ResNeSt: Split-Attention Networks 参考论文
参考文献
[1] Tensorflow Efficientnet. https://github.com/tensorflow/tpu/tree/master/models/official/efficientnet. Accessed: 2020-03-04.
中文翻译:[1] TensorFlow EfficientNet. https://github.com/tensorflow/tpu/tree/master/models/official/efficientnet. 访问日期:2020-03-04.
[2] Sean Bell, C Lawrence Zitnick, Kavita Bala, and Ross Girshick. Inside-outside net: Detecting objects in context with skip pooling and recurrent neural networks. In Proceedings of the IEEE conference on computer vision and pattern recognition, pages 2874–2883, 2016.
中文翻译:[2] 肖恩·贝尔,C·劳伦斯·齐特尼克,卡维塔·巴拉,罗斯·吉什克. 内外网:使用跳过池化和递归神经网络在上下文中检测对象. 在IEEE计算机视觉与模式识别会议论文集,第2874-2883页,2016.
[3] Han Cai, Chuang Gan, Tianzhe Wang, Zhekai Zhang, and Song Han. Once-for-all: Train one network and specialize it for efficient deployment. arXiv preprint arXiv:1908.09791, 2019.
中文翻译:[3] 韩才,创甘,天泽王,泽凯张,宋汉. 一劳永逸:训练一个网络并将其专门化以实现高效部署. arXiv预印本arXiv:1908.09791, 2019.
[4] Zhaowei Cai and Nuno Vasconcelos. Cascade r-cnn: Delving into high quality object detection. In Proceedings of the IEEE conference on computer vision and pattern recognition, pages 6154–6162, 2018.
中文翻译:[4] 赵伟才,努诺·瓦斯康塞洛斯. 级联R-CNN:深入高质量目标检测. 在IEEE计算机视觉与模式识别会议论文集,第6154-6162页,2018.
[5] Zhaowei Cai and Nuno Vasconcelos. Cascade r-cnn: High quality object detection and instance segmentation. IEEE Transactions on Pattern Analysis and Machine Intelligence, 2019.
中文翻译:[5] 赵伟才,努诺·瓦斯康塞洛斯. 级联R-CNN:高质量目标检测和实例分割. IEEE模式分析与机器智能学报,2019.
[6] Kai Chen, Jiangmiao Pang, Jiaqi Wang, Yu Xiong, Xiaoxiao Li, Shuyang Sun, Wansen Feng, Ziwei Liu, Jianping Shi, Wanli Ouyang, et al. Hybrid task cascade for instance segmentation. In Proceedings of the IEEE conference on computer vision and pattern recognition, pages 4974–4983, 2019.
中文翻译:[6] 陈凯,江苗庞,贾琪王,熊宇,李潇潇,孙树阳,冯万森,刘子维,史建平,欧阳万里等. 混合任务级联用于实例分割. 在IEEE计算机视觉与模式识别会议论文集,第4974-4983页,2019.
[7] Kai Chen, Jiaqi Wang, Jiangmiao Pang, Yuhang Cao, Yu Xiong, Xiaoxiao Li, Shuyang Sun, Wansen Feng, Ziwei Liu, Jiarui Xu, Zheng Zhang, Dazhi Cheng, Chenchen Zhu, Tianheng Cheng, Qijie Zhao, Buyu Li, Xin Lu, Rui Zhu, Yue Wu, Jifeng Dai, Jingdong Wang, Jianping Shi, Wanli Ouyang, Chen Change Loy, and Dahua Lin. MMDetection: Open mmlab detection toolbox and benchmark. arXiv preprint arXiv:1906.07155, 2019.
中文翻译:[7] 陈凯,王佳琪,庞江苗,曹宇航,熊宇,李潇潇,孙树阳,冯万森,刘子维,徐佳瑞,张正,程大志,朱晨晨,程天恒,赵奇杰,李不语,卢欣,朱锐,吴悦,戴继峰,王景东,史建平,欧阳万里,陈改变忠诚,林大华. MMDetection:开放的MMLab检测工具箱和基准. arXiv预印本arXiv:1906.07155, 2019.
[8] Liang-Chieh Chen, George Papandreou, Iasonas Kokkinos, Kevin Murphy, and Alan L Yuille. Deeplab: Semantic image segmentation with deep convolutional nets, atrous convolution, and fully connected crfs. arXiv:1606.00915, 2016.
中文翻译:[8] 梁哲陈,乔治·帕帕纳德雷欧,伊阿索纳斯·科金诺斯,凯文·墨菲,艾伦·L·尤利. Deeplab:使用深度卷积网络、扩张卷积和全连接CRFs进行语义图像分割. arXiv:1606.00915, 2016.
[9] Liang-Chieh Chen, George Papandreou, Florian Schroff, and Hartwig Adam. Rethinking atrous convolution for semantic image segmentation. arXiv preprint arXiv:1706.05587, 2017.
中文翻译:[9] 梁哲陈,乔治·帕帕纳德雷欧,弗洛里安·施罗夫,哈特维希·亚当. 重新思考用于语义图像分割的扩张卷积. arXiv预印本arXiv:1706.05587, 2017.
[10] Tianqi Chen, Mu Li, Yutian Li, Min Lin, Naiyan Wang, Minjie Wang, Tianjun Xiao, Bing Xu, Chiyuan Zhang, and Zheng Zhang. Mxnet: A flexible and efficient machine learning library for heterogeneous distributed systems. arXiv preprint arXiv:1512.01274, 2015.
中文翻译:[10] 陈天奇,李牧,李宇田,林敏,王乃岩,王敏杰,肖天军,徐冰,张驰原,张正. Mxnet:一个用于异构分布式系统的灵活高效的机器学习库. arXiv预印本arXiv:1512.01274, 2015.
[11] Marius Cordts, Mohamed Omran, Sebastian Ramos, Timo Rehfeld, Markus Enzweiler, Rodrigo Benenson, Uwe Franke, Stefan Roth, and Bernt Schiele. The cityscapes dataset for semantic urban scene understanding. In Proceedings of the IEEE conference on computer vision and pattern recognition, pages 3213–3223, 2016.
中文翻译:[11] 玛里乌斯·科德茨,穆罕默德·奥姆兰,塞巴斯蒂安·拉莫斯,蒂莫·雷夫尔德,马库斯·恩茨韦勒,罗德里戈·贝内森,乌韦·弗兰克,斯特凡·罗特,伯恩特·谢勒. Cityscapes数据集用于语义城市场景理解. 在IEEE计算机视觉与模式识别会议论文集,第3213-3223页,2016.
[12] Ekin D Cubuk, Barret Zoph, Dandelion Mane, Vijay Vasudevan, and Quoc V Le. Autoaugment: Learning augmentation strategies from data. In Proceedings of the IEEE conference on computer vision and pattern recognition, pages 113–123, 2019.
中文翻译:[12] 艾金·D·库布克,巴雷特·佐夫,蒲公英·曼,维杰·瓦苏德万,郭·V·勒. Autoaugment:从数据中学习增强策略. 在IEEE计算机视觉与模式识别会议论文集,第113-123页,2019.
[13] Jifeng Dai, Haozhi Qi, Yuwen Xiong, Yi Li, Guodong Zhang, Han Hu, and Yichen Wei. Deformable convolutional networks. In Proceedings of the IEEE international conference on computer vision, pages 764–773, 2017.
中文翻译:[13] 戴继峰,齐浩志,熊宇文,李毅,张国栋,胡汉,魏义臣. 可变形卷积网络. 在IEEE国际计算机视觉会议论文集,第764-773页,2017.
[14] J. Deng, W. Dong, R. Socher, L.-J. Li, K. Li, and L. Fei-Fei. ImageNet: A Large-Scale Hierarchical Image Database. In CVPR09, 2009.
中文翻译:[14] J.邓,W.董,R.索切尔,L.-J.李,K.李,L.费-费. ImageNet:一个大规模层次图像数据库. 在CVPR09, 2009.
[15] Jun Fu, Jing Liu, Haijie Tian, Yong Li, Yongjun Bao, Zhiwei Fang, and Hanqing Lu. Dual Attention Network for Scene Segmentation. 2019.
中文翻译:[15] 付军,刘静,田海杰,李勇,鲍永军,方志伟,卢汉清. 场景分割的双注意力网络. 2019.
[16] Jun Fu, Jing Liu, Yuhang Wang, Yong Li, Yongjun Bao, Jinhui Tang, and Hanqing Lu. Adaptive context network for scene parsing. In Proceedings of the IEEE international conference on computer vision, pages 6748–6757, 2019.
中文翻译:[16] 付军,刘静,王宇航,李勇,鲍永军,唐金辉,卢汉清. 场景解析的自适应上下文网络. 在IEEE国际计算机视觉会议论文集,第6748-6757页,2019.
[17] Golnaz Ghiasi, Tsung-Yi Lin, and Quoc V Le. Dropblock: A regularization method for convolutional networks. In Advances in Neural Information Processing Systems, pages 10727–10737, 2018.
中文翻译:[17] 戈尔纳兹·加西亚,林宗义,郭·V·勒. Dropblock:卷积网络的正则化方法. 在神经信息处理系统进展,第10727-10737页,2018.
[18] Priya Goyal, Piotr Doll´ar, Ross Girshick, Pieter Noordhuis, Lukasz Wesolowski, Aapo Kyrola, Andrew Tulloch, Yangqing Jia, and Kaiming He. Accurate, large minibatch sgd: Training imagenet in 1 hour. arXiv preprint arXiv:1706.02677, 2017.
中文翻译:[18] 普里亚·戈亚尔,皮奥特·多拉尔,罗斯·吉什克,皮特·诺德豪斯,卢卡斯·韦索洛夫斯基,阿波·基罗拉,安德鲁·图洛克,杨庆佳,何恺明. 准确的大批量SGD:1小时内训练ImageNet. arXiv预印本arXiv:1706.02677, 2017.
[19] Jian Guo, He He, Tong He, Leonard Lausen, Mu Li, Haibin Lin, Xingjian Shi, Chenguang Wang, Junyuan Xie, Sheng Zha, et al. Gluoncv and gluonnlp: Deep learning in computer vision and natural language processing. Journal of Machine Learning Research, 21(23):1–7, 2020.
中文翻译:[19] 郭建,何贺,贺同,莱昂纳德·劳森,李牧,林海斌,史兴健,王晨光,谢俊元,赵胜等. Gluoncv和Gluonnlp:计算机视觉和自然语言处理中的深度学习. 机器学习研究杂志,21(23):1-7, 2020.
[20] Jian Guo, He He, Tong He, Leonard Lausen, Mu Li, Haibin Lin, Xingjian Shi, Chenguang Wang, Junyuan Xie, Sheng Zha, Aston Zhang, Hang Zhang, Zhi Zhang, Zhongyue Zhang, Shuai Zheng, and Yi Zhu. Gluoncv and gluonnlp: Deep learning in computer vision and natural language processing. Journal of Machine Learning Research, 21(23):1–7, 2020.
中文翻译:[20] 郭建,何贺,贺同,莱昂纳德·劳森,李牧,林海斌,史兴健,王晨光,谢俊元,赵胜,张阿斯顿,张航,张志,张仲岳,郑帅,朱逸. Gluoncv和Gluonnlp:计算机视觉和自然语言处理中的深度学习. 机器学习研究杂志,21(23):1-7, 2020.
[21] Jianhua Han, Minzhe Niu, Zewei Du, Longhui Wei, Lingxi Xie, Xiaopeng Zhang, and Qi Tian. Asynchronous semisupervised learning for large vocabulary instance segmentation, 2020.
中文翻译:[21] 韩建华,牛敏哲,杜泽伟,魏龙辉,谢凌西,张晓鹏,田琦. 大词汇量实例分割的异步半监督学习, 2020.
[22] Kaiming He, Georgia Gkioxari, Piotr Doll´ar, and Ross Girshick. Mask r-cnn. arXiv preprint arXiv:1703.06870, 2017.
中文翻译:[22] 何恺明,乔治亚·吉奥克斯里,皮奥特·多拉尔,罗斯·吉什克. Mask R-CNN. arXiv预印本arXiv:1703.06870, 2017.
[23] Kaiming He, Xiangyu Zhang, Shaoqing Ren, and Jian Sun. Deep residual learning for image recognition. arXiv preprint arXiv:1512.03385, 2015.
中文翻译:[23] 何恺明,张翔宇,任少庆,孙剑. 深度残差学习用于图像识别. arXiv预印本arXiv:1512.03385, 2015.
[24] Kaiming He, Xiangyu Zhang, Shaoqing Ren, and Jian Sun. Delving deep into rectifiers: Surpassing human-level performance on imagenet classification. In Proceedings of the IEEE international conference on computer vision, pages 1026–1034, 2015.
中文翻译:[24] 何恺明,张翔宇,任少庆,孙剑. 深入研究整流器:在ImageNet分类上超越人类水平的表现. 在IEEE国际计算机视觉会议论文集,第1026-1034页,2015.
[25] Tong He, Zhi Zhang, Hang Zhang, Zhongyue Zhang, Junyuan Xie, and Mu Li. Bag of tricks to train convolutional neural networks for image classification. arXiv preprint arXiv:1812.01187, 2018.
中文翻译:[25] 贺同,张志,张航,张仲岳,谢俊元,李牧. 训练卷积神经网络用于图像分类的技巧包. arXiv预印本arXiv:1812.01187, 2018.
[26] Tong He, Zhi Zhang, Hang Zhang, Zhongyue Zhang, Junyuan Xie, and Mu Li. Bag of tricks for image classification with convolutional neural networks. In Proceedings of the IEEE Conference on Computer Vision and Pattern Recognition, pages 558–567, 2019.
中文翻译:[26] 贺同,张志,张航,张仲岳,谢俊元,李牧. 使用卷积神经网络进行图像分类的技巧包. 在IEEE计算机视觉与模式识别会议论文集,第558-567页,2019.
[27] Jie Hu, Li Shen, and Gang Sun. Squeeze-and-excitation networks. arXiv preprint arXiv:1709.01507, 2017.
中文翻译:[27] 胡杰,沈力,孙刚. 挤压-激励网络. arXiv预印本arXiv:1709.01507, 2017.
[28] Jie Hu, Li Shen, and Gang Sun. Squeeze-and-excitation networks. In Proceedings of the IEEE conference on computer vision and pattern recognition, pages 7132–7141, 2018.
中文翻译:[28] 胡杰,沈力,孙刚. 挤压-激励网络. 在IEEE计算机视觉与模式识别会议论文集,第7132-7141页,2018.
[29] Gao Huang, Zhuang Liu, Kilian Q Weinberger, and Laurens van der Maaten. Densely connected convolutional networks. arXiv preprint arXiv:1608.06993, 2016.
中文翻译:[29] 黄高,刘壮,温伯格·K·Q,马滕·范德. 密集连接卷积网络. arXiv预印本arXiv:1608.06993, 2016.
[30] David H Hubel and Torsten N Wiesel. Receptive fields, binocular interaction and functional architecture in the cat’s visual cortex. The Journal of physiology, 160(1):106, 1962.
中文翻译:[30] 大卫·H·休贝尔,托尔斯滕·N·威塞尔. 猫视觉皮层的感受野、双眼相互作用和功能结构. 生理学杂志,160(1):106, 1962.
[31] Sergey Ioffe and Christian Szegedy. Batch normalization: Accelerating deep network training by reducing internal covariate shift. In International Conference on Machine Learning, pages 448–456, 2015.
中文翻译:[31] 谢尔盖·伊奥菲,克里斯蒂安·谢盖迪. 批量归一化:通过减少内部协变量偏移加速深度网络训练. 在国际机器学习会议论文集,第448-456页,2015.
[32] Tsung-Wei Ke, Jyh-Jing Hwang, Ziwei Liu, and Stella X. Yu. Adaptive Affinity Fields for Semantic Segmentation. In European Conference on Computer Vision (ECCV), 2018.
中文翻译:[32] 柯宗玮,黄吉靖,刘子维,余晓霞. 语义分割的自适应亲和场. 在欧洲计算机视觉会议(ECCV),2018.
[33] Alex Krizhevsky, Ilya Sutskever, and Geoffrey E Hinton. Imagenet classification with deep convolutional neural networks. In Advances in neural information processing systems, pages 1097–1105, 2012.
中文翻译:[33] 亚历克斯·克里热夫斯基,伊利亚·苏茨克弗,杰弗里·E·辛顿. 使用深度卷积神经网络进行ImageNet分类. 在神经信息处理系统进展,第1097-1105页,2012.
[34] Yann LeCun, L´eon Bottou, Yoshua Bengio, and Patrick Haffner. Gradient-based learning applied to document recognition. Proceedings of the IEEE, 86(11):2278–2324, 1998.
中文翻译:[34] 扬·勒昆,莱昂·博图,约书亚·本吉奥,帕特里克·哈夫纳. 应用于文档识别的基于梯度的学习. IEEE学报,86(11):2278-2324, 1998.
[35] Mu Li. Scaling distributed machine learning with system and algorithm co-design. PhD thesis, PhD thesis, Intel, 2017.
中文翻译:[35] 李牧. 通过系统和算法协同设计扩展分布式机器学习. 博士论文,博士论文,英特尔,2017.
[36] Xiang Li, Wenhai Wang, Xiaolin Hu, and Jian Yang. Selective kernel networks. In Proceedings of the IEEE conference on computer vision and pattern recognition, pages 510–519, 2019.
中文翻译:[36] 李翔,王文海,胡晓林,杨健. 选择性核网络. 在IEEE计算机视觉与模式识别会议论文集,第510-519页,2019.
[37] Haibin Lin, Hang Zhang, Yifei Ma, Tong He, Zhi Zhang, Sheng Zha, and Mu Li. Dynamic mini-batch sgd for elastic distributed training: Learning in the limbo of resources. arXiv preprint arXiv:1904.12043, 2019.
中文翻译:[37] 林海斌,张航,马一飞,贺同,张志,赵胜,李牧. 动态小批量SGD用于弹性分布式训练:在资源的边缘学习. arXiv预印本arXiv:1904.12043, 2019.
[38] Min Lin, Qiang Chen, and Shuicheng Yan. Network in network. arXiv preprint arXiv:1312.4400, 2013.
中文翻译:[38] 林敏,陈强,严水城. 网络中的网络. arXiv预印本arXiv:1312.4400, 2013.
[39] Tsung-Yi Lin, Piotr Doll´ar, Ross Girshick, Kaiming He, Bharath Hariharan, and Serge Belongie. Feature pyramid networks for object detection. In Proceedings of the IEEE conference on computer vision and pattern recognition, pages 2117–2125, 2017.
中文翻译:[39] 林宗义,皮奥特·多拉尔,罗斯·吉什克,何恺明,哈拉里哈兰·巴拉特,塞尔日·贝洛尼. 特征金字塔网络用于目标检测. 在IEEE计算机视觉与模式识别会议论文集,第2117-2125页,2017.
[40] Tsung-Yi Lin, Michael Maire, Serge Belongie, James Hays, Pietro Perona, Deva Ramanan, Piotr Doll´ar, and C Lawrence Zitnick. Microsoft coco: Common objects in context. In European conference on computer vision, pages 740–755. Springer, 2014.
中文翻译:[40] 林宗义,迈克尔·马尔,塞尔日·贝洛尼,詹姆斯·海斯,皮埃尔·佩罗纳,德瓦·拉马南,皮奥特·多拉尔,C·劳伦斯·齐特尼克. 微软COCO:上下文中的常见物体. 在欧洲计算机视觉会议论文集,第740-755页. 施普林格,2014.
[41] Hanxiao Liu, Karen Simonyan, and Yiming Yang. Darts: Differentiable architecture search. arXiv preprint arXiv:1806.09055, 2018.
中文翻译:[41] 刘汉宵,凯伦·西蒙尼扬,杨亦明. Darts:可微架构搜索. arXiv预印本arXiv:1806.09055, 2018.
[42] Vinod Nair and Geoffrey E Hinton. Rectified linear units improve restricted boltzmann machines. In Proceedings of the 27th international conference on machine learning (ICML10), pages 807–814, 2010.
中文翻译:[42] 文诺德·奈尔,杰弗里·E·辛顿. 修正线性单元改进限制玻尔兹曼机. 在第27届国际机器学习会议(ICML10)论文集,第807-814页,2010.
[43] Alejandro Newell, Kaiyu Yang, and Jia Deng. Stacked hourglass networks for human pose estimation. In European conference on computer vision, pages 483–499. Springer, 2016.
中文翻译:[43] 亚历杭德罗·纽厄尔,杨凯宇,邓嘉. 用于人体姿态估计的堆叠沙漏网络. 在欧洲计算机视觉会议论文集,第483-499页. 施普林格,2016.
[44] Hieu Pham, Melody Y Guan, Barret Zoph, Quoc V Le, and Jeff Dean. Efficient neural architecture search via parameter sharing. arXiv preprint arXiv:1802.03268, 2018.
中文翻译:[44] 高效神经架构搜索通过参数共享. arXiv预印本arXiv:1802.03268, 2018.
[45] Rishi Rajalingham and James J DiCarlo. Reversible inactivation of different millimeter-scale regions of primate it results in different patterns of core object recognition deficits. Neuron, 102(2):493–505, 2019.
中文翻译:[45] 里希·拉贾林加姆,詹姆斯·J·迪卡洛. 灵长类动物不同毫米级区域的可逆失活导致不同的核心物体识别缺陷模式. 神经元,102(2):493-505, 2019.
[46] Esteban Real, Alok Aggarwal, Yanping Huang, and Quoc V Le. Regularized evolution for image classifier architecture search. In Proceedings of the aaai conference on artificial intelligence, volume 33, pages 4780–4789, 2019.
中文翻译:[46] 埃斯特班·雷亚尔,阿洛克·阿加瓦尔,黄艳平,郭·V·勒. 图像分类器架构搜索的正则化进化. 在AAAI人工智能会议论文集,第33卷,第4780-4789页,2019.
[47] Shaoqing Ren, Kaiming He, Ross Girshick, and Jian Sun. Faster r-cnn: Towards real-time object detection with region proposal networks. In Advances in neural information processing systems, pages 91–99, 2015.
中文翻译:[47] 任少庆,何恺明,罗斯·吉什克,孙剑. Faster R-CNN:使用区域提议网络实现实时目标检测. 在神经信息处理系统进展,第91-99页,2015.
[48] Karen Simonyan and Andrew Zisserman. Very deep convolutional networks for large-scale image recognition. arXiv preprint arXiv:1409.1556, 2014.
中文翻译:[48] 卡伦·西蒙尼扬,安德鲁·齐瑟曼. 大规模图像识别的非常深的卷积网络. arXiv预印本arXiv:1409.1556, 2014.
[49] Bharat Singh, Mahyar Najibi, and Larry S Davis. Sniper: Efficient multi-scale training. In Advances in neural information processing systems, pages 9310–9320, 2018.
中文翻译:[49] 巴拉特·辛格,马哈扬·纳吉比,拉里·S·戴维斯. Sniper:高效的多尺度训练. 在神经信息处理系统进展,第9310-9320页,2018.
[50] Nitish Srivastava, Geoffrey E Hinton, Alex Krizhevsky, Ilya Sutskever, and Ruslan Salakhutdinov. Dropout: a simple way to prevent neural networks from overfitting. Journal of machine learning research, 15(1):1929–1958, 2014.
中文翻译:[50] 尼提什·斯里瓦斯塔瓦,杰弗里·E·辛顿,亚历克斯·克里热夫斯基,伊利亚·苏茨克弗,鲁斯兰·萨拉胡丁诺夫. Dropout:防止神经网络过拟合的简单方法. 机器学习研究杂志,15(1):1929-1958, 2014.
[51] Rupesh Kumar Srivastava, Klaus Greff, and Jürgen Schmidhuber. Highway networks. arXiv preprint arXiv:1505.00387, 2015.
中文翻译:[51] 鲁佩什·库马尔·斯里瓦斯塔瓦,克劳斯·格雷夫,尤尔根·施密德胡伯. 高速网络. arXiv预印本arXiv:1505.00387, 2015.
[52] Christian Szegedy, Sergey Ioffe, Vincent Vanhoucke, and Alexander A Alemi. Inception-v4, inception-resnet and the impact of residual connections on learning. In Thirty-first AAAI conference on artificial intelligence, 2017.
中文翻译:[52] 克里斯蒂安·谢盖迪,谢尔盖·伊奥菲,文森特·范霍克,亚历山大·A·阿莱米. Inception-V4,Inception-ResNet以及残差连接对学习的影响. 在第三十一届AAAI人工智能会议,2017.
[53] Christian Szegedy, Wei Liu, Yangqing Jia, Pierre Sermanet, Scott Reed, Dragomir Anguelov, Dumitru Erhan, Vincent Vanhoucke, and Andrew Rabinovich. Going deeper with convolutions. In Proceedings of the IEEE conference on computer vision and pattern recognition, pages 1–9, 2015.
中文翻译:[53] 克里斯蒂安·谢盖迪,刘伟,贾阳庆,皮埃尔·瑟曼内特,斯科特·里德,德拉戈米尔·安格洛夫,杜米特鲁·埃汉,文森特·范霍克,安德鲁·拉宾诺维奇. 使用卷积深入研究. 在IEEE计算机视觉与模式识别会议论文集,第1-9页,2015.
[54] Christian Szegedy, Vincent Vanhoucke, Sergey Ioffe, Jon Shlens, and Zbigniew Wojna. Rethinking the inception architecture for computer vision. In Proceedings of the IEEE conference on computer vision and pattern recognition, pages 2818–2826, 2016.
中文翻译:[54] 克里斯蒂安·谢盖迪,文森特·范霍克,谢尔盖·伊奥菲,乔恩·什伦斯,齐格涅夫·沃伊纳. 重新思考用于计算机视觉的Inception架构. 在IEEE计算机视觉与模式识别会议论文集,第2818-2826页,2016.
[55] Jingru Tan, Gang Zhang, Hanming Deng, Changbao Wang, Lewei Lu, Quanquan Li, and Jifeng Dai. 1st place solution of lvis challenge 2020: A good box is not a guarantee of a good mask. arXiv preprint arXiv:2009.01559, 2020.
中文翻译:[55] 谭静如,张刚,邓汉明,王长宝,卢磊伟,李全全,戴继峰. 2020年LVIS挑战赛第一名解决方案:一个好的框并不能保证一个好的掩码. arXiv预印本arXiv:2009.01559, 2020.
[56] Mingxing Tan and Quoc V Le. Efficientnet: Rethinking model scaling for convolutional neural networks. arXiv preprint arXiv:1905.11946, 2019.
中文翻译:[56] 谭明兴,郭·V·勒. EfficientNet:重新思考卷积神经网络的模型缩放. arXiv预印本arXiv:1905.11946, 2019.
[57] Jiaqi Wang, Wenwei Zhang, Yuhang Zang, Yuhang Cao, Jiangmiao Pang, Tao Gong, Kai Chen, Ziwei Liu, Chen Change Loy, and Dahua Lin. Seesaw loss for long-tailed instance segmentation. arXiv preprint arXiv:2008.10032, 2020.
中文翻译:[57] 王佳琪,张文伟,臧宇航,曹宇航,庞江苗,龚涛,陈凯,刘子维,陈改变忠诚,林大华. 长尾实例分割的锯齿形损失. arXiv预印本arXiv:2008.10032, 2020.
[58] Xiaolong Wang, Ross Girshick, Abhinav Gupta, and Kaiming He. Non-local neural networks. In The IEEE Conference on Computer Vision and Pattern Recognition (CVPR), June 2018.
中文翻译:[58] 王小龙,罗斯·吉什克,阿宾纳夫·古普塔,何恺明. 非局部神经网络. 在IEEE计算机视觉与模式识别会议(CVPR),2018年6月.
[59] Bichen Wu, Xiaoliang Dai, Peizhao Zhang, Yanghan Wang, Fei Sun, Yiming Wu, Yuandong Tian, Peter Vajda, Yangqing Jia, and Kurt Keutzer. Fbnet: Hardware-aware efficient convnet design via differentiable neural architecture search. In Proceedings of the IEEE Conference on Computer Vision and Pattern Recognition, pages 10734–10742, 2019.
中文翻译:[59] 吴必成,戴晓亮,张培兆,王阳汉,孙飞,吴一鸣,田远东,彼得·瓦伊达,贾扬清,库尔特·凯泽. Fbnet:通过可微神经架构搜索实现硬件感知的高效卷积网络设计. 在IEEE计算机视觉与模式识别会议论文集,第10734-10742页,2019.
[60] Yuxin Wu, Alexander Kirillov, Francisco Massa, Wan-Yen Lo, and Ross Girshick. Detectron2. https://github.com/facebookresearch/detectron2, 2019.
中文翻译:[60] 吴宇欣,亚历山大·基里洛夫,弗朗西斯科·马萨,罗万-恩·洛,罗斯·吉什克. Detectron2. https://github.com/facebookresearch/detectron2, 2019.
[61] Bin Xiao, Haiping Wu, and Yichen Wei. Simple baselines for human pose estimation and tracking. In Proceedings of the European conference on computer vision (ECCV), pages 466–481, 2018.
中文翻译:[61] 萧斌,吴海平,魏义臣. 人体姿态估计和跟踪的简单基线. 在欧洲计算机视觉会议(ECCV)论文集,第466-481页,2018.
[62] Tete Xiao, Yingcheng Liu, Bolei Zhou, Yuning Jiang, and Jian Sun. Unified perceptual parsing for scene understanding. arXiv preprint arXiv:1807.10221, 2018.
中文翻译:[62] 萧特特,刘英城,周博磊,姜云宁,孙剑. 场景理解的统一感知解析. arXiv预印本arXiv:1807.10221, 2018.
[63] Saining Xie, Ross Girshick, Piotr Doll´ar, Zhuowen Tu, and Kaiming He. Aggregated residual transformations for deep neural networks. arXiv preprint arXiv:1611.05431, 2016.
中文翻译:[63] 谢赛宁,罗斯·吉什克,皮奥特·多拉尔,涂卓文,何恺明. 深度神经网络的聚合残差变换. arXiv预印本arXiv:1611.05431, 2016.
[64] Saining Xie, Ross Girshick, Piotr Doll´ar, Zhuowen Tu, and Kaiming He. Aggregated residual transformations for deep neural networks. In Proceedings of the IEEE conference on computer vision and pattern recognition, pages 1492–1500, 2017.
中文翻译:[64] 谢赛宁,罗斯·吉什克,皮奥特·多拉尔,涂卓文,何恺明. 深度神经网络的聚合残差变换. 在IEEE计算机视觉与模式识别会议论文集,第1492-1500页,2017.
[65] Fisher Yu and Vladlen Koltun. Multi-scale context aggregation by dilated convolutions. arXiv preprint arXiv:1511.07122, 2015.
中文翻译:[65] 尤费舍,科特恩·弗拉德伦. 通过扩张卷积进行多尺度上下文聚合. arXiv预印本arXiv:1511.07122, 2015.
[66] Yuhui Yuan, Xilin Chen, and Jingdong Wang. Object-contextual representations for semantic segmentation. arXiv preprint arXiv:1909.11065, 2019.
中文翻译:[66] 袁玉辉,陈西林,王景东. 语义分割的对象上下文表示. arXiv预印本arXiv:1909.11065, 2019.
[67] Hongyi Zhang, Moustapha Cisse, Yann N Dauphin, and David Lopez-Paz. mixup: Beyond empirical risk minimization. arXiv preprint arXiv:1710.09412, 2017.
中文翻译:[67] 张洪毅,穆斯塔法·西塞,扬·N·多芬,大卫·洛佩兹-帕兹. mixup:超越经验风险最小化. arXiv预印本arXiv:1710.09412, 2017.
[68] Hang Zhang, Kristin Dana, Jianping Shi, Zhongyue Zhang, Xiaogang Wang, Ambrish Tyagi, and Amit Agrawal. Context encoding for semantic segmentation. In The IEEE Conference on Computer Vision and Pattern Recognition (CVPR), June 2018.
中文翻译:[68] 张航,克里斯汀·达纳,史建平,张仲岳,王小刚,阿姆布里什·提亚吉,阿米特·阿格拉瓦尔. 语义分割的上下文编码. 在IEEE计算机视觉与模式识别会议(CVPR),2018年6月.
[69] Hang Zhang, Han Zhang, Chenguang Wang, and Junyuan Xie. Co-occurrent features in semantic segmentation. In Proceedings of the IEEE Conference on Computer Vision and Pattern Recognition, pages 548–557, 2019.
中文翻译:[69] 张航,张汉,王晨光,谢俊元. 语义分割中的共现特征. 在IEEE计算机视觉与模式识别会议论文集,第548-557页,2019.
[70] Xingcheng Zhang, Zhizhong Li, Chen Change Loy, and Dahua Lin. Polynet: A pursuit of structural diversity in very deep networks. In Proceedings of the IEEE Conference on Computer Vision and Pattern Recognition, pages 718–726, 2017.
中文翻译:[70] 张兴城,李治忠,陈改变忠诚,林大华. Polynet:在非常深的网络中追求结构多样性. 在IEEE计算机视觉与模式识别会议论文集,第718-726页,2017.
[71] Hengshuang Zhao, Jianping Shi, Xiaojuan Qi, Xiaogang Wang, and Jiaya Jia. Pyramid scene parsing network. In Proceedings of IEEE Conference on Computer Vision and Pattern Recognition (CVPR), 2017.
中文翻译:[71] 赵恒爽,史建平,齐晓娟,王小刚,贾亚家. 金字塔场景解析网络. 在IEEE计算机视觉与模式识别会议(CVPR),2017.
[72] Hengshuang Zhao, Yi Zhang, Shu Liu, Jianping Shi, Chen Change Loy, Dahua Lin, and Jiaya Jia. PSANet: Pointwise Spatial Attention Network for Scene Parsing. In European Conference on Computer Vision (ECCV), 2018.
中文翻译:[72] 赵恒爽,张一,刘树,史建平,陈改变忠诚,林大华,贾亚家. PSANet:场景解析的逐点空间注意力网络. 在欧洲计算机视觉会议(ECCV),2018.
[73] Li Zhaoping and Zhaoping Li. Understanding vision: theory, models, and data. Oxford University Press, USA, 2014.
中文翻译:[73] 李兆平,李兆平. 理解视觉:理论、模型和数据. 牛津大学出版社,美国,2014.
[74] Bolei Zhou, Agata Lapedriza, Jianxiong Xiao, Antonio Torralba, and Aude Oliva. Learning deep features for scene recognition using places database. In Advances in Neural Information Processing Systems, pages 487–495, 2014.
中文翻译:[74] 周博磊,阿加塔·拉佩德里扎,肖建雄,安东尼奥·托拉尔巴,奥黛·奥利瓦. 使用Places数据库学习深度特征进行场景识别. 在神经信息处理系统进展,第487-495页,2014.
[75] Bolei Zhou, Hang Zhao, Xavier Puig, Sanja Fidler, Adela Barriuso, and Antonio Torralba. Scene parsing through ade20k dataset. In Proc. CVPR, 2017.
中文翻译:[75] 周博磊,赵航,普伊格·哈维尔,桑贾·菲德勒,阿德拉·巴里乌索,安东尼奥·托拉尔巴. 通过ADE20K数据集进行场景解析. 在CVPR会议论文集,2017.
[76] Xizhou Zhu, Han Hu, Stephen Lin, and Jifeng Dai. Deformable convnets v2: More deformable, better results. In Proceedings of the IEEE Conference on Computer Vision and Pattern Recognition, pages 9308–9316, 2019.
中文翻译:[76] 朱西周,胡汉,林史蒂芬,戴继峰. 可变形卷积网络V2:更可变形,更好的结果. 在IEEE计算机视觉与模式识别会议论文集,第9308-9316页,2019.
[77] Yi Zhu, Karan Sapra, Fitsum A. Reda, Kevin J. Shih, Shawn Newsam, Andrew Tao, and Bryan Catanzaro. Improving Semantic Segmentation via Video Propagation and Label Relaxation. 2019.
中文翻译:[77] 朱毅,卡兰·萨普拉,菲茨姆·A·雷达,凯文·J·希,肖恩·纽萨姆,安德鲁·陶,布莱恩·卡坦扎罗. 通过视频传播和标签松弛改进语义分割. 2019.
[78] Barret Zoph, Vijay Vasudevan, Jonathon Shlens, and Quoc V Le. Learning transferable architectures for scalable image recognition. In Proceedings of the IEEE conference on computer vision and pattern recognition, pages 8697–8710, 2018.
中文翻译:[78] 巴雷特·佐夫,维杰·瓦苏德万,乔纳森·什伦斯,郭·V·勒. 学习可迁移架构用于可扩展图像识别. 在IEEE计算机视觉与模式识别会议论文集,第8697-8710页,2018.
相关文章:
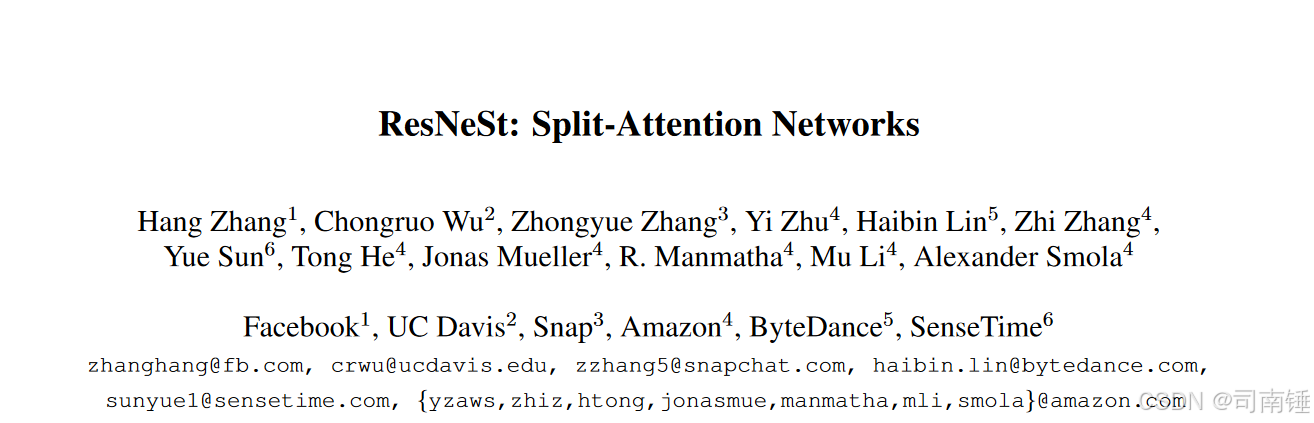
ResNeSt: Split-Attention Networks 参考论文
参考文献 [1] Tensorflow Efficientnet. https://github.com/tensorflow/tpu/tree/master/models/official/efficientnet. Accessed: 2020-03-04. 中文翻译:[1] TensorFlow EfficientNet. https://github.com/tensorflow/tpu/tree/master/models/official/efficien…...
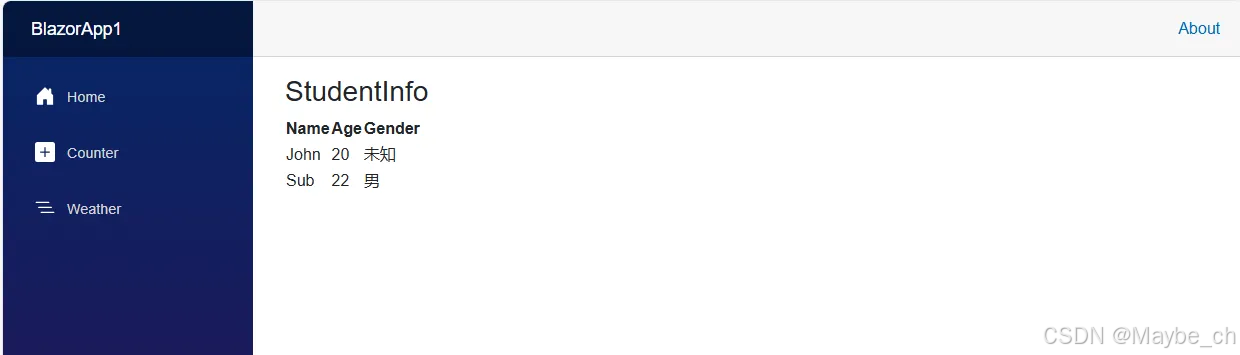
Blazor-选择循环语句
今天我们来说说Blazor选择语句和循环语句。 下面我们以一个简单的例子来讲解相关的语法,我已经创建好了一个Student类,以此类来进行语法的运用 因为我们需要交互性所以我们将类创建在*.client目录下 if 我们做一个学生信息的显示,Gender为…...
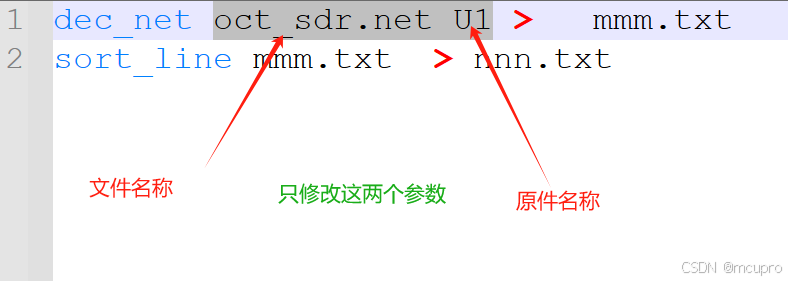
从AD的原理图自动提取引脚网络的小工具
这里跟大家分享一个我自己写的小软件,实现从AD的原理图里自动找出网络名称和引脚的对应。存成文本方便后续做表格或是使用简单行列编辑生成引脚约束文件(如.XDC .UCF .TCL等)。 我们在FPGA设计中需要引脚锁定文件,就是指示TOP层…...

苍穹外卖使用MyBatis-Plus
系列博客目录 文章目录 系列博客目录一、修改sky-take-out项目的pom.xml文件1.修改lombok依赖的版本号2.修改spring-boot-starter-parent父工程的版本号3.增加依赖 二、修改sky-server模块的pom.xml文件1.增加mysql连接的依赖(版本为8.0以上)2.增加两个依…...
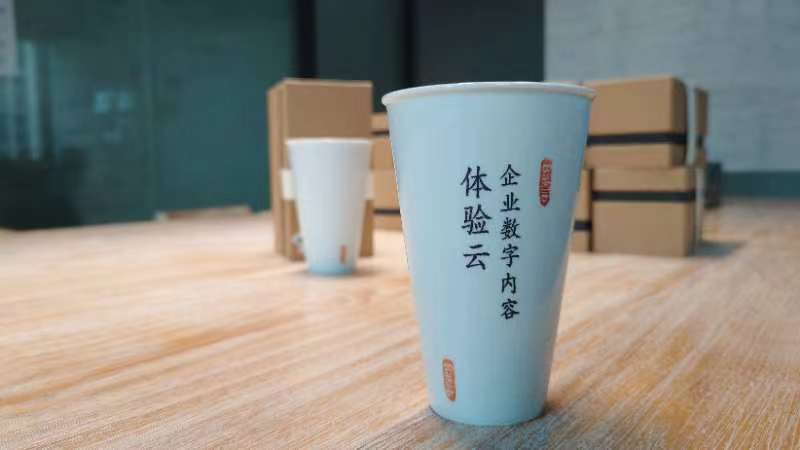
Baklib引领数字化内容管理转型提升企业运营效率
内容概要 在数字化迅速发展的背景下,企业正面临着前所未有的内容管理挑战。传统的内容管理方式已难以适应如今的信息爆炸,企业需要更加高效、智能的解决方案以应对复杂的数据处理需求。Baklib作为行业的先锋,以其创新技术对数字化内容管理进…...
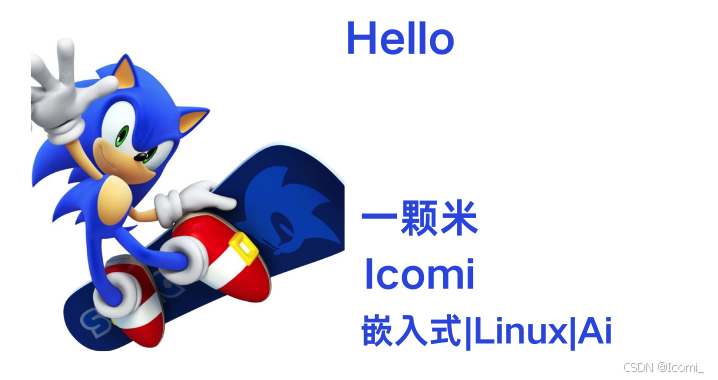
【PyTorch】4.张量拼接操作
个人主页:Icomi 在深度学习蓬勃发展的当下,PyTorch 是不可或缺的工具。它作为强大的深度学习框架,为构建和训练神经网络提供了高效且灵活的平台。神经网络作为人工智能的核心技术,能够处理复杂的数据模式。通过 PyTorch࿰…...
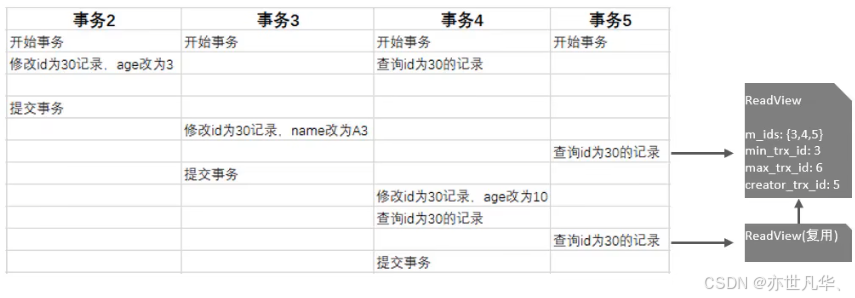
MySQL--》深度解析InnoDB引擎的存储与事务机制
目录 InnoDB架构 事务原理 MVCC InnoDB架构 从MySQL5.5版本开始默认使用InnoDB存储引擎,它擅长进行事务处理,具有崩溃恢复的特性,在日常开发中使用非常广泛,其逻辑存储结构图如下所示, 下面是InnoDB架构图…...
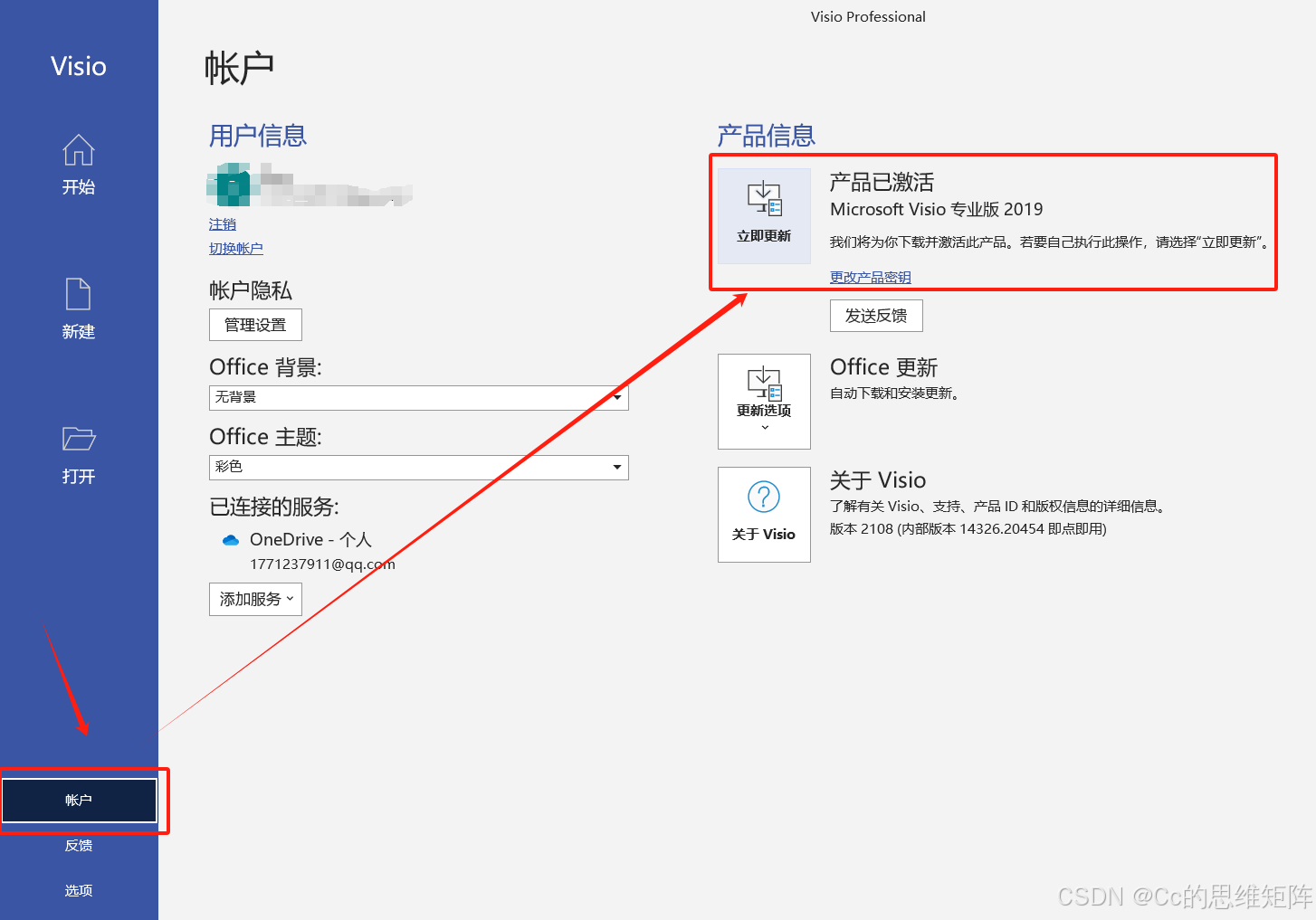
Visio2021下载与安装教程
这里写目录标题 软件下载软件介绍安装步骤 软件下载 软件名称:Visio2021软件语言:简体中文软件大小:4.28G系统要求:Windows10或更高,64位操作系统硬件要求:CPU2GHz ,RAM4G或更高下载链接&#…...

实战纪实 | 真实HW漏洞流量告警分析
视频教程在我主页简介和专栏里 目录: 一、web.xml 文件泄露 二、Fastjson 远程代码执行漏洞 三、hydra工具爆破 四、绕过验证,SQL攻击成功 五、Struts2代码执行 今年七月,我去到了北京某大厂参加HW行动,因为是重点领域—-jr&…...
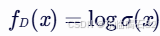
【AI论文】扩散对抗后训练用于一步视频生成总结
摘要:扩散模型被广泛应用于图像和视频生成,但其迭代生成过程缓慢且资源消耗大。尽管现有的蒸馏方法已显示出在图像领域实现一步生成的潜力,但它们仍存在显著的质量退化问题。在本研究中,我们提出了一种在扩散预训练后针对真实数据…...

重回C语言之老兵重装上阵(十六)C语言可变参数
C语言可变参数 在C语言中,标准库提供了一些函数允许接收可变数量的参数。最典型的例子就是 printf 和 scanf,它们能够处理不确定数量的参数。为了实现这一功能,C语言提供了可变参数函数的概念。 1. 可变参数函数的概念 可变参数函数是指函数…...

深拷贝、浅拷贝、移动语义
C 中的拷贝方式 1. 深拷贝(Deep Copy) 定义 深拷贝会复制对象的全部内容,包括对象中动态分配的资源。新对象与原对象完全独立,任何对新对象的修改都不会影响原对象。 实现 通常通过显式的拷贝构造函数或拷贝赋值运算符&#…...

双向链表在系统调度、游戏、文本编辑及组态方面的应用
在编程的奇妙世界里,数据结构就像是一把把神奇的钥匙(前面我们介绍过单向链表的基础了,这里我们更进一步),能帮我们打开解决各种问题的大门。今天,咱们就来聊聊其中一把特别的钥匙——双向链表。双向链表和…...

实践网络安全:常见威胁与应对策略详解
📝个人主页🌹:一ge科研小菜鸡-CSDN博客 🌹🌹期待您的关注 🌹🌹 引言 在数字化转型的浪潮中,网络安全的重要性已达到前所未有的高度。无论是个人用户、企业,还是政府机构…...

关于2024年
关于2024年 十分钟前我从床上爬起来,坐在电脑面前先后听了《黄金时代》——声音碎片和《Song F》——达达两首歌,我觉得躺着有些无聊,又或者除夕夜的晚上躺着让我觉得有些不适,我觉得自己应该爬起来,爬起来记录一下我…...
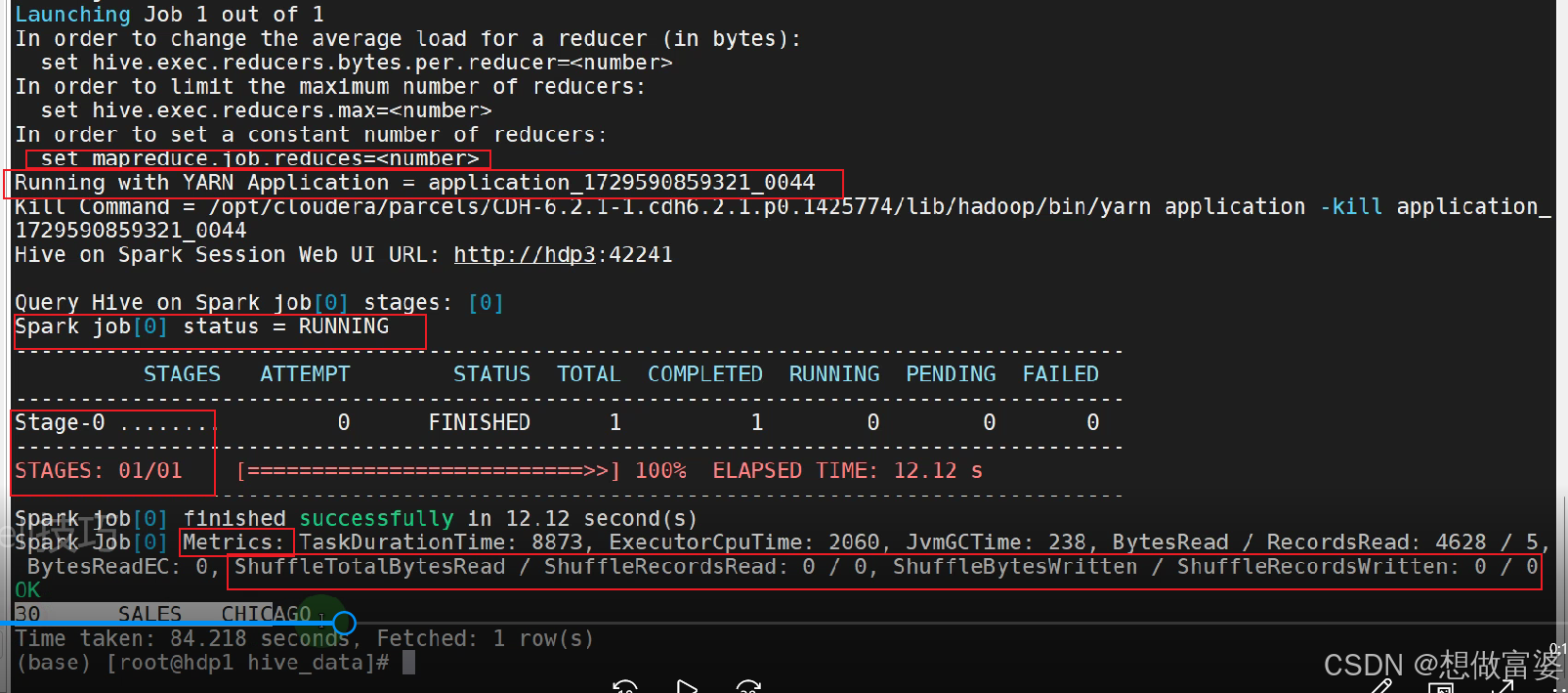
Hive:Hive Shell技巧
在终端命令窗口不能直接执行select,creat等HQL命令,需要先进入hive之后才能执行,比较麻烦,但是如果使用Hive Shell就可以直接执行 在终端只执行一次Hive命令 -e 参数, "execute"(执行),使用-e参数后会在执行完Hive的命令后退出Hive 使用场景:…...
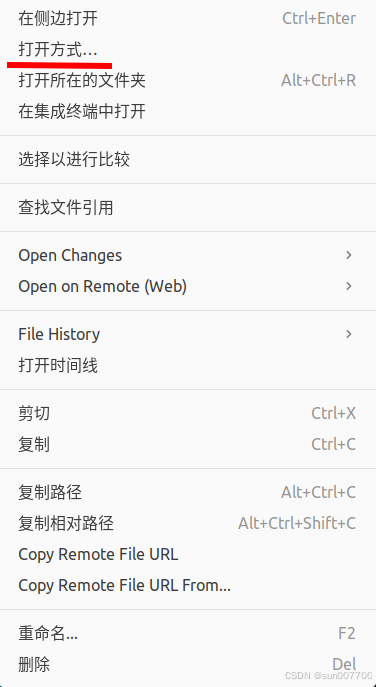
Markdown Viewer 浏览器, vscode
使用VS Code插件打造完美的MarkDown编辑器(插件安装、插件配置、markdown语法)_vscode markdown-CSDN博客 右键 .md 文件,选择打开 方式 (安装一些markdown的插件) vscode如何预览markdown文件 | Fromidea GitCode - 全球开发者…...
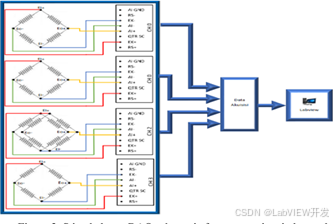
快速分析LabVIEW主要特征进行判断
在LabVIEW中,快速分析程序特征进行判断是提升开发效率和减少调试时间的重要技巧。本文将介绍如何高效地识别和分析程序的关键特征,从而帮助开发者在编写和优化程序时做出及时的判断,避免不必要的错误。 数据流和并行性分析 LabVIEW的图形…...
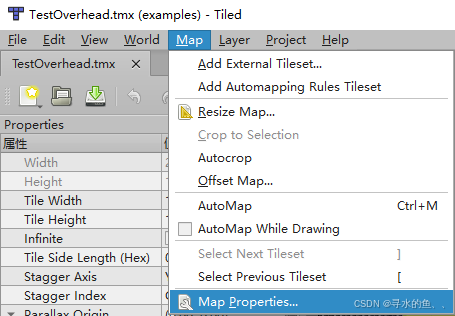
【Super Tilemap Editor使用详解】(十五):从 TMX 文件导入地图(Importing from TMX files)
Super Tilemap Editor 支持从 TMX 文件(Tiled Map Editor 的文件格式)导入图块地图。通过导入 TMX 文件,你可以将 Tiled 中设计的地图快速转换为 Unity 中的图块地图,并自动创建图块地图组(Tilemap Group)。以下是详细的导入步骤和准备工作。 一、导入前的准备工作 在导…...

JavaScript系列(45)--响应式编程实现详解
JavaScript响应式编程实现详解 🔄 今天,让我们深入探讨JavaScript的响应式编程实现。响应式编程是一种基于数据流和变化传播的编程范式,它使我们能够以声明式的方式处理异步数据流。 响应式编程基础概念 🌟 💡 小知识…...

Lustre Core 语法 - 布尔表达式
Lustre v6 中的 Lustre Core 部分支持的表达式种类中,支持布尔表达式。相关的表达式包括and, or, xor, not, #, nor。 相应的文法定义为 Expression :: not Expression| Expression and Expression| Expression or Expression | Expression xor Expression | # (…...
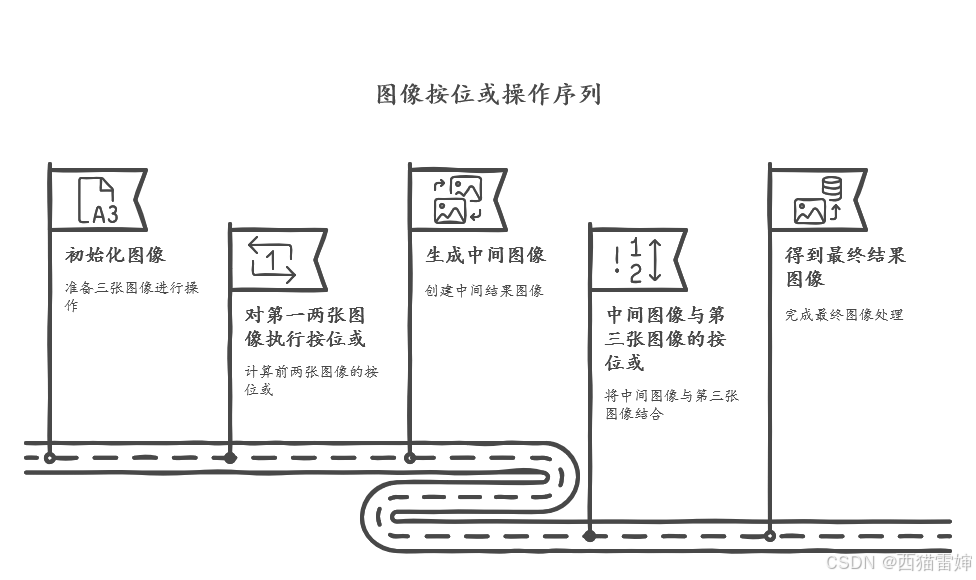
python学opencv|读取图像(四十六)使用cv2.bitwise_or()函数实现图像按位或运算
【0】基础定义 按位与运算:全1取1,其余取0。按位或运算:全0取0,其余取1。 【1】引言 前序学习进程中,已经对图像按位与计算进行了详细探究,相关文章链接如下: python学opencv|读取图像&…...
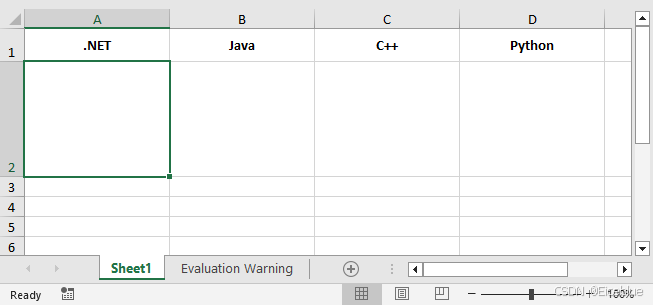
C# 添加、替换、提取、或删除Excel中的图片
在Excel中插入与数据相关的图片,能将关键数据或信息以更直观的方式呈现出来,使文档更加美观。此外,对于已有图片,你有事可能需要更新图片以确保信息的准确性,或者将Excel 中的图片单独保存,用于资料归档、备…...

工作总结:压测篇
前言 压测是测试需要会的一项技能,作为开发,有点时候也要会一点压测。也是被逼着现学现卖的。 一、压测是什么,以及压测工具的选择 压测,即压力测试,是一种性能测试手段,通过模拟大量用户同时访问系统&am…...

11JavaWeb——SpringBootWeb案例02
前面我们已经实现了员工信息的条件分页查询以及删除操作。 关于员工管理的功能,还有两个需要实现: 新增员工 修改员工 首先我们先完成"新增员工"的功能开发,再完成"修改员工"的功能开发。而在"新增员工"中…...
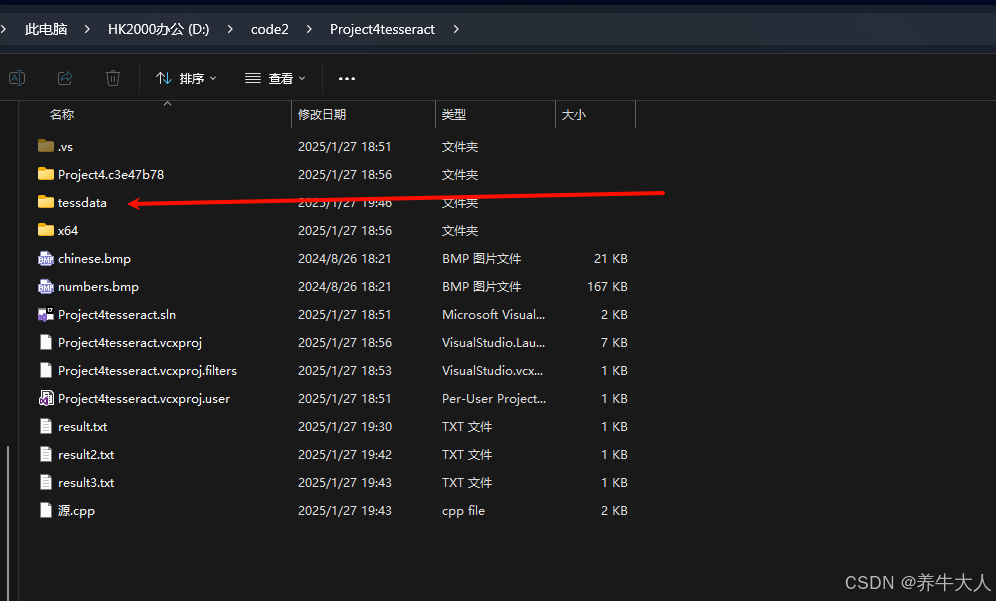
vs2022+tesseract ocr识别中英文 编译好的库下载
测试图片 效果 编译其实挺麻烦的,可参考:在Windows上用Visual Studio编译Tesseract_windows编译tesseract-CSDN博客 #include "baseapi.h" #include "allheaders.h" #include <iostream> #include <fstream> // 用于文…...
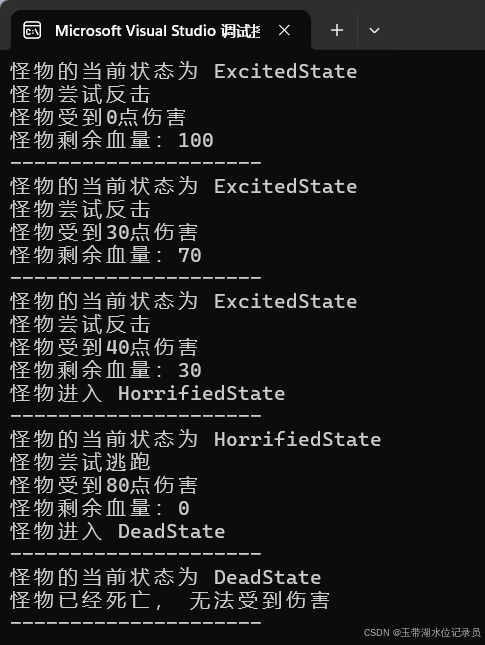
状态模式——C++实现
目录 1. 状态模式简介 2. 代码示例 3. 单例状态对象 4. 状态模式与策略模式的辨析 1. 状态模式简介 状态模式是一种行为型模式。 状态模式的定义:状态模式允许对象在内部状态改变时改变它的行为,对象看起来好像修改了它的类。 通俗的说就是一个对象…...

3.观察者模式(Observer)
组件协作模式 现代软件专业分工之后的第一个结果是 “框架与应用程序的划分”,“组件协作” 模式通过晚期绑定,来实现框架与应用程序直接的松耦合,是二者之间协作时常用的模式 典型模式 Template Method Strategy Observer /Event 动机(M…...

Kotlin判空辅助工具
1)?.操作符 //执行逻辑 if (person ! null) {person.doSomething() } //表达式 person?.doSomething() 2)?:操作符 //执行逻辑 val c if (a ! null) {a } else {b } //表达式 val c a ?: b 3)!!表达式 var message: String? &qu…...
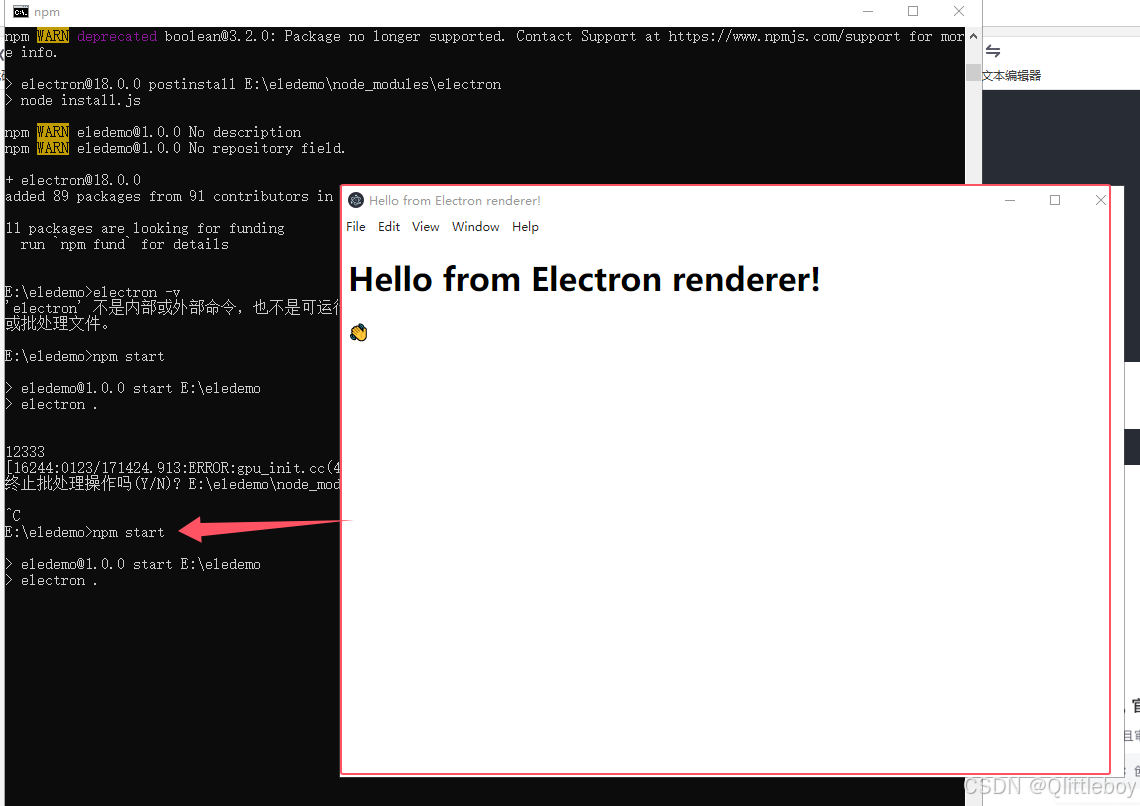
Electron学习笔记,安装环境(1)
1、支持win7的Electron 的版本是18,这里node.js用的是14版本(node-v14.21.3-x86.msi)云盘有安装包 Electron 18.x (截至2023年仍在维护中): Chromium: 96 Node.js: 14.17.0 2、安装node环境,node-v14.21.3-x86.msi双击运行选择安…...