ARJ_DenseNet BMR模型训练
废话不多数,模型训练代码
densenet_arj_BMR.py
:
import timefrom tensorflow.keras.applications.xception import Xception
from tensorflow.keras.applications.densenet import DenseNet169
from tensorflow.keras.preprocessing.image import ImageDataGenerator
import tensorflow.keras as keras
from arj_t.plt_graph import show_graph
from common_para import train_dir, val_dir, station, EPOCHS_1,EPOCHS_2, batch_size, CLASS_WEIGHT, classesinput_shape = (224, 224)
date_ = time.strftime('%Y%m%d', time.localtime())
cpkt_path = f'./ckpt/ARJ_Densenet_ckpt{station}_20231017-1.h5'
model_path = f'./ckpt/ARJ_Densenet_MODEL{station}_{date_}.h5'class ArjDensenetModel(object):def __init__(self):self.base_model = DenseNet169(weights='imagenet', include_top=False)# 泛化能力不行,进行图像增强测试self.train_gen = ImageDataGenerator(rescale=1.0 / 255.0,# rotation_range=45,# width_shift_range=0.2,# height_shift_range=0.2,# brightness_range=(0, 0.3),# shear_range=0.2, # 浮点数。剪切强度(以弧度逆时针方向剪切角度)# zoom_range=[0.5, 1.5], # 小于1.0的缩放将放大图像,大于1.0的缩放将缩小图像。# horizontal_flip=True,# vertical_flip=True,# fill_mode='constant',# cval=0)# self.train_gen = ImageDataGenerator(rescale=1.0 / 255.0)self.val_gen = ImageDataGenerator(rescale=1.0 / 255.0)# 获取本地训练和验证图片,生成generatordef get_local_data(self):self.train_gen = self.train_gen.flow_from_directory(directory=train_dir,target_size=input_shape,batch_size=batch_size,class_mode='binary', # binary 改为 categoricalshuffle=True,# save_to_dir=r'D:\AOI Gray Image-OA\dataset\BMR\train_trans2',# save_format='jpg',# save_prefix='trans_')self.val_gen = self.val_gen.flow_from_directory(directory=val_dir,target_size=input_shape,batch_size=batch_size,class_mode='binary', # binary 改为 categorical 2022/5/15shuffle=True)return Nonedef refine_basemode(self):"""获取VGG16 basemode只获取全连接层以前的卷积和池化层,并进行参数冻结,也就是使用原有训练好的参数自主增加隐藏层和全连接层进行训练,获得目标模型:return:"""# 获取除全连接层以外的层数,no-top modelx = self.base_model.outputs[0]# 加入全局池化、隐藏层、全连接层x = keras.layers.GlobalAveragePooling2D()(x)x = keras.layers.Dense(2048, activation='relu')(x)# x = keras.layers.BatchNormalization()(x)x = keras.layers.Dense(1024, activation='relu')(x)out = keras.layers.Dense(2, activation='softmax')(x)# 生成新的模型new_model = keras.models.Model(inputs=self.base_model.inputs, outputs=out)# 冻结vgg模型原有参数self.freeze_base_model()# 对new_model进行编译# 学习效果不佳,初始学习率加大尝试# 初始学习率0.01->0.001opt = keras.optimizers.Adam(learning_rate=0.001)new_model.compile(# optimizer=opt, # 优化器# # 因为class_mode使用了categorical, 此时返回one-hot编码标签# # 那么这里就需要使用categorical_crossentropy,多类对数交叉熵损失计算# # 如果class_mode使用binary, 此时返回1D的二值标签,loss就需要使用sparse_categorical_crossentropy# loss='sparse_categorical_crossentropy', # 使用交叉熵损失函数 分类# metrics=['accuracy']# binary_crossentropy与sigmoid联合使用二分类# categorical_crossentropy与softmax联合使用optimizer='adam',loss='sparse_categorical_crossentropy',metrics=['accuracy'])return new_model# 冻结模型训练层数def freeze_base_model(self):for layer in self.base_model.layers:layer.trainable = Falsereturn None# # 对new_model进行trainingdef fit(self, model):# 获取本地数据self.get_local_data()# 定义checkpointckpt = keras.callbacks.ModelCheckpoint(filepath=cpkt_path,monitor='val_accuracy',save_freq='epoch',save_weights_only=True,save_best_only=True)# 早停法用起来el1 = keras.callbacks.EarlyStopping(monitor='val_accuracy',patience=15,verbose=2,mode='auto')# 定义学习率缩小规则rc1 = keras.callbacks.ReduceLROnPlateau(monitor='val_accuracy',factor=0.1, # 学习率缩小倍数 new_lr = lr*factorpatience=5, # 耐心吗,5次迭代不增加就缩小学习率mode='auto',verbose=1, # 1代表更新信息,0代表不更新# epsilon=0.0001, # 确认是否进入平原区min_lr=0,cooldown=0)# 模型训练# 加入class_weight权重# 暂时注释。his1 = model.fit(self.train_gen, validation_data=self.val_gen,epochs=EPOCHS_1, callbacks=[ckpt, rc1, el1])# his1 = model.fit(self.train_gen, validation_data=self.val_gen,# epochs=EPOCHS_1, callbacks=[ckpt, rc1, el1], class_weight=CLASS_WEIGHT)print('first step end')# 解冻所有layer,进行参数微调for layer in model.layers:layer.trainable = True# 早停法用起来el2 = keras.callbacks.EarlyStopping(monitor='val_accuracy',patience=11,verbose=2,mode='auto')# 定义学习率缩小规则rc2 = keras.callbacks.ReduceLROnPlateau(monitor='val_accuracy',factor=0.1, # 学习率缩小倍数 new_lr = lr*factorpatience=5, # 耐心吗,5次迭代不增加就缩小学习率mode='auto',verbose=1, # 1代表更新信息,0代表不更新# epsilon=0.0001, # 确认是否进入平原区min_lr=0,cooldown=0)opt = keras.optimizers.Adam(learning_rate=0.001)model.compile(optimizer=opt,loss='sparse_categorical_crossentropy',metrics=['accuracy'])# 模型训练# model.load_weights(cpkt_path)his2 = model.fit(self.train_gen, validation_data=self.val_gen,epochs=EPOCHS_2, callbacks=[ckpt, rc2, el2], class_weight={0: 1, 1: 1.5})# # 模型训练# his2 = model.fit(self.train_gen, validation_data=self.val_gen,# epochs=EPOCHS_2, callbacks=[ckpt, rc2, el2], class_weight={0: 1, 1: 2, 2: 3})print('END STEP')return his1, his2if __name__ == '__main__':arj_model = ArjDensenetModel()model = arj_model.refine_basemode()his1, his2 = arj_model.fit(model)# # 保存模型# model.save(model_path)show_graph(his1)show_graph(his2)
common_para.py代码
train_dir = r"D:\new_data\BMR_TRAIN\train"
val_dir = r"D:\new_data\BMR_TRAIN\validate"
station = '_ALL_BMR'
batch_size = 32
EPOCHS_1 = 10
EPOCHS_2 = 40
CLASS_WEIGHT = {0: 1., 1: 1., 2: 1.}
threshold_value = 0
classes = 2
模型预测代码
BMR_IPS_135K_predict.py
import osimport numpy as np
from tensorflow.keras.preprocessing.image import load_img, img_to_arrayimport densenet_arj_BMR
import inceptionRestnet_arj_t
import resnet101_arj_BMR
import xception_arj_BMRMODEL_NAME = 'densenet'val_path = r'D:\AOI Gray Image-OA\dataset\case1\135K-ISR-IPS\validate'
# val_path = r'D:\AOI Gray Image-OA\dataset\error\W_to_G'
other_path = r'D:\AOI Gray Image-OA\AOI IMAGE-20220513\A6Q\ISR\复判后-G'
test_path = r'D:\new_data\BMR表外测试\BMR\A1A\P'
# TARGET_SIZE = (299, 299)
# DEFECT_TYPE = 'P'
# error_path = fr"D:\AOI Gray Image-OA\dataset\BMR\{MODEL_NAME}"def get_ckptpath_model():# arj_model = tensorflow.keras.models.Model()ckpt_path = ''# target_size = (224, 224)if MODEL_NAME == 'xception':ckpt_path = xception_arj_BMR.cpkt_patharj_model = xception_arj_BMR.ArjResnet101Model()target_size = xception_arj_BMR.input_shapeelif MODEL_NAME == 'inceptionRestnet':ckpt_path = inceptionRestnet_arj_t.cpkt_patharj_model = inceptionRestnet_arj_t.ArjInceptionRestnetModel()target_size = inceptionRestnet_arj_t.input_shapeelif MODEL_NAME == 'densenet':ckpt_path = densenet_arj_BMR.cpkt_patharj_model = densenet_arj_BMR.ArjDensenetModel()target_size = densenet_arj_BMR.input_shapeelif MODEL_NAME == 'resnet101':ckpt_path = resnet101_arj_BMR.cpkt_patharj_model = resnet101_arj_BMR.ArjResnet101Model()target_size = resnet101_arj_BMR.input_shapereturn ckpt_path, arj_model, target_size# 获取想要预测的图片绝对路径,包含文件名
def get_img_paths(defect_type, path):img_path = os.path.join(path, defect_type)img_paths = []for root, dirs, files in os.walk(img_path):for file in files:# print(file[-3:])if file[-3:] == 'jpg':img_paths.append(os.path.join(root, file))return img_pathsdef bmr_ips_predict(img_paths, error_path, defect_type='G'):ckpt_path, arj_model, input_shape = get_ckptpath_model()model = arj_model.refine_basemode()print(ckpt_path)model.load_weights(ckpt_path)print(model.summary())predict_dict = {0: 'G', 1: 'P', 2: 'W'}# 加载图片,预测white_cnt = 0good_cnt = 0repair_cnt = 0threshold_ls = []for img_path in img_paths:img_arr = load_img(img_path, target_size=input_shape)img = img_arr# print(img_path)# 转化为矩阵img_arr = img_to_array(img_arr)# print(img.shape)# 归一化# img_arr = preprocess_input(img_arr)img_arr /= 255.# print(type(img_arr))# img_arr = preprocess_input(img_arr)# img_arr /= 127.5# img_arr -= 1.# 形状修改img_arr = img_arr.reshape(1, img_arr.shape[0], img_arr.shape[1], img_arr.shape[2])# print(img.shape)# print(img_arr)y_predict = model.predict(img_arr)index = np.argmax(y_predict)# 加入阈值threshold = y_predict[0][index]# print(img_path.split('\\')[-1])# print(y_predict[0], ' >> ', threshold)# threshold_ls.append(threshold)# print(y_predict)y_predict = predict_dict[index]# print(index)# print(y_predict)# if index == 0:# good_cnt += 1# else:# repair_cnt += 1# 保存判错的图片# 预测结果G# save_img_name = str(round(threshold,2))+'_'+img_path.split('\\')[-1]save_img_name = img_path.split('\\')[-1]if index == 0:# 加入阈值调节判G能力if threshold > 0:good_cnt += 1# print(good_cnt)# print(img_path[-10:])# 如果原本P文件夹if defect_type == 'P':threshold_ls.append(threshold)img.save(os.path.join(error_path, 'AI_P_TO_G', save_img_name))os.remove(img_path)# 如果原本W文件夹if defect_type == 'W':img.save(os.path.join(error_path, 'AI_W_TO_G', save_img_name))os.remove(img_path)else:repair_cnt += 1elif index == 1:repair_cnt += 1if defect_type == 'G':threshold_ls.append(threshold)img.save(os.path.join(error_path, 'AI_G_TO_P', save_img_name))os.remove(img_path)if defect_type == 'W':img.save(os.path.join(error_path, 'AI_W_TO_P', save_img_name))os.remove(img_path)elif index == 2:white_cnt += 1if defect_type == 'G':img.save(os.path.join(error_path, 'AI_G_TO_W', save_img_name))os.remove(img_path)if defect_type == 'P':img.save(os.path.join(error_path, 'AI_P_TO_W', save_img_name))os.remove(img_path)else:print('还有第四种可能??!!')# print(y_predict)# print('**************************')# pd.DataFrame(data=threshold_ls).to_csv('./threshold.csv', encoding='utf-8')print(threshold_ls)print('good_cnt : %d' % good_cnt)print('repair_cnt : %d' % repair_cnt)# print('white_cnt : %d' % white_cnt)# if __name__ == '__main__':
# paths = get_img_paths(DEFECT_TYPE, test_path)
# bmr_ips_predict(paths,error_path)
模型总预测代码
predict_all.py
import BMR_IPS_135K_predict# 此程序用来进行所有模型预测2023/10/17img_path = r'D:\new_data\BMR_TRAIN\test\WHITE'DEFECT_TYPE = 'P'paths = BMR_IPS_135K_predict.get_img_paths(DEFECT_TYPE, img_path)BMR_IPS_135K_predict.bmr_ips_predict(paths, img_path, DEFECT_TYPE)
相关文章:
ARJ_DenseNet BMR模型训练
废话不多数,模型训练代码 densenet_arj_BMR.py : import timefrom tensorflow.keras.applications.xception import Xception from tensorflow.keras.applications.densenet import DenseNet169 from tensorflow.keras.preprocessing.image import Im…...
React之Hook
一、是什么 Hook 是 React 16.8 的新增特性。它可以让你在不编写 class 的情况下使用 state 以及其他的 React 特性 至于为什么引入hook,官方给出的动机是解决长时间使用和维护react过程中常遇到的问题,例如: 难以重用和共享组件中的与状态…...
OSG嵌入QT的简明总结2
正文 我之前在这篇博文《OSG嵌入QT的简明总结》中论述了OSG在QT中显示的可视化问题。其中提到官方提供的osgQt项目(地址:https://github.com/openscenegraph/osgQt )很久前已经更新了。但是我一直没有时间同步更新,最近重新尝试了…...
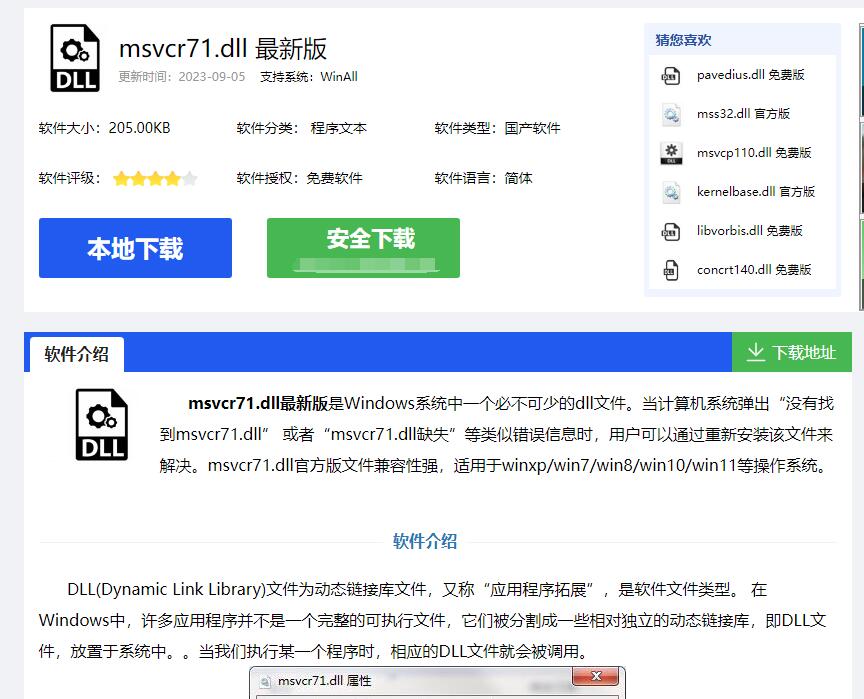
日常中msvcp71.dll丢失怎样修复?分享5个修复方法
在 Windows 系统中,msvcp71.dll 是一个非常重要的动态链接库文件,它承载了许多应用程序和游戏的运行。如果您的系统中丢失了这个文件,那么您可能会遇到无法打开程序、程序崩溃或出现错误提示等问题。本文将介绍 5 个快速修复 msvcp71.dll 丢失…...
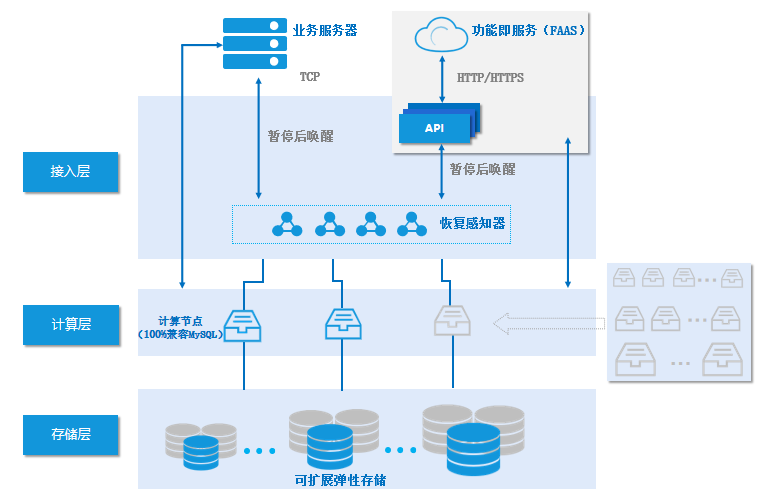
【腾讯云TDSQL-C Serverless 产品体验】使用 Python向TDSQL-C添加读取数据实现词云图
关于TDSQL-C Serverless介绍 TDSQL-C 是腾讯云自主研发的新一代云原生关系型数据库。 它融合了传统数据库、云计算和新硬件技术的优势,100%兼容 MySQL,为用户提供具有极致弹性、高性能、高可用性、高可靠性和安全性的数据库服务。 TDSQL-C 实现了超过百万每秒的高吞吐量,支持…...
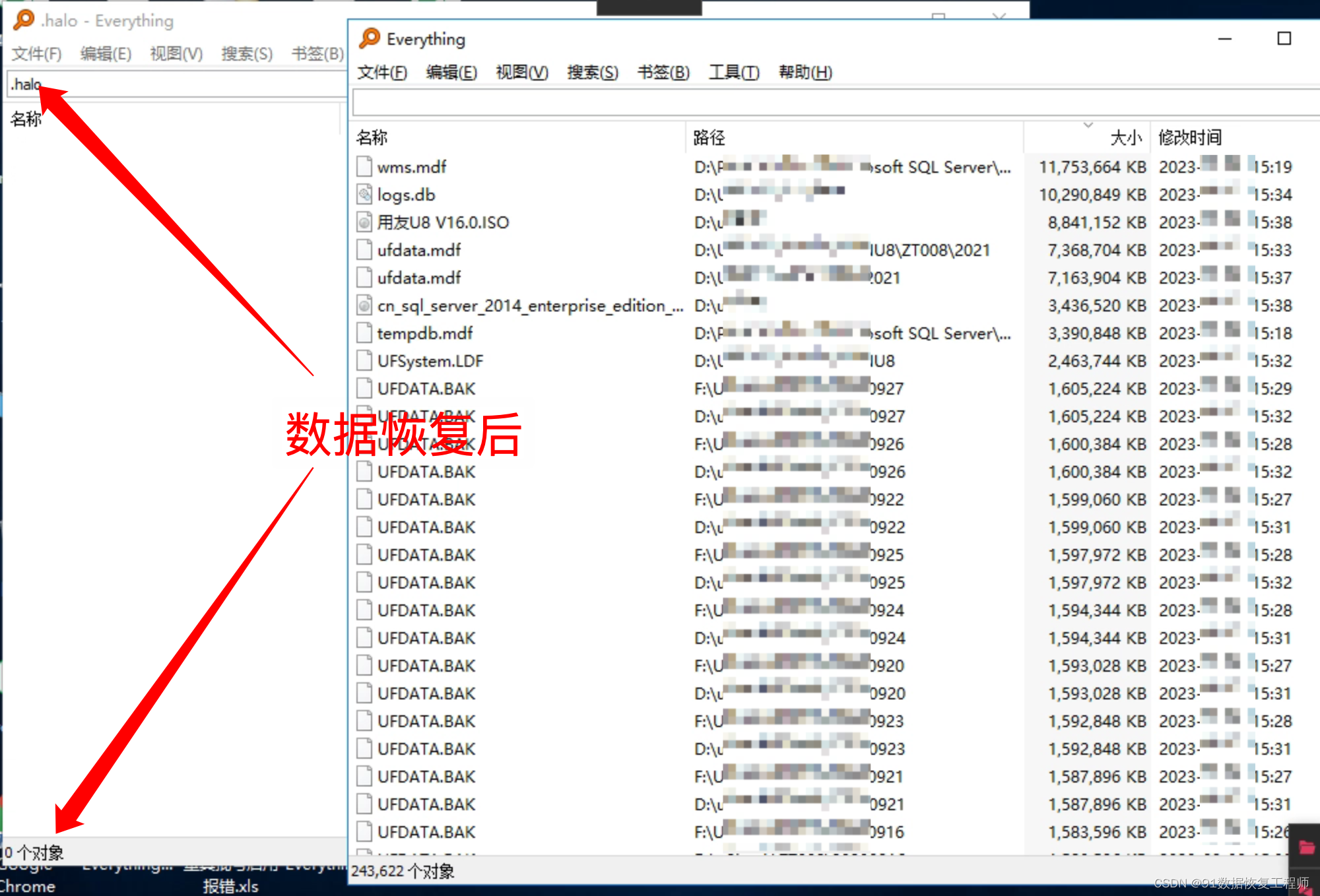
服务器感染了.360、.halo勒索病毒,如何确保数据文件完整恢复?
导言: 数据的安全性至关重要,但威胁不断进化,.360、.halo勒索病毒是其中的令人担忧的勒索软件。本文91数据恢复将深入介绍.360、.halo勒索病毒,包括其威胁本质、数据恢复方法和如何采取预防措施来保护您的数据。 如果受感染的数据…...
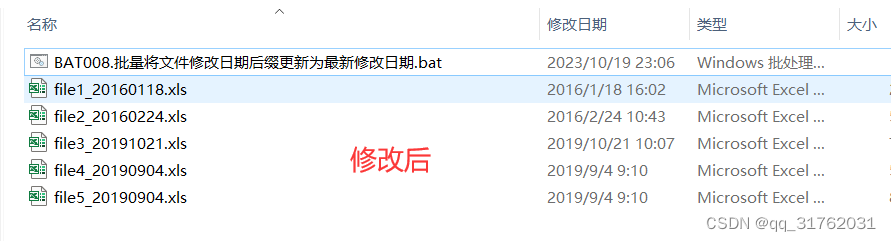
BAT028:批量将文件修改日期后缀更新为最新修改日期
引言:编写批处理程序,实现批量将文件修改日期后缀更新为最新修改日期。 一、新建Windows批处理文件 参考博客: CSDNhttps://mp.csdn.net/mp_blog/creation/editor/132137544 二、写入批处理代码 1.右键新建的批处理文件,点击【…...
Visual Studio C++ 的 头文件和源文件
在Visual Studio C中,头文件(Header Files)和源文件(Source Files)是两种不同的文件类型,用于组织和管理C代码。 头文件(Header Files): 后缀名为.h或.hpp的文件…...
Scrapy框架中的Middleware扩展与Scrapy-Redis分布式爬虫
在爬虫开发中,Scrapy框架是一个非常强大且灵活的选择。在本文中,我将与大家分享两个关键的主题:Scrapy框架中的Middleware扩展和Scrapy-Redis分布式爬虫。这些主题将帮助你更好地理解和应用Scrapy框架,并提升你的爬虫开发技能。 …...
[论文笔记]Sentence-BERT[v2]
引言 本文是SBERT(Sentence-BERT)论文1的笔记。SBERT主要用于解决BERT系列模型无法有效地得到句向量的问题。很久之前写过该篇论文的笔记,但不够详细,今天来重新回顾一下。 BERT系列模型基于交互式计算输入两个句子之间的相似度是非常低效的(但效果是很好的)。当然可以通过…...

虚拟机ubantu系统突然重启失去网络
1.进入 root用户 cd /var/lib/NetworkManager然后查看网络服务状态 如果网络状态和我一样不可用 ,就先停止网络服务 service ModemManager stop#删除状态rm networker.stateservice ModemManager start此时右上交的网络标志回复正常...
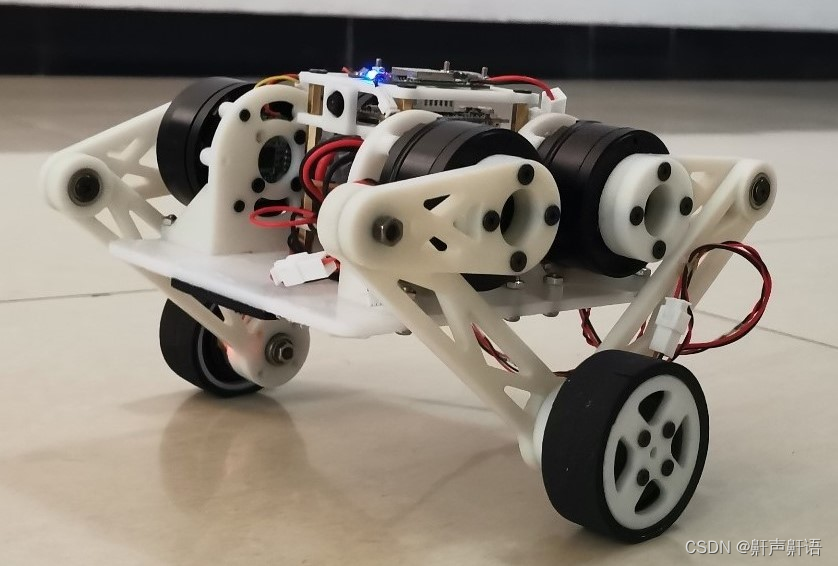
三款经典的轮式/轮足机器人讲解,以及学习EG2133产生A/B/C驱动电机。个人机器人学习和开发路线(推荐)
1,灯哥开源(有使用指南,适合刚入门新手) 机械部分:2个foc无刷电机 硬件和软件部分:没有驱动板子。只有驱动器,主控板esp32和驱动器通过pwm直接通讯。驱动器板子上有蓝色电机接口,直…...
apache开启https
本文基于windows平台。 个人感觉使用apache配置起来比较繁琐,而使用upupw或者xmpp等集成开发工具更方便。 在httpd.conf中,将下一行的注释去掉:LoadModule ssl_module modules/mod_ssl.so。另外,千万不要注释掉下面的一行&#…...
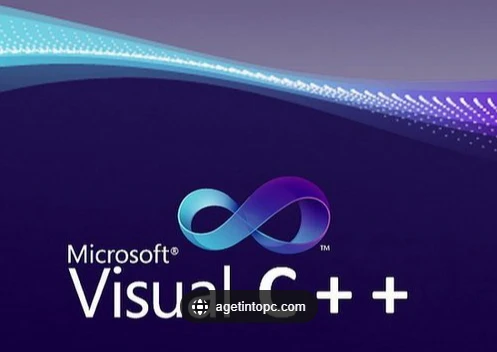
绝地求生游戏缺少msvcp140.dll丢失打不开怎么办?这6个方法都能修复
计算机系统中,我们经常遇到各种错误和问题。其中,“MSCVCP140.DLL丢失”是一个常见的错误,它通常出现在运行某些程序或游戏时。这个DLL文件是Microsoft Visual C 2015 Redistributable的一部分,如果它丢失或损坏,可能会…...
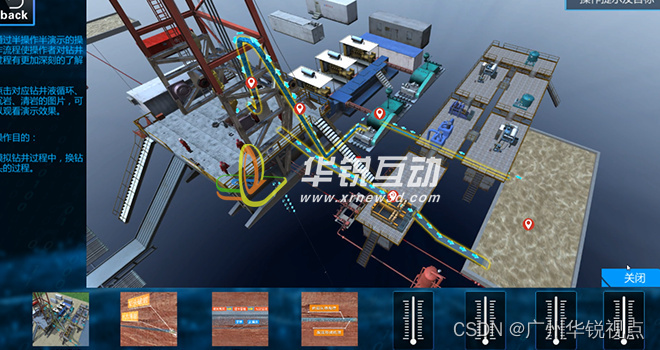
【广州华锐互动】石油钻井井控VR互动实训系统
随着科技的不断发展,虚拟现实(VR)技术已经逐渐渗透到各个领域,为人们的生活和工作带来了前所未有的便利。在石油钻井行业,VR技术的应用也日益受到重视,为钻井工人提供了更加安全、高效的培训方式。 广州华锐…...
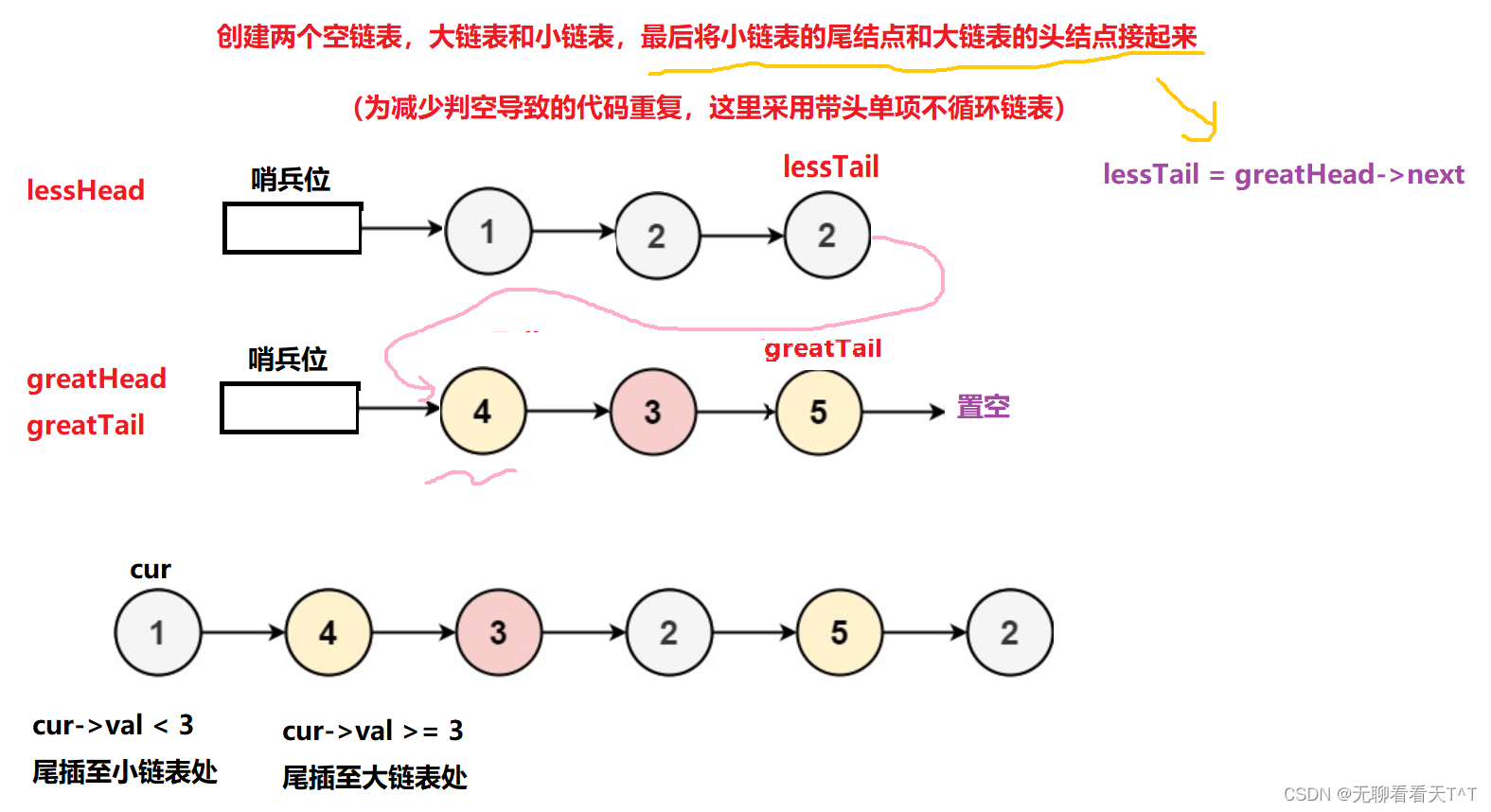
单链表算法经典OJ题
目录 1、移除链表元素 2、翻转链表 3、合并两个有序链表 4、获取链表的中间结点 5、环形链表解决约瑟夫问题 6、分割链表 1、移除链表元素 203. 移除链表元素 - 力扣(LeetCode) typedef struct ListNode LSNode; struct ListNode* remove…...
Picnic master project interview
picnic Picnic master project interview1. Topics1.1 Systematically identify similar/interchangeable articles1.2 Understanding changing customer behaviour 2. interview等后续 Picnic master project interview 1. Topics 1.1 Systematically identify similar/inte…...
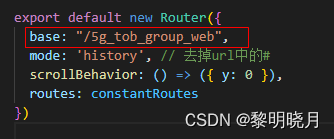
nginx部署vue项目(访问路径加前缀)
nginx部署vue项目(访问路径加前缀) nginx部署vue项目,访问路径加前缀分为两部分: (1)修改vue项目; (2)修改nginx配置; vue项目修改 需注意,我这是vue-cli3配置&#x…...
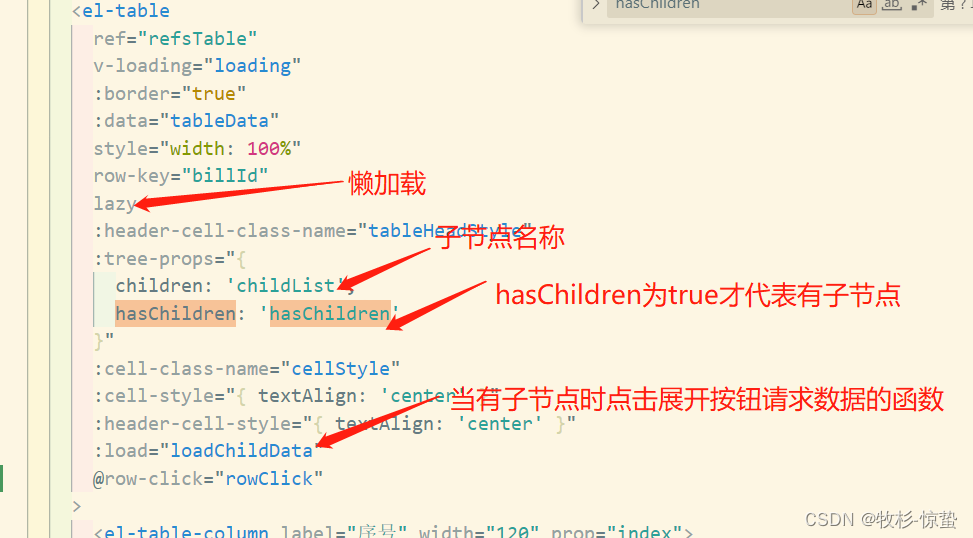
element-ui中表格树类型数据的显示
项目场景: 1:非懒加载的情况 1:效果展示 2:问题描述以及解决 1:图片展示 2:html <-- default-expand-all 代表默认展开 如果不展开删除就行 --> <el-tableref"refsTable"v-loadin…...
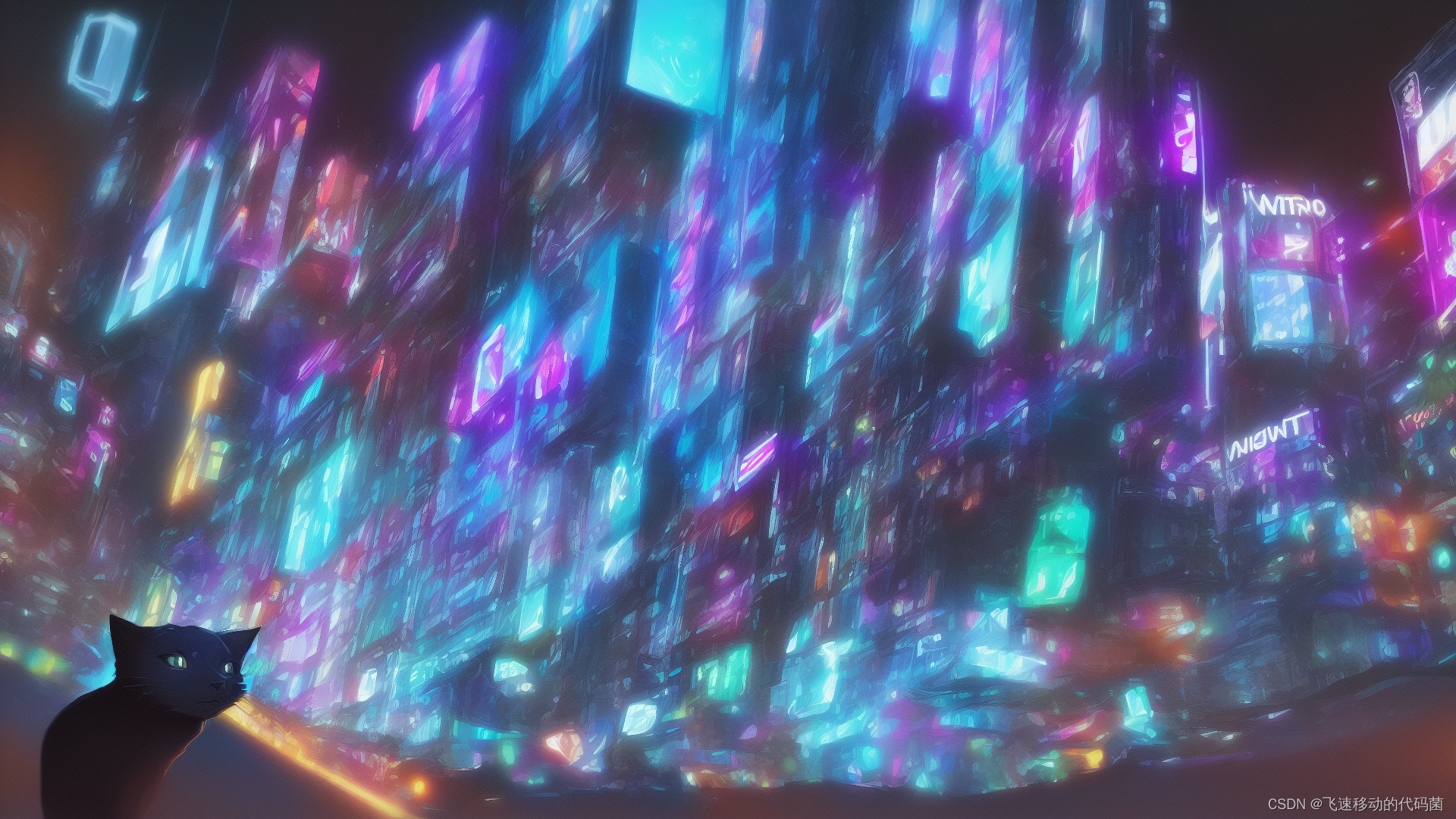
【扩散模型】如何用最几毛钱生成壁纸
通过学习扩散模型了解到了统计学的美好,然后顺便记录下我之前文生图的基础流程~ 扩散模型简介 这次是在DataWhale的组队学习里学习的,HuggingFace开放扩散模型学习地址 扩散模型训练时通过对原图增加高斯噪声,在推理时通过降噪来得到原图&…...
Ubuntu系统下交叉编译openssl
一、参考资料 OpenSSL&&libcurl库的交叉编译 - hesetone - 博客园 二、准备工作 1. 编译环境 宿主机:Ubuntu 20.04.6 LTSHost:ARM32位交叉编译器:arm-linux-gnueabihf-gcc-11.1.0 2. 设置交叉编译工具链 在交叉编译之前&#x…...
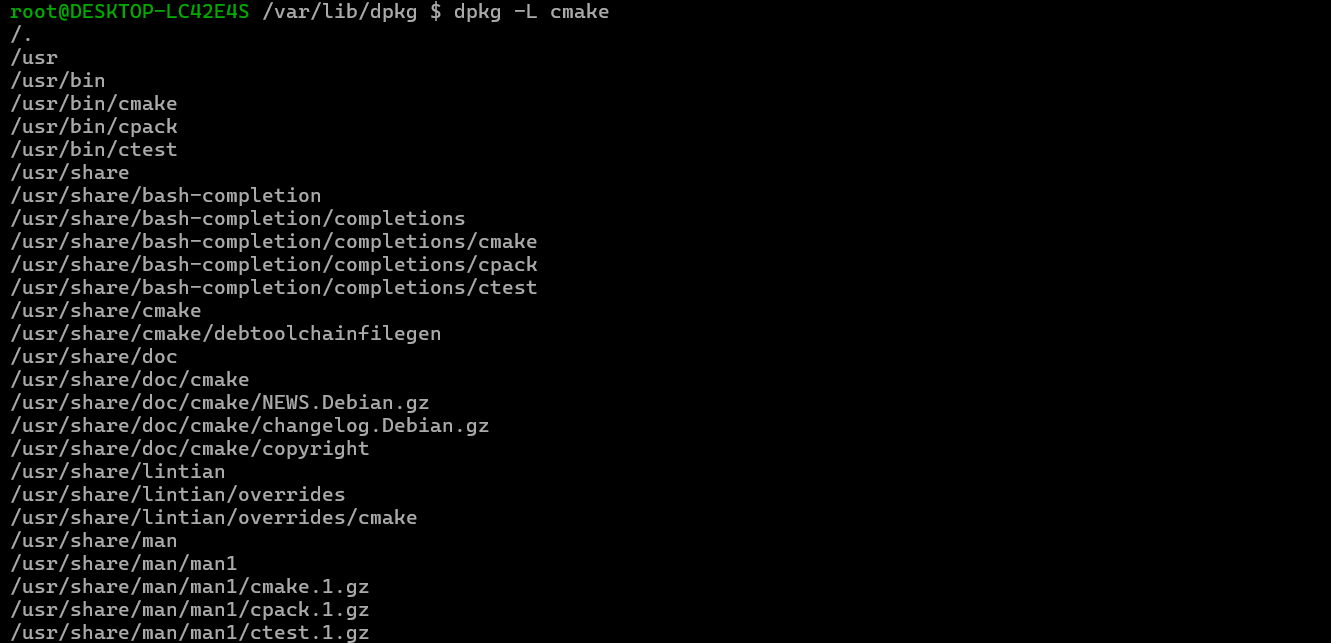
Debian系统简介
目录 Debian系统介绍 Debian版本介绍 Debian软件源介绍 软件包管理工具dpkg dpkg核心指令详解 安装软件包 卸载软件包 查询软件包状态 验证软件包完整性 手动处理依赖关系 dpkg vs apt Debian系统介绍 Debian 和 Ubuntu 都是基于 Debian内核 的 Linux 发行版ÿ…...
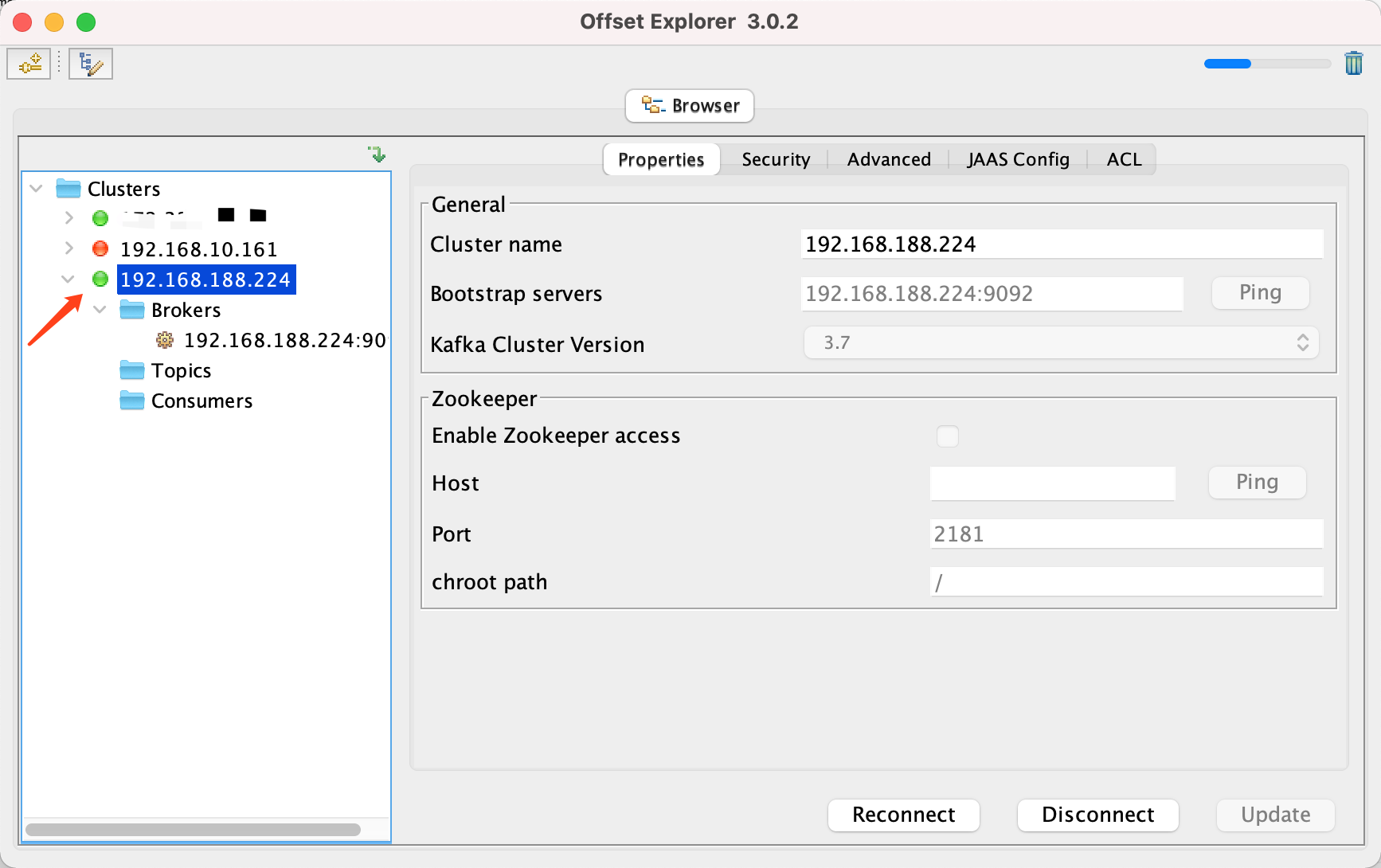
Docker 运行 Kafka 带 SASL 认证教程
Docker 运行 Kafka 带 SASL 认证教程 Docker 运行 Kafka 带 SASL 认证教程一、说明二、环境准备三、编写 Docker Compose 和 jaas文件docker-compose.yml代码说明:server_jaas.conf 四、启动服务五、验证服务六、连接kafka服务七、总结 Docker 运行 Kafka 带 SASL 认…...
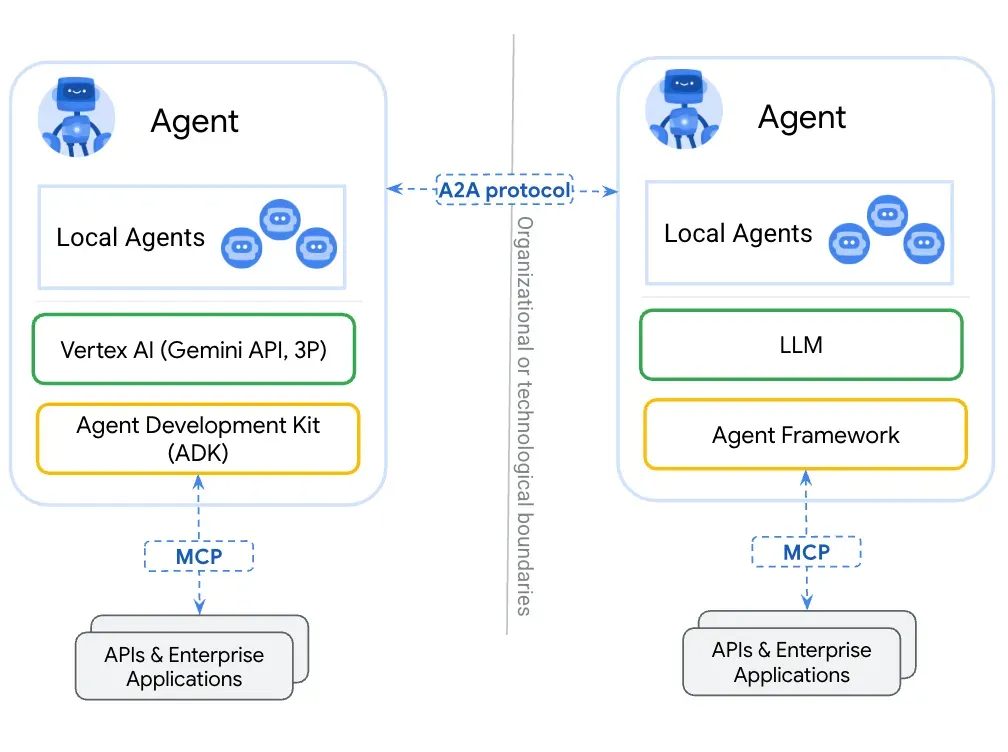
第一篇:Agent2Agent (A2A) 协议——协作式人工智能的黎明
AI 领域的快速发展正在催生一个新时代,智能代理(agents)不再是孤立的个体,而是能够像一个数字团队一样协作。然而,当前 AI 生态系统的碎片化阻碍了这一愿景的实现,导致了“AI 巴别塔问题”——不同代理之间…...
聊一聊接口测试的意义有哪些?
目录 一、隔离性 & 早期测试 二、保障系统集成质量 三、验证业务逻辑的核心层 四、提升测试效率与覆盖度 五、系统稳定性的守护者 六、驱动团队协作与契约管理 七、性能与扩展性的前置评估 八、持续交付的核心支撑 接口测试的意义可以从四个维度展开,首…...
rnn判断string中第一次出现a的下标
# coding:utf8 import torch import torch.nn as nn import numpy as np import random import json""" 基于pytorch的网络编写 实现一个RNN网络完成多分类任务 判断字符 a 第一次出现在字符串中的位置 """class TorchModel(nn.Module):def __in…...
SQL慢可能是触发了ring buffer
简介 最近在进行 postgresql 性能排查的时候,发现 PG 在某一个时间并行执行的 SQL 变得特别慢。最后通过监控监观察到并行发起得时间 buffers_alloc 就急速上升,且低水位伴随在整个慢 SQL,一直是 buferIO 的等待事件,此时也没有其他会话的争抢。SQL 虽然不是高效 SQL ,但…...

Unity UGUI Button事件流程
场景结构 测试代码 public class TestBtn : MonoBehaviour {void Start(){var btn GetComponent<Button>();btn.onClick.AddListener(OnClick);}private void OnClick(){Debug.Log("666");}}当添加事件时 // 实例化一个ButtonClickedEvent的事件 [Formerl…...
深入浅出Diffusion模型:从原理到实践的全方位教程
I. 引言:生成式AI的黎明 – Diffusion模型是什么? 近年来,生成式人工智能(Generative AI)领域取得了爆炸性的进展,模型能够根据简单的文本提示创作出逼真的图像、连贯的文本,乃至更多令人惊叹的…...
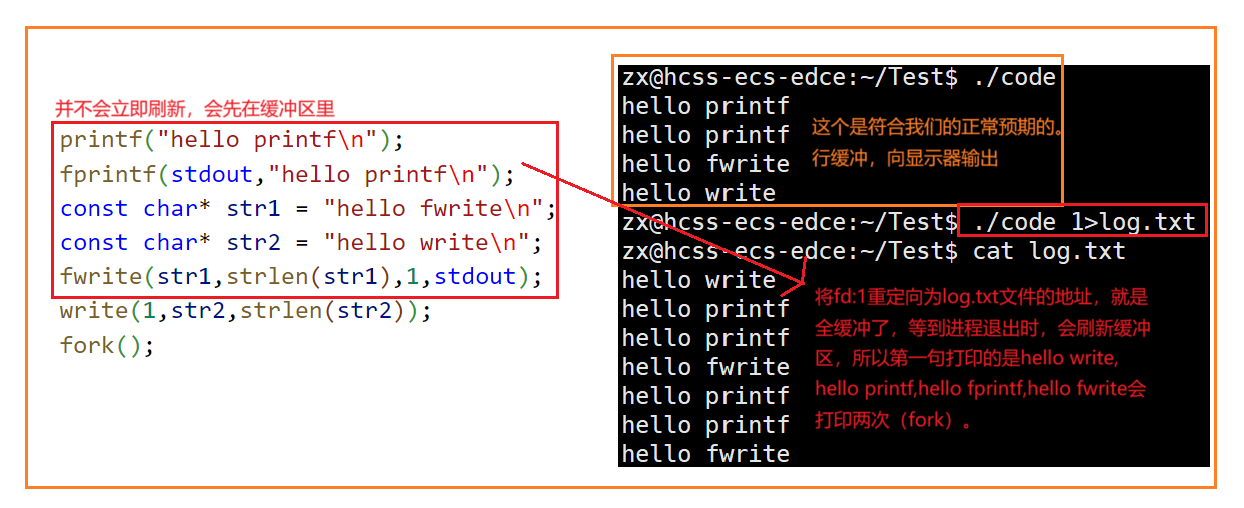
Linux中《基础IO》详细介绍
目录 理解"文件"狭义理解广义理解文件操作的归类认知系统角度文件类别 回顾C文件接口打开文件写文件读文件稍作修改,实现简单cat命令 输出信息到显示器,你有哪些方法stdin & stdout & stderr打开文件的方式 系统⽂件I/O⼀种传递标志位…...