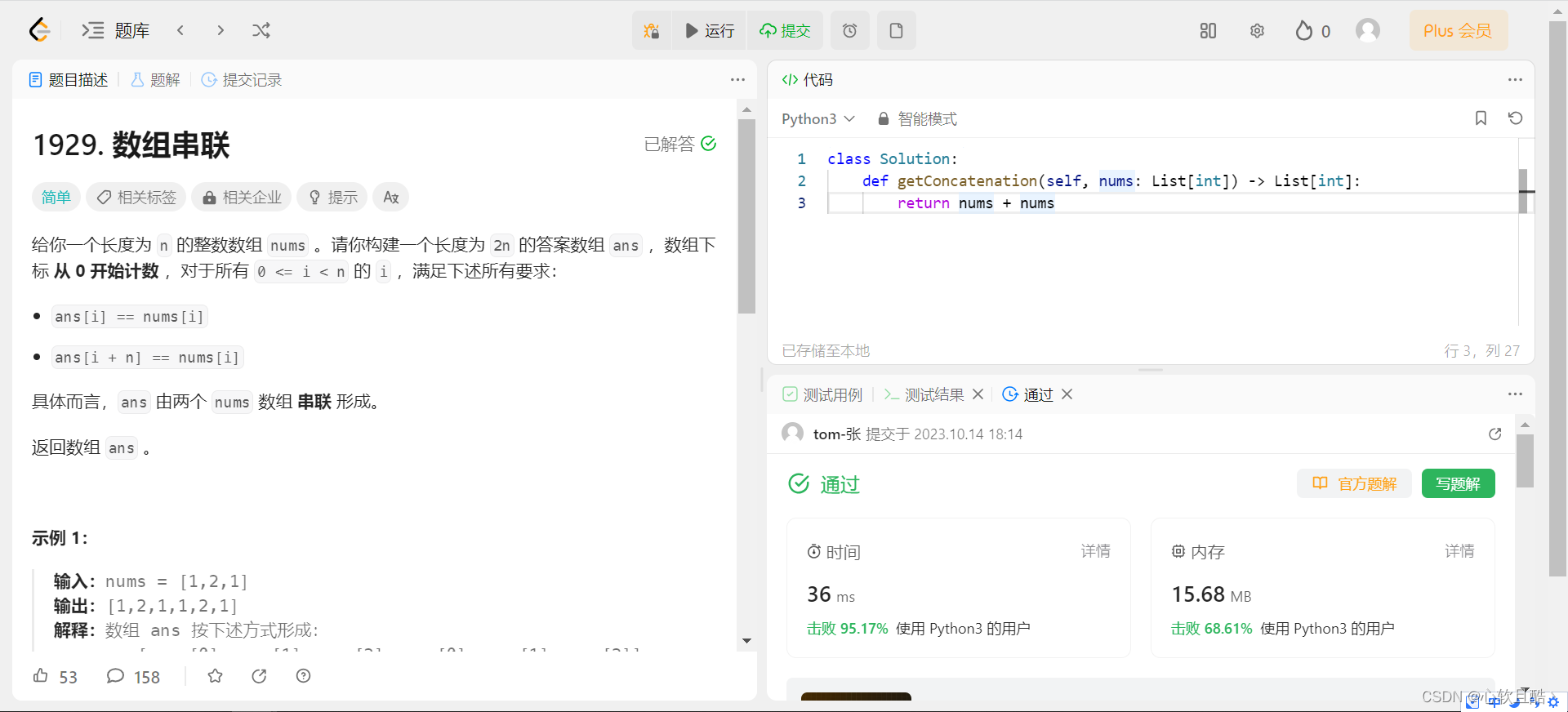
leetcode:1929. 数组串联(python3解法)
难度:简单 给你一个长度为 n 的整数数组 nums 。请你构建一个长度为 2n 的答案数组 ans ,数组下标 从 0 开始计数 ,对于所有 0 < i < n 的 i ,满足下述所有要求: ans[i] nums[i]ans[i n] nums[i] 具体而言&am…...
Epoch和episodes的区别
“Epoch” 和 “episode” 是两个不同的概念,通常在不同领域中使用。 Epoch(周期): Epoch 是一个在机器学习和深度学习中常用的术语,通常用于表示训练数据集中的一个完整遍历。在每个 epoch 中,整个训练数据…...
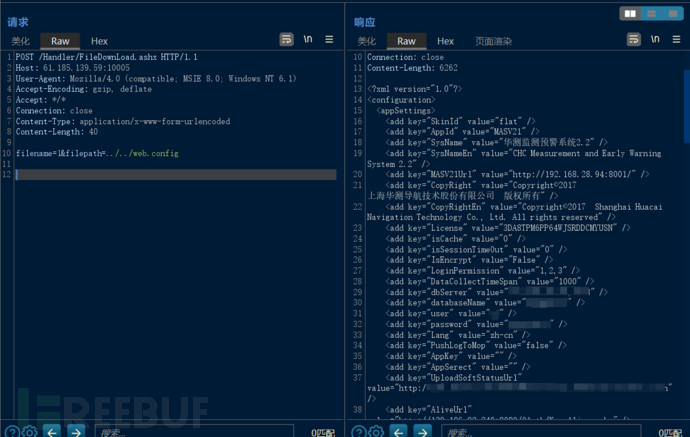
漏洞复现--华测监测预警系统2.2任意文件读取
免责声明: 文章中涉及的漏洞均已修复,敏感信息均已做打码处理,文章仅做经验分享用途,切勿当真,未授权的攻击属于非法行为!文章中敏感信息均已做多层打马处理。传播、利用本文章所提供的信息而造成的任何直…...
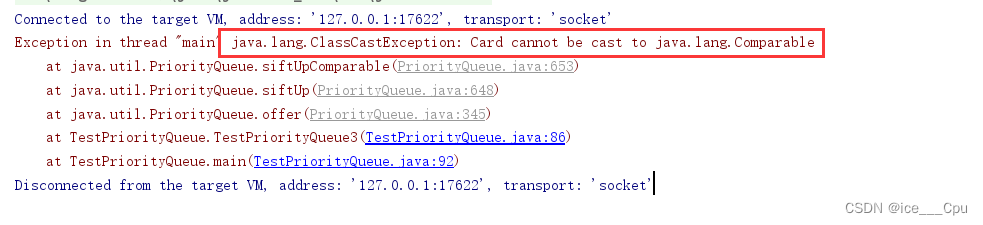
数据结构 - 6(优先级队列(堆)13000字详解)
一:堆 1.1 堆的基本概念 堆分为两种:大堆和小堆。它们之间的区别在于元素在堆中的排列顺序和访问方式。 大堆(Max Heap): 在大堆中,父节点的值比它的子节点的值要大。也就是说,堆的根节点是堆…...
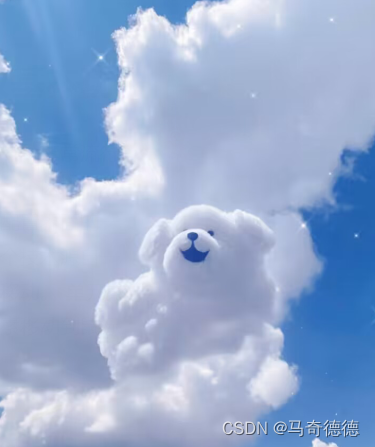
Js高级技巧—拖放
拖放基本功能实现 拖放是一种非常流行的用户界面模式。它的概念很简单:点击某个对象,并按住鼠标按钮不放,将 鼠标移动到另一个区域,然后释放鼠标按钮将对象“放”在这里。拖放功能也流行到了 Web 上,成为 了一些更传统…...
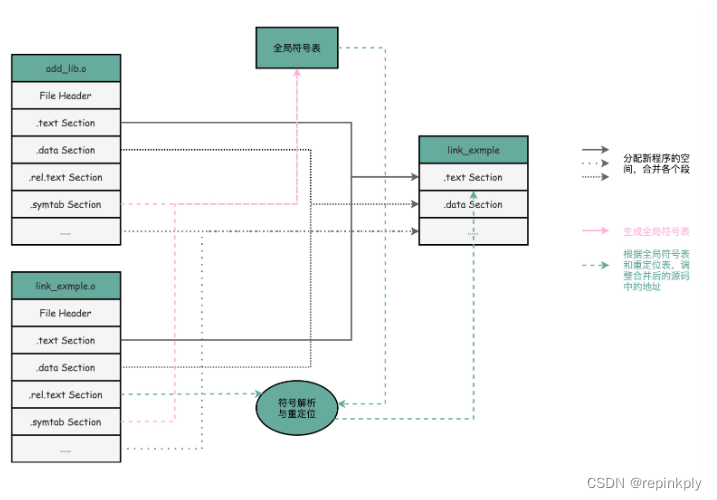
ELF和静态链接:为什么程序无法同时在Linux和Windows下运行?
目录 疑问 编译、链接和装载:拆解程序执行 ELF 格式和链接:理解链接过程 小结 疑问 既然我们的程序最终都被变成了一条条机器码去执行,那为什么同一个程序,在同一台计算机上,在 Linux 下可以运行,而在…...
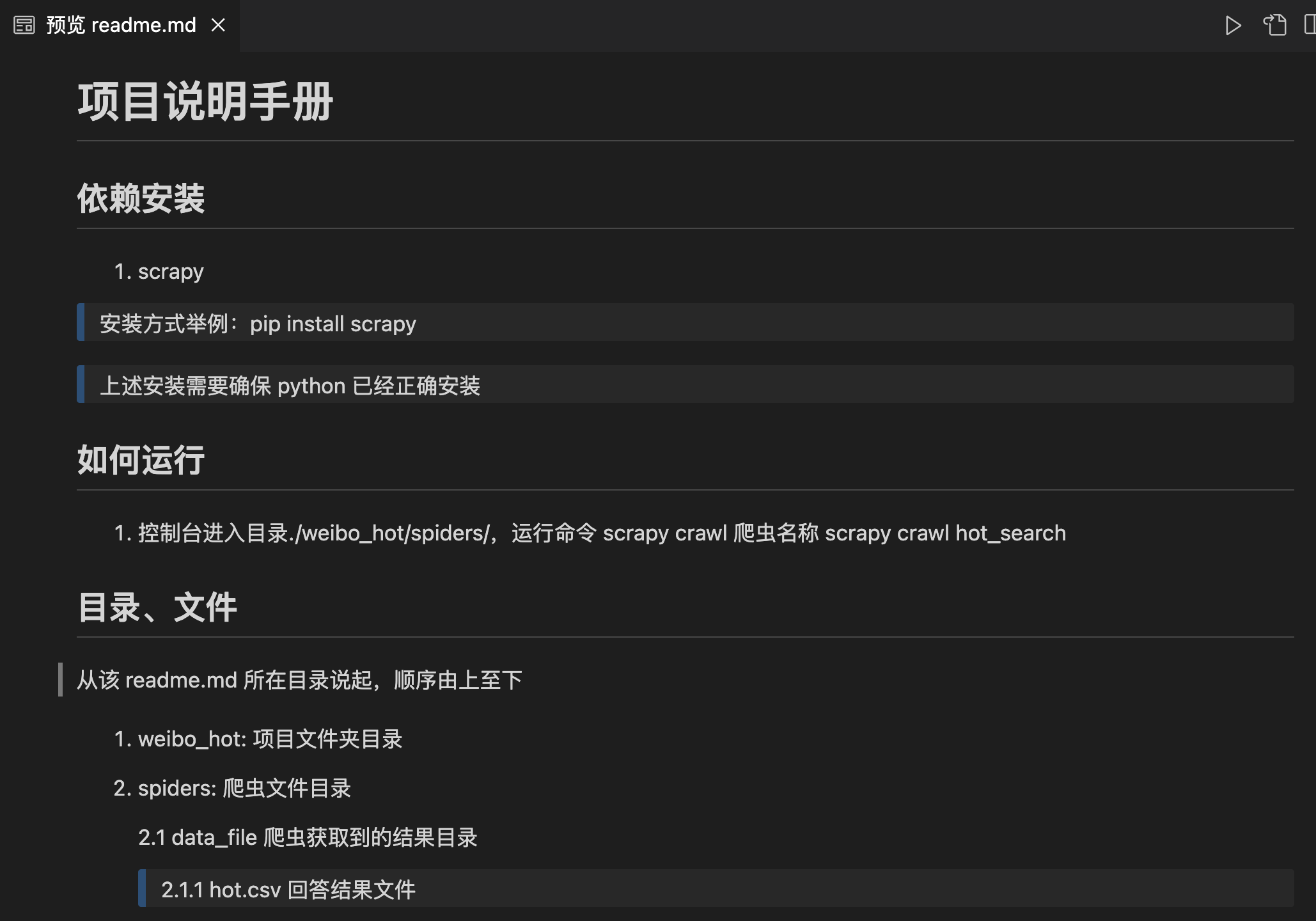
【爬虫实战】python微博热搜榜Top50
一.最终效果 二.项目代码 2.1 新建项目 本文使用scrapy分布式、多线程爬虫框架编写的高性能爬虫,因此新建、运行scrapy项目3步骤: 1.新建项目: scrapy startproject weibo_hot 2.新建 spider: scrapy genspider hot_search "weibo.com" 3…...
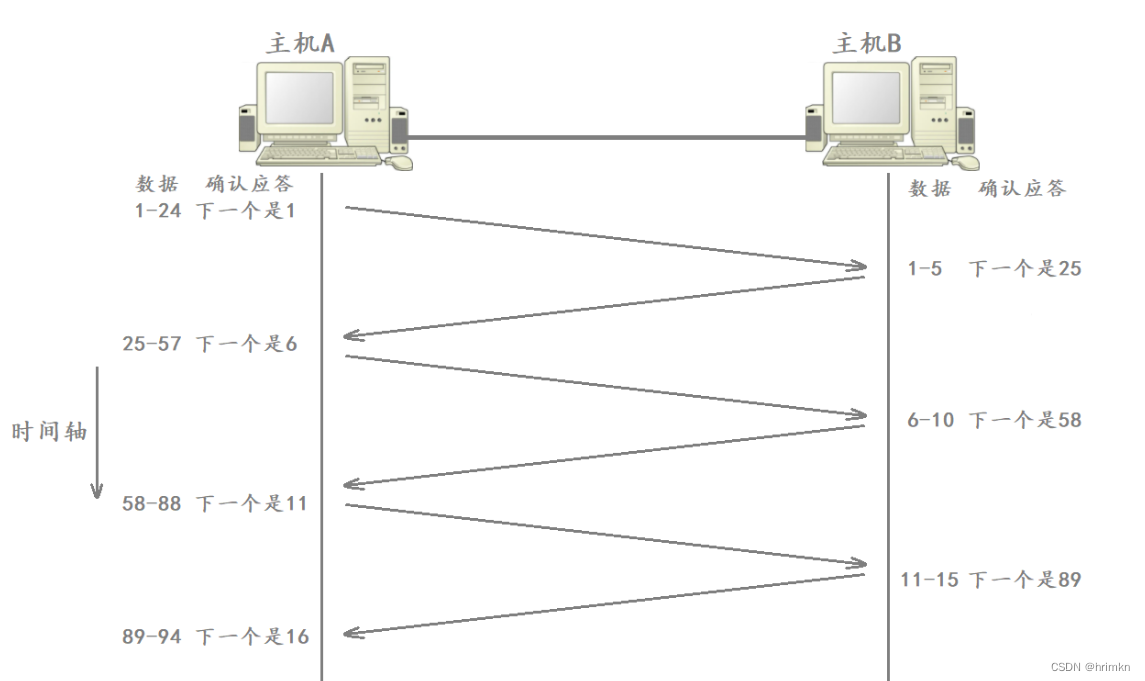
【网络基础】——传输层
目录 前言 传输层 端口号 端口号范围划分 知名端口号 进程与端口号的关系 netstat UDP协议 UDP协议位置 UDP协议格式 UDP协议特点 面向数据报 UDP缓冲区 UDP的使用注意事项 基于UDP的应用层协议 TCP协议 TCP简介 TCP协议格式 确认应答机制&#…...
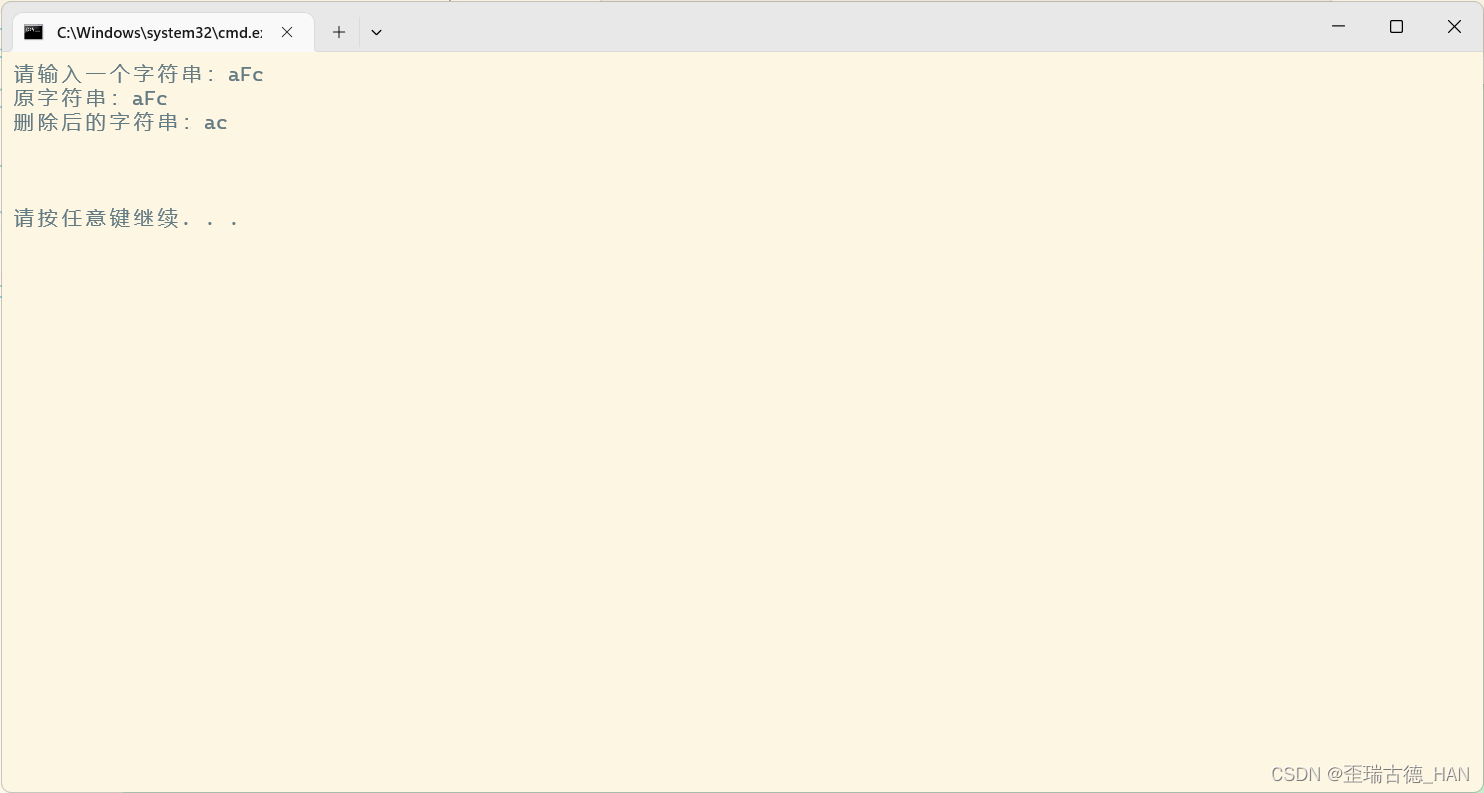
删除字符串特定的字符(fF)C语言
代码: #include <stdio.h> void funDel(char *str) {int i, j;for (i j 0; str[i] ! \0; i)if (str[i] ! f && str[i] ! F)str[j] str[i];str[j] \0; }int main() {char str[100];printf("请输入一个字符串:");gets(str);pr…...
C++入门(1):命名空间,IO流 输入输出,缺省参数
一、命名空间 1.1 命名空间的作用: 避免标识符命名冲突 1.2 命名空间定义: 关键字:namespace namespace test {// 命名空间内可以定义变量/函数/类型int a 10;int Add(int x, int y){return x y;}struct Stack{int* a;int top;int …...
Go 语言面试题(三):并发编程
文章目录 Q1 无缓冲的 channel 和 有缓冲的 channel 的区别?Q2 什么是协程泄露(Goroutine Leak)?Q3 Go 可以限制运行时操作系统线程的数量吗? Q1 无缓冲的 channel 和 有缓冲的 channel 的区别? 对于无缓冲的 channel,…...

Linux - make命令 和 makefile
make命令和 makefile 如果之前用过 vim 的话,应该会对 vim 又爱又恨吧,刚开始使用感觉非常的别扭,因为这种编写代码的方式,和在 windows 当中用图形化界面的方式编写代码的方式差别是不是很大。当你把vim 用熟悉的之后࿰…...
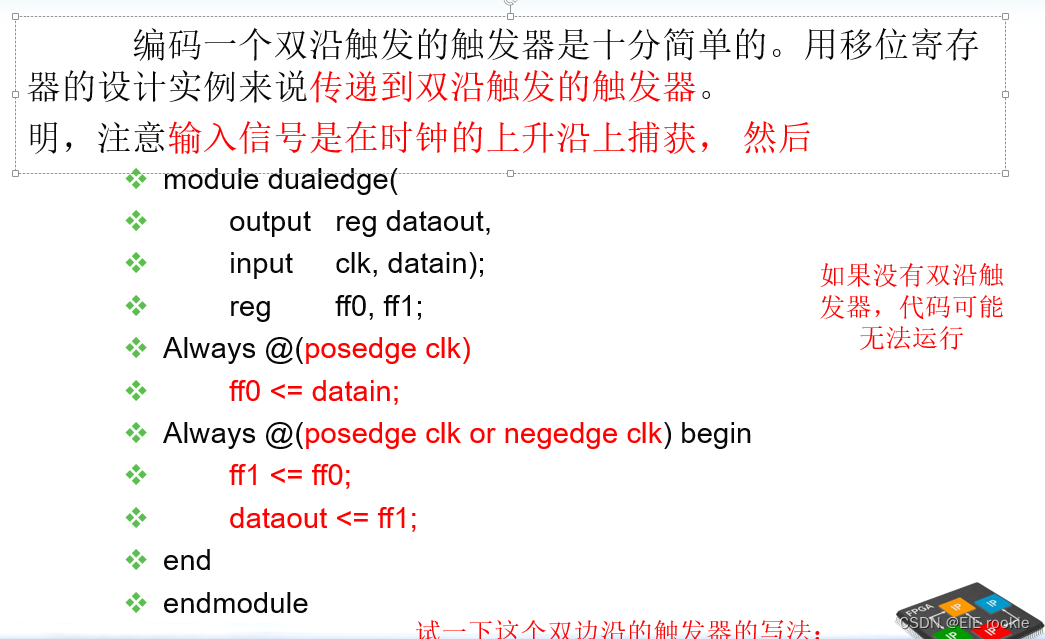
FPGA复习(功耗)
减小功耗 就得减小电流 电流和CF有关( C: 电容(被门数目和布线长度影响) F:时钟频率) 方法大纲 减小功耗:1 时钟控制 2输入控制 3减小供电电压 4双沿触发器 5修改终端 同步数字电路降低动态功耗:动态禁止…...
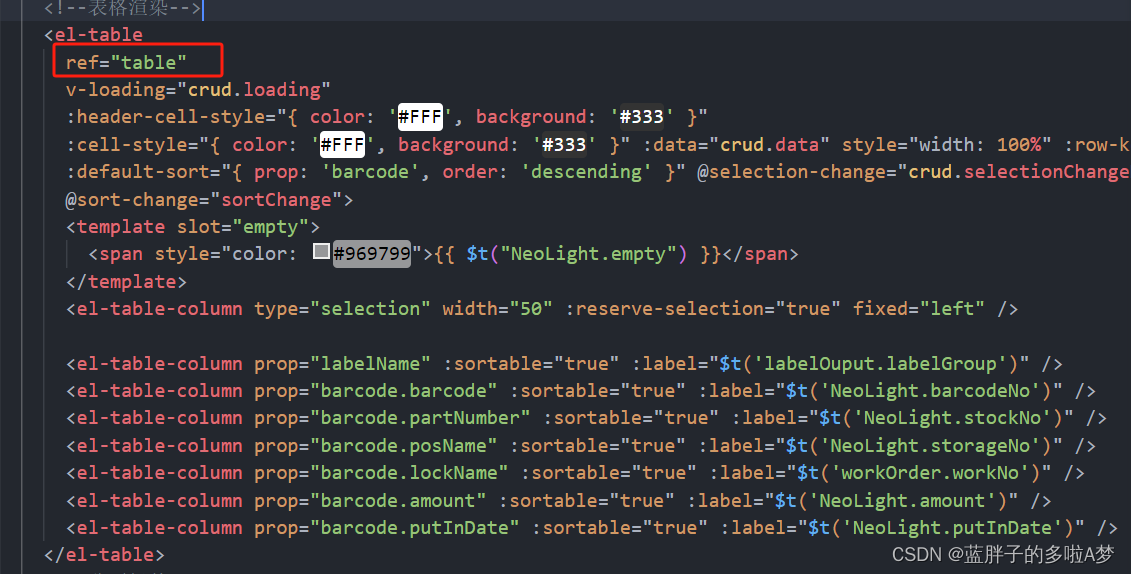
element ui el-table表格复选框,弹框关闭取消打勾选择
//弹框表格复选框清空 this.$nextTick(()>{this.$refs.table.clearSelection();})<el-table ref"table" v-loading"crud.loading" :header-cell-style"{ color: #FFF, background: #333 }":cell-style"{ color: #FFF, background: #3…...
数据结构——队列
1.队列元素逆置 【问题描述】 已知Q是一个非空队列,S是一个空栈。仅使用少量工作变量以及对队列和栈的基本操作,编写一个算法,将队列Q中的所有元素逆置。 【输入形式】 输入的第一行为队列元素个数,第二行为队列从首至尾的元素…...

【Unity引擎核心-Object,序列化,资产管理,内存管理】
文章目录 整体介绍Native & Managed Objects什么是序列化序列化用来做什么Editor和运行时序列化的区别脚本序列化针对序列化的使用建议 Unity资产管理导入Asset Process为何要做引擎资源文件导入Main-Assets和 Sub-Assets资产的导入管线Hook,AssetPostprocessor…...
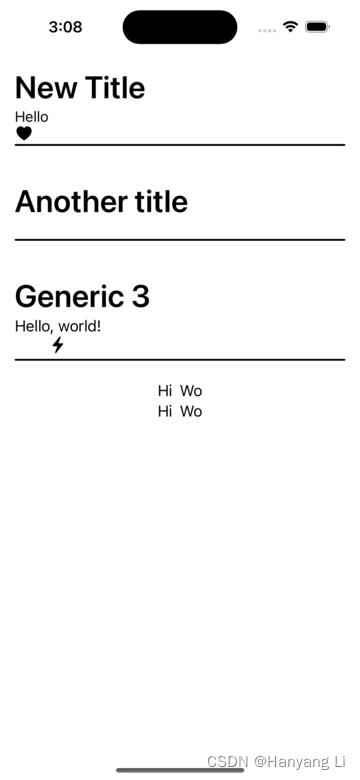
Generics/泛型, ViewBuilder/视图构造器 的使用
1. Generics 泛型的定义及使用 1.1 创建使用泛型的实例 GenericsBootcamp.swift import SwiftUIstruct StringModel {let info: String?func removeInfo() -> StringModel{StringModel(info: nil)} }struct BoolModel {let info: Bool?func removeInfo() -> BoolModel…...
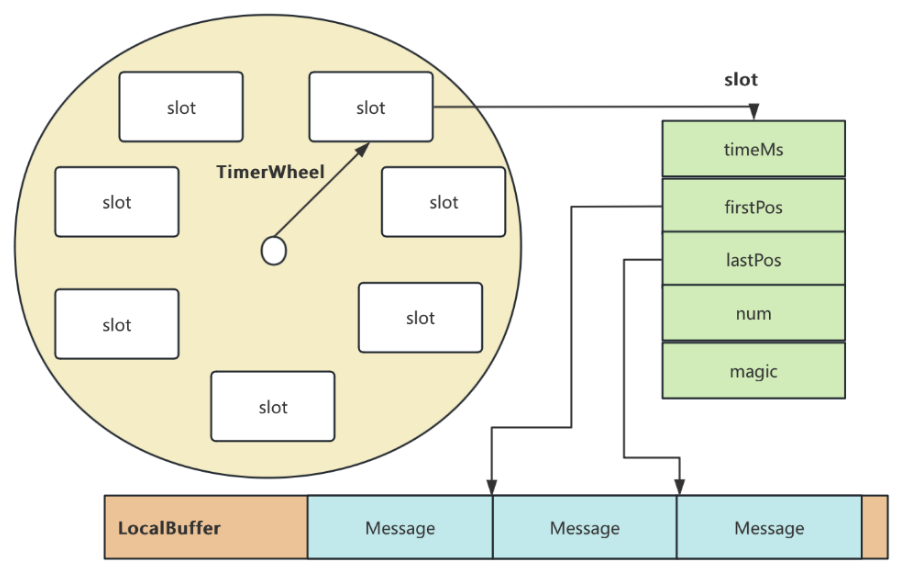
RocketMQ延迟消息机制
两种延迟消息 RocketMQ中提供了两种延迟消息机制 指定固定的延迟级别 通过在Message中设定一个MessageDelayLevel参数,对应18个预设的延迟级别指定时间点的延迟级别 通过在Message中设定一个DeliverTimeMS指定一个Long类型表示的具体时间点。到了时间点后…...
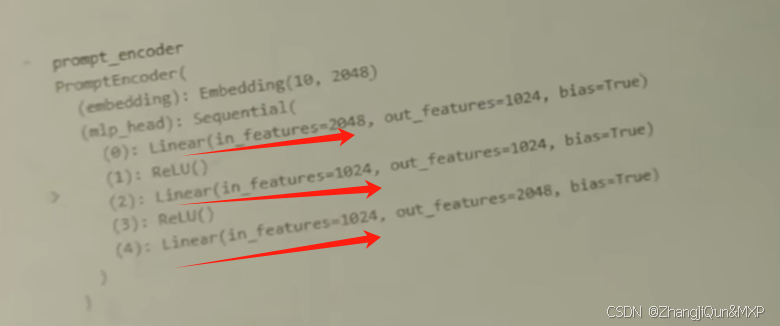
Prompt Tuning、P-Tuning、Prefix Tuning的区别
一、Prompt Tuning、P-Tuning、Prefix Tuning的区别 1. Prompt Tuning(提示调优) 核心思想:固定预训练模型参数,仅学习额外的连续提示向量(通常是嵌入层的一部分)。实现方式:在输入文本前添加可训练的连续向量(软提示),模型只更新这些提示参数。优势:参数量少(仅提…...
golang循环变量捕获问题
在 Go 语言中,当在循环中启动协程(goroutine)时,如果在协程闭包中直接引用循环变量,可能会遇到一个常见的陷阱 - 循环变量捕获问题。让我详细解释一下: 问题背景 看这个代码片段: fo…...
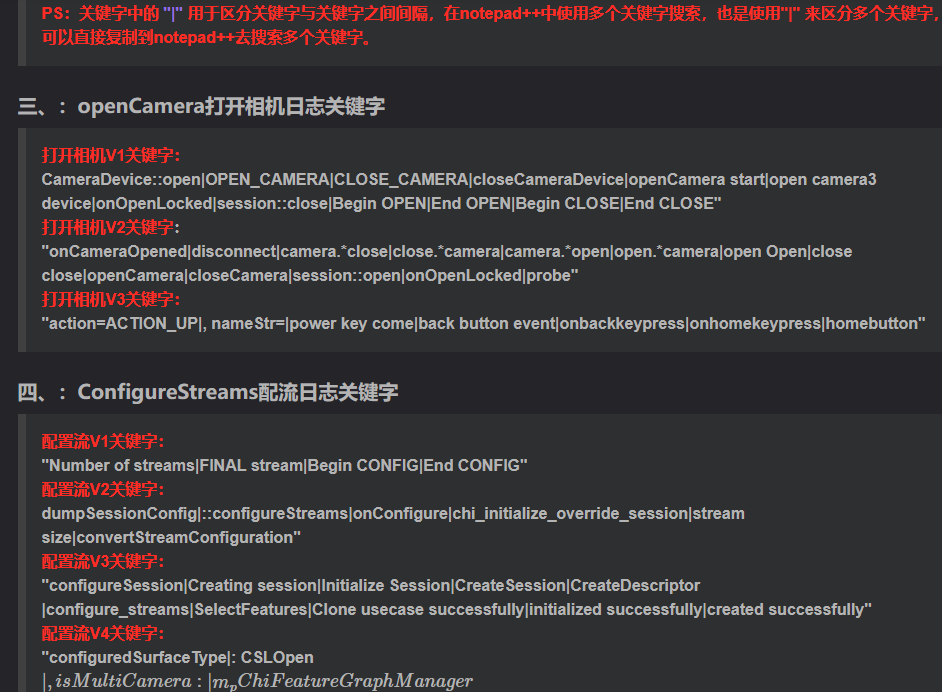
相机Camera日志实例分析之二:相机Camx【专业模式开启直方图拍照】单帧流程日志详解
【关注我,后续持续新增专题博文,谢谢!!!】 上一篇我们讲了: 这一篇我们开始讲: 目录 一、场景操作步骤 二、日志基础关键字分级如下 三、场景日志如下: 一、场景操作步骤 操作步…...
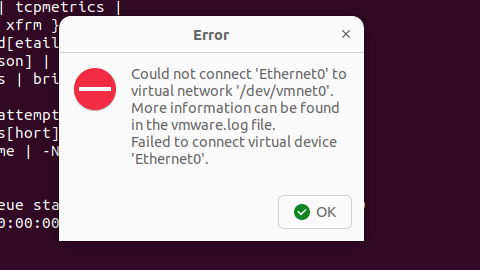
解决Ubuntu22.04 VMware失败的问题 ubuntu入门之二十八
现象1 打开VMware失败 Ubuntu升级之后打开VMware上报需要安装vmmon和vmnet,点击确认后如下提示 最终上报fail 解决方法 内核升级导致,需要在新内核下重新下载编译安装 查看版本 $ vmware -v VMware Workstation 17.5.1 build-23298084$ lsb_release…...
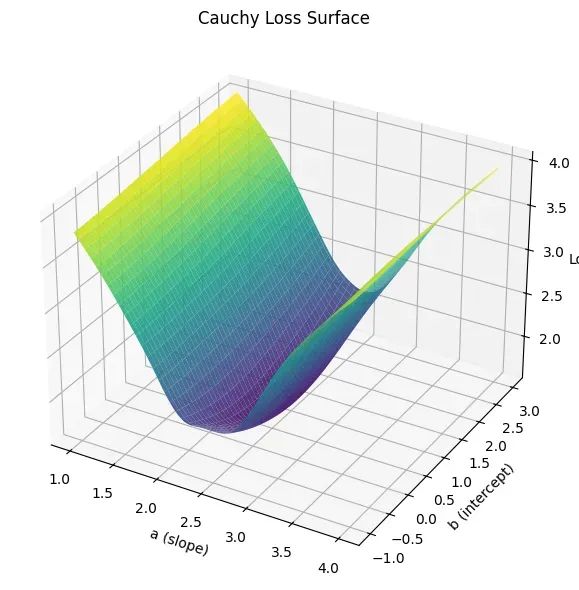
让回归模型不再被异常值“带跑偏“,MSE和Cauchy损失函数在噪声数据环境下的实战对比
在机器学习的回归分析中,损失函数的选择对模型性能具有决定性影响。均方误差(MSE)作为经典的损失函数,在处理干净数据时表现优异,但在面对包含异常值的噪声数据时,其对大误差的二次惩罚机制往往导致模型参数…...
Fabric V2.5 通用溯源系统——增加图片上传与下载功能
fabric-trace项目在发布一年后,部署量已突破1000次,为支持更多场景,现新增支持图片信息上链,本文对图片上传、下载功能代码进行梳理,包含智能合约、后端、前端部分。 一、智能合约修改 为了增加图片信息上链溯源,需要对底层数据结构进行修改,在此对智能合约中的农产品数…...
C#学习第29天:表达式树(Expression Trees)
目录 什么是表达式树? 核心概念 1.表达式树的构建 2. 表达式树与Lambda表达式 3.解析和访问表达式树 4.动态条件查询 表达式树的优势 1.动态构建查询 2.LINQ 提供程序支持: 3.性能优化 4.元数据处理 5.代码转换和重写 适用场景 代码复杂性…...
Webpack性能优化:构建速度与体积优化策略
一、构建速度优化 1、升级Webpack和Node.js 优化效果:Webpack 4比Webpack 3构建时间降低60%-98%。原因: V8引擎优化(for of替代forEach、Map/Set替代Object)。默认使用更快的md4哈希算法。AST直接从Loa…...

CVPR2025重磅突破:AnomalyAny框架实现单样本生成逼真异常数据,破解视觉检测瓶颈!
本文介绍了一种名为AnomalyAny的创新框架,该方法利用Stable Diffusion的强大生成能力,仅需单个正常样本和文本描述,即可生成逼真且多样化的异常样本,有效解决了视觉异常检测中异常样本稀缺的难题,为工业质检、医疗影像…...