Pytorch 实现图片分类
CNN 网络适用于图片识别,卷积神经网络主要用于图片的处理识别。卷积神经网络,包括一下几部分,输入层、卷积层、池化层、全链接层和输出层。
使用 CIFAR-10 进行训练, CIFAR-10 中图片尺寸为 32 * 32。卷积层通过卷积核移动进行计算最终生成特征图。
通过池化层进行降维度
卷积网络结构从输入到输出, 3* 32*32 --> 10:
类型 | Weight | BIAS |
---|---|---|
卷积(3, 12, 5) | (12, 3, 5, 5) | 12 |
卷积(12, 12, 5) | (12, 12, 5, 5) | 12 |
Norm | 12 | 12 |
卷积(12, 24, 5) | (24, 12, 5, 5) | 24 |
卷积(24 24, 5) | (24, 24, 5, 5) | 24 |
Norm | 24 | 24 |
Linear | (10, 2400) | 10 |
训练分类模型
准备数据
from torchvision.datasets import CIFAR10
from torchvision.transforms import transforms
from torch.utils.data import DataLoader# Loading and normalizing the data.
# Define transformations for the training and test sets
transformations = transforms.Compose([transforms.ToTensor(),transforms.Normalize((0.5, 0.5, 0.5), (0.5, 0.5, 0.5))
])# CIFAR10 dataset consists of 50K training images. We define the batch size of 10 to load 5,000 batches of images.
batch_size = 10
number_of_labels = 10 # Create an instance for training.
# When we run this code for the first time, the CIFAR10 train dataset will be downloaded locally.
train_set =CIFAR10(root="./data",train=True,transform=transformations,download=True)# Create a loader for the training set which will read the data within batch size and put into memory.
train_loader = DataLoader(train_set, batch_size=batch_size, shuffle=True, num_workers=0)
print("The number of images in a training set is: ", len(train_loader)*batch_size)# Create an instance for testing, note that train is set to False.
# When we run this code for the first time, the CIFAR10 test dataset will be downloaded locally.
test_set = CIFAR10(root="./data", train=False, transform=transformations, download=True)# Create a loader for the test set which will read the data within batch size and put into memory.
# Note that each shuffle is set to false for the test loader.
test_loader = DataLoader(test_set, batch_size=batch_size, shuffle=False, num_workers=0)
print("The number of images in a test set is: ", len(test_loader)*batch_size)print("The number of batches per epoch is: ", len(train_loader))
classes = ('plane', 'car', 'bird', 'cat', 'deer', 'dog', 'frog', 'horse', 'ship', 'truck')
创建网络
import torch
import torch.nn as nn
import torchvision
import torch.nn.functional as F# Define a convolution neural network
class Network(nn.Module):def __init__(self):super(Network, self).__init__()self.conv1 = nn.Conv2d(in_channels=3, out_channels=12, kernel_size=5, stride=1, padding=1)self.bn1 = nn.BatchNorm2d(12)self.conv2 = nn.Conv2d(in_channels=12, out_channels=12, kernel_size=5, stride=1, padding=1)self.bn2 = nn.BatchNorm2d(12)self.pool = nn.MaxPool2d(2,2)self.conv4 = nn.Conv2d(in_channels=12, out_channels=24, kernel_size=5, stride=1, padding=1)self.bn4 = nn.BatchNorm2d(24)self.conv5 = nn.Conv2d(in_channels=24, out_channels=24, kernel_size=5, stride=1, padding=1)self.bn5 = nn.BatchNorm2d(24)self.fc1 = nn.Linear(24*10*10, 10)def forward(self, input):output = F.relu(self.bn1(self.conv1(input))) output = F.relu(self.bn2(self.conv2(output))) output = self.pool(output) output = F.relu(self.bn4(self.conv4(output))) output = F.relu(self.bn5(self.conv5(output))) output = output.view(-1, 24*10*10)output = self.fc1(output)return output# Instantiate a neural network model
model = Network()
定义损失函数
使用交叉熵函数作为损失函数,交叉熵分为两种
- 二分类交叉熵函数
- 多分类交叉熵函数
loss_fn = nn.CrossEntropyLoss()
optimizer = Adam(model.parameters(), lr=0.001, weight_decay=0.0001)
模型训练
from torch.autograd import Variable# Function to save the model
def saveModel():path = "./myFirstModel.pth"torch.save(model.state_dict(), path)# Function to test the model with the test dataset and print the accuracy for the test images
def testAccuracy():model.eval()accuracy = 0.0total = 0.0device = torch.device("cuda:0" if torch.cuda.is_available() else "cpu")with torch.no_grad():for data in test_loader:images, labels = data# run the model on the test set to predict labelsoutputs = model(images.to(device))# the label with the highest energy will be our prediction_, predicted = torch.max(outputs.data, 1)total += labels.size(0)accuracy += (predicted == labels.to(device)).sum().item()# compute the accuracy over all test imagesaccuracy = (100 * accuracy / total)return(accuracy)# Training function. We simply have to loop over our data iterator and feed the inputs to the network and optimize.
def train(num_epochs):best_accuracy = 0.0# Define your execution devicedevice = torch.device("cuda:0" if torch.cuda.is_available() else "cpu")print("The model will be running on", device, "device")# Convert model parameters and buffers to CPU or Cudamodel.to(device)for epoch in range(num_epochs): # loop over the dataset multiple timesrunning_loss = 0.0running_acc = 0.0for i, (images, labels) in enumerate(train_loader, 0):# get the inputsimages = Variable(images.to(device))labels = Variable(labels.to(device))# zero the parameter gradientsoptimizer.zero_grad()# predict classes using images from the training setoutputs = model(images)# compute the loss based on model output and real labelsloss = loss_fn(outputs, labels)# backpropagate the lossloss.backward()# adjust parameters based on the calculated gradientsoptimizer.step()# Let's print statistics for every 1,000 imagesrunning_loss += loss.item() # extract the loss valueif i % 1000 == 999: # print every 1000 (twice per epoch) print('[%d, %5d] loss: %.3f' %(epoch + 1, i + 1, running_loss / 1000))# zero the lossrunning_loss = 0.0# Compute and print the average accuracy fo this epoch when tested over all 10000 test imagesaccuracy = testAccuracy()print('For epoch', epoch+1,'the test accuracy over the whole test set is %d %%' % (accuracy))# we want to save the model if the accuracy is the bestif accuracy > best_accuracy:saveModel()best_accuracy = accuracy
测试模型
import matplotlib.pyplot as plt
import numpy as np# Function to show the images
def imageshow(img):img = img / 2 + 0.5 # unnormalizenpimg = img.numpy()plt.imshow(np.transpose(npimg, (1, 2, 0)))plt.show()# Function to test the model with a batch of images and show the labels predictions
def testBatch():# get batch of images from the test DataLoader images, labels = next(iter(test_loader))# show all images as one image gridimageshow(torchvision.utils.make_grid(images))# Show the real labels on the screen print('Real labels: ', ' '.join('%5s' % classes[labels[j]] for j in range(batch_size)))# Let's see what if the model identifiers the labels of those exampleoutputs = model(images)# We got the probability for every 10 labels. The highest (max) probability should be correct label_, predicted = torch.max(outputs, 1)# Let's show the predicted labels on the screen to compare with the real onesprint('Predicted: ', ' '.join('%5s' % classes[predicted[j]] for j in range(batch_size)))
执行模型
if __name__ == "__main__":# Let's build our modeltrain(5)print('Finished Training')# Test which classes performed welltestAccuracy()# Let's load the model we just created and test the accuracy per labelmodel = Network()path = "myFirstModel.pth"model.load_state_dict(torch.load(path))# Test with batch of imagestestBatch()
总结
pytorch 搭建一个 CNN 模型比较简单,5 轮训练之后,效果就可以达到 60%,10 张图片中预测对了 6 张。
相关文章:
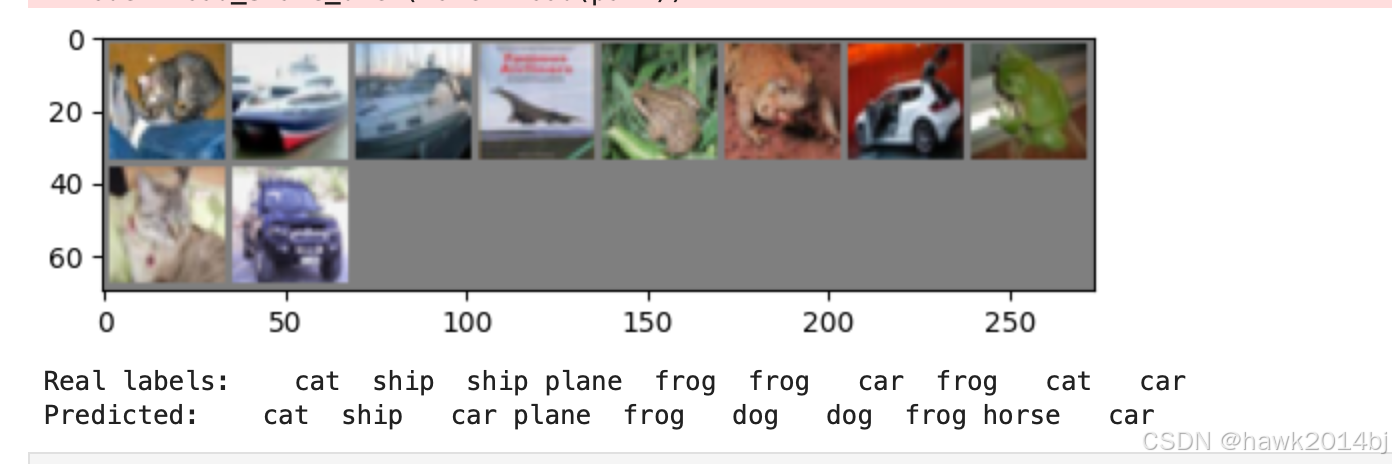
Pytorch 实现图片分类
CNN 网络适用于图片识别,卷积神经网络主要用于图片的处理识别。卷积神经网络,包括一下几部分,输入层、卷积层、池化层、全链接层和输出层。 使用 CIFAR-10 进行训练, CIFAR-10 中图片尺寸为 32 * 32。卷积层通过卷积核移动进行计…...
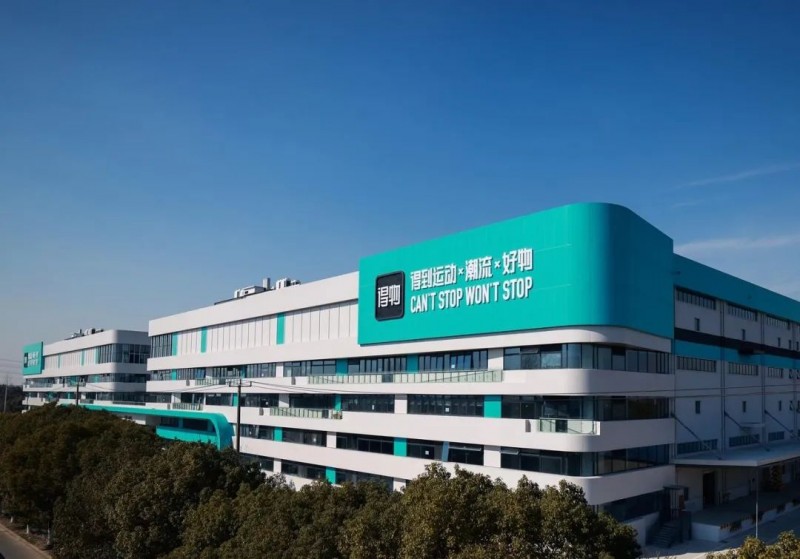
得物App获评新奖项,正品保障夯实供应链创新水平
近日,得物App再度获评新奖项——“2024上海市供应链创新与应用优秀案例”。 本次奖项为上海市供应链领域最高奖项,旨在评选出在供应链创新成效上处于领先地位、拥有成功模式和经验的企业。今年以来,得物App已接连获得“上海市质量金奖”、“科…...
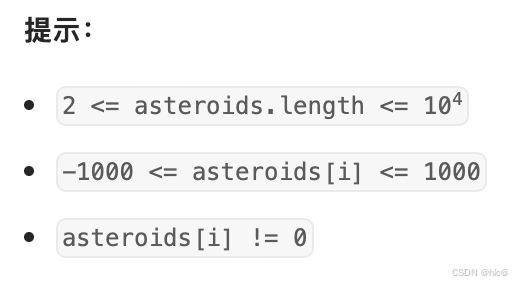
【数据结构-邻项消除】力扣735. 小行星碰撞
给定一个整数数组 asteroids,表示在同一行的小行星。 对于数组中的每一个元素,其绝对值表示小行星的大小,正负表示小行星的移动方向(正表示向右移动,负表示向左移动)。每一颗小行星以相同的速度移动。 找…...
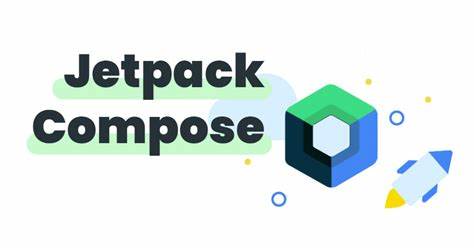
002-Kotlin界面开发之Kotlin旋风之旅
Kotlin旋风之旅 Compose Desktop中哪些Kotlin知识是必须的? 在学习Compose Desktop中,以下Kotlin知识是必须的: 基础语法:包括变量声明、数据类型、条件语句、循环等。面向对象编程:类与对象、继承、接口、抽象类等。…...
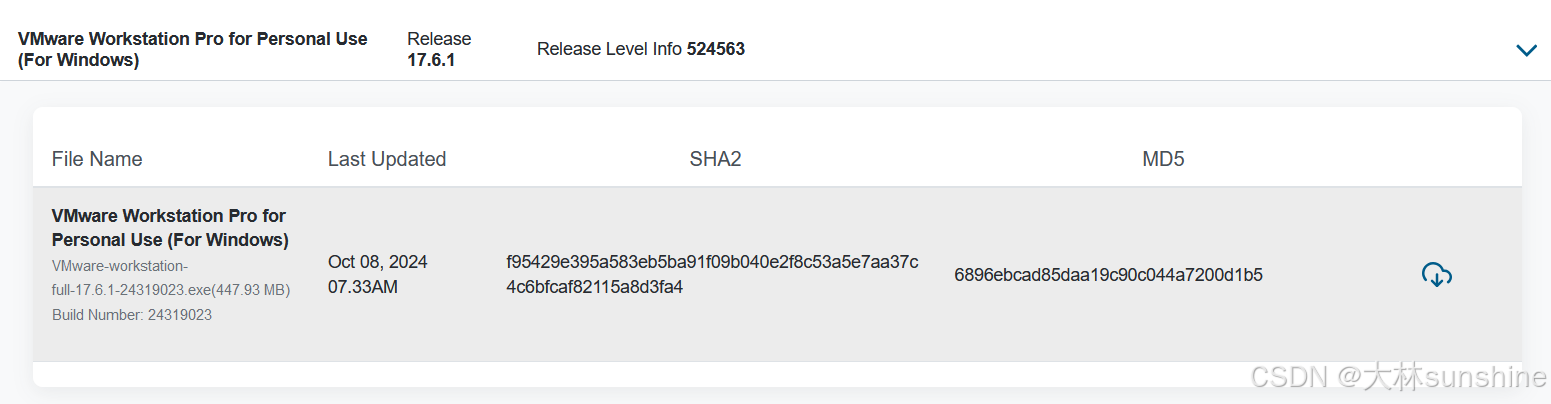
VMware Workstation Pro for Personal Use (For Windows)
这是从broadcom.com网下载的个人版本的Vmware 17.6.1,存分享不要分。 VMware-workstation-full-17.6.1-24319023.exe(447.93 MB) Build Number: 24319023 Oct 08, 2024 07.33AM SHA2: f95429e395a583eb5ba91f09b040e2f8c53a5e7aa37c4c6bfcaf82115a8…...
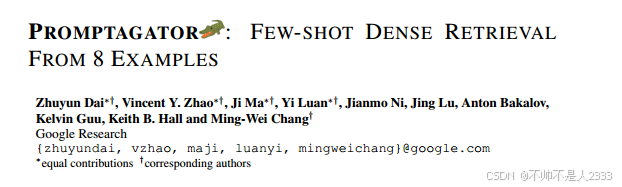
论文 | PROMPTAGATOR : FEW-SHOT DENSE RETRIEVAL FROM 8 EXAMPLES
1. 背景信息 在信息检索领域,传统的方法往往依赖于大量的标注数据来训练模型,以便在各种任务中表现良好。然而,许多实际应用中的监督数据是有限的,尤其是在不同的检索任务中。最近的研究开始关注如何从一个拥有丰富监督数据的任务…...
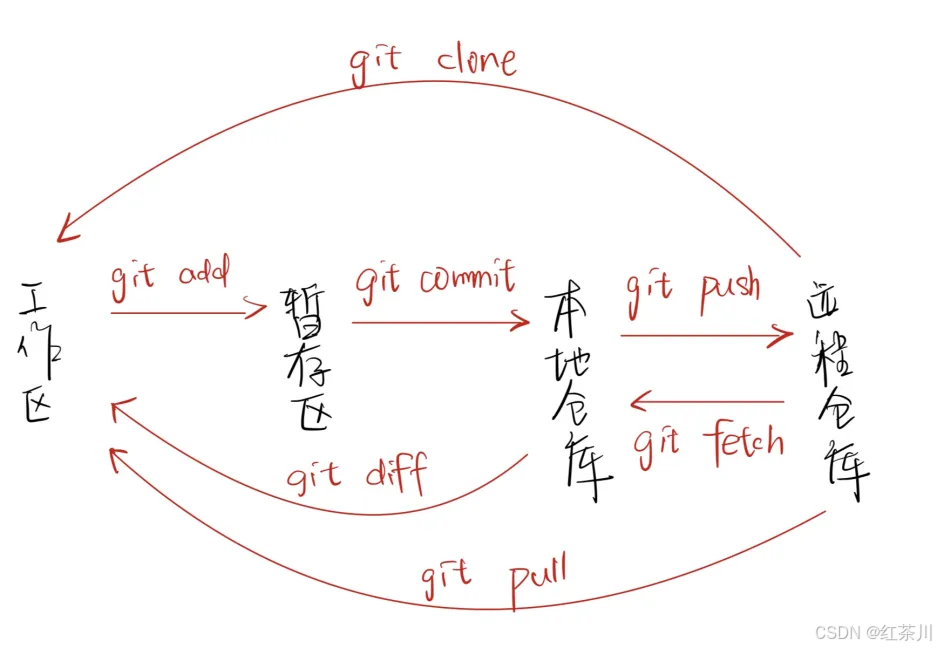
使用 Github 进行项目管理
GitHub 是一个广泛使用的代码托管和协作平台,它提供了强大的工具来支持项目管理和团队协作。在项目开发和工作中,避免不了 Github 的使用,然鹅我一直没有稍微系统地学习过 github 的整个工作流程,对这些操作都是一知半解的&#x…...
企业SRC挖掘选择与信息收集指南
内容预览 ≧∀≦ゞ 企业SRC挖掘选择与信息收集指南导语1. 企业SRC的选择2. 信息收集2.1 集团与子公司2.2 小程序与APP2.3 Web端信息收集 3. 信息收集常用模板总结 企业SRC挖掘选择与信息收集指南 导语 近年来,企业的安全响应中心(SRC)已逐渐…...
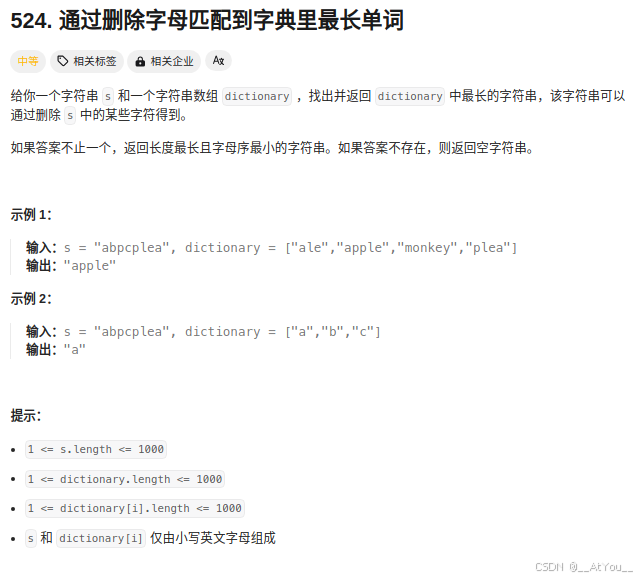
Golang | Leetcode Golang题解之第524题通过删除字母匹配到字典里最长单词
题目: 题解: func findLongestWord(s string, dictionary []string) (ans string) {m : len(s)f : make([][26]int, m1)for i : range f[m] {f[m][i] m}for i : m - 1; i > 0; i-- {f[i] f[i1]f[i][s[i]-a] i}outer:for _, t : range dictionary …...
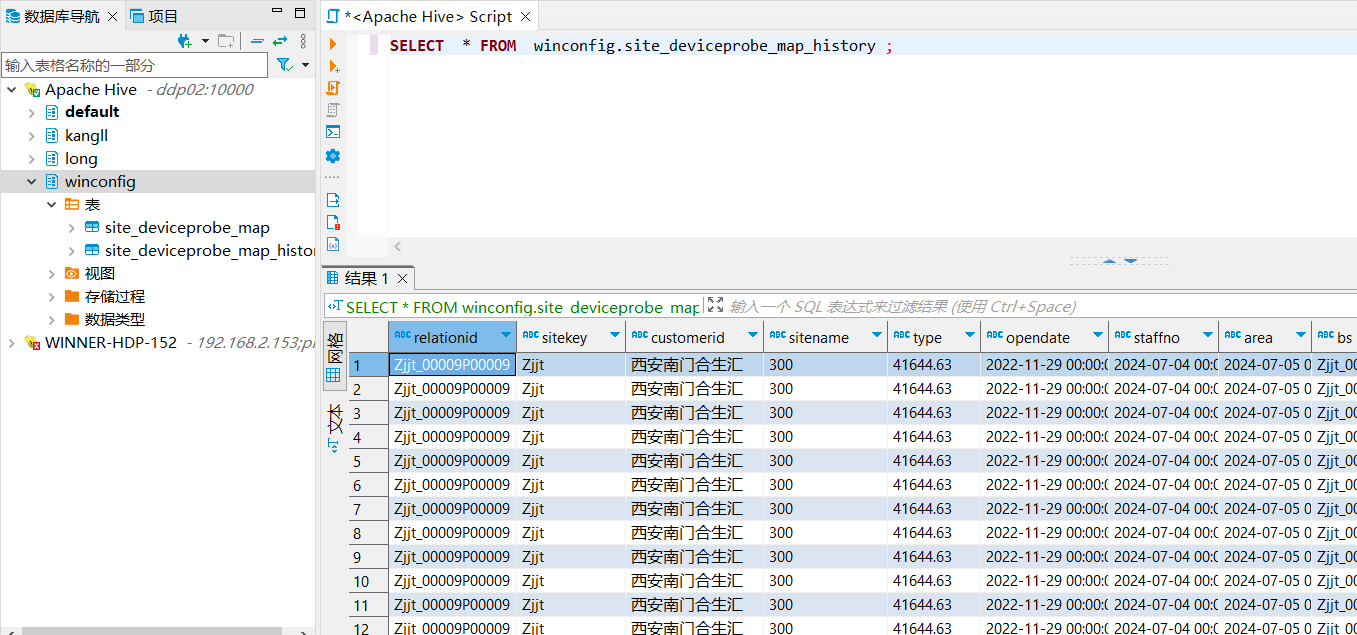
【DBeaver】连接带kerberos的hive[Apache|HDP]
目录 一、安装配置Kerberos客户端环境 1.1 安装Kerberos客户端 1.2 环境配置 二、基于Cloudera驱动创建连接 三、基于Hive原生驱动创建连接 一、安装配置Kerberos客户端环境 1.1 安装Kerberos客户端 在Kerberos官网下载,地址如下:https://web.mit.edu/kerberos…...
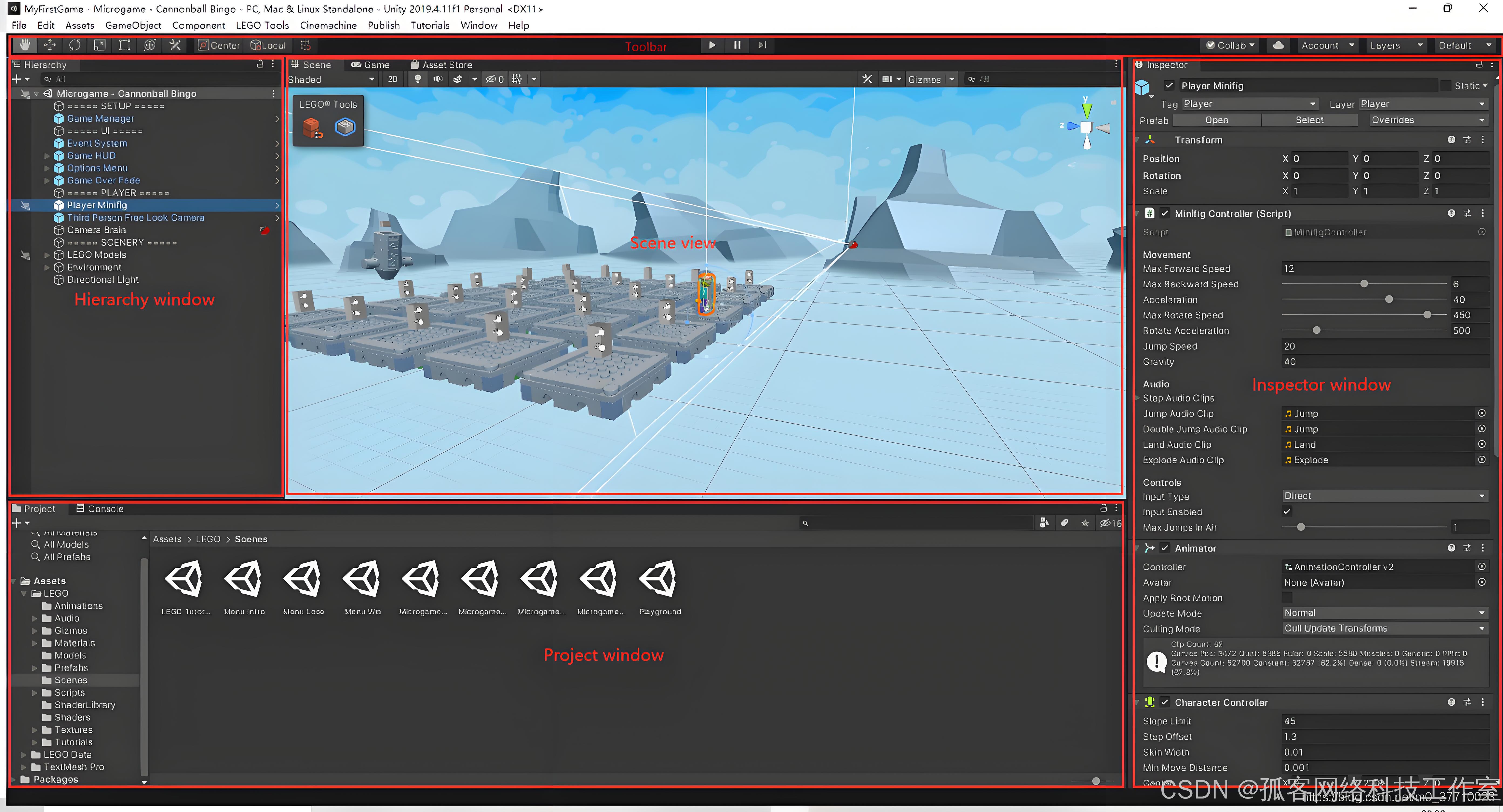
Unity3D 开发教程:从入门到精通
Unity3D 开发教程:从入门到精通 Unity3D 是一款强大的跨平台游戏引擎,广泛应用于游戏开发、虚拟现实、增强现实等领域。本文将详细介绍 Unity3D 的基本概念、开发流程以及一些高级技巧,帮助你从零基础到掌握 Unity3D 开发。 目录 Unity3D…...
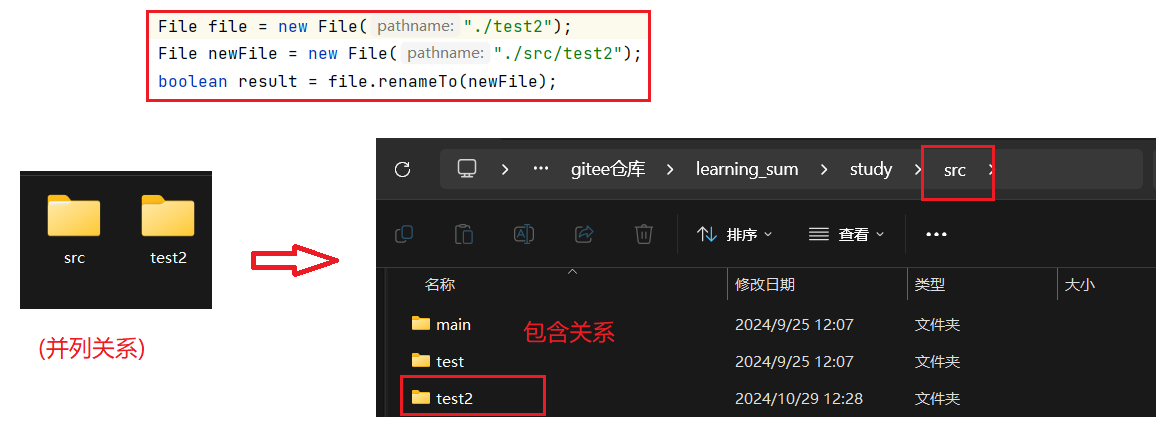
文件操作和 IO(一):文件基础知识 文件系统操作 => File类
目录 1. 什么是文件 1.1 概念 1.2 硬盘, 内存, 寄存器之间的区别 1.3 机械硬盘和固态硬盘 2. 文件路径 2.1 绝对路径 2.2 相对路径 3. 文件分类 4. File 类 4.1 属性 4.2 构造方法 4.3 方法 1. 什么是文件 1.1 概念 狭义上的文件: 保存在硬盘上的文件广义的上的文…...
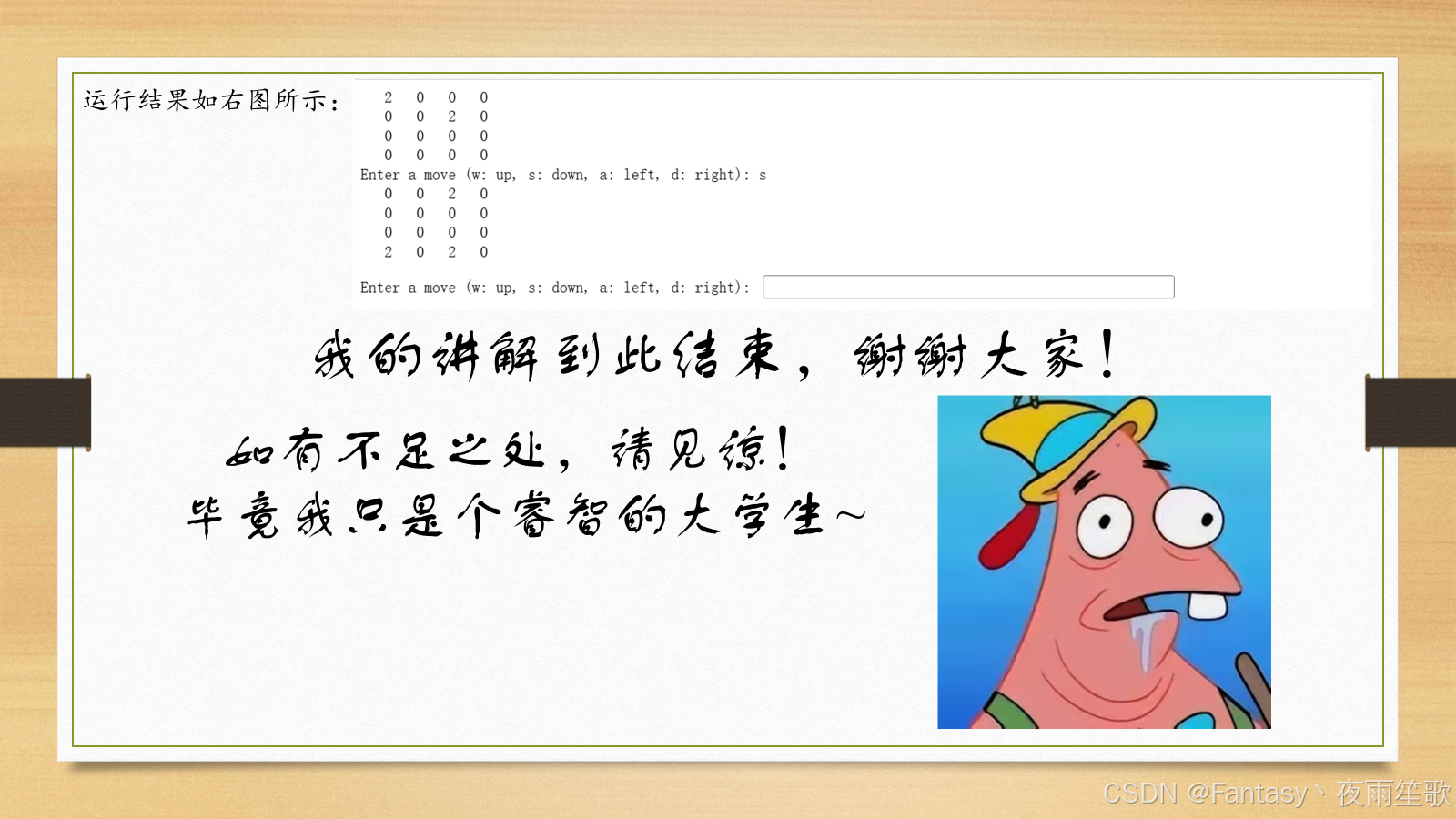
用Pyhon写一款简单的益智类小游戏——2048
文字版——代码及讲解 代码—— import random# 初始化游戏棋盘 def init_board():return [[0] * 4 for _ in range(4)]# 在棋盘上随机生成一个2或4 def add_new_tile(board):empty_cells [(i, j) for i in range(4) for j in range(4) if board[i][j] 0]if empty_cells:i,…...
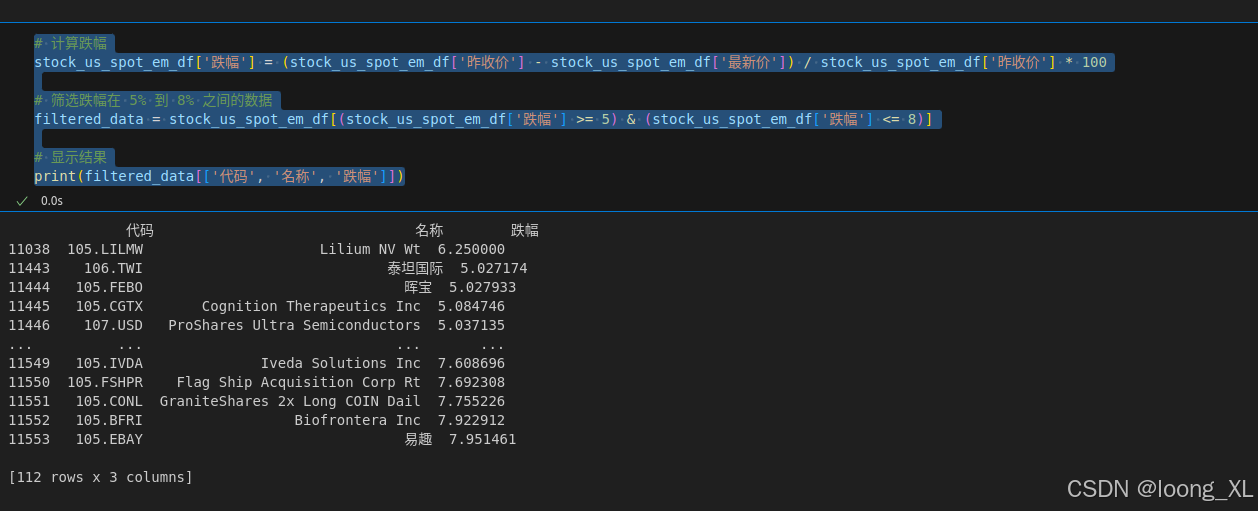
akshare股票涨跌幅自定义范围查询:A股、港股、美股
参看:https://stock.hexun.com/2024-10-31/215251914.html 涨幅计算公式:(当前价格 - 上一个交易日收盘价) 上一个交易日收盘价 100% 。 跌幅计算公式:(上一个交易日收盘价 - 当前价格) 上一个…...
通过js控制修改css变量
在JavaScript中,你可以通过操作CSS变量(也称为自定义属性)来动态改变样式。CSS变量在CSS中使用 – 前缀定义,例如 --main-color: red;。在JavaScript中,你可以使用 document.documentElement.style.setProperty 方法来…...
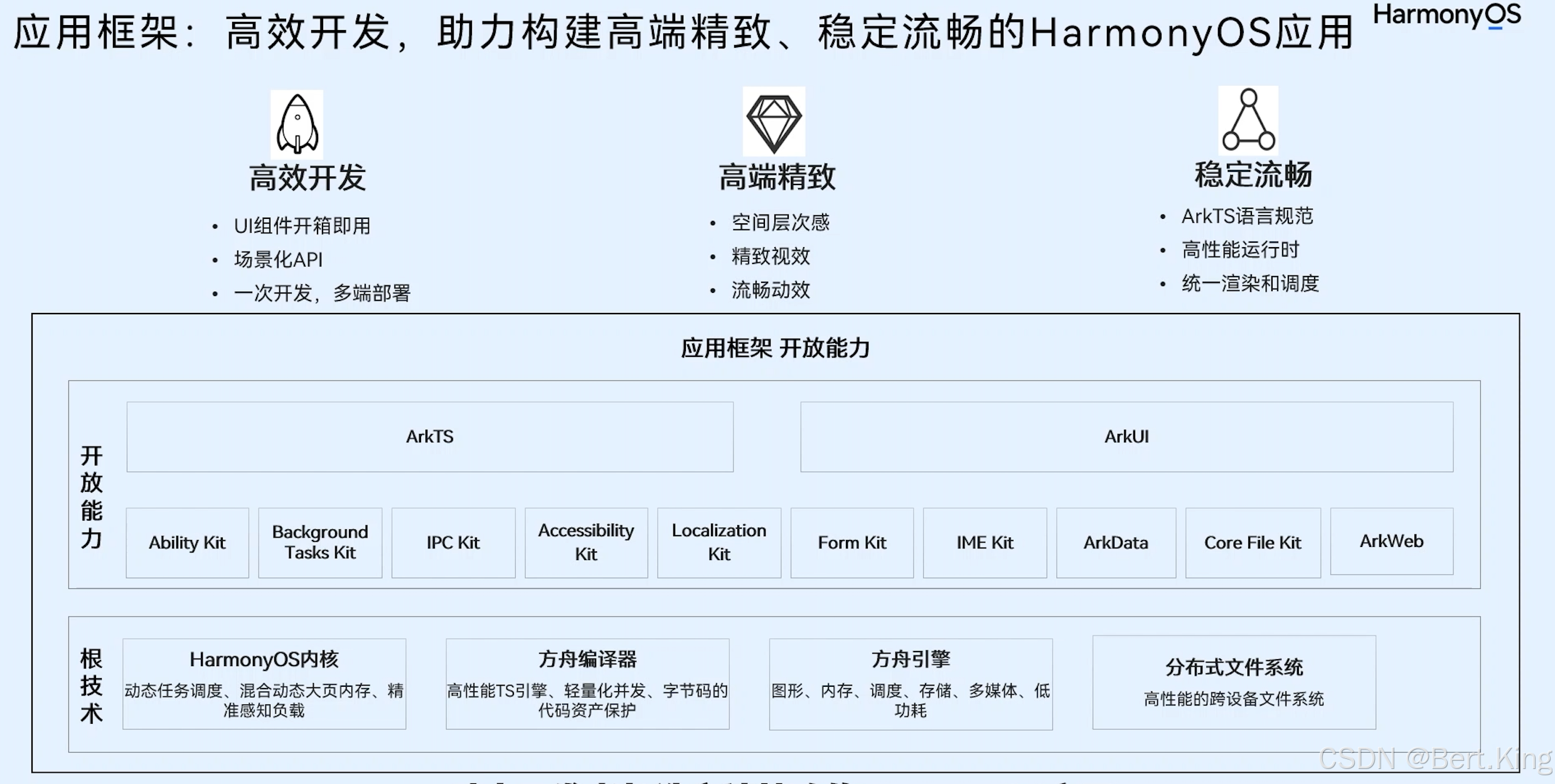
<HarmonyOS第一课>HarmonyOS SDK开放能力简介的课后习题
不出户,知天下; 不窥牖,见天道。 其出弥远,其知弥少。 是以圣人不行而知,不见而明,不为而成。 本篇<HarmonyOS第一课>HarmonyOS SDK开放能力简介是简单介绍了HarmonyOS SDK,不需要大家过多…...
深度学习:yolo的使用--图像处理
定义了一个名为 ListDataset 的类,它继承自 PyTorch 的 Dataset 类,这个数据集从一个包含图像文件路径的列表中读取图像和对应的标签文件 class ListDataset(Dataset):def __init__(self, list_path, img_size416, augmentTrue, multiscaleTrue, normalized_labelsT…...
TypeScript实用笔记(一):初始化、类型定义与函数使用
文章目录 一、ts初始化1. 初始化.json文件一2. 启动方式2.1 直接运行.ts文件2.2 转换运行 二、类型1. 参数类型1.1 常规参数1.2 symbol1.3 数组\[]1.4 元组\[]1.5 用字面量定义数据类型 2. Object3. 枚举类型\[Enum]3.1 数字枚举3.2 字符串枚举 三、 类型别名1. 数组别名使用2.…...
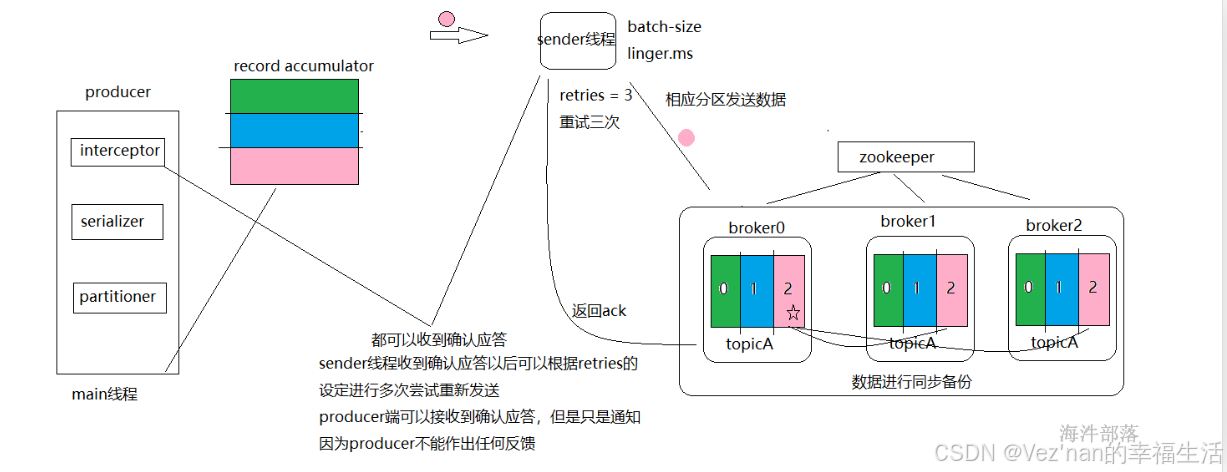
【大数据学习 | kafka】producer之拦截器,序列化器与分区器
1. 自定义拦截器 interceptor是拦截器,可以拦截到发送到kafka中的数据进行二次处理,它是producer组成部分的第一个组件。 public static class MyInterceptor implements ProducerInterceptor<String,String>{Overridepublic ProducerRecord<…...
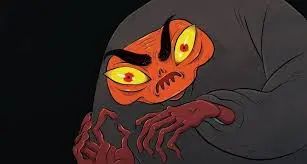
零基础学西班牙语,柯桥专业小语种培训泓畅学校
No te comas el coco, seguro que te ha salido bien la entrevista. Ya te llamarn. 别瞎想了!我保证你的面试很顺利。他们会给你打电话的。 这里的椰子是"头"的比喻。在西班牙的口语中,我们也可以听到其他同义表达,比如&#x…...
解锁数据库简洁之道:FastAPI与SQLModel实战指南
在构建现代Web应用程序时,与数据库的交互无疑是核心环节。虽然传统的数据库操作方式(如直接编写SQL语句与psycopg2交互)赋予了我们精细的控制权,但在面对日益复杂的业务逻辑和快速迭代的需求时,这种方式的开发效率和可…...
1688商品列表API与其他数据源的对接思路
将1688商品列表API与其他数据源对接时,需结合业务场景设计数据流转链路,重点关注数据格式兼容性、接口调用频率控制及数据一致性维护。以下是具体对接思路及关键技术点: 一、核心对接场景与目标 商品数据同步 场景:将1688商品信息…...
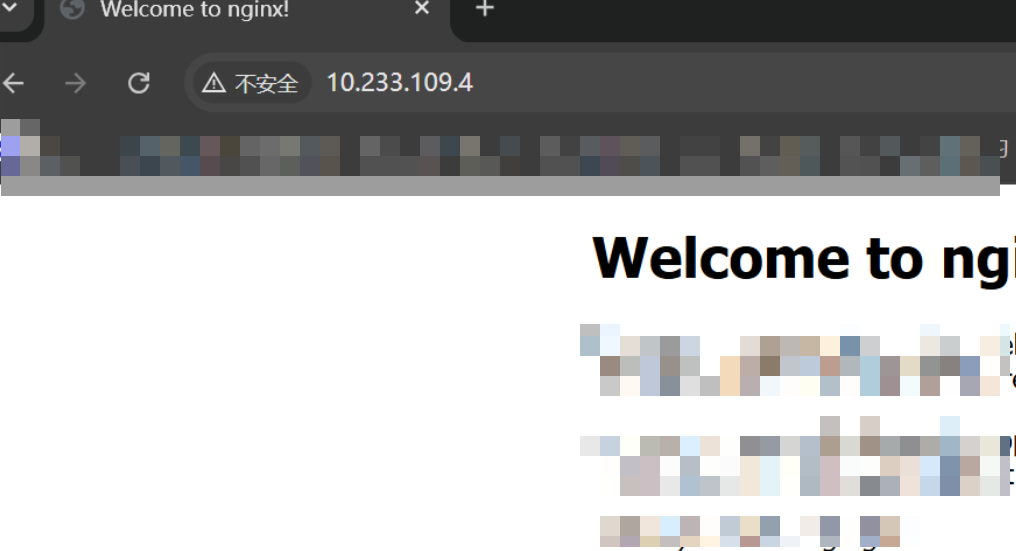
k8s业务程序联调工具-KtConnect
概述 原理 工具作用是建立了一个从本地到集群的单向VPN,根据VPN原理,打通两个内网必然需要借助一个公共中继节点,ktconnect工具巧妙的利用k8s原生的portforward能力,简化了建立连接的过程,apiserver间接起到了中继节…...
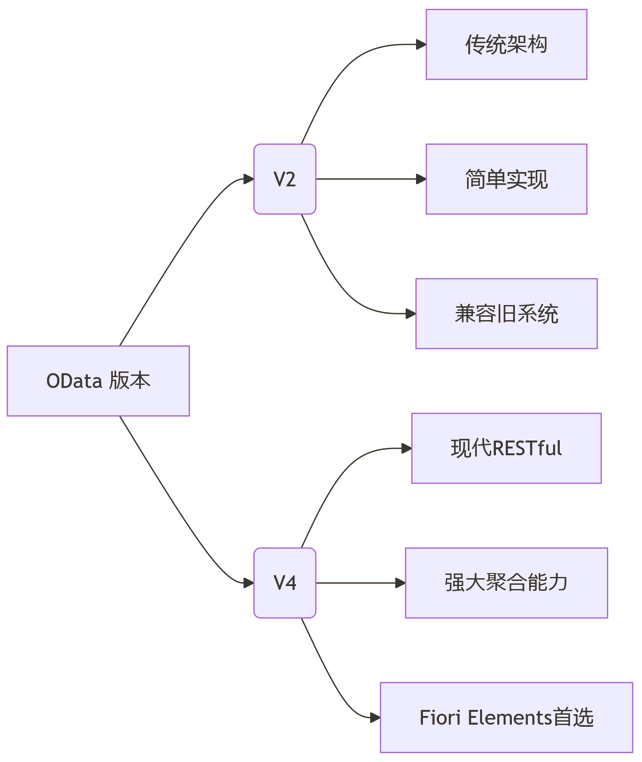
SAP学习笔记 - 开发26 - 前端Fiori开发 OData V2 和 V4 的差异 (Deepseek整理)
上一章用到了V2 的概念,其实 Fiori当中还有 V4,咱们这一章来总结一下 V2 和 V4。 SAP学习笔记 - 开发25 - 前端Fiori开发 Remote OData Service(使用远端Odata服务),代理中间件(ui5-middleware-simpleproxy)-CSDN博客…...
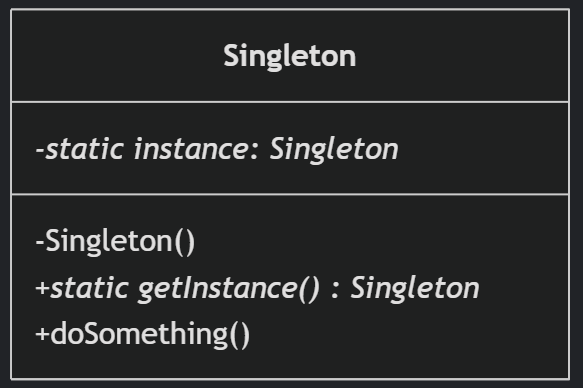
(一)单例模式
一、前言 单例模式属于六大创建型模式,即在软件设计过程中,主要关注创建对象的结果,并不关心创建对象的过程及细节。创建型设计模式将类对象的实例化过程进行抽象化接口设计,从而隐藏了类对象的实例是如何被创建的,封装了软件系统使用的具体对象类型。 六大创建型模式包括…...
为什么要创建 Vue 实例
核心原因:Vue 需要一个「控制中心」来驱动整个应用 你可以把 Vue 实例想象成你应用的**「大脑」或「引擎」。它负责协调模板、数据、逻辑和行为,将它们变成一个活的、可交互的应用**。没有这个实例,你的代码只是一堆静态的 HTML、JavaScript 变量和函数,无法「活」起来。 …...
WebRTC从入门到实践 - 零基础教程
WebRTC从入门到实践 - 零基础教程 目录 WebRTC简介 基础概念 工作原理 开发环境搭建 基础实践 三个实战案例 常见问题解答 1. WebRTC简介 1.1 什么是WebRTC? WebRTC(Web Real-Time Communication)是一个支持网页浏览器进行实时语音…...
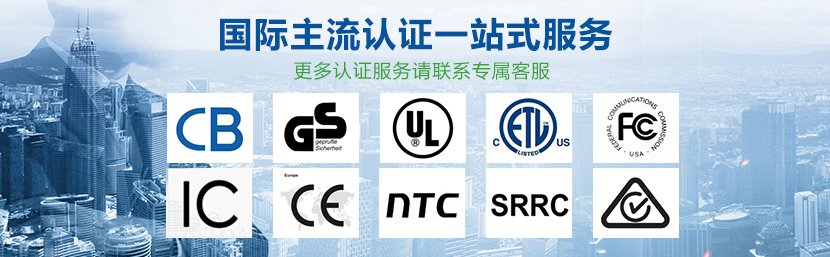
手机平板能效生态设计指令EU 2023/1670标准解读
手机平板能效生态设计指令EU 2023/1670标准解读 以下是针对欧盟《手机和平板电脑生态设计法规》(EU) 2023/1670 的核心解读,综合法规核心要求、最新修正及企业合规要点: 一、法规背景与目标 生效与强制时间 发布于2023年8月31日(OJ公报&…...
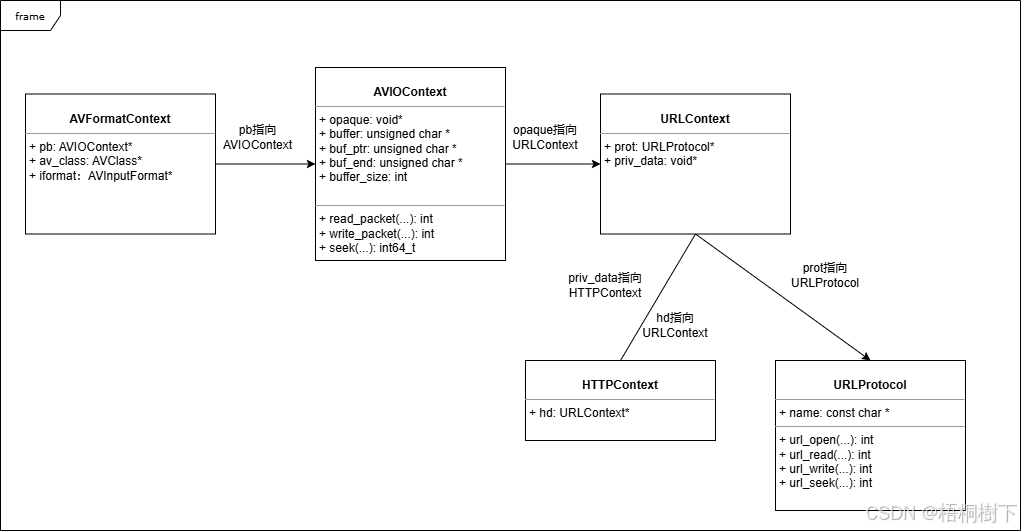
FFmpeg avformat_open_input函数分析
函数内部的总体流程如下: avformat_open_input 精简后的代码如下: int avformat_open_input(AVFormatContext **ps, const char *filename,ff_const59 AVInputFormat *fmt, AVDictionary **options) {AVFormatContext *s *ps;int i, ret 0;AVDictio…...
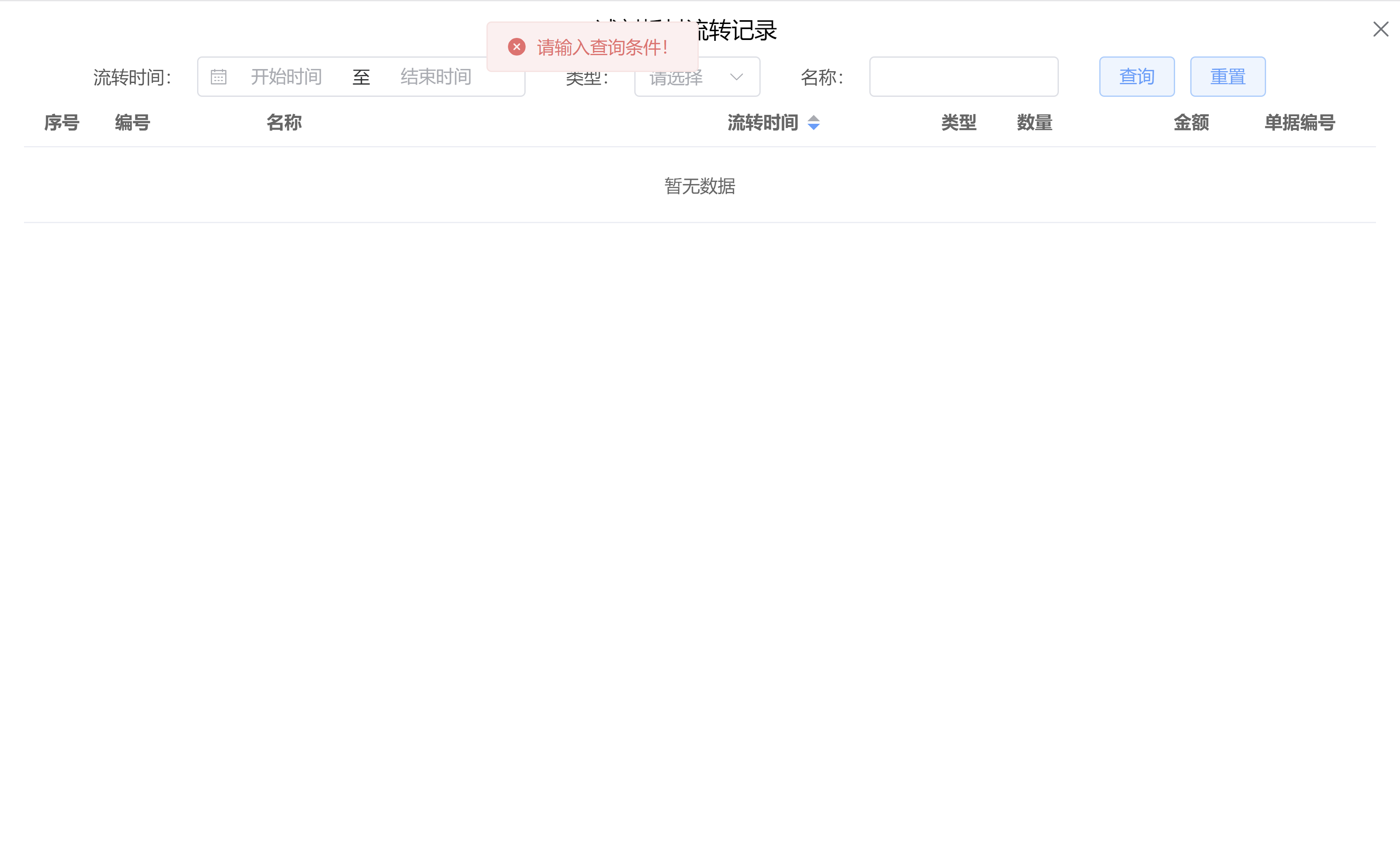
Java后端检查空条件查询
通过抛出运行异常:throw new RuntimeException("请输入查询条件!");BranchWarehouseServiceImpl.java // 查询试剂交易(入库/出库)记录Overridepublic List<BranchWarehouseTransactions> queryForReagent(Branch…...