科研学习|论文解读——智能体最新研究进展
从2024-12-13到2024-12-18的45篇文章中精选出5篇优秀的工作分享
-
Can Modern LLMs Act as Agent Cores in Radiology~Environments?
-
Achieving Collective Welfare in Multi-Agent Reinforcement Learning via Suggestion Sharing
-
A systematic review of norm emergence in multi-agent systems
-
Agent-based Video Trimming
-
GROOT-2: Weakly Supervised Multi-Modal Instruction Following Agents
一、Can Modern LLMs Act as Agent Cores in Radiology~Environments?
Authors: Qiaoyu Zheng, Chaoyi Wu, Pengcheng Qiu, Lisong Dai, Ya Zhang, Yanfeng Wang, Weidi Xie
https://arxiv.org/abs/2412.09529
1.1 论文摘要
Advancements in large language models (LLMs) have paved the way for LLM-based agent systems that offer enhanced accuracy and interpretability across various domains. Radiology, with its complex analytical requirements, is an ideal field for the application of these agents. This paper aims to investigate the pre-requisite question for building concrete radiology agents which is, ‘Can modern LLMs act as agent cores in radiology environments?’ To investigate it, we introduce RadABench with three-fold contributions: First, we present RadABench-Data, a comprehensive synthetic evaluation dataset for LLM-based agents, generated from an extensive taxonomy encompassing 6 anatomies, 5 imaging modalities, 10 tool categories, and 11 radiology tasks. Second, we propose RadABench-EvalPlat, a novel evaluation platform for agents featuring a prompt-driven workflow and the capability to simulate a wide range of radiology toolsets. Third, we assess the performance of 7 leading LLMs on our benchmark from 5 perspectives with multiple metrics. Our findings indicate that while current LLMs demonstrate strong capabilities in many areas, they are still not sufficiently advanced to serve as the central agent core in a fully operational radiology agent system. Additionally, we identify key factors influencing the performance of LLM-based agent cores, offering insights for clinicians on how to apply agent systems in real-world radiology practices effectively. All of our code and data are open-sourced in https://github.com/MAGIC-AI4Med/RadABench.
1.2 论文简评
这篇论文评估了现代大型语言模型(LLM)作为医学影像环境中代理核心的潜力。研究中提出了一个名为RadABench的数据集,包含复杂的放射学任务,并引入了一个新的评估平台,即RadABench-EvalPlat。论文通过评估7个领先的LLM,揭示了它们在特定放射学任务方面的强项和弱点。 文章的关键点在于以下几点:首先,引入的RadABench-Data为评估LLM在放射学环境中的能力提供了全面且结构化的数据,这对于理解LLM在这一领域表现至关重要;其次,提出的新评估平台RadABench-EvalPlat模拟真实的临床场景,为评估LLM的能力提供了一套详细的框架,用于分解任务、选择工具和生成响应;最后,对多个LLM的系统性性能分析提供了宝贵的见解,有助于了解当前限制和潜在的改进空间,以支持临床应用。综上所述,这篇文章深入探讨了现代大型语言模型在医疗影像领域的潜力,为未来的研究和实践提供了有价值的指导。
二、Achieving Collective Welfare in Multi-Agent Reinforcement Learning via Suggestion Sharing
Authors: Yue Jin, Shuangqing Wei, Giovanni Montana
https://arxiv.org/abs/2412.12326
2.1 论文摘要
In human society, the conflict between self-interest and collective well-being often obstructs efforts to achieve shared welfare. Related concepts like the Tragedy of the Commons and Social Dilemmas frequently manifest in our daily lives. As artificial agents increasingly serve as autonomous proxies for humans, we propose using multi-agent reinforcement learning (MARL) to address this issue—learning policies to maximise collective returns even when individual agents' interests conflict with the collective one. Traditional MARL solutions involve sharing rewards, values, and policies or designing intrinsic rewards to encourage agents to learn collectively optimal policies. We introduce a novel MARL approach based on Suggestion Sharing (SS), where agents exchange only action suggestions. This method enables effective cooperation without the need to design intrinsic rewards, achieving strong performance while revealing less private information compared to sharing rewards, values, or policies. Our theoretical analysis establishes a bound on the discrepancy between collective and individual objectives, demonstrating how sharing suggestions can align agents' behaviours with the collective objective. Experimental results demonstrate that SS performs competitively with baselines that rely on value or policy sharing or intrinsic rewards.
2.2 论文简评
Suggestion Sharing(SS)是多智能体强化学习(MARL)中的一种新颖策略,旨在通过协调各代理的利益来实现集体福利最大化。本文作者强调传统方法需共享奖励或政策的不足,并提出仅分享行动建议的新观点。理论分析与实证研究支持了SS的有效性,展示了其在多个环境中的竞争力,同时保护了隐私。这些成果为MARL领域提供了重要的贡献。总体而言,该文提出了一种创新的方法来促进合作,而无需共享敏感信息,从而增强了其在MARL领域的影响力。
三、A systematic review of norm emergence in multi-agent systems
Authors: Carmengelys Cordova, Joaquin Taverner, Elena Del Val, Estefania Argente
https://arxiv.org/abs/2412.10609
3.1 论文摘要
Multi-agent systems (MAS) have gained relevance in the field of artificial intelligence by offering tools for modelling complex environments where autonomous agents interact to achieve common or individual goals. In these systems, norms emerge as a fundamental component to regulate the behaviour of agents, promoting cooperation, coordination and conflict resolution. This article presents a systematic review, following the PRISMA method, on the emergence of norms in MAS, exploring the main mechanisms and factors that influence this process. Sociological, structural, emotional and cognitive aspects that facilitate the creation, propagation and reinforcement of norms are addressed. The findings highlight the crucial role of social network topology, as well as the importance of emotions and shared values in the adoption and maintenance of norms. Furthermore, opportunities are identified for future research that more explicitly integrates emotional and ethical dynamics in the design of adaptive normative systems. This work provides a comprehensive overview of the current state of research on norm emergence in MAS, serving as a basis for advancing the development of more efficient and flexible systems in artificial and real-world contexts.
3.2 论文简评
这篇论文是关于多代理系统(MAS)中规范出现的系统性综述。作者采用PRISMA方法对该主题进行了深入研究,探讨了影响这一过程的各种机制和因素,包括社会学、结构、情感和认知等多个方面。此外,文中还强调了社交网络拓扑结构以及共同价值观对规范采纳和维护的重要性。通过全面的文献回顾,本文为相关领域的研究提供了有价值的信息,并有助于加深我们对规范出现现象的理解。总的来说,这篇文章是一个重要的研究成果,填补了这一领域的空白,并为其他学者的研究提供了良好的参考框架。
四、Agent-based Video Trimming
Authors: Lingfeng Yang, Zhenyuan Chen, Xiang Li, Peiyang Jia, Liangqu Long, Jian Yang
https://arxiv.org/abs/2412.09513
4.1 论文摘要
As information becomes more accessible, user-generated videos are increasing in length, placing a burden on viewers to sift through vast content for valuable insights. This trend underscores the need for an algorithm to extract key video information efficiently. Despite significant advancements in highlight detection, moment retrieval, and video summarization, current approaches primarily focus on selecting specific time intervals, often overlooking the relevance between segments and the potential for segment arranging. In this paper, we introduce a novel task calledVideo Trimming (VT), which focuses on detecting wasted footage, selecting valuable segments, and composing them into a final video with a coherent story. To address this task, we proposeAgent-based Video Trimming (AVT), structured into three phases: Video Structuring, Clip Filtering, and Story Composition. Specifically, we employ a Video Captioning Agent to convert video slices into structured textual descriptions, a Filtering Module to dynamically discard low-quality footage based on the structured information of each clip, and a Video Arrangement Agent to select and compile valid clips into a coherent final narrative. For evaluation, we develop a Video Evaluation Agent to assess trimmed videos, conducting assessments in parallel with human evaluations. Additionally, we curate a new benchmark dataset for video trimming using raw user videos from the internet. As a result, AVT received more favorable evaluations in user studies and demonstrated superior mAP and precision on the YouTube Highlights, TVSum, and our own dataset for the highlight detection task. The code and models are available at https://ylingfeng.github.io/AVT.
4.2 论文简评
该篇论文关注一个新颖的任务——视频剪辑(Video Trimming, VT),提出了一种基于代理的剪辑框架(Agent-Based Video Trimming, AVT),该框架包括视频结构化、片段过滤和故事构建等阶段。目标是通过选择高价值片段来提高视频编辑的效率,并排除冗余素材,同时保持故事情节的一致性。研究者通过对现有亮点检测方法的评估,展示了AVT的有效性。
该文的主要优点在于对当前视频处理问题的关注,以及创新性地采用代理机制来解决视频编辑中的挑战。此外,用户研究和量化评估也显示了AVT相对于现有技术的有效性。总体而言,这篇论文为视频编辑领域提供了新的视角,具有重要的理论价值和实践意义。
五、GROOT-2: Weakly Supervised Multi-Modal Instruction Following Agents
Authors: Shaofei Cai, Bowei Zhang, Zihao Wang, Haowei Lin, Xiaojian Ma, Anji Liu, Yitao Liang
https://arxiv.org/abs/2412.10410
5.1 论文摘要
Developing agents that can follow multimodal instructions remains a fundamental challenge in robotics and AI. Although large-scale pre-training on unlabeled datasets (no language instruction) has enabled agents to learn diverse behaviors, these agents often struggle with following instructions. While augment ing the dataset with instruction labels can mitigate this issue, acquiring such high-quality annotations at scale is impractical. To address this issue, we frame the problem as a semi-supervised learning task and introduce GROOT-2, a multimodal instructable agent trained using a novel approach that combines weak supervision with latent variable models. Our method consists of two key components: constrained self-imitating, which utilizes large amounts of unlabeled demonstrations to enable the policy to learn diverse behaviors, and human intention alignment, which uses a smaller set of labeled demonstrations to ensure the latent space reflects human intentions. GROOT-2’s effectiveness is validated across four diverse environments, ranging from video games to robotic manipulation, demonstrating its robust multimodal instruction-following capabilities.
5.2 论文简评
GROOT-2是一种创新的多模态指令跟随代理,旨在解决在复杂环境中获取高质量标注的问题。该工作的主要贡献在于利用自我模仿学习与人类意图对齐的策略,提升代理的表现。通过利用大量未标记数据,所提方法显著提高了代理的训练效率和效果。研究表明,GROOT-2在多种场景中的广泛适用性,显示出对未来人工智能和机器人研究的潜在影响。总体而言,本文全面探讨了多模态指令跟随领域面临的挑战,并通过实施GROOT-2提供了有希望的解决方案。
相关文章:
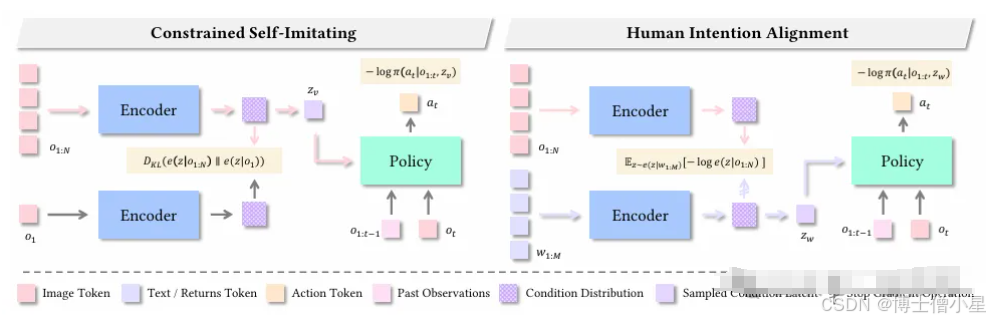
科研学习|论文解读——智能体最新研究进展
从2024-12-13到2024-12-18的45篇文章中精选出5篇优秀的工作分享 Can Modern LLMs Act as Agent Cores in Radiology~Environments? Achieving Collective Welfare in Multi-Agent Reinforcement Learning via Suggestion Sharing A systematic review of norm emergence in …...
面试小札:Java后端闪电五连鞭_8
1. Kafka消息模型及其组成部分 - 消息(Message):是Kafka中最基本的数据单元。消息包含一个键(key)、一个值(value)和一个时间戳(timestamp)。键可以用于对消息进行分区等…...
java error(2)保存时间带时分秒,回显时分秒变成00:00:00
超简单,顺带记录一下 1.入参实体类上使用注释:JsonFormat(pattern “yyyy-MM-dd”) 导致舍弃了 时分秒的部分。 2.数据库字段对应的类型是 date。date就是日期,日期就不带时分秒。 3.返参实体类使用了JsonFormat(pattern “yyyy-MM-dd”) 导…...
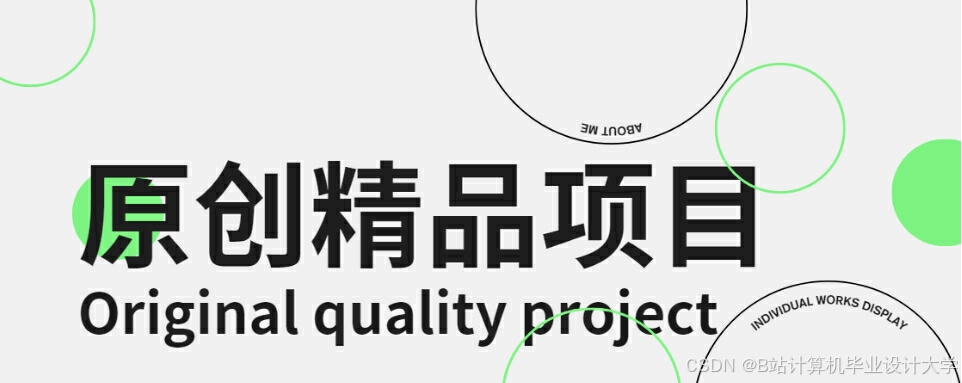
计算机毕业设计python+spark+hive动漫推荐系统 漫画推荐系统 漫画分析可视化大屏 漫画爬虫 漫画推荐系统 漫画爬虫 知识图谱 大数据毕设
温馨提示:文末有 CSDN 平台官方提供的学长联系方式的名片! 温馨提示:文末有 CSDN 平台官方提供的学长联系方式的名片! 温馨提示:文末有 CSDN 平台官方提供的学长联系方式的名片! 作者简介:Java领…...
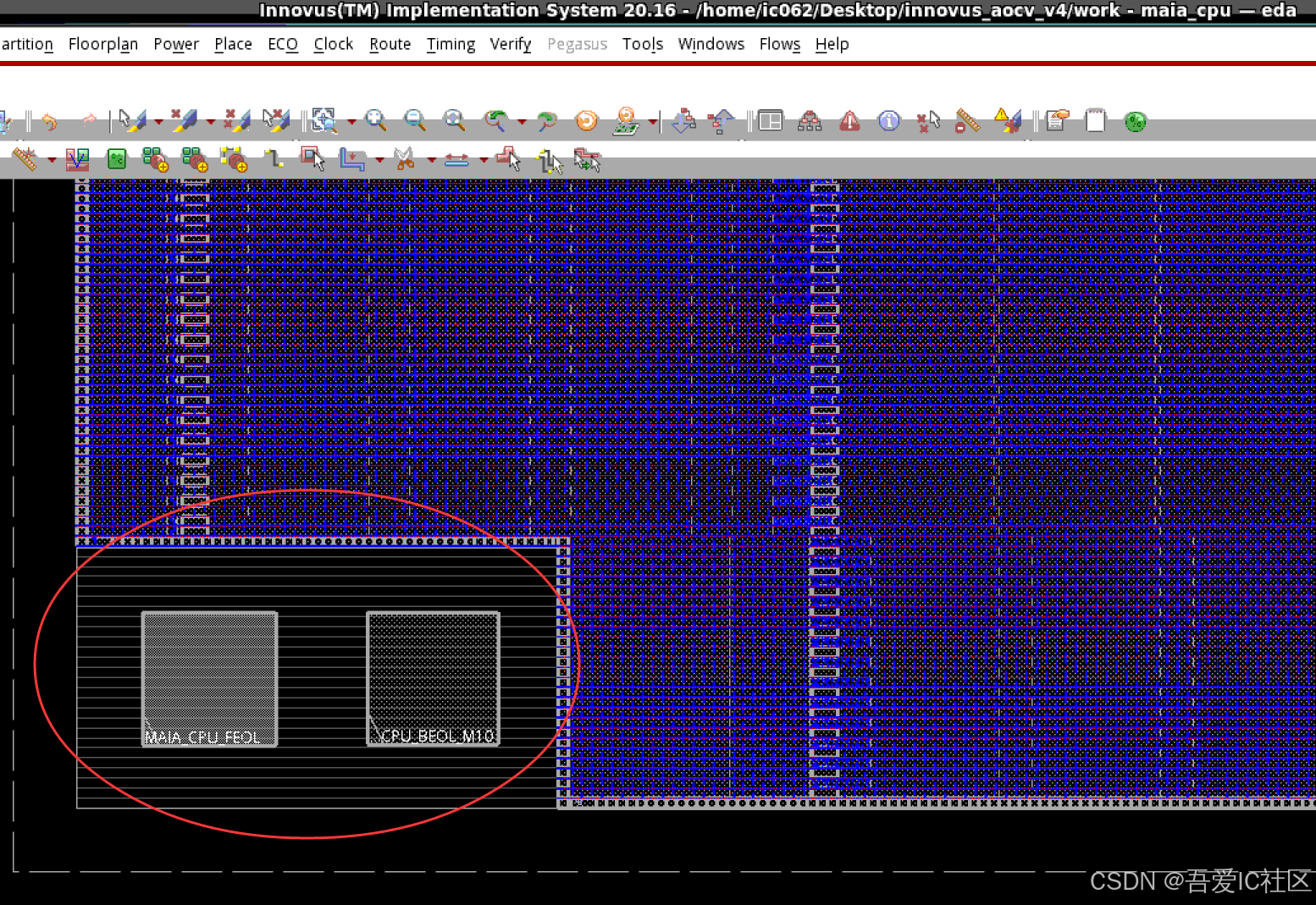
数字IC后端设计实现篇之TSMC 12nm TCD cell(Dummy TCD Cell)应该怎么加?
TSMC 12nm A72项目我们需要按照foundary的要求提前在floorplan阶段加好TCD Cell。这个cell是用来做工艺校准的。这个dummy TCD Cell也可以等后续Calibre 插dummy自动插。但咱们项目要求提前在floorplan阶段就先预先规划好位置。 TSCM12nm 1P9M的metal stack结构图如下图所示。…...
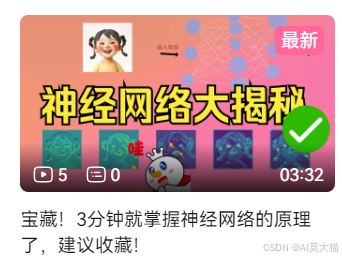
(8)YOLOv6算法基本原理
一、YOLOv6 模型原理 发布日期:2022年6月 作者:美团技术团队 骨干网络:参考了 RepVGG 的设计,将重参数化能力进行补强,增强了模型结构的重参数化能力。使用了深度可分离卷积和跨阶段连接等技术,旨在提升…...
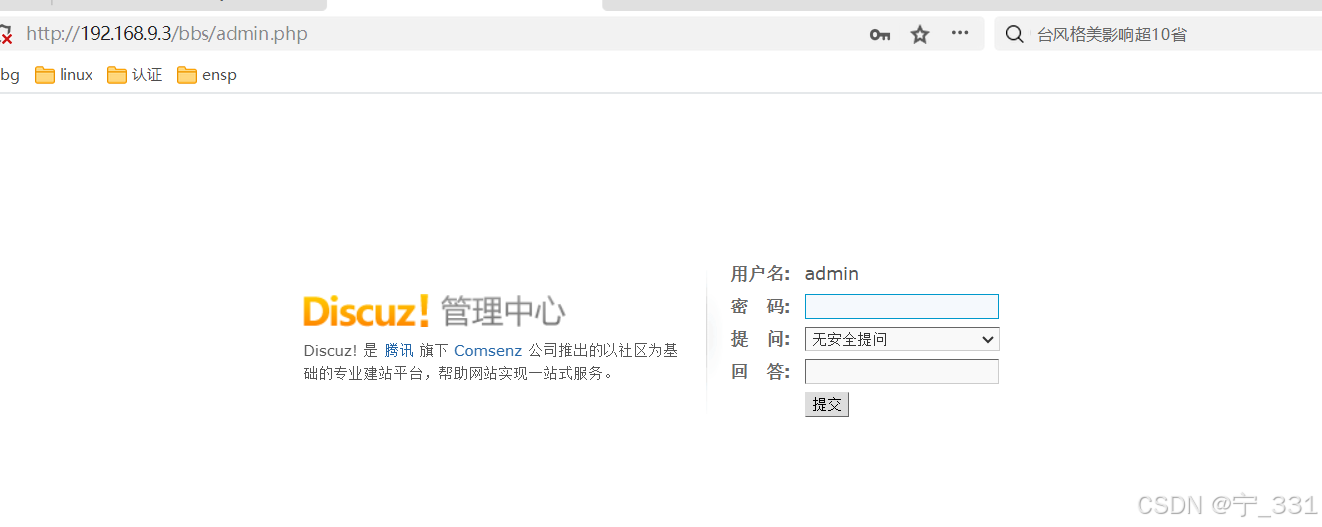
LNMP+discuz论坛
0.准备 文章目录 0.准备1.nginx2.mysql2.1 mysql82.2 mysql5.7 3.php4.测试php访问mysql5.部署 Discuz6.其他 yum源: # 没有wget,用这个 # curl -o /etc/yum.repos.d/CentOS-Base.repo https://mirrors.aliyun.com/repo/Centos-7.repo[rootlocalhost ~]#…...
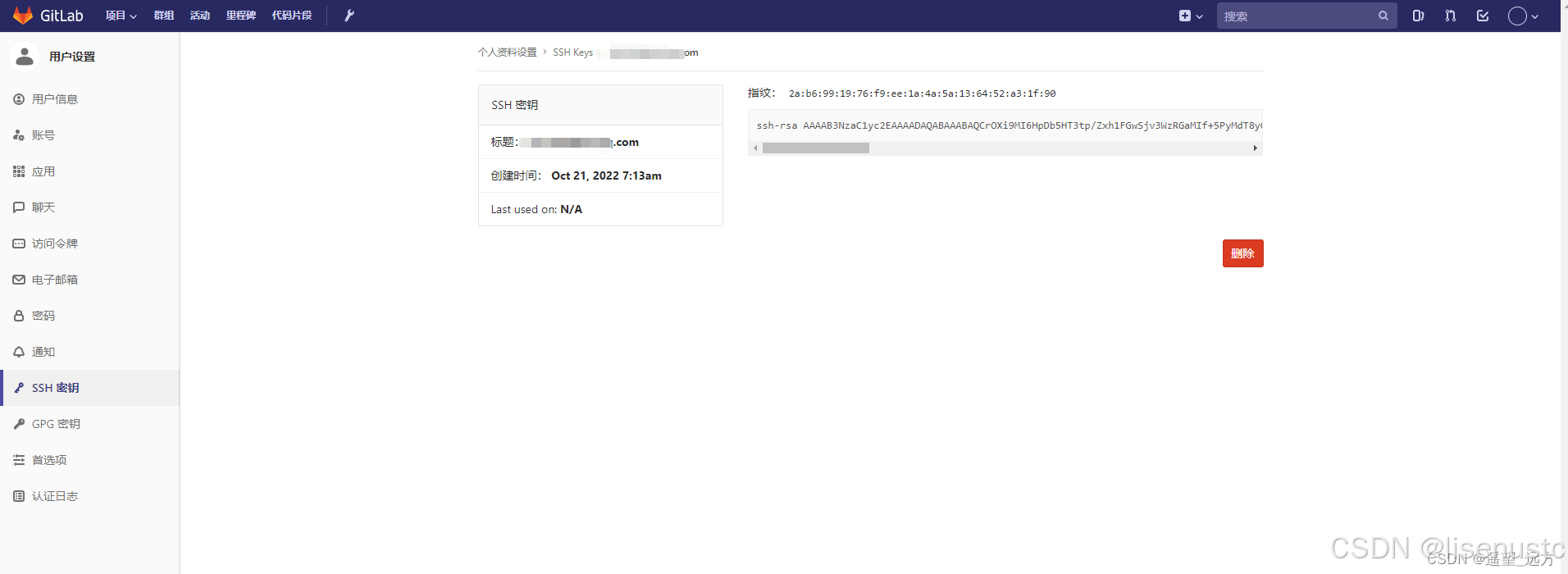
在linux系统的docker中安装GitLab
一、安装GitLab: 在安装了docker之后就是下载安装GitLab了,在linux系统中输入命令:docker search gitlab就可以看到很多项目,一般安装第一个,它是英文版的,如果英文不好可以安装twang2218/gitlab-ce-zh。 …...
Python面试常见问题及答案12
问题: 请解释Python中的GIL(全局解释器锁)是什么? ○ 答案: GIL是Python解释器中的一种机制,用于确保任何时候只有一个线程在执行Python字节码。这在多线程场景下可能影响性能优化,但对于单线程…...
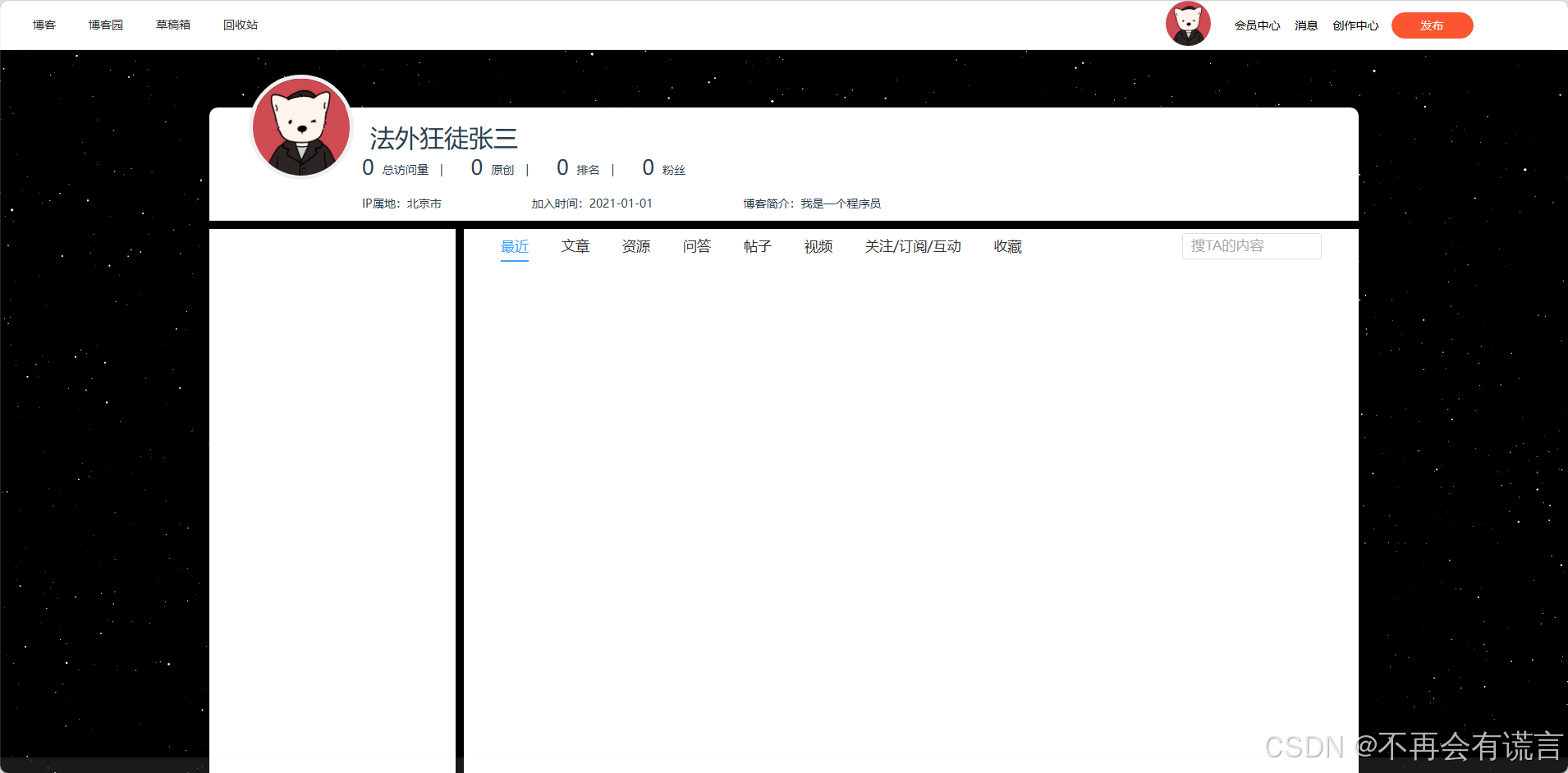
从0-1开发一个Vue3前端系统页面-9.博客页面布局
本节主要实现了博客首页界面的基本布局并完善了响应式布局,因为完善了响应式布局故对前面的页面布局有所改动,这里会将改动后的源码同步上传。 1.对页面头部的用户信息进行设计和美化 布局设计参考 :通常初级前端的布局会通过多个div划分区域…...

[手机Linux] 六,ubuntu18.04私有网盘(NextCloud)安装
一,LNMP介绍 LNMP一键安装包是一个用Linux Shell编写的可以为CentOS/RHEL/Fedora/Debian/Ubuntu/Raspbian/Deepin/Alibaba/Amazon/Mint/Oracle/Rocky/Alma/Kali/UOS/银河麒麟/openEuler/Anolis OS Linux VPS或独立主机安装LNMP(Nginx/MySQL/PHP)、LNMPA(Nginx/MySQ…...
白话java设计模式
创建模式 单例模式(Singleton Pattern): 就是一次创建多次使用,它的对象不会重复创建,可以全局来共享状态。 工厂模式(Factory Method Pattern): 可以通过接口来进行实例化创建&a…...
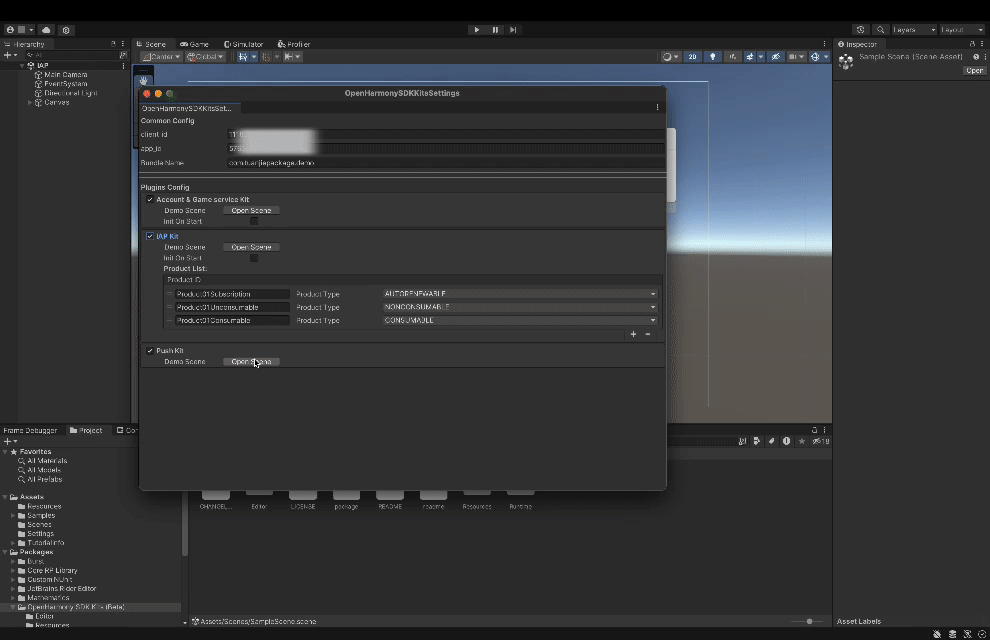
助力 Tuanjie OpenHarmony 开发:如何使用工具包 Hilog 和 SDK Kits Package?
随着团结引擎从 1.0.0 迭代至 1.3.0,越来越多的开发者开始使用团结引擎开发 OpenHarmony 应用。 在开发的过程中,我们也收到了大量反馈,尤其是在日志、堆栈和性能数据方面,这些信息对开发和调试过程至关重要。同时,我…...
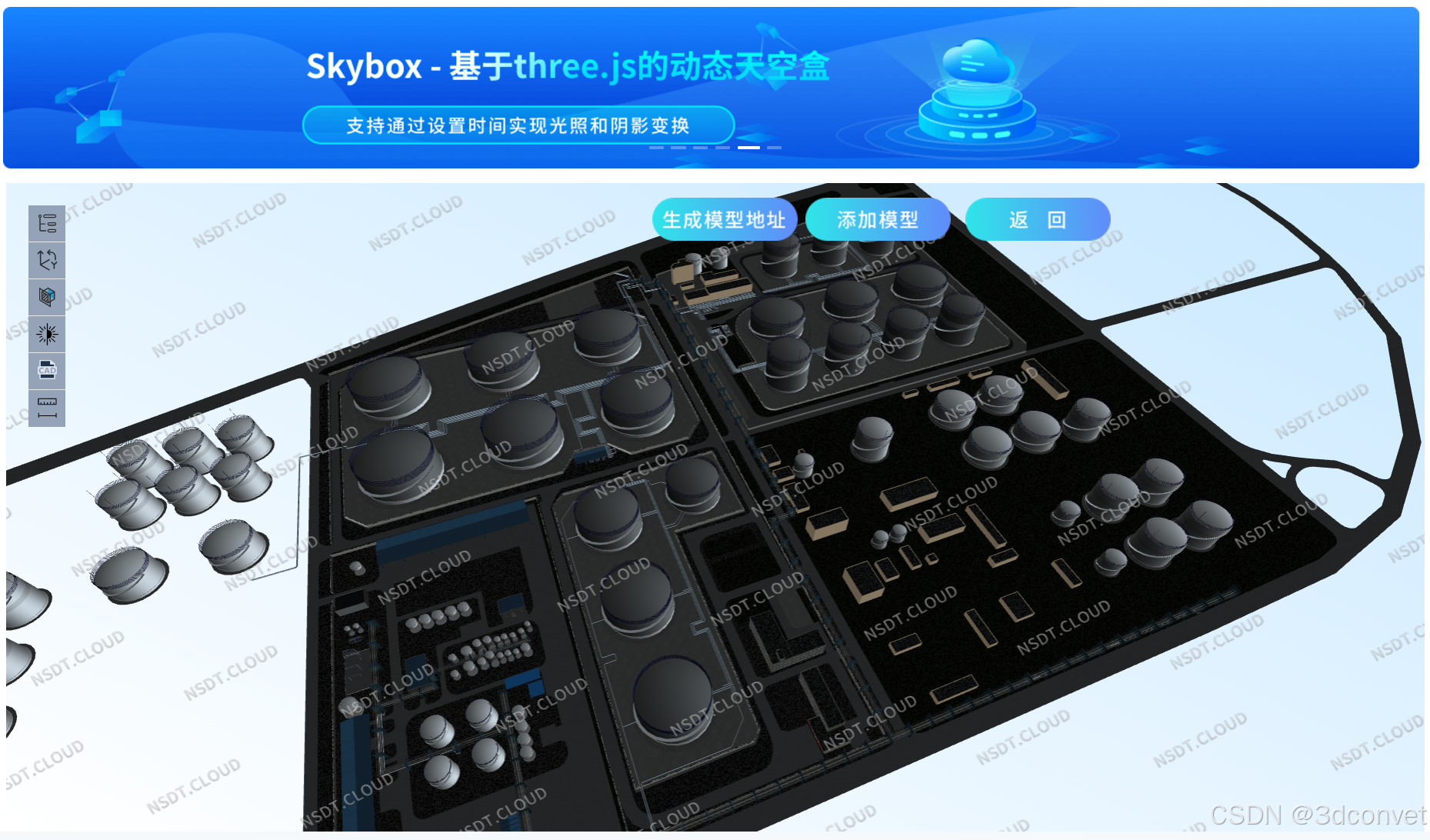
NSDT 3DConvert:高效实现大模型文件在线预览与转换
NSDT 3DConvert 作为一个 WebGL 展示平台,能够实现多种模型格式免费在线预览,并支持大于1GB的OBJ、STL、GLTF、点云等模型进行在线查看与交互,这在3D模型展示领域是一个相当强大的功能。 平台特点 多格式支持 NSDT 3DConvert兼容多种3D模型…...
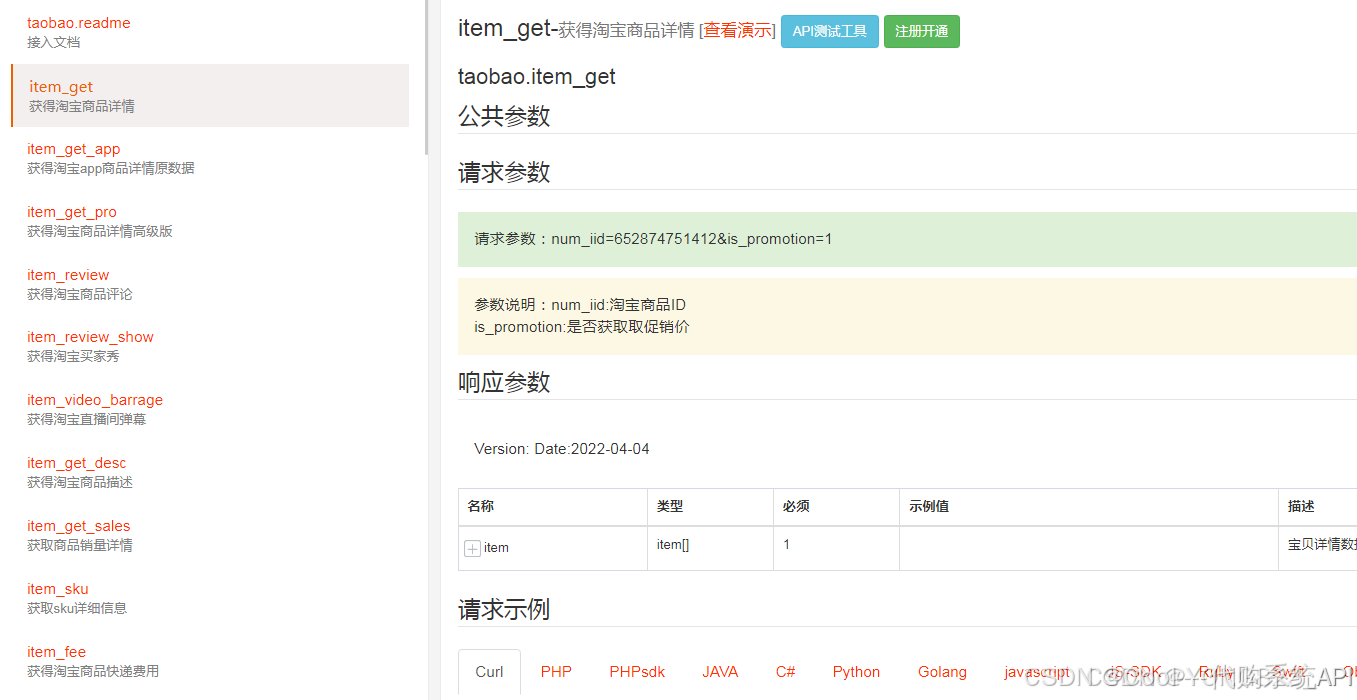
电商数据采集电商,行业数据分析,平台数据获取|稳定的API接口数据
电商数据采集可以通过多种方式完成,其中包括人工采集、使用电商平台提供的API接口、以及利用爬虫技术等自动化工具。以下是一些常用的电商数据采集方法: 人工采集:人工采集主要是通过基本的“复制粘贴”的方式在电商平台上进行数据的收集&am…...
VUE+Node.js+mysq实现响应式个人博客|项目初始化+路由配置+基础组件搭建
Day 1 开发文档:项目初始化与基础架构搭建 一、项目初始化 1. 创建项目 首先,我们使用 Vite 创建一个基于 Vue 3 的项目: # 创建项目 npm create vitelatest my-blog -- --template vue # 这条命令会创建一个名为 my-blog 的新项目&#…...
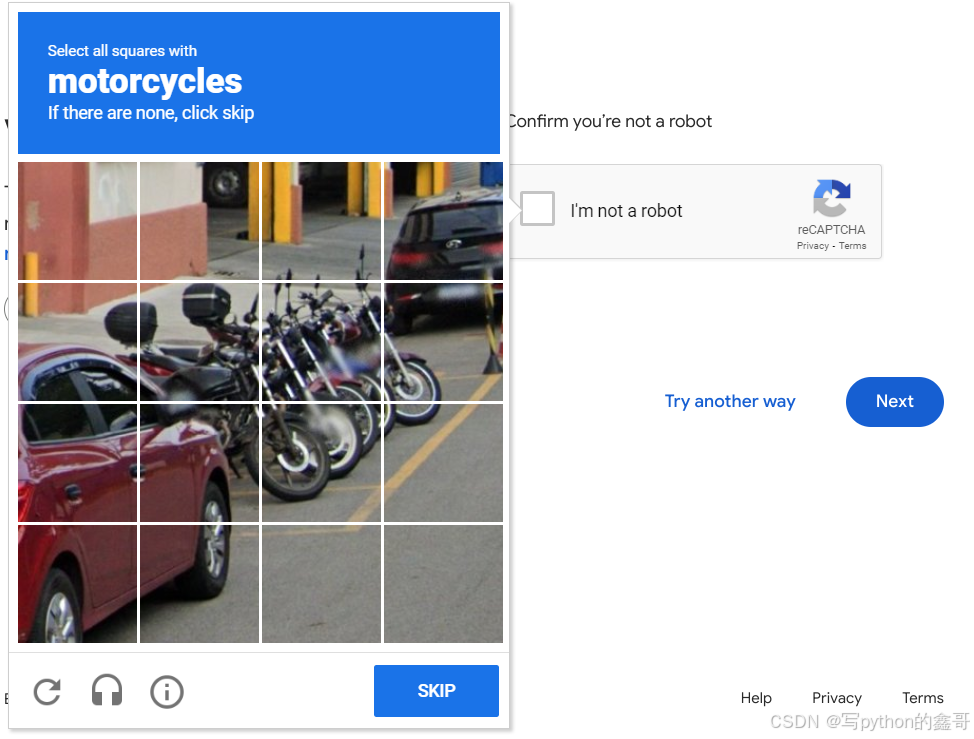
Python如何正确解决reCaptcha验证码(9)
前言 本文是该专栏的第73篇,后面会持续分享python爬虫干货知识,记得关注。 我们在处理某些国内外平台项目的时候,相信很多同学或多或少都见过,如下图所示的reCaptcha验证码。 而本文,笔者将重点来介绍在实战项目中,遇到上述中的“reCaptcha验证码”,如何正确去处理并解…...
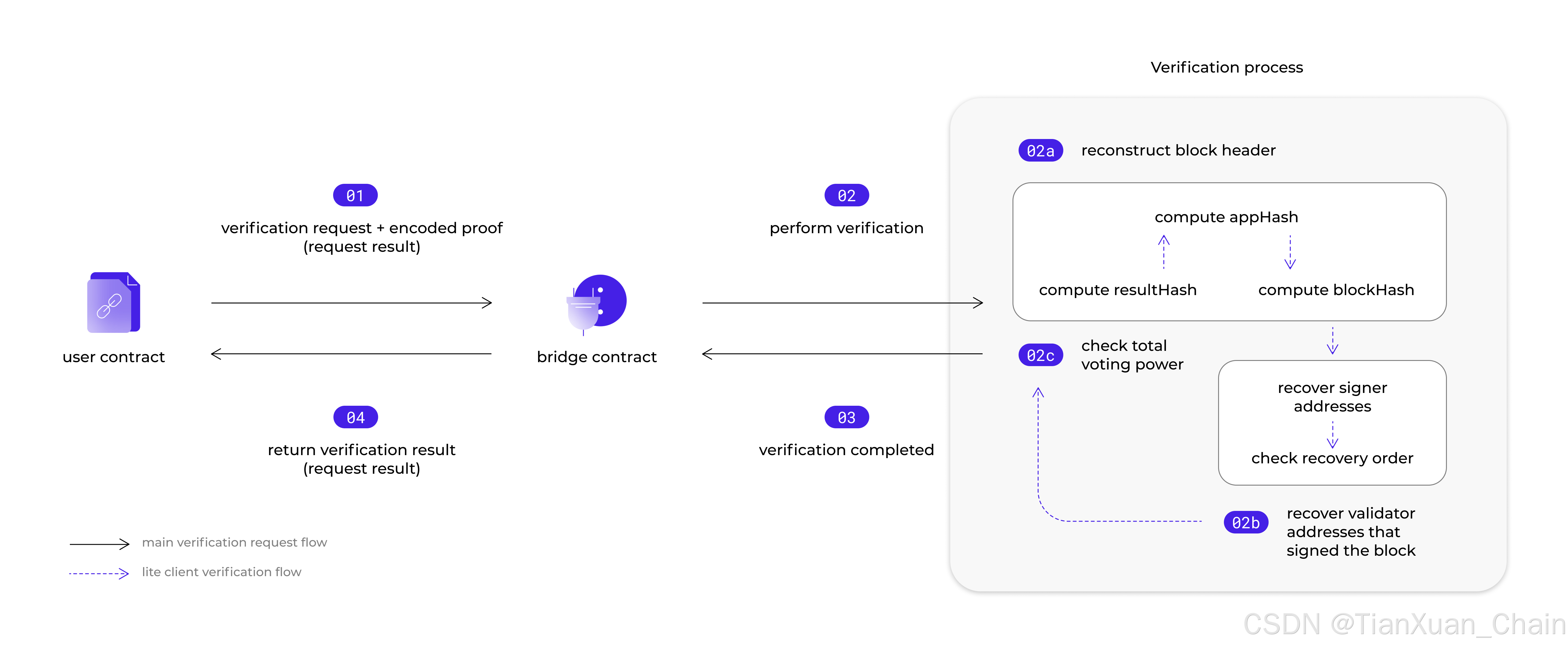
web3跨链预言机协议-BandProtocol
项目简介 Band Protocol 项目最初于 2017年成立并建立在 ETH 之上。后于2020年转移到了 Cosmos 网络上,基于 Cosmos SDK 搭建了一条 Band Chain 。这是一条 oracle-specific chain,主要功能是提供跨链预言机服务。Cosmos生态上第一个,也是目…...
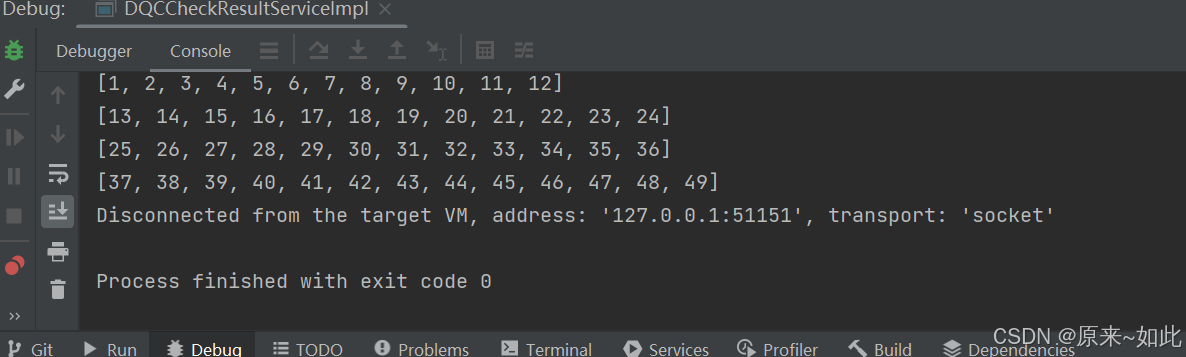
JAVA将集合切分成指定份数(简易)
JAVA将集合切分成指定份数 主要方法 /** * 主要方法* param list 切分的集合* param count 切成的份数* return*/ public static List<List> splitList(List list,int count){if(count <0 ){return Lists.newArrayList();}List<List> result Lists.newArrayL…...
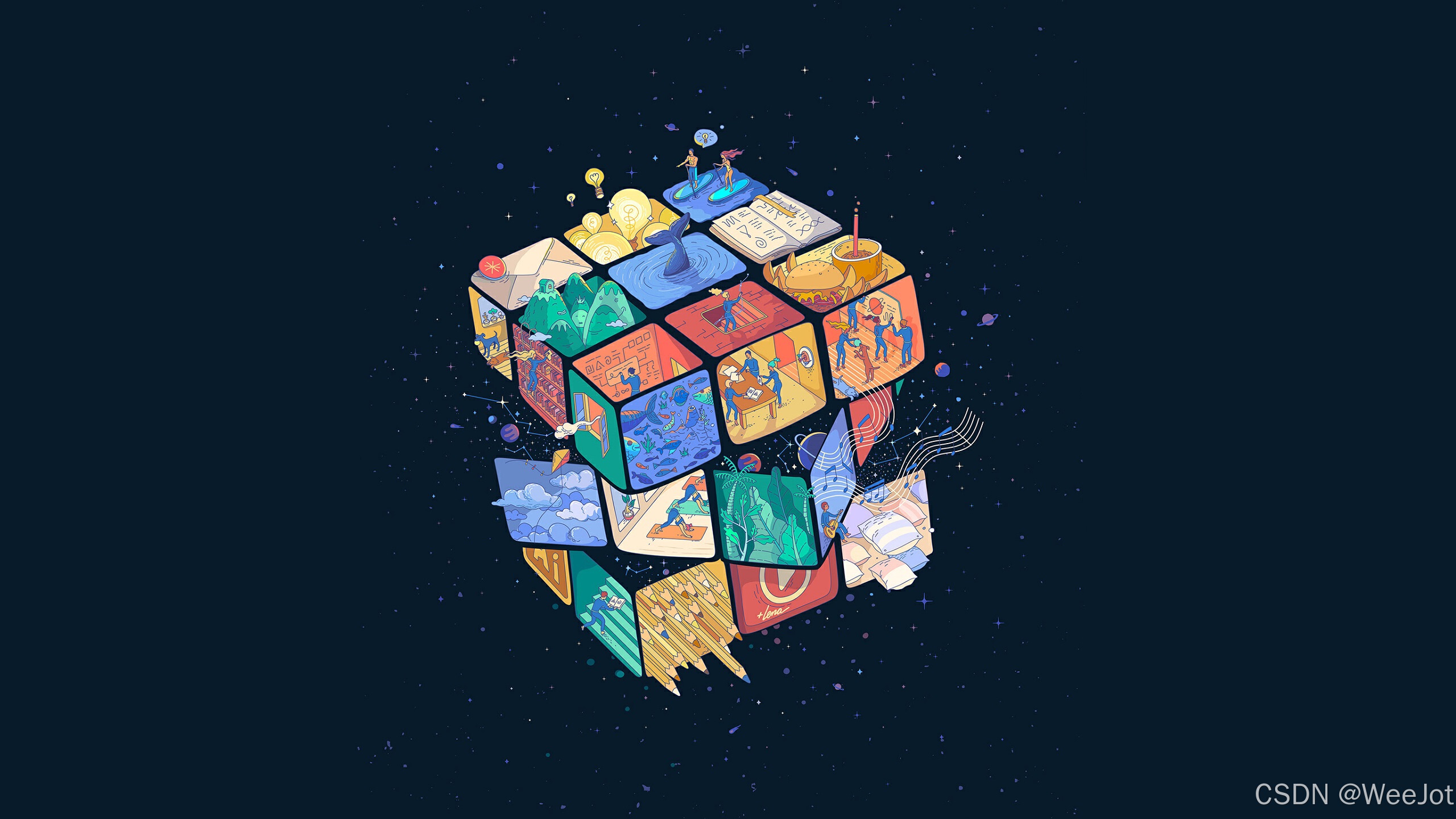
深度神经网络(DNN)在时序预测中的应用与缺陷
目录 编辑 一、DNN在时序预测中的应用 二、DNN的缺陷 三、技术挑战与未来趋势 四、结论 随着大数据时代的到来,深度学习技术在时序预测领域扮演着越来越重要的角色。深度神经网络(DNN)因其强大的非线性拟合能力和自动特征提取能力&…...
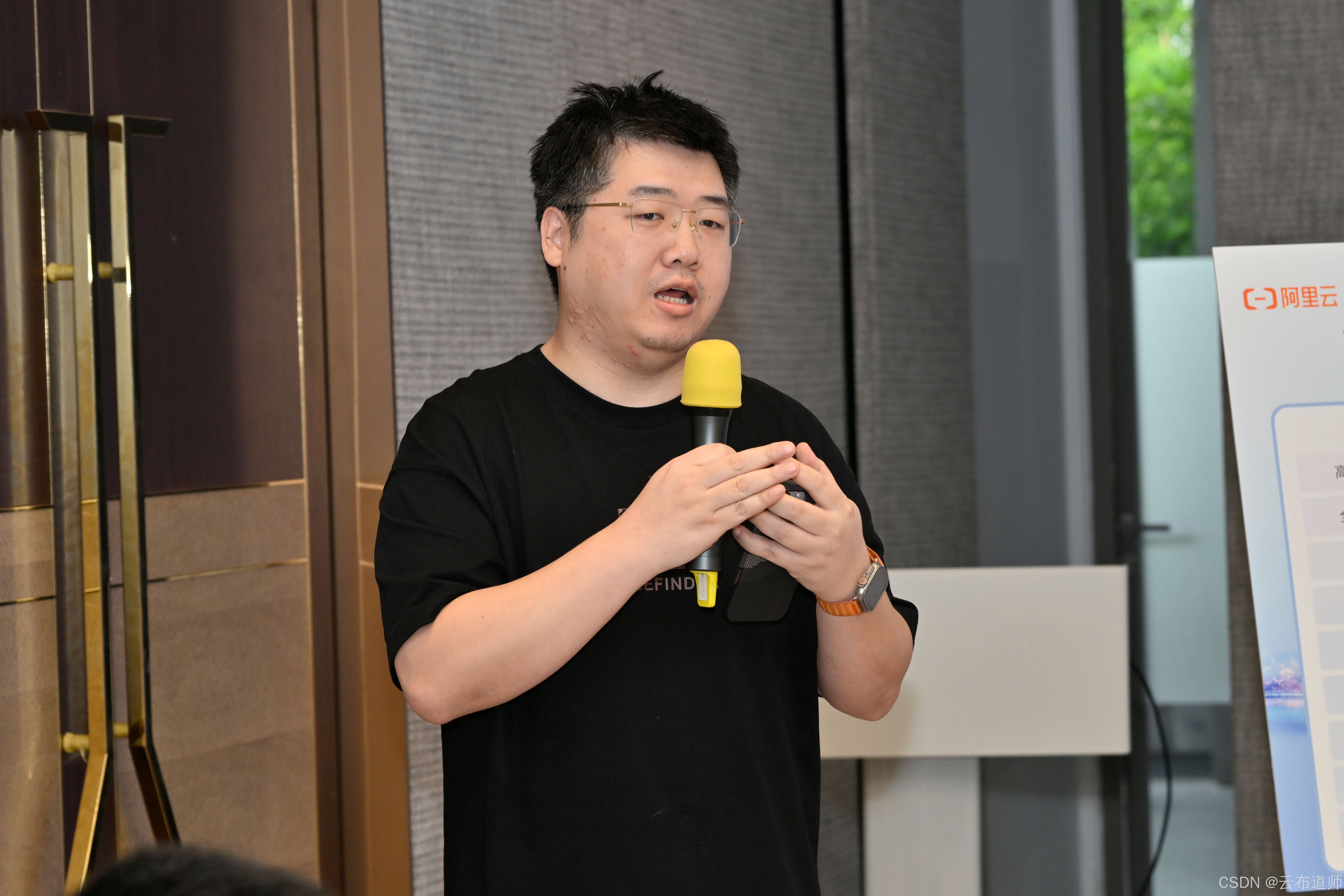
云启出海,智联未来|阿里云网络「企业出海」系列客户沙龙上海站圆满落地
借阿里云中企出海大会的东风,以**「云启出海,智联未来|打造安全可靠的出海云网络引擎」为主题的阿里云企业出海客户沙龙云网络&安全专场于5.28日下午在上海顺利举办,现场吸引了来自携程、小红书、米哈游、哔哩哔哩、波克城市、…...
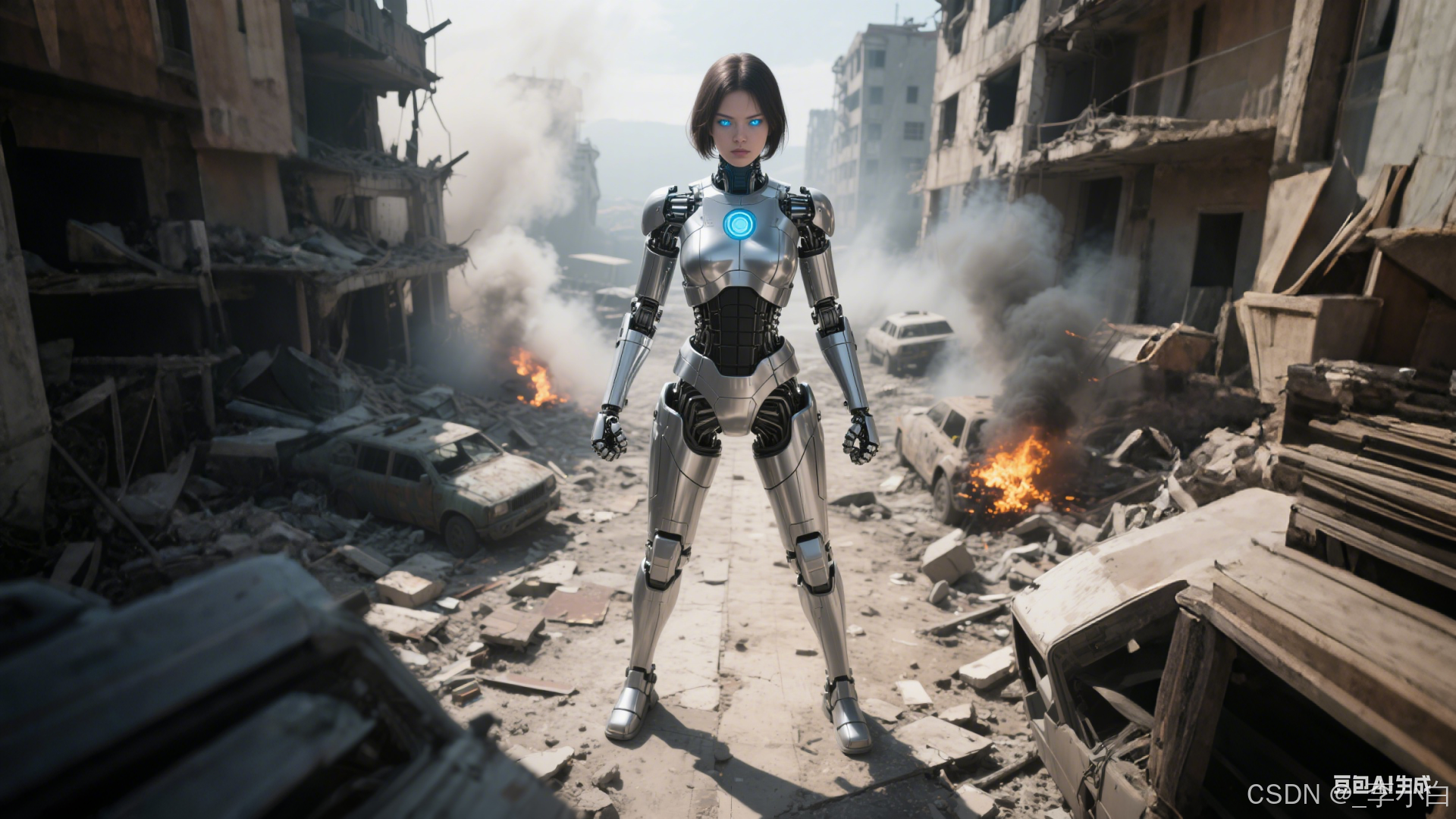
【OSG学习笔记】Day 16: 骨骼动画与蒙皮(osgAnimation)
骨骼动画基础 骨骼动画是 3D 计算机图形中常用的技术,它通过以下两个主要组件实现角色动画。 骨骼系统 (Skeleton):由层级结构的骨头组成,类似于人体骨骼蒙皮 (Mesh Skinning):将模型网格顶点绑定到骨骼上,使骨骼移动…...
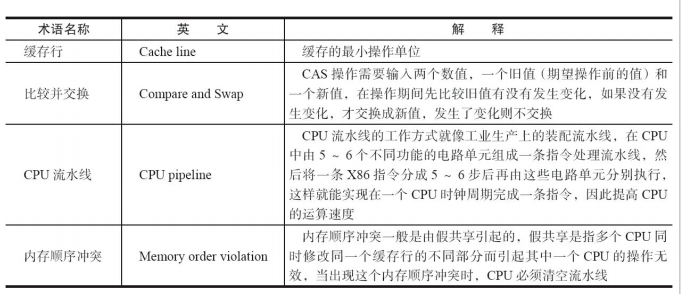
JUC笔记(上)-复习 涉及死锁 volatile synchronized CAS 原子操作
一、上下文切换 即使单核CPU也可以进行多线程执行代码,CPU会给每个线程分配CPU时间片来实现这个机制。时间片非常短,所以CPU会不断地切换线程执行,从而让我们感觉多个线程是同时执行的。时间片一般是十几毫秒(ms)。通过时间片分配算法执行。…...
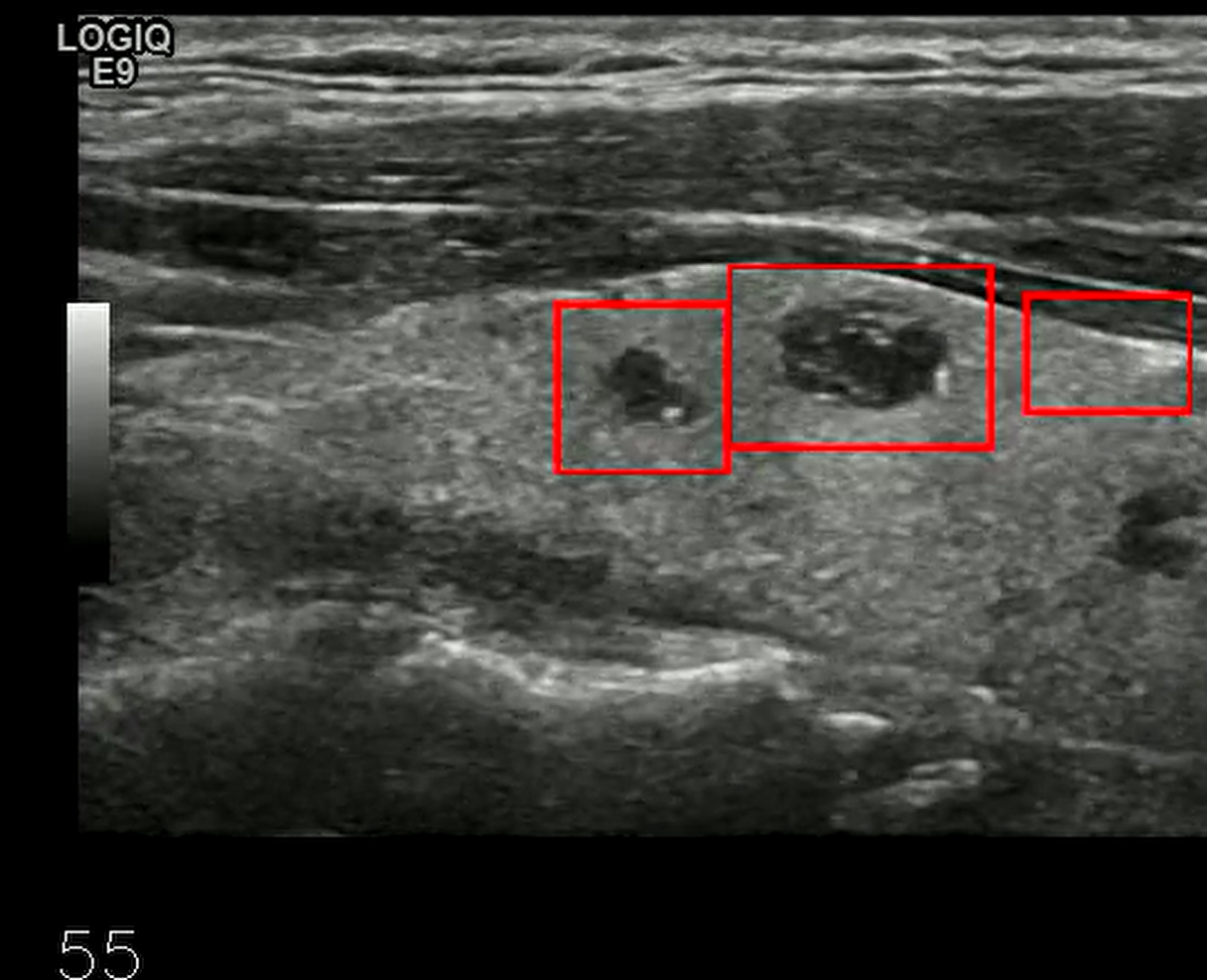
【开发技术】.Net使用FFmpeg视频特定帧上绘制内容
目录 一、目的 二、解决方案 2.1 什么是FFmpeg 2.2 FFmpeg主要功能 2.3 使用Xabe.FFmpeg调用FFmpeg功能 2.4 使用 FFmpeg 的 drawbox 滤镜来绘制 ROI 三、总结 一、目的 当前市场上有很多目标检测智能识别的相关算法,当前调用一个医疗行业的AI识别算法后返回…...
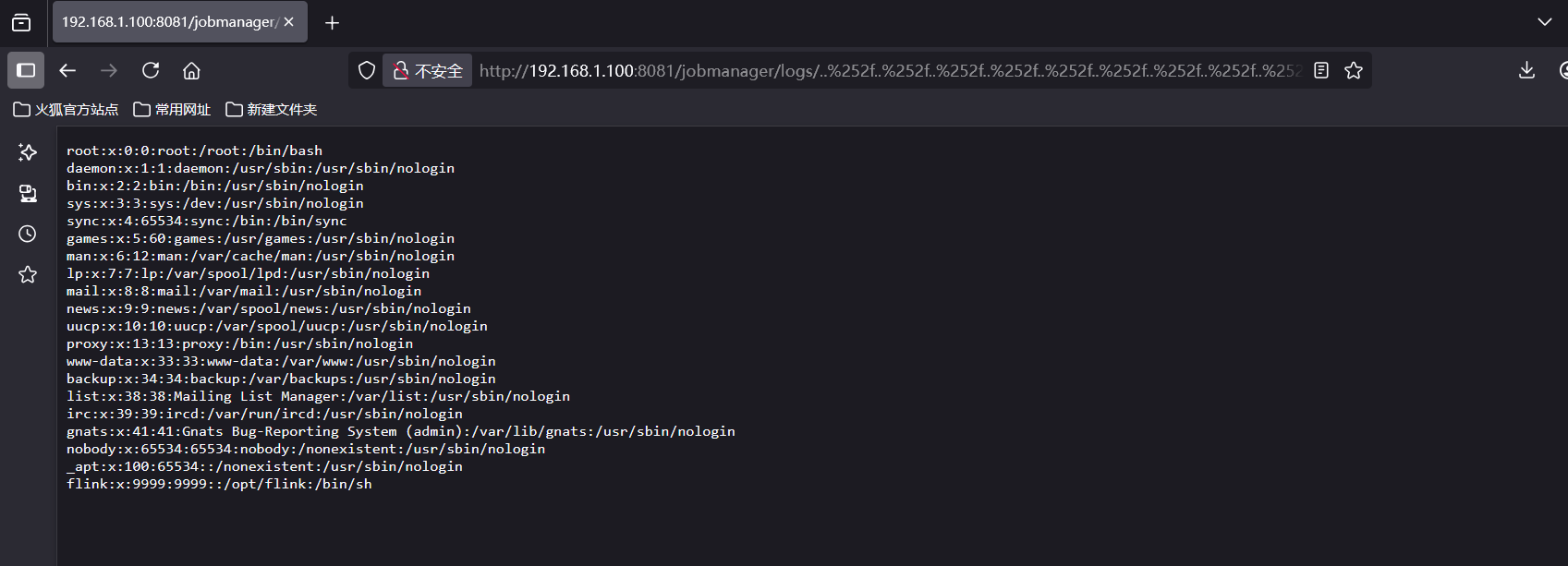
CVE-2020-17519源码分析与漏洞复现(Flink 任意文件读取)
漏洞概览 漏洞名称:Apache Flink REST API 任意文件读取漏洞CVE编号:CVE-2020-17519CVSS评分:7.5影响版本:Apache Flink 1.11.0、1.11.1、1.11.2修复版本:≥ 1.11.3 或 ≥ 1.12.0漏洞类型:路径遍历&#x…...
SQL慢可能是触发了ring buffer
简介 最近在进行 postgresql 性能排查的时候,发现 PG 在某一个时间并行执行的 SQL 变得特别慢。最后通过监控监观察到并行发起得时间 buffers_alloc 就急速上升,且低水位伴随在整个慢 SQL,一直是 buferIO 的等待事件,此时也没有其他会话的争抢。SQL 虽然不是高效 SQL ,但…...
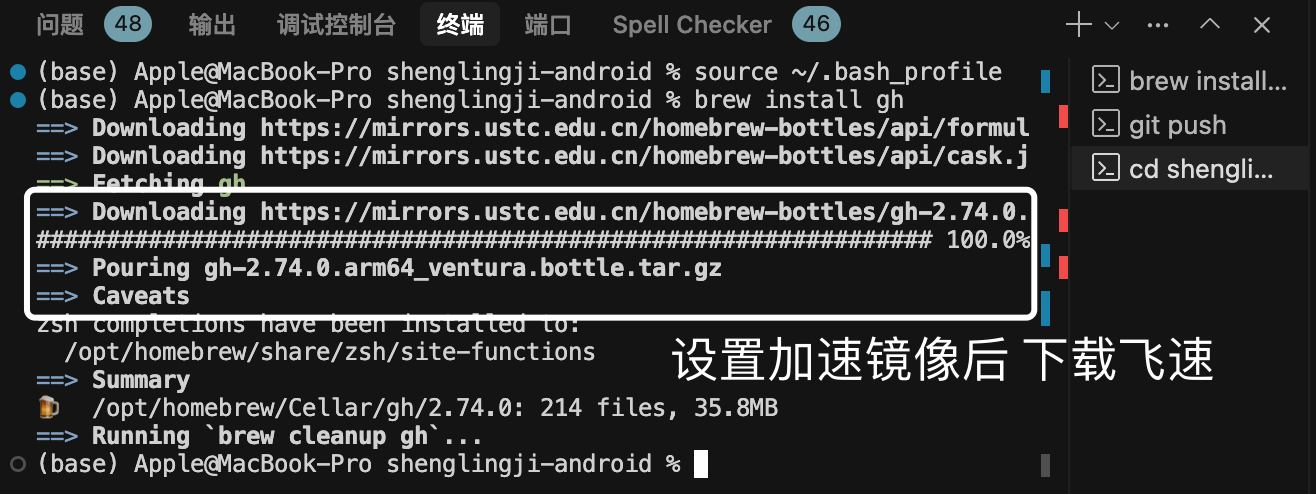
MacOS下Homebrew国内镜像加速指南(2025最新国内镜像加速)
macos brew国内镜像加速方法 brew install 加速formula.jws.json下载慢加速 🍺 最新版brew安装慢到怀疑人生?别怕,教你轻松起飞! 最近Homebrew更新至最新版,每次执行 brew 命令时都会自动从官方地址 https://formulae.…...
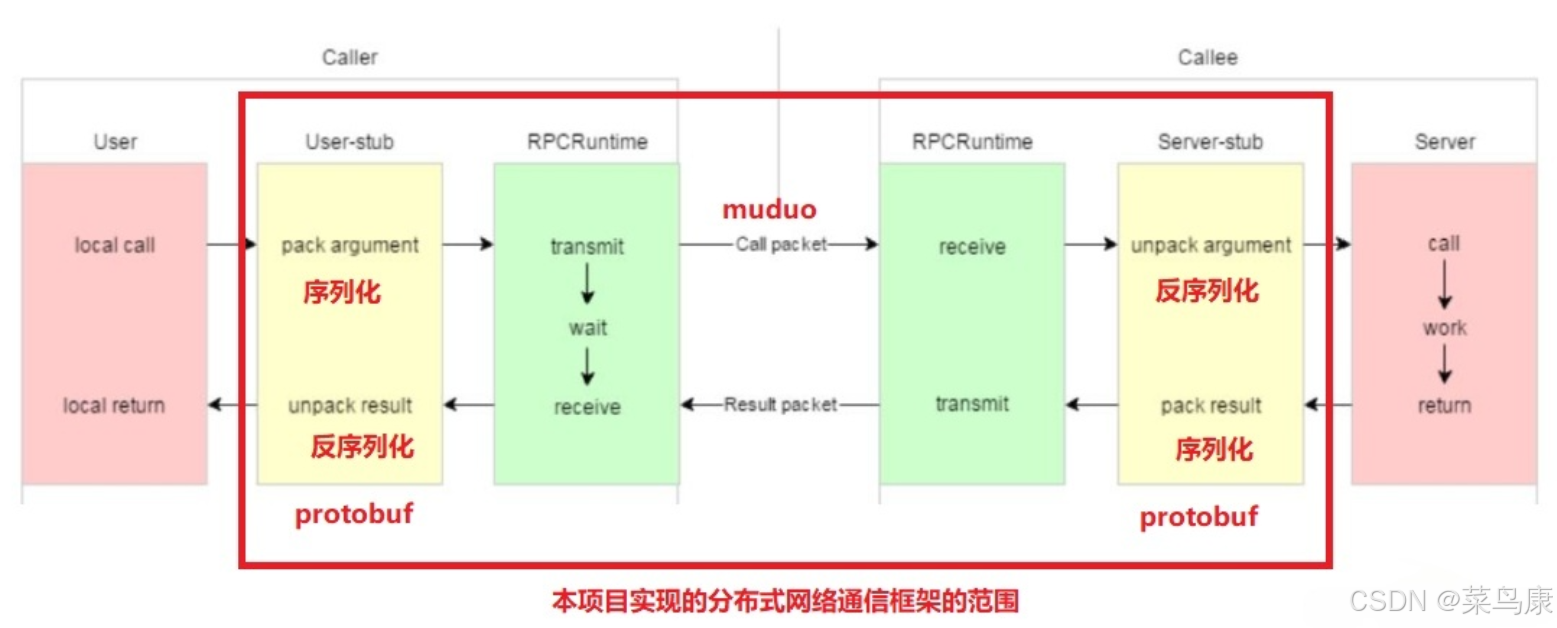
C++实现分布式网络通信框架RPC(2)——rpc发布端
有了上篇文章的项目的基本知识的了解,现在我们就开始构建项目。 目录 一、构建工程目录 二、本地服务发布成RPC服务 2.1理解RPC发布 2.2实现 三、Mprpc框架的基础类设计 3.1框架的初始化类 MprpcApplication 代码实现 3.2读取配置文件类 MprpcConfig 代码实现…...
如何配置一个sql server使得其它用户可以通过excel odbc获取数据
要让其他用户通过 Excel 使用 ODBC 连接到 SQL Server 获取数据,你需要完成以下配置步骤: ✅ 一、在 SQL Server 端配置(服务器设置) 1. 启用 TCP/IP 协议 打开 “SQL Server 配置管理器”。导航到:SQL Server 网络配…...
微服务通信安全:深入解析mTLS的原理与实践
🔥「炎码工坊」技术弹药已装填! 点击关注 → 解锁工业级干货【工具实测|项目避坑|源码燃烧指南】 一、引言:微服务时代的通信安全挑战 随着云原生和微服务架构的普及,服务间的通信安全成为系统设计的核心议题。传统的单体架构中&…...