头颈肿瘤在PET/CT中的分割:HECKTOR挑战赛| 文献速递-深度学习肿瘤自动分割
Title
题目
Head and neck tumor segmentation in PET/CT: The HECKTOR challenge
头颈肿瘤在PET/CT中的分割:HECKTOR挑战赛
01
文献速递介绍
高通量医学影像分析,常被称为放射组学,已显示出其在揭示定量影像生物标志物与癌症预后之间关系的潜力,包括在头颈(H&N)癌症的背景下(Vallieres等,2017;Bogowicz等,2017)。头颈癌是发病率第五高的癌症(Parkin等,2005),其治疗通常基于放射治疗与系统治疗(例如赛妥昔单抗)的组合(Bonner等,2010)。然而,治疗这种癌症仍具挑战性,因为大约40%的患者在治疗后的前两年内会发生局部失败(Chajon等,2013)。开发非侵入性和个性化的方法(例如放射组学)对于改善疾病特征化至关重要,并有望导致基于表型肿瘤特征的更有针对性的治疗。2-[18F]氟代脱氧葡萄糖正电子发射断层扫描(FDG-PET)和计算机断层扫描(CT)在疾病特征化中占有特殊地位,因为它们包含有关癌症的代谢和解剖的互补信息。此外,它们用于头颈癌的初步分期和随访。因此,这些模式易于用于基于临床获得的图像创建和评估放射组学模型。典型的放射组学分析依赖于在已勾画的病变或兴趣体积(VOI)内的局部特征提取(Lambin等,2017;Gillies等,2016)。
阻碍强大模型开发的原因之一是耗时且容易出错的手动勾画这些VOI。为此,自动分割头颈原发肿瘤(GTVt)和淋巴结(GTVn)的总肿瘤体积构成了一种非常有前景的方法,用于标注和分析非常大的队列,这对于实现放射组学模型的强大和可重复性验证至关重要。此外,自动分割还有潜力让放射肿瘤科医生通过减少肿瘤勾画所需时间以及改善观察者间的再现性,来提高治疗计划的效率。
头颈肿瘤(HECKTOR)挑战赛的目标是建立并评估最佳性能方法,用于头颈病变分割,同时利用联合PET/CT的丰富的双模态信息。在这一挑战赛的首届中,参与者被要求开发用于分割患有口咽癌患者FDG-PET/CT图像上的GTVt2的自动方法。值得注意的是,要成为正式排名的一部分,参与者必须提供一篇描述其方法的论文。
Abstract
摘要
This paper relates the post-analysis of the first edition of the HEad and neCK TumOR (HECKTOR) challenge. This challenge was held as a satellite event of the 23rd International Conference on Medical ImageComputing and Computer-Assisted Intervention (MICCAI) 2020, and was the first of its kind focusing onlesion segmentation in combined FDG-PET and CT image modalities. The challenge’s task is the automatic segmentation of the Gross Tumor Volume (GTV) of Head and Neck (H&N) oropharyngeal primarytumors in FDG-PET/CT images. To this end, the participants were given a training set of 201 cases fromfour different centers and their methods were tested on a held-out set of 53 cases from a fifth center.The methods were ranked according to the Dice Score Coefficient (DSC) averaged across all test cases. Anadditional inter-observer agreement study was organized to assess the difficulty of the task from a human perspective. 64 teams registered to the challenge, among which 10 provided a paper detailing theirapproach. The best method obtained an average DSC of 0.7591, showing a large improvement over ourproposed baseline method and the inter-observer agreement, associated with DSCs of 0.6610 and 0.61, respectively. The automatic methods proved to successfully leverage the wealth of metabolic and structuralproperties of combined PET and CT modalities, significantly outperforming human inter-observer agree
这篇论文讲述了第一届头颈肿瘤(HECKTOR)挑战赛的后期分析。该挑战赛作为第23届国际医学影像计算和计算机辅助干预会议(MICCAI 2020)的一个卫星活动举行,是首次专注于联合FDG-PET和CT影像模式的病变分割。挑战的任务是自动分割头颈(H&N)口咽部原发肿瘤的总肿瘤体积(GTV)在FDG-PET/CT图像中。为此,参与者获得了来自四个不同中心的201例训练集,他们的方法在第五个中心保留的53例病例上进行了测试。
方法根据所有测试病例的Dice得分系数(DSC)平均值进行排名。此外,还组织了一项观察者间一致性研究,以评估任务的难度从人类的角度来看。共有64支队伍注册参加挑战赛,其中10支提供了详细描述他们方法的论文。最佳方法获得了平均DSC为0.7591,较我们提出的基线方法和观察者间一致性的DSC分别为0.6610和0.61,显示出显著的改进。自动化方法成功地利用了联合PET和CT模式的丰富代谢和结构特性,显著超过了人类观察者间的一致性。
Conclusion
结论
This paper presents the HECKTOR 2020 challenge on the segmentation of the primary tumor of oropharyngeal H&N cancer inFDG PET/CT. Detailed information was reported on the dataset, participation, and segmentation performance. Good participation with18 teams and 10 participants’ publications allowed us to comparestate-of-the-art segmentation methods on this challenging task.The results are very satisfactory with the winning team achievingan average DSC of 0.7591, which is superior to the inter-observeragreement (average DSC 0.6110). These results were obtained witha strict testing scheme as the test cases were all from an unseencenter. It is reasonable to expect better results if the proposedmethods are fine-tuned on few examples from this center. All participants used U-Net based deep learning models, most of themwith a 3D architecture and standard pre-processing techniques.
本文介绍了HECKTOR 2020挑战赛,这是关于FDG PET/CT中口咽部头颈癌原发肿瘤的分割。文章详细报告了数据集、参与情况和分割性能。良好的参与度,有18支团队和10位参与者的出版物,使我们能够在这一具有挑战性的任务上比较最先进的分割方法。
结果非常令人满意,获胜团队的平均DSC为0.7591,优于观察者间一致性(平均DSC为0.6110)。这些结果是在严格的测试方案下获得的,因为测试案例全部来自一个未见过的中心。如果在这个中心的少数样本上对所提方法进行微调,可以合理预期会有更好的结果。所有参与者都使用了基于U-Net的深度学习模型,其中大多数采用了3D架构和标准的预处理技术。
Results
结果
This section regroups results in terms of challenge participation, algorithms used, segmentation performance, inter-observer agree ment, ensembling “super-algorithm”, simple PET thresholding, the relation between tumor size and segmentation performance, false positive analysis, and alternative ranking of the methods.
这部分汇总了挑战赛的参与情况、使用的算法、分割性能、观察者间一致性、集成“超级算法”、简单的PET阈值设定、肿瘤大小与分割性能之间的关系、假阳性分析以及方法的替代排名。
Figure
图
Fig. 1. Case examples of 2D sagittal slices of fused PET/CT images from each of the five centers. These images are obtained after resampling the PET image and the CT image to 1x1x1 mm3 with a tricubic interpolation. The CT window in Hounsfield unit is [−140, 260] and the PET window in SUV is .
图 1. 来自五个中心的融合PET/CT图像的2D矢状切片案例示例。这些图像在将PET图像和CT图像重新采样到1x1x1 mm³,并使用三次立方插值后获得。CT窗口的赫氏单位为[−140, 260],PET窗口的SUV为。
Fig. 2. Examples of results of the winning algorithm (andrei.iantsen (Iantsen et al., 2021b)). The automatic segmentation results (green) and ground truth annotations(red) are displayed on 2D slices of PET (right) and CT (left) images. The reported DSC is computed on the entire image (see Eq. 1). (a), (b) Excellent segmentation results,detecting the GTVt of the primary oropharyngeal tumor localized at the bse of the tongue and discarding the laterocervical lymph nodes despite high FDG uptake onPET. (c) Incorrect segmentation of the top volume at the level of the soft palate; (d) Incorrect segmentation of the smaller volume below the level of the hyoid bone.
图 2. 获胜算法的结果示例(andrei.iantsen (Iantsen等,2021b))。自动分割结果(绿色)和地面真实标注(红色)显示在PET(右侧)和CT(左侧)图像的2D切片上。报告的DSC是在整个图像上计算的(见公式1)。(a) 和 (b) 优秀的分割结果,检测到位于舌根的口咽部原发肿瘤的GTVt,并且尽管PET上FDG摄取量高,也排除了侧颈淋巴结。(c) 在软腭层面的顶部体积分割不正确;(d) 在舌骨下层面的较小体积分割不正确。
Fig. 3. Box plots of the distribution of the 53 test DSCs for each participant, ordered by decreasing rank.
图 3. 按降序排列的每位参与者的53个测试DSC分布的箱形图。
Fig. 4. Box plots of the distribution of DSCs across the 10 participants for each of the 53 patients in the test set.
图 4. 测试集中每位患者的10名参与者DSC分布的箱形图。
Fig. 5. Segmentation performance of PET thresholding-based method at different percentages of maximum SUV. Three results are reported: the automatic PET threshold, thesemi-automatic PET threshold (indicating the location of the ground truth GTVt), and the semi-automatic PET and CT (for removing the air) threshold.
图 5. 基于不同最大SUV百分比的PET阈值法的分割性能。报告了三个结果:自动PET阈值、半自动PET阈值(指示地面真实GTVt的位置),以及半自动PET和CT(用于去除空气)阈值。
Fig. 6. Scatter plot of DSC vs. tumor volume (voxel count in the VOI) for 10 participants. The corresponding Spearman correlation is 0.43.
图 6。10名参与者中DSC与肿瘤体积(感兴趣区域内的体素计数)的散点图。相应的Spearman相关系数为0.43。
Fig. 7. Average DSC of each team’s algorithm in function of the volume of the tumors. This figure was generated by distributing the 53 test volumes in 4 bins of n =13, 13,13, and 14 each and then computing the average DSC for each bin.
图 7. 每个团队算法的平均DSC与肿瘤体积的关系。此图通过将53个测试体积分布在每个包含13, 13, 13和14个体积的4个区间中,然后计算每个区间的平均DSC生成。
Fig. 8. Histogram of the Euclidean distance of the FP voxels to the closest ground truth GTVt voxel and GTVn voxel. We evaluate here the prediction of the first rankedparticipant (andrei.iantsen) (a) and our baseline 3D PET/CT (b). For comparison, the False Discovery Rate (FDR), i.e. FP/(FP+TP) is 0.15, with 544,343 TPs in (a) and FDR= 0.37 with 621,413 TPs in (b).
图 8. 假阳性体素到最近的地面真实GTVt体素和GTVn体素的欧几里得距离的直方图。这里我们评估第一名参与者(andrei.iantsen)的预测结果(a)和我们的基线3D PET/CT(b)。作为比较,假发现率(FDR),即 FP/(FP+TP) 在 (a) 中为0.15,有544,343个TPs,在 (b) 中为0.37,有621,413个TPs。
Fig. 9. Ranking robustness against changes in test data. The robustness is assessed by ranking 1000 bootstraps of the test set. The size of the circles is proportional tothe number of times a team obtained the corresponding rank for each bootstrap. The dashed lines represent the confidence intervals at 95% computed from the bootstrapanalysis. The current ranking, i.e. the one used in this challenge, is obtained by averaging the DSCs across all test cases. The alternative ranking is computed by averagingthe rankings of each team across the test cases.
图 9. 排名对测试数据变化的稳健性。通过对测试集的1000个自助样本进行排名来评估稳健性。圆圈的大小与每个自助样本中团队获得相应排名的次数成正比。虚线代表由自助分析计算出的95%置信区间。当前排名,即本挑战赛中使用的排名,是通过平均所有测试案例的DSCs获得的。替代排名是通过平均每个团队在测试案例中的排名计算得出的。
Table
表
Table 1List of scanners used in the different centers.
表 1 不同中心使用的扫描仪列表。
Table 2Summary of the algorithms in terms of main components used: 2D or 3D U-Net, resampling, preprocessing, training or testing data augmentation, loss used for optimization,an ensemble of multiple models for test prediction and postprocessing of the results. We use the following abbreviations for the preprocessing: Clipping (C), Standardization(S), and if it is applied only to one modality, it is specified in parentheses. For the image resampling, we specify whether the algorithms use Isotropic (I) or Anisotropic (A)resampling and Nearest Neighbor (NN), Linear (L), or Cubic (Cu) interpolation. We use the following abbreviation for the losses: Cross-Entropy (CE), Mumford-Shah (MS),and Mean Absolute Error (MAE). More details can be found in the respective participants’ publications.
表 2 关于主要组件使用的算法总结:2D或3D U-Net、重采样、预处理、训练或测试数据增强、用于优化的损失函数、测试预测的多模型集成以及结果的后处理。我们使用以下缩写表示预处理:剪切(C)、标准化(S),如果仅应用于一种模式,则在括号中指定。对于图像重采样,我们指定算法使用的是等距(I)还是非等距(A)重采样以及最近邻(NN)、线性(L)或立方(Cu)插值。我们使用以下缩写表示损失函数:交叉熵(CE)、Mumford-Shah(MS)和平均绝对误差(MAE)。更多详情可以在各参与者的出版物中找到。
Table 3Summary of the challenge results as of April 2021. The average DSC, precision, recall, SDSC and median HD95 are reported for the baseline algorithms and every team (thebest result of each team). The unit of the HD95 is [mm]. The participant names are reported when no team name was provided. The ranking is only provided for teamsthat presented their method in a paper submission. The post-challenge results are denoted by an asterisk ∗. Bold values represent the best scores for each metric, excludingpost-challenge results since we do not have any information about their method.
表 3截至2021年4月的挑战赛结果总结。报告了基线算法和每个团队(每个团队的最佳结果)的平均DSC、精度、召回率、SDSC和中位HD95。HD95的单位是[毫米]。如果没有提供团队名称,则报告参与者名称。只为提交了方法论文的团队提供排名。挑战赛后的结果由星号∗表示。粗体值代表除挑战赛后结果外每个指标的最佳分数,因为我们没有关于它们方法的任何信息。
相关文章:
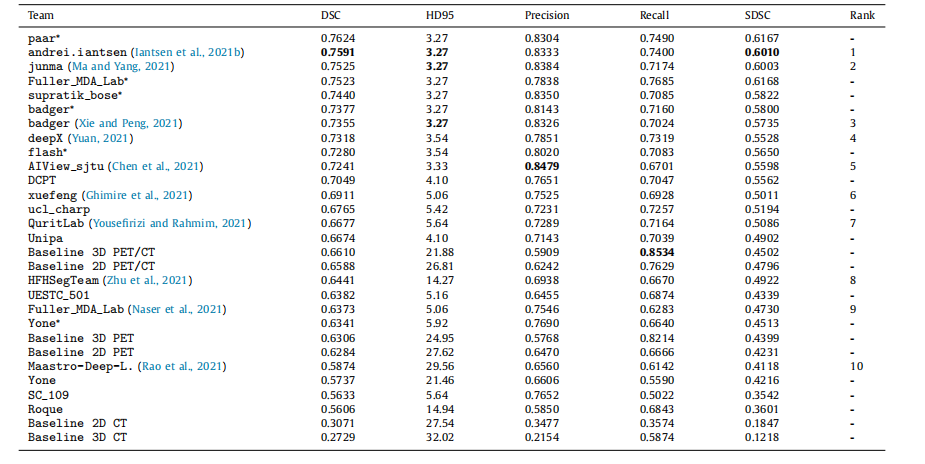
头颈肿瘤在PET/CT中的分割:HECKTOR挑战赛| 文献速递-深度学习肿瘤自动分割
Title 题目 Head and neck tumor segmentation in PET/CT: The HECKTOR challenge 头颈肿瘤在PET/CT中的分割:HECKTOR挑战赛 01 文献速递介绍 高通量医学影像分析,常被称为放射组学,已显示出其在揭示定量影像生物标志物与癌症预后之间关…...

Kafka重平衡导致无限循环消费问题
1. 问题描述 Kafka消费者消费消息超过了5分钟,不停的触发重平衡,消费者的offset因为重平衡提交失败,重复拉取消费,重复消费。 2. 问题原因 kafka默认的消息消费超时时间max.poll.interval.ms 300000, 也就是5分钟,…...

执行shell脚本时为什么要写成./test.sh,而不是test.sh?
一定要写成 ./test.sh,而不是 test.sh 运行其它二进制的程序也一样! 直接写 test.sh,linux 系统会去 PATH (系统环境)里寻找有没有叫 test.sh 的! 而只有 /bin, /sbin, /usr/bin,/usr/sbin 这…...

【人工智能】第一部分:ChatGPT的基本概念和技术背景
人不走空 🌈个人主页:人不走空 💖系列专栏:算法专题 ⏰诗词歌赋:斯是陋室,惟吾德馨 目录 🌈个人主页:人不走空 💖系列专栏:算法专题 ⏰诗词歌…...

雪花算法详解及源码分析
雪花算法的简介: 雪花算法用来实现全局唯一ID的业务主键,解决分库分表之后主键的唯一性问题,所以就单从全局唯一性来说,其实有很多的解决方法,比如说UUID、数据库的全局表的自增ID 但是在实际的开发过程中࿰…...
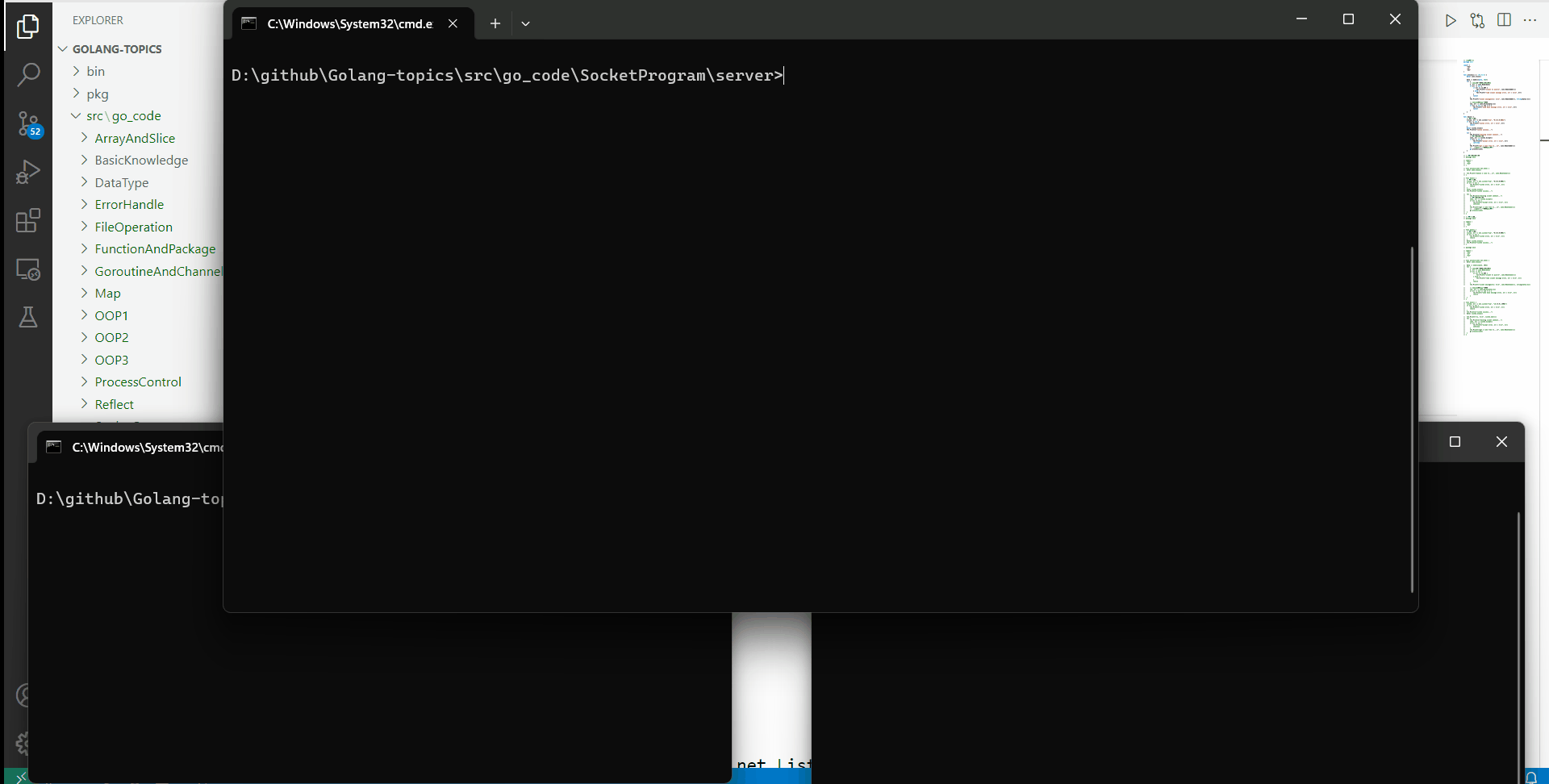
Golang TCP网络编程
文章目录 网络编程介绍TCP网络编程服务器监听客户端连接服务器服务端获取连接向连接中写入数据从连接中读取数据关闭连接/监听器 简易的TCP回声服务器效果展示服务端处理逻辑客户端处理逻辑 网络编程介绍 网络编程介绍 网络编程是指通过计算机网络实现程序间通信的一种编程技术…...

先进制造aps专题十 aps项目成功指南
aps项目成功指南 为了保证aps项目的成功 现在国内的aps项目 一是看aps软件本身是不是实现了复杂的排程算法和优化算法,算法引擎使用c高性能编译语言开发,支持工序的复杂关系,考虑副资源约束和特殊规格约束,提供了能考虑各种约束…...

实现Dropdown下拉菜单监听键盘上下键选中功能-React
用过ant design的小伙伴都知道,select组件是支持联想搜索跟上下键选中的效果的,但是在项目中我们可能会遇到用select组件无法实现我们的需求的情况,比如说一个div框,里面有input,又有tag标签,在input中输入…...

Ubuntu系统升级k8s节点的node节点遇到的问题
从1.23版本升级到1.28版本 node节点的是Ubuntu系统20.04的版本 Q1 node节点版本1.23升级1.28失败 解决办法: # 改为阿里云镜像 vim /etc/apt/sources.list.d/kubernetes.list# 新增 deb https://mirrors.aliyun.com/kubernetes/apt/ kubernetes-xenial main# 执…...

前端将DOM元素导出为图片
前端工作中经常会用到把一些元素导出,比如表格,正好项目有遇到导出为excel和导出为图片,就都封装实现了一下,以供其他需求的开发者使用: 1.导出为文档 这个说白了就是下载的功能,传过去检索参数ÿ…...
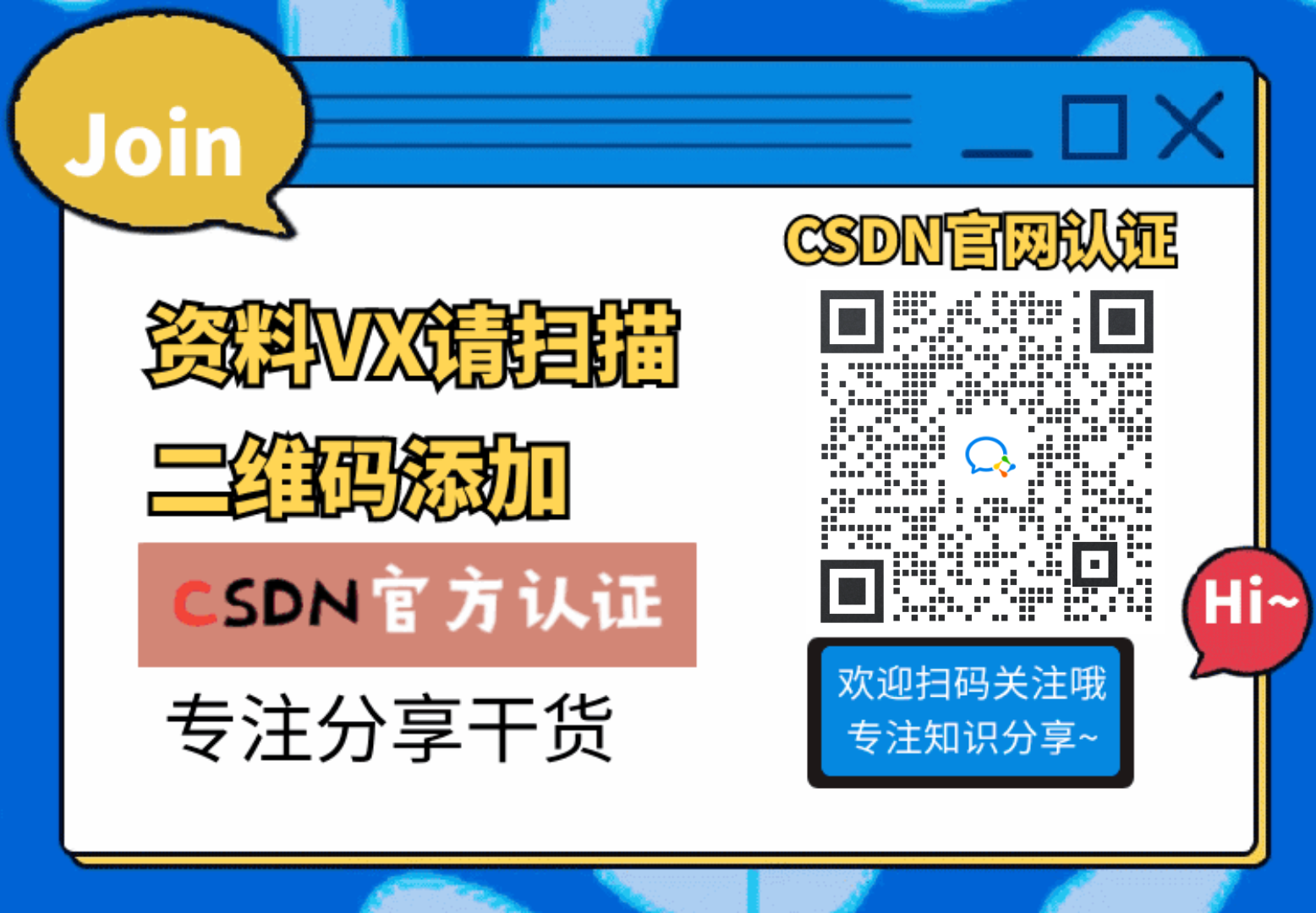
变现 5w+,一个被严重低估的 AI 蓝海赛道,居然用这个免费的AI绘画工具就能做!
大家好,我是画画的小强,致力于分享各类的 AI 工具,包括 AI 绘画工具、AI 视频工具、AI 写作工具等等。 但单纯地为了学而学,是没有任何意义的。 这些 AI 工具,学会了,用起来,才能发挥出他们的…...
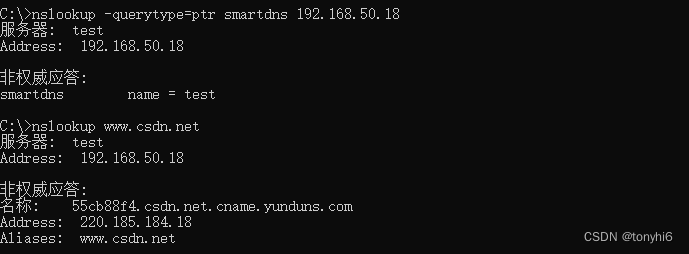
Ubuntu server 24 (Linux) 安装部署smartdns 搭建智能DNS服务器
SmartDNS是推荐本地运行的DNS服务器,SmartDNS接受本地客户端的DNS查询请求,从多个上游DNS服务器获取DNS查询结果,并将访问速度最快的结果返回给客户端,提高网络访问速度和准确性。 支持指定域名IP地址,达到禁止过滤的效…...
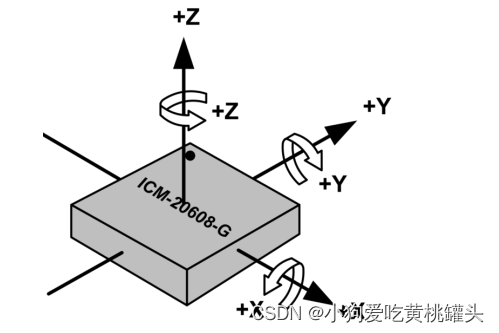
正点原子[第二期]Linux之ARM(MX6U)裸机篇学习笔记-24.5,6 SPI驱动实验-ICM20608 ADC采样值
前言: 本文是根据哔哩哔哩网站上“正点原子[第二期]Linux之ARM(MX6U)裸机篇”视频的学习笔记,在这里会记录下正点原子 I.MX6ULL 开发板的配套视频教程所作的实验和学习笔记内容。本文大量引用了正点原子教学视频和链接中的内容。…...
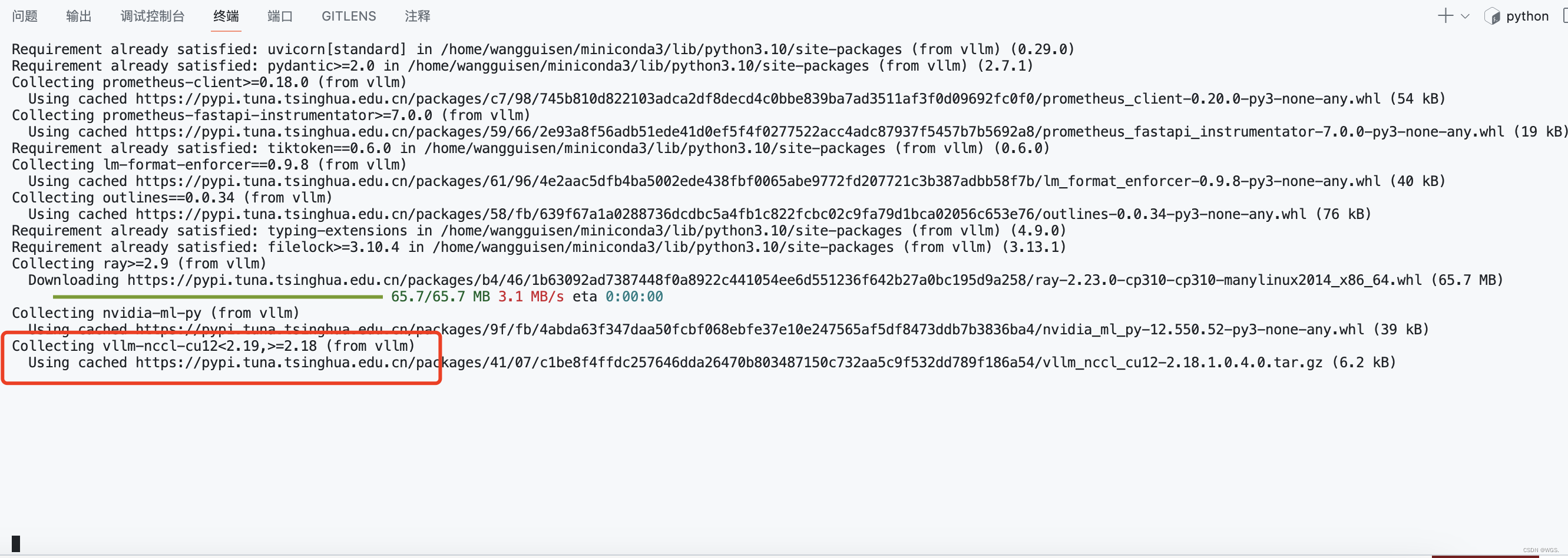
安装vllm的时候卡主:Collecting vllm-nccl-cu12<2.19,>=2.18 (from vllm)
按照vllm的时候卡主: ... Requirement already satisfied: typing-extensions in /home/wangguisen/miniconda3/lib/python3.10/site-packages (from vllm) (4.9.0) Requirement already satisfied: filelock>3.10.4 in /home/wangguisen/miniconda3/lib/python…...
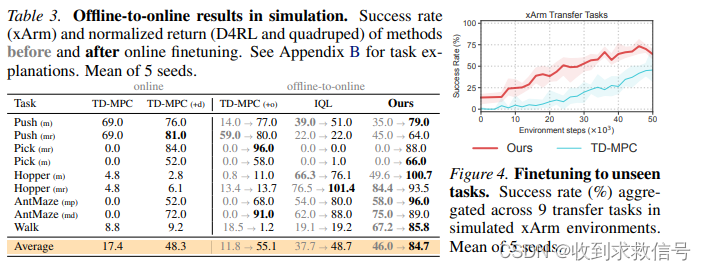
O2O : Finetuning Offline World Models in the Real World
CoRL 2023 Oral paper code Intro 算法基于TD-MPC,利用离线数据训练世界模型,然后在线融合基于集成Q的不确定性估计实现Planning。得到的在线数据将联合离线数据共同训练目标策略。 Method TD-MPC TD-MPC由五部分构成: 状态特征提取 z h θ ( s ) …...

嵌入式学习(Day:31 网络编程2:TCP)
client, server browser b/s http p2p peer TCP的特征:1.有链接;2.可靠传输;3.流式套接字 1、模式 C/S 模式 》服务器/客户端模型(服务端1个,客户端很多个) server:socket()-->bind()---…...

正则表达式 0.1v
正则表达式 扩展 --> :% s/\///g //文件里面所有的 / 去掉 * 通配符 \ //转义,让字符变成原本的意思 ^ //行首 $ //行尾 [0-9] //数字 [a-z] //小写字母 [A-Z] //大写字母 把文件的小写字母替换为大写字母? 固定写法 :% s/[a-…...
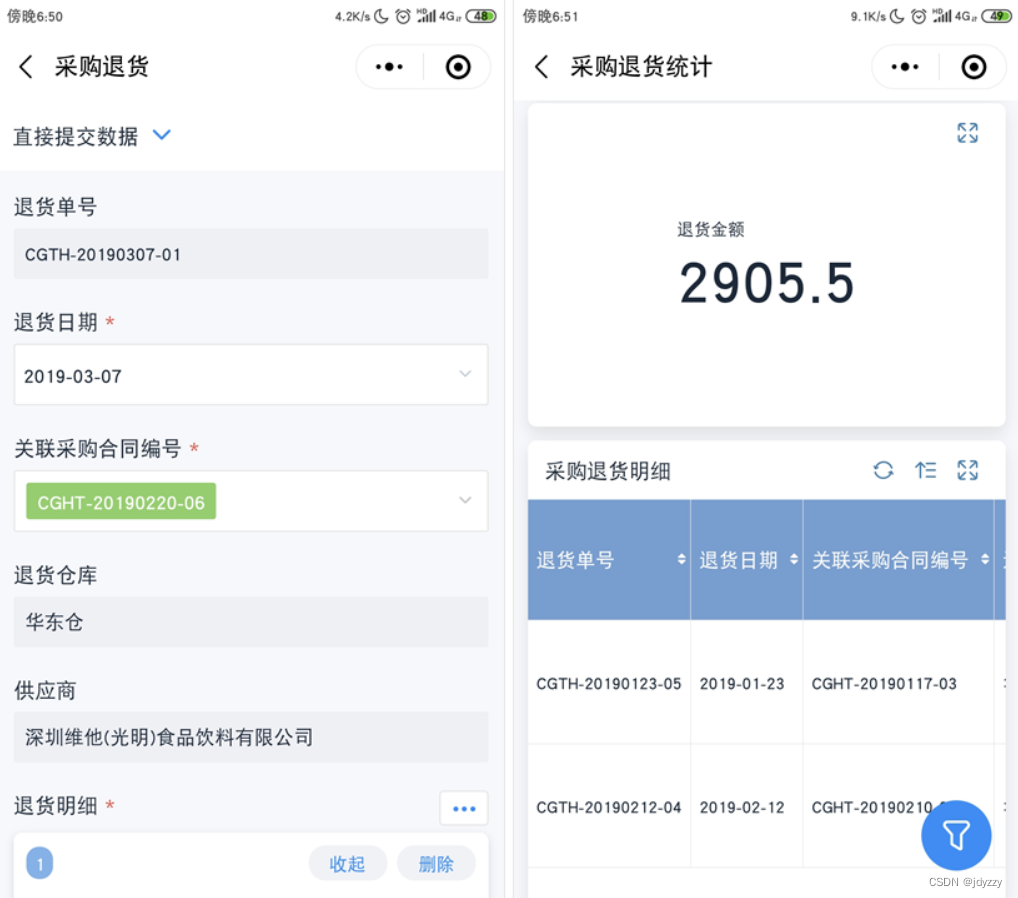
免费的仓库出入库管理软件有哪些?
中小企业因为预算有限,所以希望能在出入库管理软件方面能够减少成本。 但我们必须清醒地认识到,所谓的“永久免费”往往只是一个幌子。这些软件要么是新上市的、功能尚未完善的产品,试图通过免费吸引用户试用;要么在数据安全和客…...
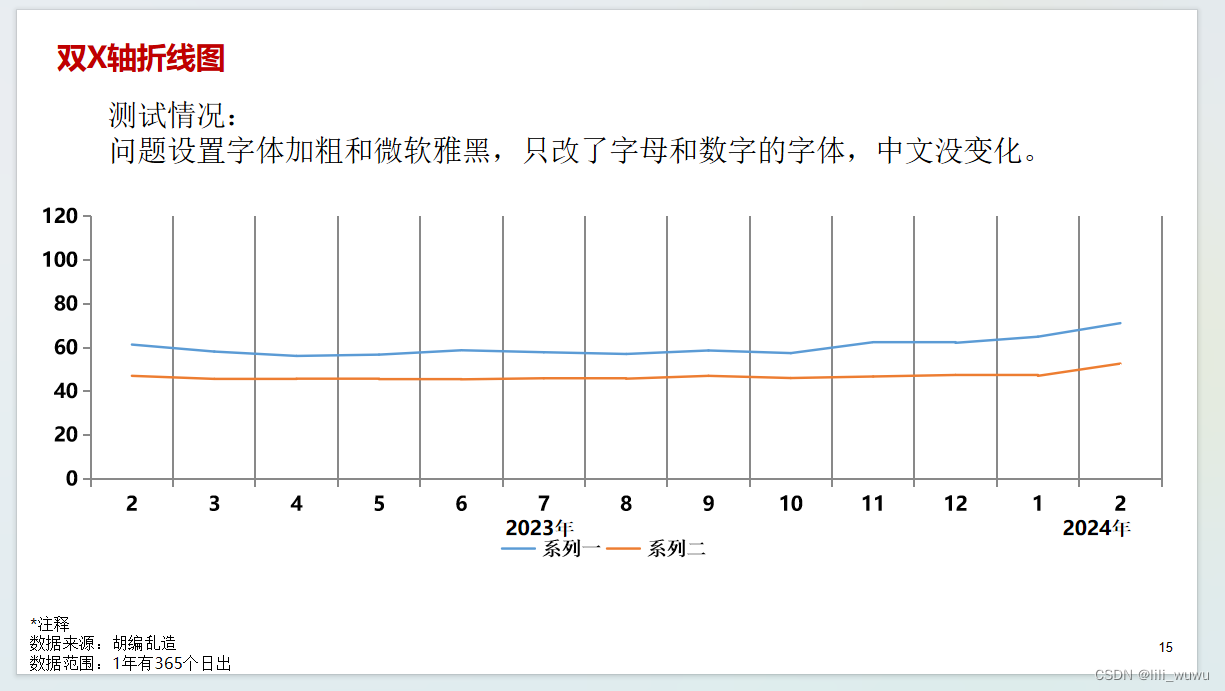
python 办公自动化-生成ppt文本和图
最终样式 代码实现 # 可编辑折线写入文字 成功 # 问题: 设置字体类型和加粗和字体为微软雅黑,是只改了字母和数字的字体,中文没变化 pip install pptx_ea_font 这个库可以解决这个问题 import pandas as pd import pptx_ea_font import mat…...

「动态规划」买卖股票的最佳时机
力扣原题链接,点击跳转。 给定一个整数数组prices,prices[i]表示股票在第i天的价格。你最多完成2笔交易。你不能同时参与多笔交易(你必须在再次购买前出售掉之前的股票)。设计一个算法计算最大利润。 我们用动态规划的思想来解决…...

Java 并发编程面试二
目录 一、并发编程三要素? 二、实现可见性的方法有哪些? 三、多线程的价值? 四、创建线程的有哪些方式? 五、创建线程的三种方式的对比? 六、Java 线程具有五中基本状态 七、什么是线程池?有哪几种创建方式 八、四种线程池的创建 九、线程池的优点? 十、常用的…...
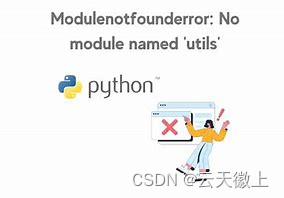
成功解决“ModuleNotFoundError: No Module Named ‘utils’”错误的全面指南
成功解决“ModuleNotFoundError: No Module Named ‘utils’”错误的全面指南 在Python编程中,遇到ModuleNotFoundError: No Module Named utils这样的错误通常意味着Python解释器无法找到名为utils的模块。这可能是由于多种原因造成的,比如模块确实不存…...
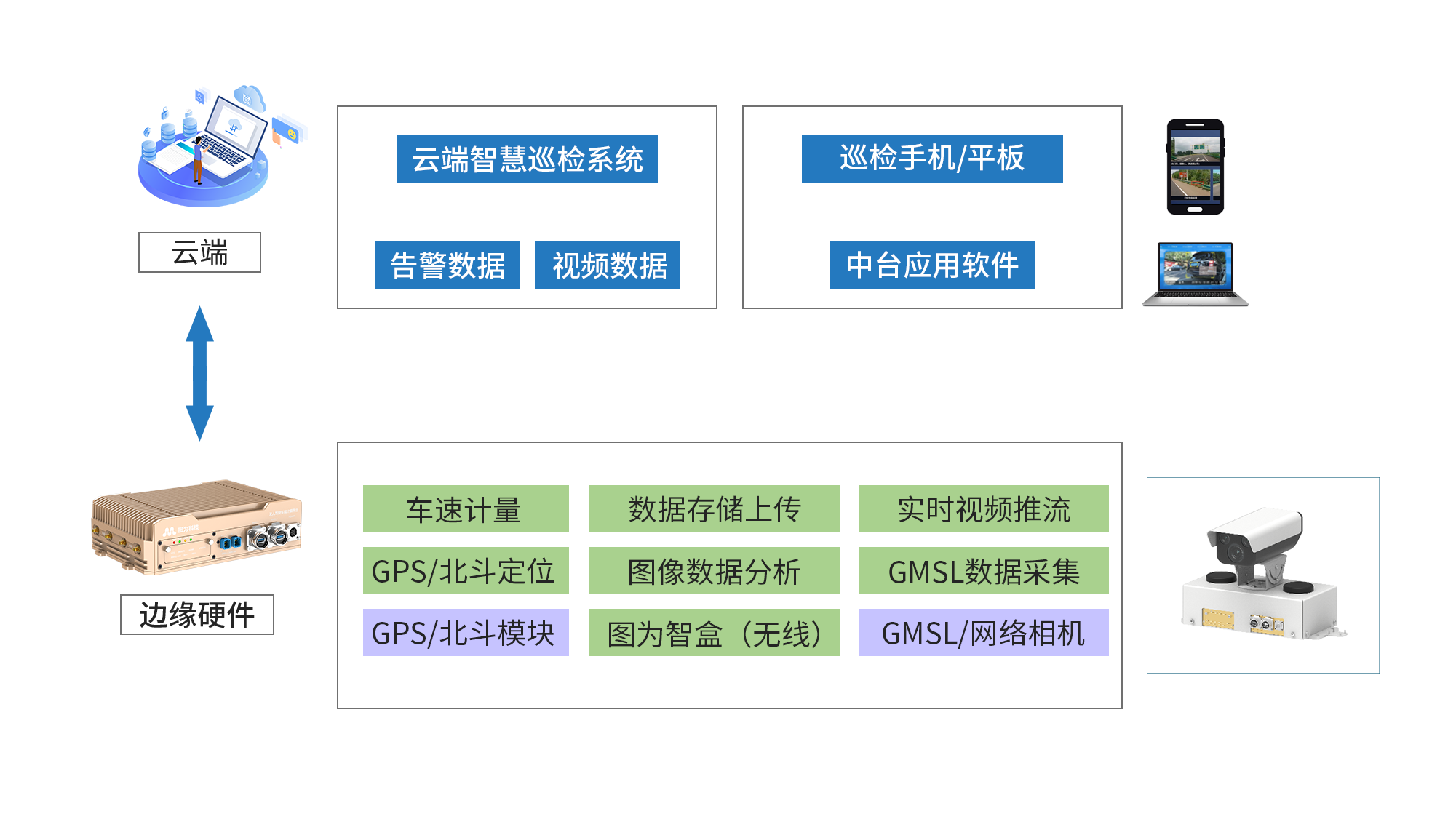
Nvidia Jetson/Orin +FPGA+AI大算力边缘计算盒子:公路智能巡检解决方案
项目背景 中国公路网络庞大,总里程超过535万公里,高速公路里程位居世界前列。面对基础设施存量的不断增长,公路养护管理已迈入“建管养并重”的新时代。随着养护支出的逐年攀升,如何提升养护效率、降低管理成本,成为亟…...

【Maxcompute】geohash转经纬度,经纬度转geohash,计算geohash九宫格
1.梳理、总结经纬度处理在Maxcompute平台上的实战应用,如geohash转经纬度,经纬度转geohash,计算geohash九宫格等。 2.欢迎批评指正,跪谢一键三连! 文章目录 1.部署代码1.部署代码 部署至Maxcompute(ODPS)-DataWorks平台,去掉代码注释即可#coding:utf-8 # from odps.udf…...
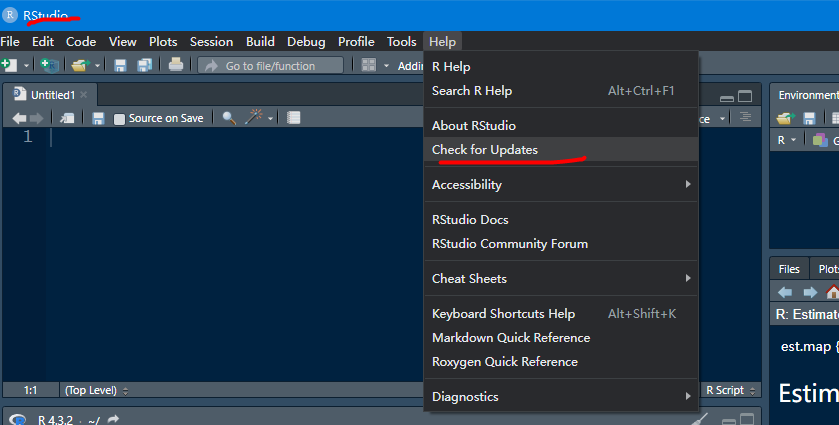
【R语言基础】如何更新R版本
文章目录 概要流程细节具体步骤 概要 提示:由于软件包的更新,所以需要更新R至新版本 流程细节 查看当前R版本 R.version下载更新包:installr install.packages("installr")library(installr)跟着向导一步步执行安装 具体步骤 …...

Python知识点10---函数
提前说一点:如果你是专注于Python开发,那么本系列知识点只是带你入个门再详细的开发点就要去看其他资料了,而如果你和作者一样只是操作其他技术的Python API那就足够了。 Python的函数和Scala的函数很像,语法很简单,注…...
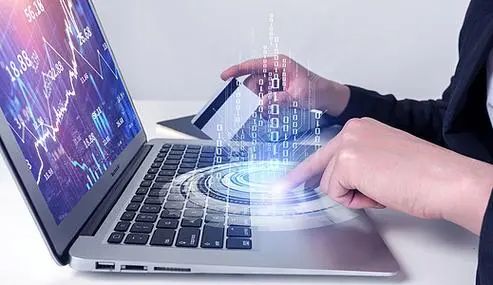
有哪些挣钱软件一天能赚几十元?盘点十个能长期做下去的挣钱软件
在这个信息爆炸的时代,每个人都在寻找快速赚钱的秘诀。很多人做兼职副业的目标并不是获得很大的成功,大部分人一天能赚几十就心满意足了。 今天,我要带你一探究竟,揭秘那些能让你日赚几十元的挣钱软件。准备好了吗?让我…...

CentOS7安装MySQL教程
第一章 检查是否安装了Mysql 1.1 yum检查 yum list installed | grep mysql 1.2 安装则直接删除 yum remove xxx 1.3 rpm检查 rpm -qa | grep -i mysql # 有则直接删除 rpm -e --nodeps xxx 第二章 正式安装MySQL 2.1 yum安装,下载mysql wget --no-check-ce…...
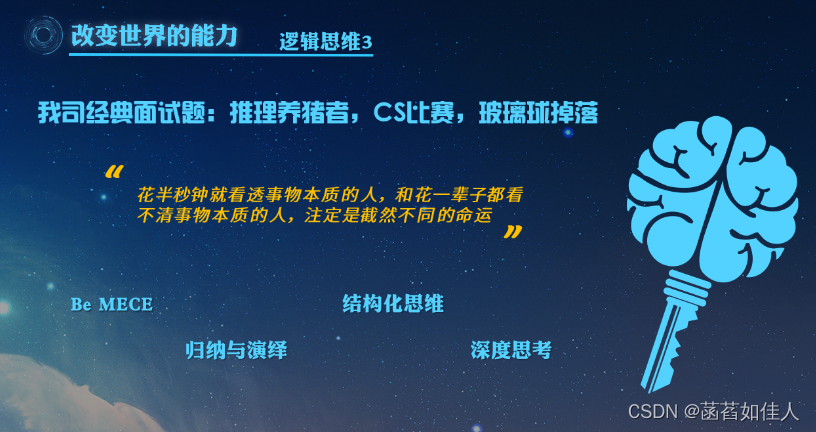
师彼长技以助己(3)逻辑思维
师彼长技以助己(3)逻辑思维 前言 上一篇文章进行了工程思维和产品思维的测试,并介绍了几个比较重要的产品思维模型。接下来本篇介绍工程思维。(注意产品思维并不代表产品经理思维,工程思维也并不代表工程师思维&…...

LeetCode:反转链表I
文章收录于LeetCode专栏 LeetCode地址 反转链表I 题目 给你单链表的头节点head,请你反转链表,并返回反转后的链表。 示例 1: #mermaid-svg-IYmD16EKuu3CZWwV {font-family:"trebuchet ms",verdana,arial,sans-serif;font-size…...