Activation Functions
Chapter4:Activation Functions
声明:本篇博客笔记来源于《Neural Networks from scratch in Python》,作者的youtube
其实关于神经网络的入门博主已经写过几篇了,这里就不再赘述,附上链接。
1.一文窥见神经网络
2.神经网络入门(上)
3.神经网络入门(下)
前三章内容:
1.Coding Our First Neurons
2.Adding Hidden Layers
4.1 Activation functions
激活函数的作用:将输入值映射到输出,如果激活函数是非线性的,那么将输入进行非线性映射
如果激活函数是线性的,例如f(x)=x,即输入什么就输出什么,那么由这种神经元组成的网络就会输入什么就输出什么
The activation function is applied to the output of a neuron (or layer of neurons), which modifies outputs.
We use activation functions because if the activation function itself is nonlinear, it allows for neural networks with usually two or more hidden layers to map nonlinear functions.
激活函数用在两个方面:1.隐藏层中的神经元、2.输出层的神经元
In general, your neural network will have two types of activation functions. The first will be the activation function used in hidden layers, and the second will be used in the output layer.
The Step Activation Function
如果输入值大于0的数,那么将输出为1,如果输入值小于等于0,那么将输出0
This activation function has been used historically in hidden layers, but nowadays, it is rarely a choice.
The Linear Activation Function
This activation function is usually applied to the last layer’s output in the case of a regression model — a model that outputs a scalar value instead of a classification.
The Sigmoid Activation Function
当输入值小于0时,输出值范围为0~0.5,当输入值大于0时,输出值范围0.5~1
The Rectified Linear Activation Function
The Sigmoid function, historically used in hidden layers, was eventually replaced by the Rectified Linear Units activation function (or ReLU).
4.2 Linear Activation in the Hidden Layers
偏置和权重对激活函数的作用
the bias to offset the function horizontally, and the weight to influence the slope of the activation.
we’re also able to control whether the function is one for determining where the neuron activates or deactivates
从下面情况看,这个神经元的权重和偏置(权重和偏置这种组合模拟了y=x这个函数的作用)
4.3 ReLU Activation in a Pair of Neurons
下面这个神经元的权重和偏置组合模拟了ReLU函数
如果数量大于一个神经元,则每个偏置和权重对激活函数的联合作用
第二个神经元的偏置相当于对ReLU函数进行了上下平移
将第二个神经元的权重取负数,相当于把激活函数进行了垂直方向的翻转
4.4 ReLU Activation in the Hidden Layers
使用2个隐藏层,每层8个神经元,激活函数使用ReLU、这些元素组成的神经网络来模拟sine函数
该模型输入一个值,输出一个类似经过sine函数的函数值。隐藏层使用激活函数ReLU
我们尝试手动调节权重和偏置来使得该模型最终达到模拟sine函数的能力
Fig 4.20–Fig4.25
Fig 4.26–Fig 4.31
Fig 4.32–Fig37
4.5 ReLU Activation Function Code
inputs = [0, 2, -1, 3.3, -2.7, 1.1, 2.2, -100]
outputs = []
# ReLU激活函数 当x>0时输出x,当x<=0时输出0
for i in inputs:if i > 0:outputs.append(i)else:outputs.append(0)print(outputs)
inputs = [0, 2, -1, 3.3, -2.7, 1.1, 2.2, -100]
outputs = []
for i in inputs:outputs.append(max(0, i))
print(outputs)
import numpy as np
inputs = [0, 2, -1, 3.3, -2.7, 1.1, 2.2, -100]
outputs = []
outputs = np.maximum(0, inputs)
print(outputs)
# import numpy as np
# inputs = [0, 2, -1, 3.3, -2.7, 1.1, 2.2, -100]
# outputs = []
# ReLU激活函数 当x>0时输出x,当x<=0时输出0
# for i in inputs:
# if i > 0:
# outputs.append(i)
# else:
# outputs.append(0)
#
# print(outputs)# for i in inputs:
# outputs.append(max(0, i))
# print(outputs)# outputs = np.maximum(0, inputs)
# print(outputs)import numpy as np
import nnfs
from nnfs.datasets import spiral_data
# The spiral_data function allows us to create a dataset with as many classes as we want. The
# function has parameters to choose the number of classes and the number of points/observations
# per class in the resulting non-linear dataset.
'''
nnfs.init() does three things:
(1)it sets the random seed to 0 (by the default),
(2)creates a float32 dtype default, and
(3)overrides the original dot product from NumPy.
All of these are meant to ensure repeatable results for following along.
'''
nnfs.init()class Layer_Dense:def __init__(self, n_inputs, n_neurons):self.weights = 0.01 * np.random.randn(n_inputs, n_neurons)self.biases = np.zeros((1, n_neurons))def forward(self, inputs):self.outputs = np.dot(inputs, self.weights) + self.biasesclass ReLU:def forward(self, inputs):self.output = np.maximum(0, inputs)'''X是每个数据(x,y)坐标值X (0,1)0 0.00299, 0.009641 0.01288, 0.01556y表示类别y 00 01 02 1
'''X, y = spiral_data(samples=100, classes=3)
network = Layer_Dense(2, 3)
network.forward(X) # 将输入前向传播
activation = ReLU() # 实例化激活函数
activation.forward(network.outputs) # 将神经元的值输入到将期货函数
print(activation.output[:5]) # 显示0-4行结果
4.6 The Softmax Activation Function
如果想要让模型具备分类能力,可以使用softmax激活函数,此函数将输入值映射到0~1范围内,输出值代表归属于某个类的概率,即输入值属于某个类的概率是多少,在输出层中的神经元中有一个最大值,即输入值大概率归属于该神经元对应的类别
S i , j = e z i , j ∑ l = 1 L e z i , j S_{i,j}=\frac{e^{z_{i,j}}}{\sum_{l=1}^Le^{z_{i,j}}} Si,j=∑l=1Lezi,jezi,j
先对输入到某层神经元的所有值进行指数运算,将这些指数运算后的值加和,将每个神经元指数运算后的值除以这个所有值指数运算值加和,完成对所有值的归一化
import numpy as np
layer_outputs = [4.8, 1.21, 2.385]
exp_values = np.exp(layer_outputs)
print(exp_values) # 对每个神经元的值进行指数运算
norm_exp = exp_values / np.sum(exp_values) # 对每个指数运算后的值进行归一化
print(norm_exp)
print('sum of norm_values:', np.sum(norm_exp)) # 归一化后的所有值总和为1,概率和为1
========
[121.51041752 3.35348465 10.85906266]
[0.89528266 0.02470831 0.08000903]
sum of norm_values: 0.9999999999999999
To train in batches, we need to convert this functionality to accept layer outputs in batches.
import numpy as np
layer_outputs = np.array([[4.8, 1.21, 2.385],[8.9, -1.81, 0.2],[1.41, 1.051, 0.025]])
print('sum without axis')
print(np.sum(layer_outputs))
print('this will be identical to the above since default is None')
print(np.sum(layer_outputs, axis=None)) # 所有值求和
# in a 2D array/matrix, axis 0 refers to the rows, and axis 1 refers to the columns
print('Another way to think of it w/ a matrix == axis 0: columns:')
print(np.sum(layer_outputs, axis=0)) # 按列求和
print('sum of raws')
print(np.sum(layer_outputs, axis=1)) # 按行求和(结果默认转为一行)
print('Sum axis 1, but keep the same dimensions as input:')
print(np.sum(layer_outputs, axis=1, keepdims=True)) # 按行求和后,保持与输入值维度一致(可以仍然保持一列)
===========
sum without axis
18.171
this will be identical to the above since default is None
18.171
Another way to think of it w/ a matrix == axis 0: columns:
[15.11 0.451 2.61 ]
sum of raws
[8.395 7.29 2.486]
Sum axis 1, but keep the same dimensions as input:
[[8.395][7.29 ][2.486]]
我们知道指数函数在其输入值接近负无穷时趋向于0,并且当输入为0时,输出为1,我们可以利用这个性质来防止指数爆炸,也就是说如果给指数函数输入一个较大值,输出可能超过浮点数能够表示的范围,造成overflow
假设我们从输入值列表中减去最大值,则输出值会落在 [负值,0] 的范围内,归一化后将范围映射到[0,1]
class Activation_Softmax:def forward(self, inputs):exp_vals = np.exp(inputs - np.max(inputs, axis=1, keepdims=True))probability = exp_vals / np.sum(exp_vals, axis=1, keepdims=True)self.output = probabilitysoftmax = Activation_Softmax()
softmax.forward([[1, 2, 3]]) # inputs [[1, 2, 3]] max(inputs)=3 exp(1-3, 2-3, 3-3)=[[0.135, 0.3678, 1]]
print(softmax.output)
softmax.forward([[-2, -1, 0]]) # inputs [[-2, -1, 0]] max(inputs)=-1 exp(-2-0, -1-0, 0-0)=[[0.135, 0.3678, 1]]
print(softmax.output)
softmax.forward([[0.5, 1, 1.5]]) # 将inputs[[1, 2, 3]]除以2变为[[0.5, 1, 1.5]],输出softmax的值并不是[[0.135, 0.3678, 1]]除以2得到的结果
print(softmax.output)
=========
[[0.09003057 0.24472847 0.66524096]]
[[0.09003057 0.24472847 0.66524096]]
[[0.18632372 0.30719589 0.50648039]]
我们创造一个神经网络,输入层2个神经元,第二层(隐藏层)3个神经元(该层神经元使用ReLU激活函数),第三层(输出层)3个神经元(使用softmax激活函数,该函数可以接收未归一化的数据,并输出概率值)
给神经网络输入3个类别的样本,每个类别100个样本,输出层输出3个概率值,神经元由上到下分别对于三个类别,三个输出值对于归属于类别的概率值
# add a dense layer as output layer
import numpy as np
import nnfs
from nnfs.datasets import spiral_data
nnfs.init()# nn结构
class Layer_Dense:def __init__(self, n_inputs, n_neurons):self.weights = 0.01 * np.random.randn(n_inputs, n_neurons) # 使用正态分布随机初始化权重self.biases = np.zeros((1, n_neurons)) # 将偏置初始化为0def forward(self, inputs):self.ouput = np.dot(inputs, self.weights) + self.biases# ReLU
class Activation_ReLU:def forward(self, inputs):self.output = np.maximum(0, inputs)# Softmax
class Activation_Softmax:def forward(self, inputs):exp_vals = np.exp(inputs - np.max(inputs, axis=1, keepdims=True))probability = exp_vals / np.sum(exp_vals, axis=1, keepdims=True)self.output = probabilityX, y = spiral_data(samples=100, classes=3)
dense1 = Layer_Dense(2, 3) # 输入层2,第一层3个neurons
dense1.forward(X) # 将样本输入到输入层,并传递到第一层
activation1 = Activation_ReLU() # 实例化ReLU激活函数
activation1.forward(dense1.ouput) # 对第一层的所有值输入到第一层的激活函数中
dense2 = Layer_Dense(3, 3) # 第二层3个神经元,第三层3个神经元
dense2.forward(activation1.output) # 将第一层激活后的值输入到第三层
activation2 = Activation_Softmax() # 实例化softmax激活函数
activation2.forward(dense2.ouput) # 将到达第三层的值输入到激活函数
print(activation2.output[:5]) # 只输出5个样本(下标0-4)的结果
========
[[0.33333334 0.33333334 0.33333334][0.33333316 0.3333332 0.33333367][0.3333329 0.33333293 0.3333342 ][0.3333326 0.33333263 0.33333474][0.3333323 0.33333242 0.33333525]]
我们构建的神经网络其权重是随机初始化的,该网络并不具备实际应用的能力,假设将该神经网络用于分类,它的预测误差将会很大,我们要想办法调节权重和偏置使得该神经网络的分类误差减小,由此引入loss(误差,损失),我们想让模型的误差尽量小以逼近0
相关文章:
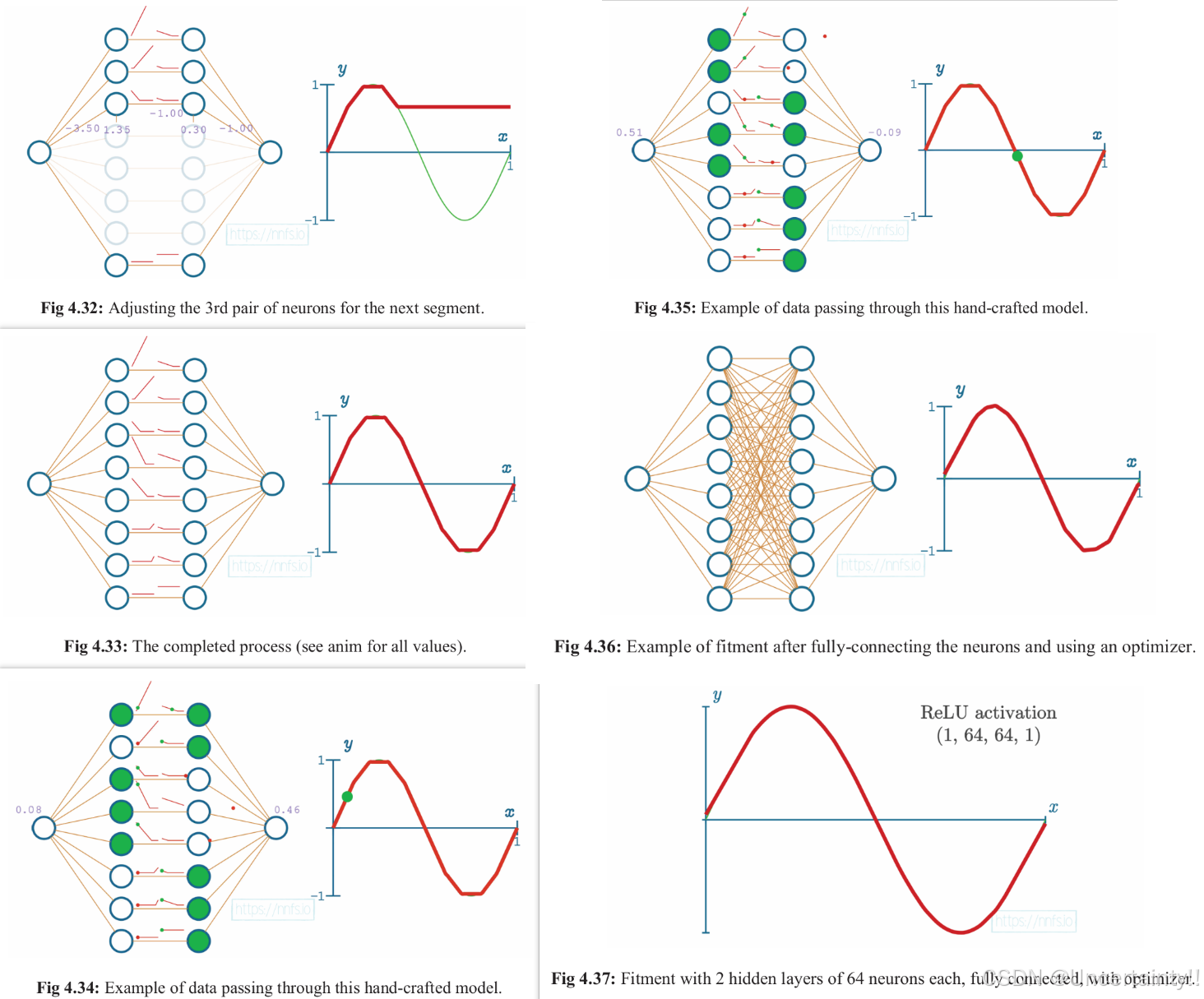
Activation Functions
Chapter4:Activation Functions 声明:本篇博客笔记来源于《Neural Networks from scratch in Python》,作者的youtube 其实关于神经网络的入门博主已经写过几篇了,这里就不再赘述,附上链接。 1.一文窥见神经网络 2.神经…...
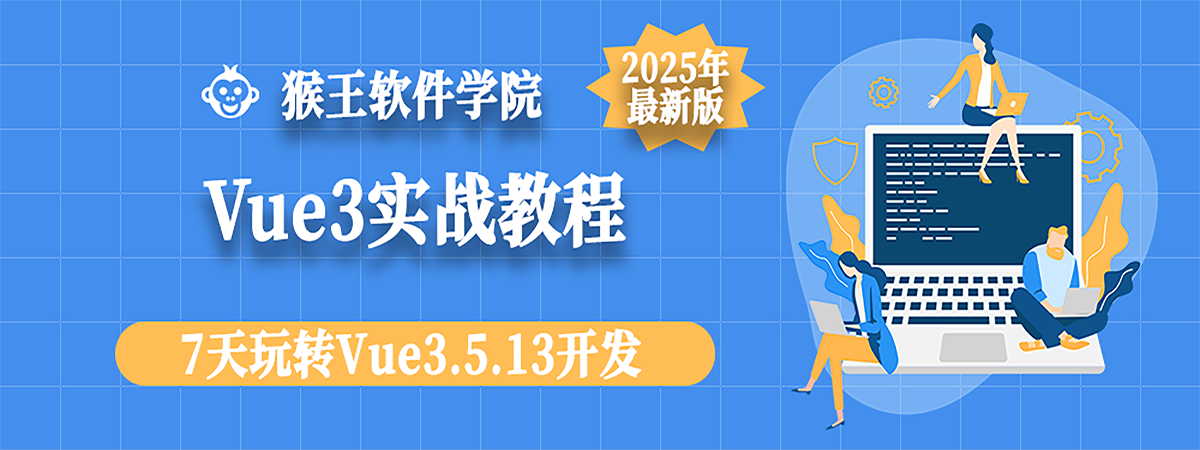
《Vue3实战教程》37:Vue3生产部署
如果您有疑问,请观看视频教程《Vue3实战教程》 生产部署 开发环境 vs. 生产环境 在开发过程中,Vue 提供了许多功能来提升开发体验: 对常见错误和隐患的警告对组件 props / 自定义事件的校验响应性调试钩子开发工具集成 然而ÿ…...
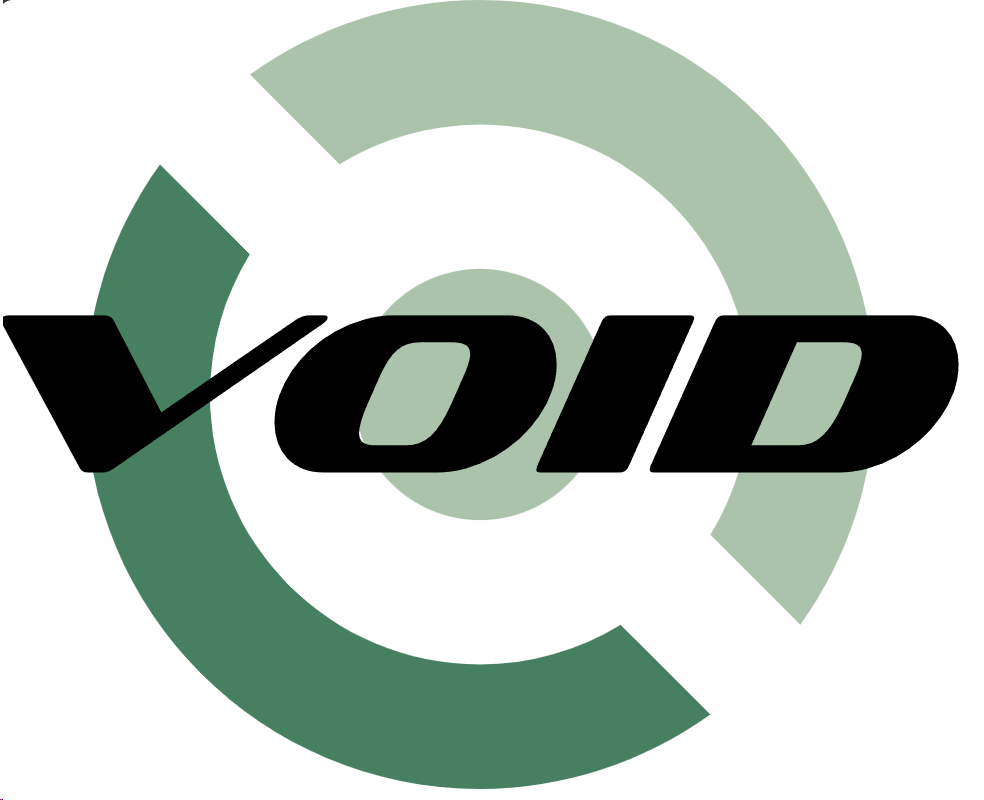
Linux:各发行版及其包管理工具
相关阅读 Linuxhttps://blog.csdn.net/weixin_45791458/category_12234591.html?spm1001.2014.3001.5482 Debian 包管理工具:dpkg(低级包管理器)、apt(高级包管理器,建立在dpkg基础上)包格式:…...
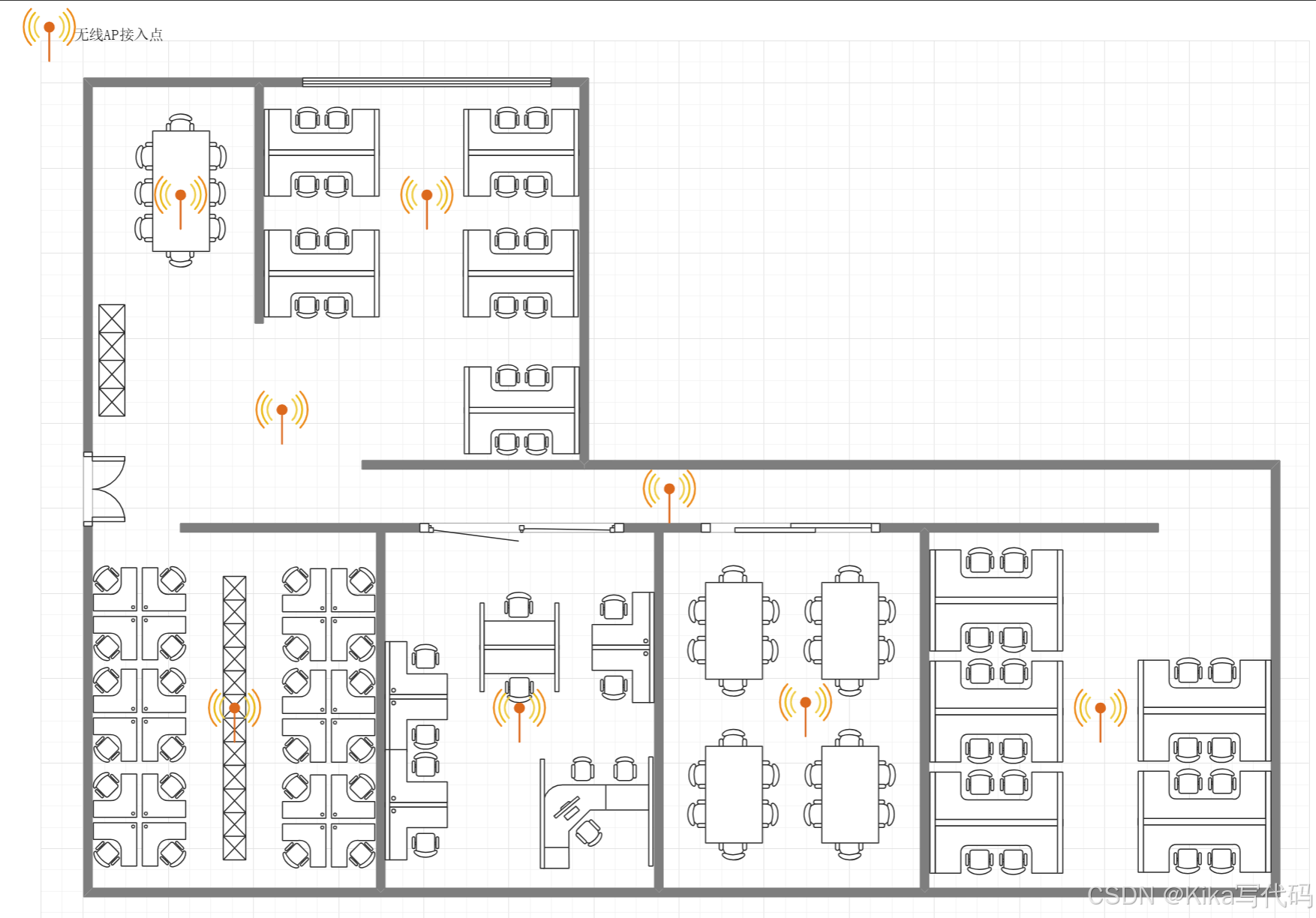
【计算机网络】课程 作业一 搭建连续覆盖的办公网络
作业一 搭建连续覆盖的办公网络 题目:论述题(共1题,100分) 充分利用所学习的数据链路层局域网知识,加上物理层的基础知识,请给一个办公场所(三层,每层约100平方)…...
C++ 设计模式:单例模式(Singleton Pattern)
链接:C 设计模式 链接:C 设计模式 - 享元模式 单例模式(Singleton Pattern)是创建型设计模式,它确保一个类只有一个实例,并提供一个全局访问点来访问这个实例。单例模式在需要全局共享资源或控制实例数量的…...
OpenCV调整图像亮度和对比度
【欢迎关注编码小哥,学习更多实用的编程方法和技巧】 1、基本方法---线性变换 // 亮度和对比度调整 cv::Mat adjustBrightnessContrast(const cv::Mat& src, double alpha, int beta) {cv::Mat dst;src.convertTo(dst, -1, alpha, beta);return dst; }// 使用…...
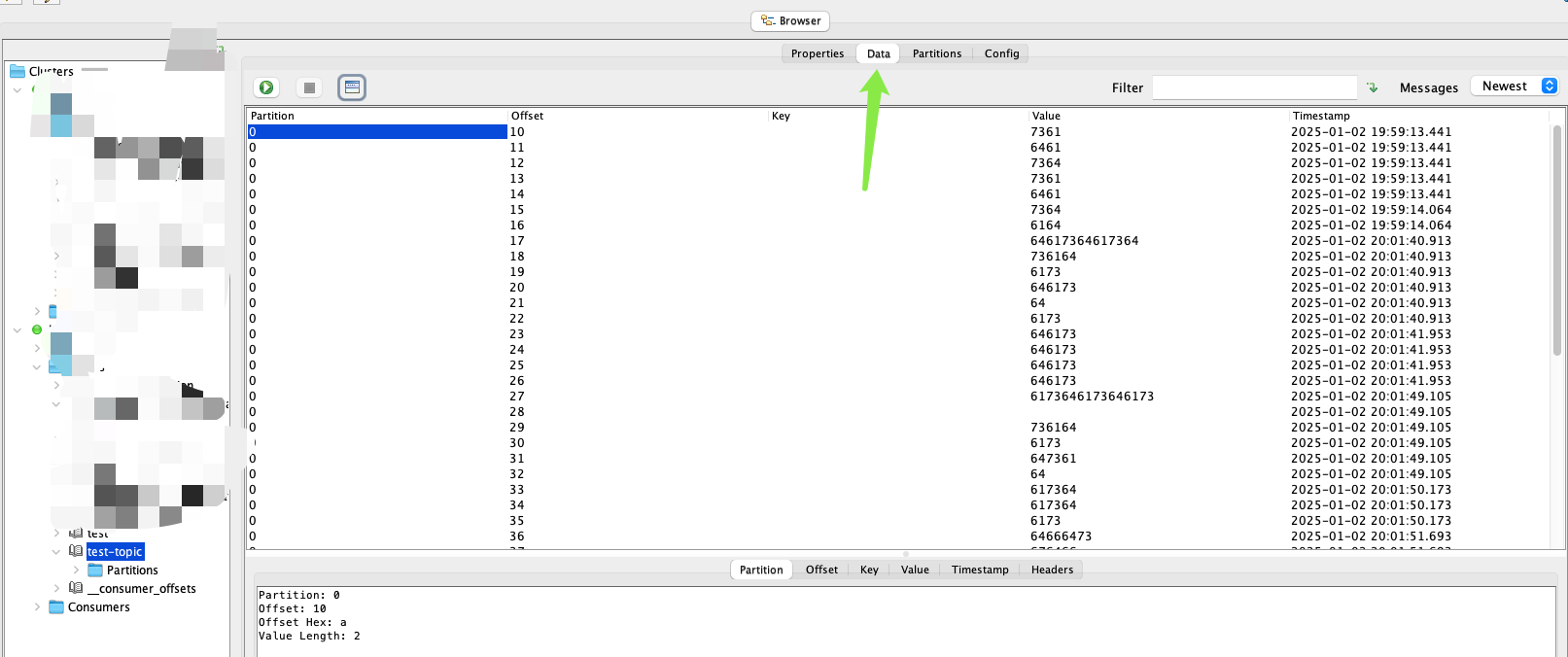
Kafka Offset explorer使用
Kafka集群配置好以后以后运维这边先用工具测试一下,便于rd展开后续的工作,本地调试时一般使用Offset explorer工具进行连接 使用SASL(Simple Authentication and Security Layer)验证方式 使用SCRAM-SHA-256(Salted Challenge Response Authentication…...
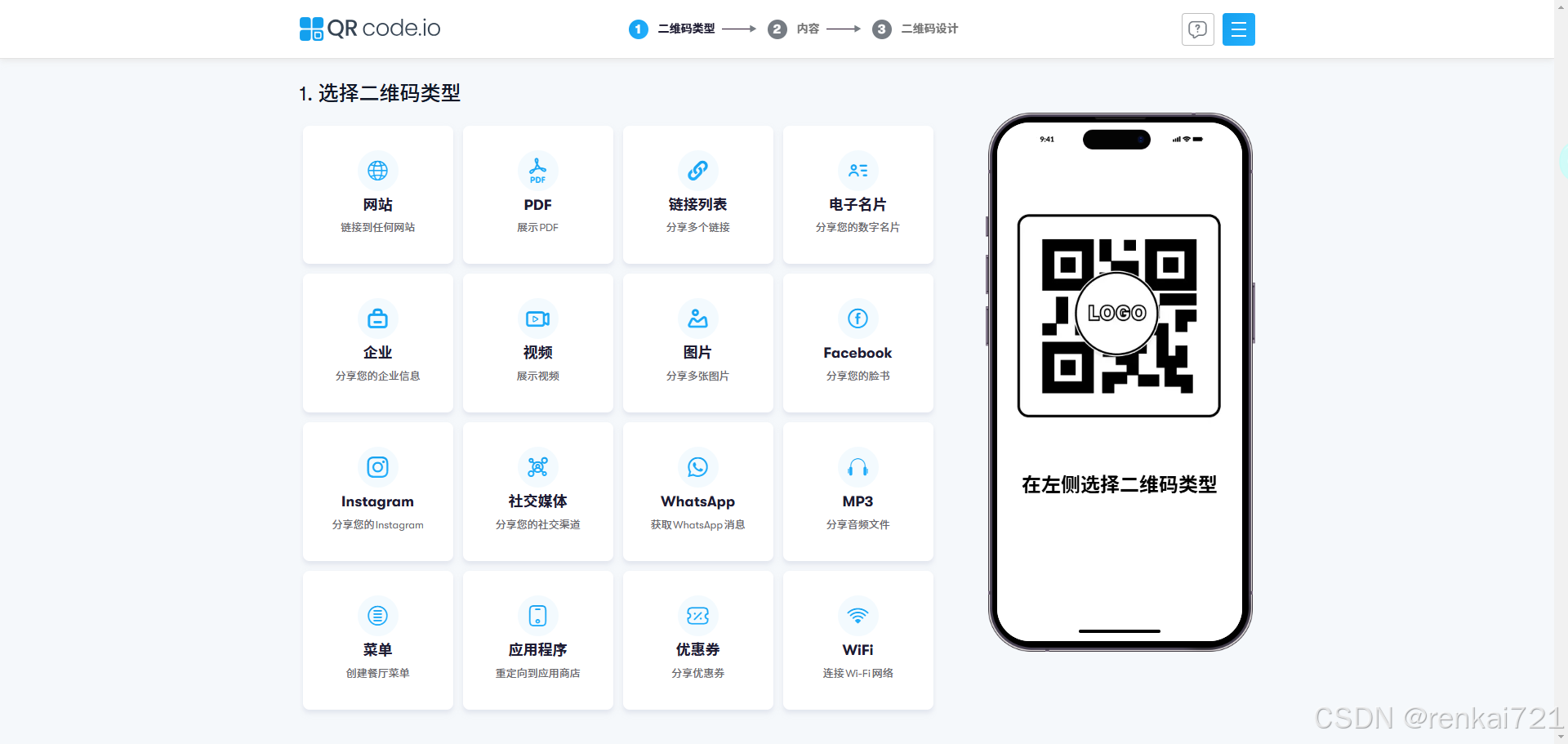
二维码文件在线管理系统-收费版
需求背景 如果大家想要在网上管理自己的文件,而且需要生成二维码,下面推荐【草料二维码】,这个系统很好。特别适合那些制造业,实体业的使用手册,你可以生成一个二维码,贴在设备上,然后这个二维码…...
UE4.27 Android环境下获取手机电量
获取电量方法 使用的方法时FAndroidMisc::GetBatteryLevel(); 出现的问题 但是在电脑上编译时发现,会发现编译无法通过。 因为安卓环境下编译时,包含 #include "Android/AndroidPlatformMisc.h" 头文件是可以正常链接的,但在电…...
vue-i18n报错
1. 开发环境报错Uncaught (in promise) TypeError: ‘set’ on proxy: trap returned falsish for property ‘$t’ legacy需要设置为false const i18n createI18n({legacy: false,// 默认语言locale: lang,// 设置语言环境messages, })2. 打包配置tsc --noEmit时报错&#…...
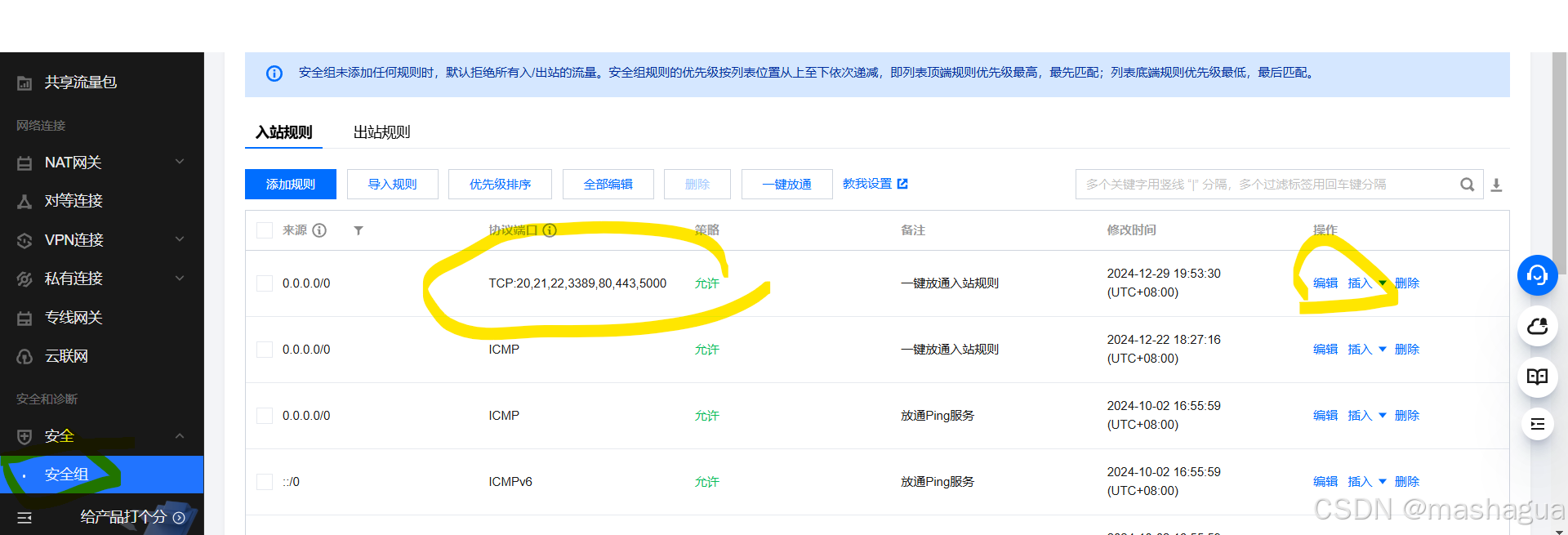
Docker新手:在tencent云上实现Python服务打包到容器
1 使用docker的原因 一致性和可移植性:Docker 容器可以在任何支持 Docker 的环境中运行,无论是开发者的笔记本电脑、测试服务器还是生产环境。这确保了应用在不同环境中的行为一致,减少了“在我的机器上可以运行”的问题。 隔离性ÿ…...
React基础知识学习
学习React前端框架是一个系统而深入的过程,以下是一份详细的学习指南: 一、React基础知识 React简介 React是一个用于构建用户界面的JavaScript库,由Facebook开发和维护。它强调组件化和声明式编程,使得构建复杂的用户界面变得更…...
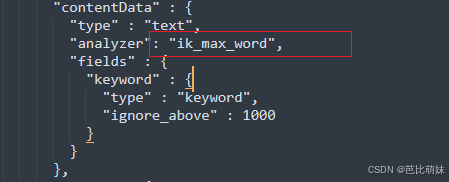
ES IK分词器插件
前言 ES中默认了许多分词器,但是对中文的支持并不友好,IK分词器是一个专门为中文文本设计的分词工具,它不是ES的内置组件,而是一个需要单独安装和配置的插件。 Ik分词器的下载安装(Winows 版本) 下载地址:…...
二十三种设计模式-抽象工厂模式
抽象工厂模式(Abstract Factory Pattern)是一种创建型设计模式,它提供了一种方式,用于创建一系列相关或相互依赖的对象,而不需要指定它们具体的类。这种模式主要用于系统需要独立于其产品的创建逻辑时,并且…...
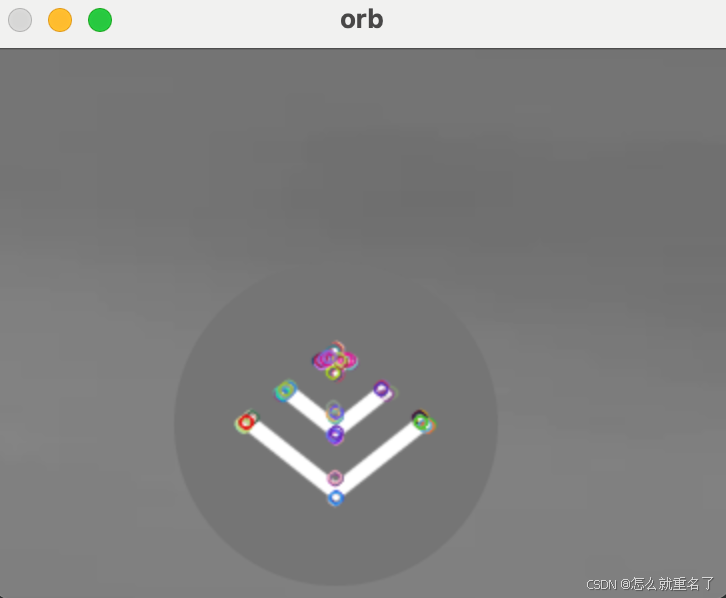
python opencv的orb特征检测(Oriented FAST and Rotated BRIEF)
官方文档:https://docs.opencv.org/4.10.0/d1/d89/tutorial_py_orb.html SIFT/SURF/ORB对比 https://www.bilibili.com/video/BV1Yw411S7hH?spm_id_from333.788.player.switch&vd_source26bb43d70f463acac2b0cce092be2eaa&p80 ORB代码 import numpy a…...
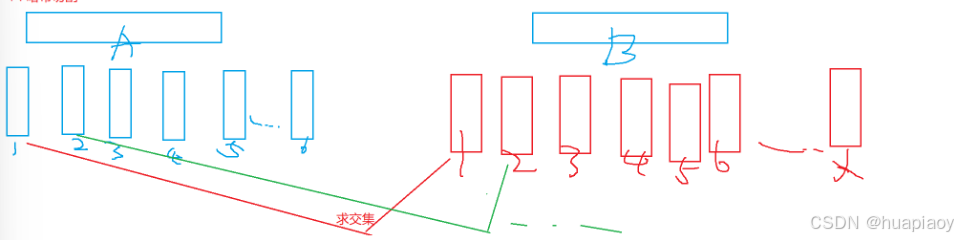
高阶数据结构----布隆过滤器和位图
(一)位图 位图是用来存放某种状态的,因为一个bit上只能存0和1所以一般只有两种状态的情况下适合用位图,所以非常适合判断数据在或者不在,而且位图十分节省空间,很适合于海量数据,且容易存储&…...
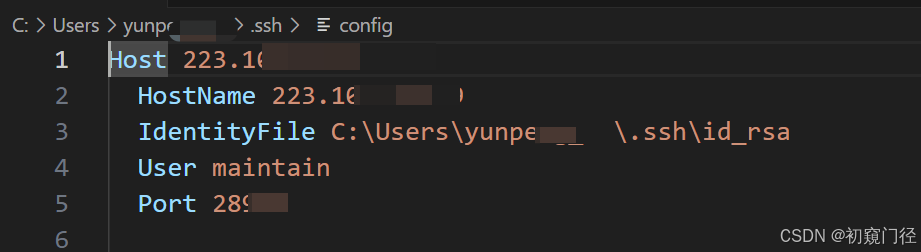
VScode使用密钥进行ssh连接服务器方法
如何正常连接ssh的方式可以看我原来那篇文章:Windows上使用VSCode连接远程服务器ssh 1.连接 点击ssh加号,然后关键点在第2步的书写上 2.命令 2的位置写命令: ssh -i "C:\Users\userName\.ssh\id_rsa" usernameIP -p 端口号 端…...
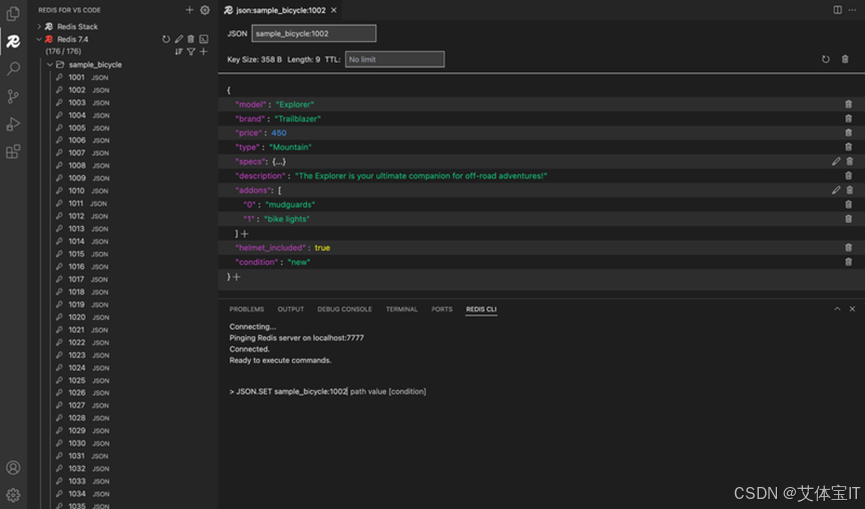
艾体宝产品丨加速开发:Redis 首款 VS Code 扩展上线!
Redis 宣布推出其首款专为 VS Code 设计的 Redis 扩展。这一扩展将 Redis 功能直接整合进您的集成开发环境(IDE),旨在简化您的工作流程,提升工作效率。 我们一直致力于构建强大的开发者生态系统,并在您工作的每一步提…...
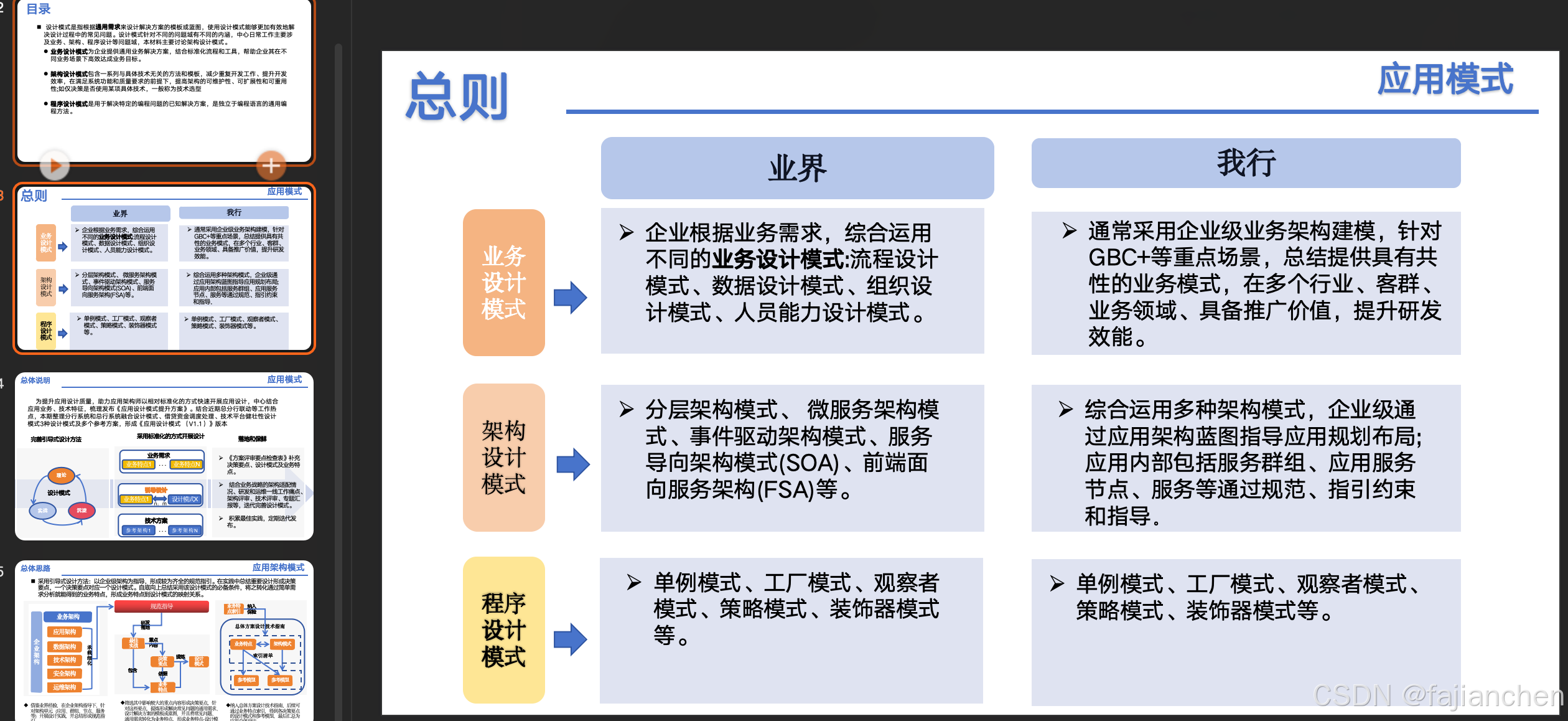
应用架构模式
设计模式 设计模式是指根据通用需求来设计解决方案的模板或蓝图,使用设计模式能够更加有效地解决设计过程中的常见问题。设计模式针对不同的问题域有不同的内涵,主要涉及业务、架构、程序设计等问题域,本文主要讨论架构设计模式。 业务设计模…...
注入少量可学习的向量参数: 注入适配器IA3
注入少量可学习的向量参数: 注入适配器IA3 简介:IA3通过学习向量来对激活层加权进行缩放,从而获得更强的性能,同时仅引入相对少量的新参数。它的诞生背景是为了改进LoRA,与LoRA不同的是,IA3直接处理学习向量,而不是学习低秩权重矩阵,这使得可训练参数的数量更少,并且原…...
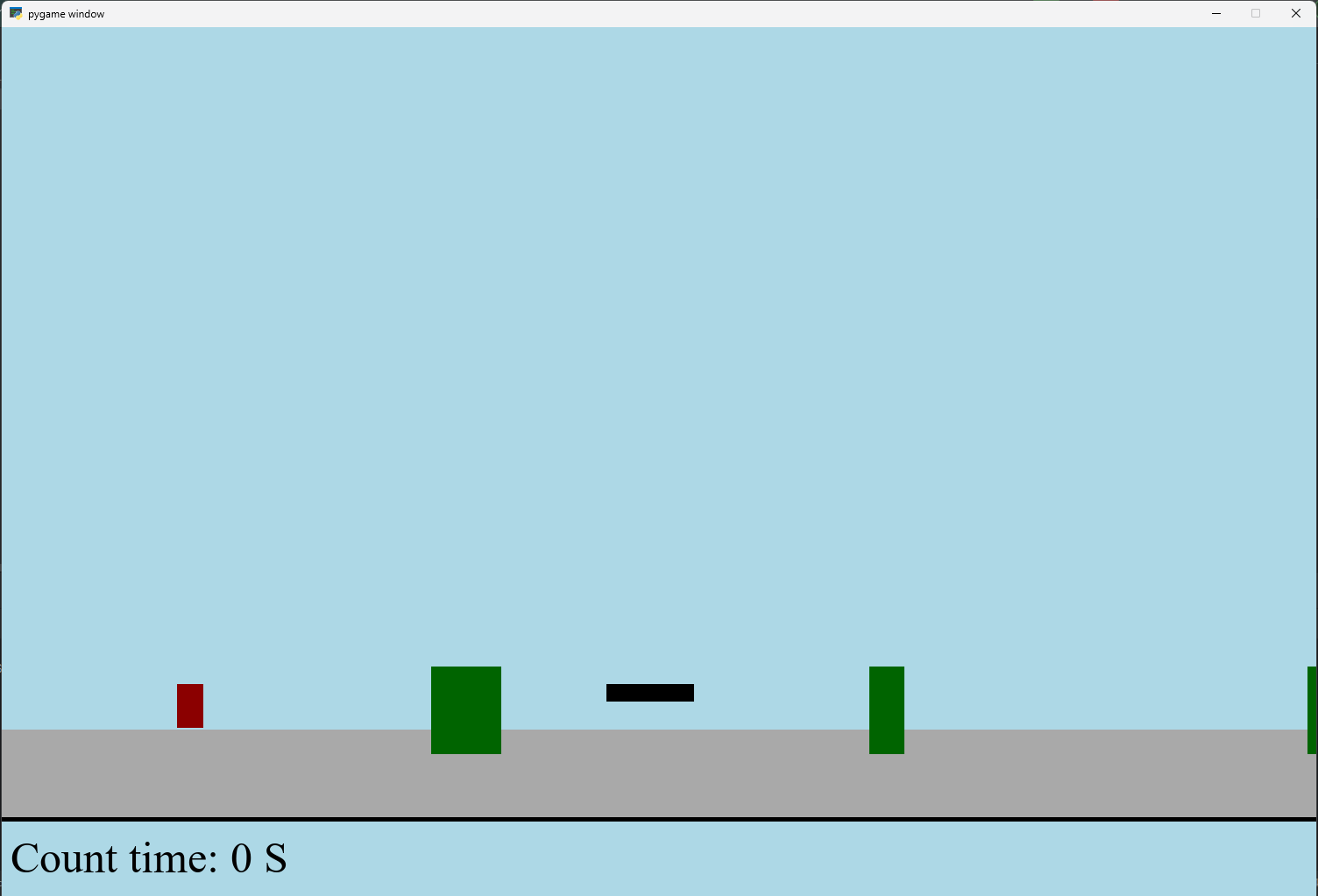
【Python】 -- 趣味代码 - 小恐龙游戏
文章目录 文章目录 00 小恐龙游戏程序设计框架代码结构和功能游戏流程总结01 小恐龙游戏程序设计02 百度网盘地址00 小恐龙游戏程序设计框架 这段代码是一个基于 Pygame 的简易跑酷游戏的完整实现,玩家控制一个角色(龙)躲避障碍物(仙人掌和乌鸦)。以下是代码的详细介绍:…...
脑机新手指南(八):OpenBCI_GUI:从环境搭建到数据可视化(下)
一、数据处理与分析实战 (一)实时滤波与参数调整 基础滤波操作 60Hz 工频滤波:勾选界面右侧 “60Hz” 复选框,可有效抑制电网干扰(适用于北美地区,欧洲用户可调整为 50Hz)。 平滑处理&…...
C++.OpenGL (10/64)基础光照(Basic Lighting)
基础光照(Basic Lighting) 冯氏光照模型(Phong Lighting Model) #mermaid-svg-GLdskXwWINxNGHso {font-family:"trebuchet ms",verdana,arial,sans-serif;font-size:16px;fill:#333;}#mermaid-svg-GLdskXwWINxNGHso .error-icon{fill:#552222;}#mermaid-svg-GLd…...
浅谈不同二分算法的查找情况
二分算法原理比较简单,但是实际的算法模板却有很多,这一切都源于二分查找问题中的复杂情况和二分算法的边界处理,以下是博主对一些二分算法查找的情况分析。 需要说明的是,以下二分算法都是基于有序序列为升序有序的情况…...
DeepSeek 技术赋能无人农场协同作业:用 AI 重构农田管理 “神经网”
目录 一、引言二、DeepSeek 技术大揭秘2.1 核心架构解析2.2 关键技术剖析 三、智能农业无人农场协同作业现状3.1 发展现状概述3.2 协同作业模式介绍 四、DeepSeek 的 “农场奇妙游”4.1 数据处理与分析4.2 作物生长监测与预测4.3 病虫害防治4.4 农机协同作业调度 五、实际案例大…...
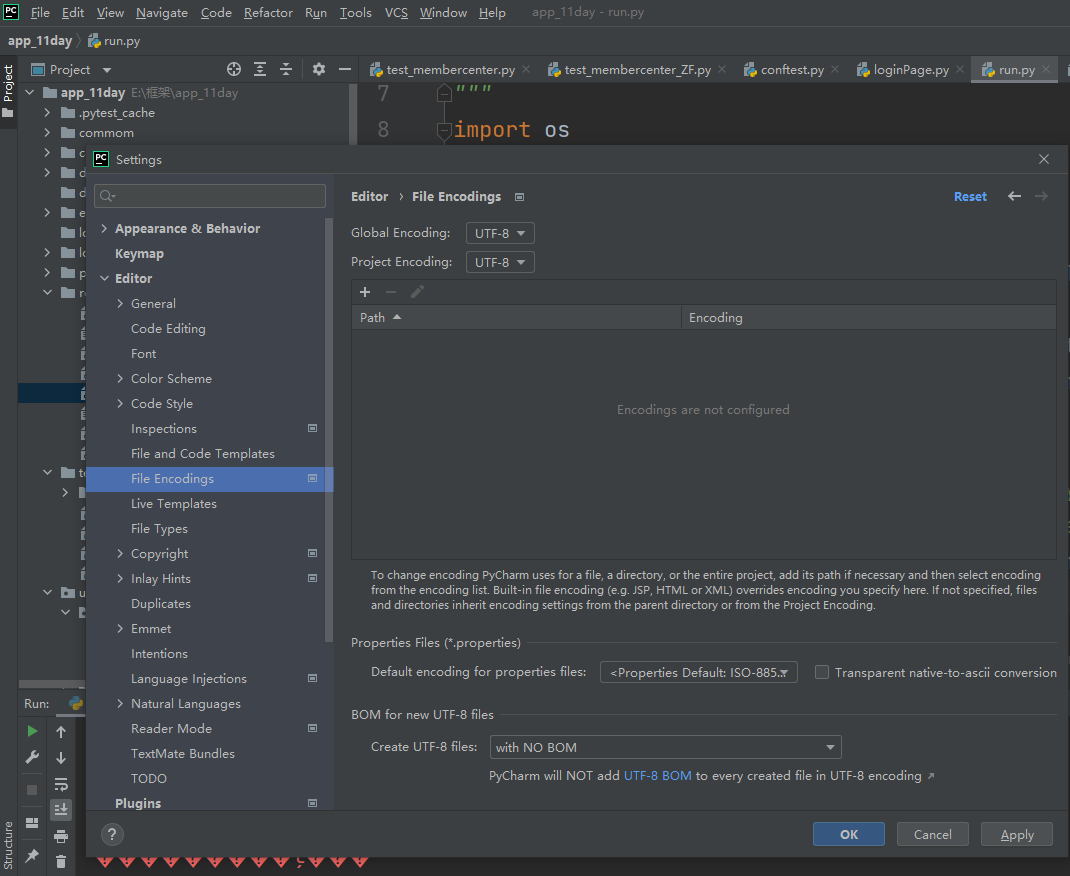
python执行测试用例,allure报乱码且未成功生成报告
allure执行测试用例时显示乱码:‘allure’ �����ڲ����ⲿ���Ҳ���ǿ�&am…...
Java数值运算常见陷阱与规避方法
整数除法中的舍入问题 问题现象 当开发者预期进行浮点除法却误用整数除法时,会出现小数部分被截断的情况。典型错误模式如下: void process(int value) {double half = value / 2; // 整数除法导致截断// 使用half变量 }此时...
JS手写代码篇----使用Promise封装AJAX请求
15、使用Promise封装AJAX请求 promise就有reject和resolve了,就不必写成功和失败的回调函数了 const BASEURL ./手写ajax/test.jsonfunction promiseAjax() {return new Promise((resolve, reject) > {const xhr new XMLHttpRequest();xhr.open("get&quo…...
为什么要创建 Vue 实例
核心原因:Vue 需要一个「控制中心」来驱动整个应用 你可以把 Vue 实例想象成你应用的**「大脑」或「引擎」。它负责协调模板、数据、逻辑和行为,将它们变成一个活的、可交互的应用**。没有这个实例,你的代码只是一堆静态的 HTML、JavaScript 变量和函数,无法「活」起来。 …...
高防服务器价格高原因分析
高防服务器的价格较高,主要是由于其特殊的防御机制、硬件配置、运营维护等多方面的综合成本。以下从技术、资源和服务三个维度详细解析高防服务器昂贵的原因: 一、硬件与技术投入 大带宽需求 DDoS攻击通过占用大量带宽资源瘫痪目标服务器,因此…...