LitGPT - 20多个高性能LLM,具有预训练、微调和大规模部署的recipes
文章目录
- 一、关于 LitGPT
- 二、快速启动
- 安装LitGPT
- 高级安装选项
- 从20多个LLM中进行选择
- 三、工作流程
- 1、所有工作流程
- 2、微调LLM
- 3、部署LLM
- 4、评估LLM
- 5、测试LLM
- 6、预训练LLM
- 7、继续预训练LLM
- 四、最先进的功能
- 五、训练方法
- 示例
- 六、项目亮点
- 教程
一、关于 LitGPT
LitGPT 用于 使用、微调、预训练和部署LLM Lightning快速⚡⚡
每个LLM都是从头开始实现的,没有抽象和完全控制,使它们在企业规模上非常快速、最小化和高性能。
- github : https://github.com/Lightning-AI/litgpt
- 快速启动•模型•Finetune•部署•所有工作流程•功能•配方(YAML)•闪电AI•教程
✅**企业就绪-**Apache 2.0可无限企业使用。
✅**开发人员友好-**无需抽象层和单个文件实现即可轻松调试。
✅**优化性能-**旨在最大化性能、降低成本和加快训练速度的模型。
✅**经过验证的配方-**在企业规模测试的高度优化的训练/微调配方。
✅ From scratch implementations ✅ No abstractions ✅ Beginner friendly
✅ Flash attention ✅ FSDP ✅ LoRA, QLoRA, Adapter
✅ Reduce GPU memory (fp4/8/16/32) ✅ 1-1000+ GPUs/TPUs ✅ 20+ LLMs
二、快速启动
安装LitGPT
pip install 'litgpt[all]'
加载和使用20+LLM中的任何一个:
from litgpt import LLMllm = LLM.load("microsoft/phi-2")
text = llm.generate("Fix the spelling: Every fall, the familly goes to the mountains.")
print(text)
# Corrected Sentence: Every fall, the family goes to the mountains.
✅针对快速推理进行了优化
✅量化
✅在低内存GPU上运行
✅没有内部抽象层
✅针对生产规模进行了优化
高级安装选项
从源代码安装:
git clone https://github.com/Lightning-AI/litgpt
cd litgpt
pip install -e '.[all]'
探索完整的Python API文档。
从20多个LLM中进行选择
每个模型都是从头开始编写的,以最大限度地提高性能并删除抽象层:
Model | Model size | Author | Reference |
---|---|---|---|
Llama 3, 3.1, 3.2 | 1B, 3B, 8B, 70B, 405B | Meta AI | Meta AI 2024 |
Code Llama | 7B, 13B, 34B, 70B | Meta AI | Rozière et al. 2023 |
Mixtral MoE | 8x7B, 8x22B | Mistral AI | Mistral AI 2023 |
Mistral | 7B, 123B | Mistral AI | Mistral AI 2023 |
CodeGemma | 7B | Google Team, Google Deepmind | |
Gemma 2 | 2B, 9B, 27B | Google Team, Google Deepmind | |
Phi 3 & 3.5 | 3.8B | Microsoft | Abdin et al. 2024 |
… | … | … | … |
三、工作流程
Finetune•预训练•持续预训练•评估•部署•测试
使用命令行界面运行高级工作流,例如对您自己的数据进行预训练或微调。
1、所有工作流程
安装LitGPT后,选择要运行的模型和工作流程(微调、预训练、评估、部署等…):
# ligpt [action] [model]
litgpt serve meta-llama/Llama-3.2-3B-Instruct
litgpt finetune meta-llama/Llama-3.2-3B-Instruct
litgpt pretrain meta-llama/Llama-3.2-3B-Instruct
litgpt chat meta-llama/Llama-3.2-3B-Instruct
litgpt evaluate meta-llama/Llama-3.2-3B-Instruct
2、微调LLM
Run on Studios : https://lightning.ai/lightning-ai/studios/litgpt-finetune
微调是采用预训练的AI模型并在为特定任务或应用程序量身定制的较小、专门的数据集上进一步训练它的过程。
# 0) setup your dataset
curl -L https://huggingface.co/datasets/ksaw008/finance_alpaca/resolve/main/finance_alpaca.json -o my_custom_dataset.json# 1) Finetune a model (auto downloads weights)
litgpt finetune microsoft/phi-2 \--data JSON \--data.json_path my_custom_dataset.json \--data.val_split_fraction 0.1 \--out_dir out/custom-model# 2) Test the model
litgpt chat out/custom-model/final# 3) Deploy the model
litgpt serve out/custom-model/final
阅读完整的微调文档
3、部署LLM
Deploy on Studios : https://lightning.ai/lightning-ai/studios/litgpt-serve
部署预训练或微调LLM以在实际应用程序中使用它。部署,自动设置可由网站或应用程序访问的Web服务器。
# deploy an out-of-the-box LLM
litgpt serve microsoft/phi-2# deploy your own trained model
litgpt serve path/to/microsoft/phi-2/checkpoint
向查询服务器显示代码:
在单独的终端中测试服务器并将模型API集成到您的AI产品中:
# 3) Use the server (in a separate Python session)
import requests, json
response = requests.post("http://127.0.0.1:8000/predict",json={"prompt": "Fix typos in the following sentence: Exampel input"}
)
print(response.json()["output"])
阅读完整的部署文档。
4、评估LLM
评估一个LLM来测试它在各种任务上的表现,看看它理解和生成文本的程度。简单地说,我们可以评估它在大学水平的化学、编码等方面的表现…(MMLU、真实质量保证等…)
litgpt evaluate microsoft/phi-2 --tasks 'truthfulqa_mc2,mmlu'
阅读完整的评估文档。
5、测试LLM
Run on Studios : <https://lightning.ai/lightning-ai/studios/litgpt-chat)
通过交互式聊天测试模型的工作情况。使用chat
命令聊天、提取嵌入等…
这是一个展示如何使用Phi-2 LLM的示例:
litgpt chat microsoft/phi-2>> Prompt: What do Llamas eat?
完整代码:
# 1) List all supported LLMs
litgpt download list# 2) Use a model (auto downloads weights)
litgpt chat microsoft/phi-2>> Prompt: What do Llamas eat?
某些型号的下载需要额外的访问令牌。您可以在下载文档中信息。
阅读完整的聊天文档。
6、预训练LLM
Run on Studios : https://lightning.ai/lightning-ai/studios/litgpt-pretrain
预训练是在针对特定任务进行微调之前通过将AI模型暴露于大量数据来教授AI模型的过程。
显示代码:
mkdir -p custom_texts
curl https://www.gutenberg.org/cache/epub/24440/pg24440.txt --output custom_texts/book1.txt
curl https://www.gutenberg.org/cache/epub/26393/pg26393.txt --output custom_texts/book2.txt# 1) Download a tokenizer
litgpt download EleutherAI/pythia-160m \--tokenizer_only True# 2) Pretrain the model
litgpt pretrain EleutherAI/pythia-160m \--tokenizer_dir EleutherAI/pythia-160m \--data TextFiles \--data.train_data_path "custom_texts/" \--train.max_tokens 10_000_000 \--out_dir out/custom-model# 3) Test the model
litgpt chat out/custom-model/final
阅读完整的预训练文档
7、继续预训练LLM
Run on Studios : <https://lightning.ai/lightning-ai/studios/litgpt-continue-pretraining)
继续预训练是另一种微调方式,它通过对自定义数据进行训练来专门化已经预训练的模型:
显示代码:
mkdir -p custom_texts
curl https://www.gutenberg.org/cache/epub/24440/pg24440.txt --output custom_texts/book1.txt
curl https://www.gutenberg.org/cache/epub/26393/pg26393.txt --output custom_texts/book2.txt# 1) Continue pretraining a model (auto downloads weights)
litgpt pretrain EleutherAI/pythia-160m \--tokenizer_dir EleutherAI/pythia-160m \--initial_checkpoint_dir EleutherAI/pythia-160m \--data TextFiles \--data.train_data_path "custom_texts/" \--train.max_tokens 10_000_000 \--out_dir out/custom-model# 2) Test the model
litgpt chat out/custom-model/final
阅读完整的持续预训练文档
四、最先进的功能
✅最先进的优化:闪存注意力v2、通过完全分片数据并行支持多GPU、可选CPU卸载以及TPU和XLA支持。
✅预训练、微调和部署
✅通过低精度设置降低计算要求:FP16、BF16和FP16/FP32混合。
✅通过量化降低内存需求:4位浮点数、8位整数和双重量化。
✅配置文件具有出色的开箱即用性能。
✅参数高效微调:LoRA、QLoRA、Adapter和Adapter v2。
✅导出到其他流行的模型重量格式。
✅许多流行的数据集用于预训练和微调,并支持自定义数据集。
✅可读且易于修改的代码,以试验最新的研究思想。
五、训练方法
LitGPT带有经过验证的配方(YAML配置)来训练不同条件下的模型。我们根据我们发现的在不同训练条件下表现最佳的参数生成了这些食谱。
浏览所有训练食谱在这里。
示例
litgpt finetune \--config https://raw.githubusercontent.com/Lightning-AI/litgpt/main/config_hub/finetune/llama-2-7b/lora.yaml
✅使用配置自定义训练
配置可让您自定义所有粒度参数的训练,例如:
# The path to the base model's checkpoint directory to load for finetuning. (type: <class 'Path'>, default: checkpoints/stabilityai/stablelm-base-alpha-3b)
checkpoint_dir: checkpoints/meta-llama/Llama-2-7b-hf# Directory in which to save checkpoints and logs. (type: <class 'Path'>, default: out/lora)
out_dir: out/finetune/qlora-llama2-7b# The precision to use for finetuning. Possible choices: "bf16-true", "bf16-mixed", "32-true". (type: Optional[str], default: null)
precision: bf16-true...
✅示例:LoRA微调配置
# The path to the base model's checkpoint directory to load for finetuning. (type: <class 'Path'>, default: checkpoints/stabilityai/stablelm-base-alpha-3b)
checkpoint_dir: checkpoints/meta-llama/Llama-2-7b-hf# Directory in which to save checkpoints and logs. (type: <class 'Path'>, default: out/lora)
out_dir: out/finetune/qlora-llama2-7b# The precision to use for finetuning. Possible choices: "bf16-true", "bf16-mixed", "32-true". (type: Optional[str], default: null)
precision: bf16-true# If set, quantize the model with this algorithm. See ``tutorials/quantize.md`` for more information. (type: Optional[Literal['nf4', 'nf4-dq', 'fp4', 'fp4-dq', 'int8-training']], default: null)
quantize: bnb.nf4# How many devices/GPUs to use. (type: Union[int, str], default: 1)
devices: 1# How many nodes to use. (type: int, default: 1)
num_nodes: 1# The LoRA rank. (type: int, default: 8)
lora_r: 32# The LoRA alpha. (type: int, default: 16)
lora_alpha: 16# The LoRA dropout value. (type: float, default: 0.05)
lora_dropout: 0.05# Whether to apply LoRA to the query weights in attention. (type: bool, default: True)
lora_query: true# Whether to apply LoRA to the key weights in attention. (type: bool, default: False)
lora_key: false# Whether to apply LoRA to the value weights in attention. (type: bool, default: True)
lora_value: true# Whether to apply LoRA to the output projection in the attention block. (type: bool, default: False)
lora_projection: false# Whether to apply LoRA to the weights of the MLP in the attention block. (type: bool, default: False)
lora_mlp: false# Whether to apply LoRA to output head in GPT. (type: bool, default: False)
lora_head: false# Data-related arguments. If not provided, the default is ``litgpt.data.Alpaca``.
data:class_path: litgpt.data.Alpaca2kinit_args:mask_prompt: falseval_split_fraction: 0.05prompt_style: alpacaignore_index: -100seed: 42num_workers: 4download_dir: data/alpaca2k# Training-related arguments. See ``litgpt.args.TrainArgs`` for details
train:# Number of optimizer steps between saving checkpoints (type: Optional[int], default: 1000)save_interval: 200# Number of iterations between logging calls (type: int, default: 1)log_interval: 1# Number of samples between optimizer steps across data-parallel ranks (type: int, default: 128)global_batch_size: 8# Number of samples per data-parallel rank (type: int, default: 4)micro_batch_size: 2# Number of iterations with learning rate warmup active (type: int, default: 100)lr_warmup_steps: 10# Number of epochs to train on (type: Optional[int], default: 5)epochs: 4# Total number of tokens to train on (type: Optional[int], default: null)max_tokens:# Limits the number of optimizer steps to run (type: Optional[int], default: null)max_steps:# Limits the length of samples (type: Optional[int], default: null)max_seq_length: 512# Whether to tie the embedding weights with the language modeling head weights (type: Optional[bool], default: null)tie_embeddings:# (type: float, default: 0.0003)learning_rate: 0.0002# (type: float, default: 0.02)weight_decay: 0.0# (type: float, default: 0.9)beta1: 0.9# (type: float, default: 0.95)beta2: 0.95# (type: Optional[float], default: null)max_norm:# (type: float, default: 6e-05)min_lr: 6.0e-05# Evaluation-related arguments. See ``litgpt.args.EvalArgs`` for details
eval:# Number of optimizer steps between evaluation calls (type: int, default: 100)interval: 100# Number of tokens to generate (type: Optional[int], default: 100)max_new_tokens: 100# Number of iterations (type: int, default: 100)max_iters: 100# The name of the logger to send metrics to. (type: Literal['wandb', 'tensorboard', 'csv'], default: csv)
logger_name: csv# The random seed to use for reproducibility. (type: int, default: 1337)
seed: 1337
✅覆盖CLI中的任何参数:
litgpt finetune \--config https://raw.githubusercontent.com/Lightning-AI/litgpt/main/config_hub/finetune/llama-2-7b/lora.yaml \--lora_r 4
六、项目亮点
LitGPT为许多伟大的AI项目、计划、挑战,当然还有企业提供支持。请提交拉取请求以考虑某个功能。
- 📊SAMBA:用于高效无限上下文语言建模的简单混合状态空间模型
微软研究人员的Samba项目建立在LitGPT代码库之上,将状态空间模型与滑动窗口注意力相结合,优于纯状态空间模型。 - 🏆NeurIPS 2023大型语言模型效率挑战:1个LLM+1个GPU+1天
LitGPT存储库是NeurIPS 2023 LLM效率挑战赛的官方入门套件,该比赛的重点是在单个GPU上微调现有的非指令调整LLM 24小时。 - 🦙TinyLlama:一个开源的小语言模型
LitGPT支持TinyLlama项目和TinyLlama:开源小语言模型研究论文。 - 🍪MicroLlama:MicroLlama-300M
MicroLlama是在TinyLlama和LitGPT支持的50Btoken 上预训练的300M骆驼模型。 - 🔬预训练较少token 的小型基本LM
研究论文“预训练具有更少令牌的小型基本LM”利用LitGPT,通过从较大模型继承一些转换器块并对较大模型使用的一小部分数据进行训练来开发较小的基本语言模型。它证明,尽管使用的训练数据和资源明显较少,但这些较小的模型可以与较大的模型相比。
教程
🚀开始
⚡微调,包括LoRA、QLoRA和适配器
🤖预训练
💬模型评估
📘支持和自定义数据集
🧹量化
🤯处理内存不足(OOM)错误的提示
🧑🏽💻使用云TPU
2025-01-27(一)
相关文章:

LitGPT - 20多个高性能LLM,具有预训练、微调和大规模部署的recipes
文章目录 一、关于 LitGPT二、快速启动安装LitGPT高级安装选项 从20多个LLM中进行选择 三、工作流程1、所有工作流程2、微调LLM3、部署LLM4、评估LLM5、测试LLM6、预训练LLM7、继续预训练LLM 四、最先进的功能五、训练方法示例 六、项目亮点教程 一、关于 LitGPT LitGPT 用于 …...
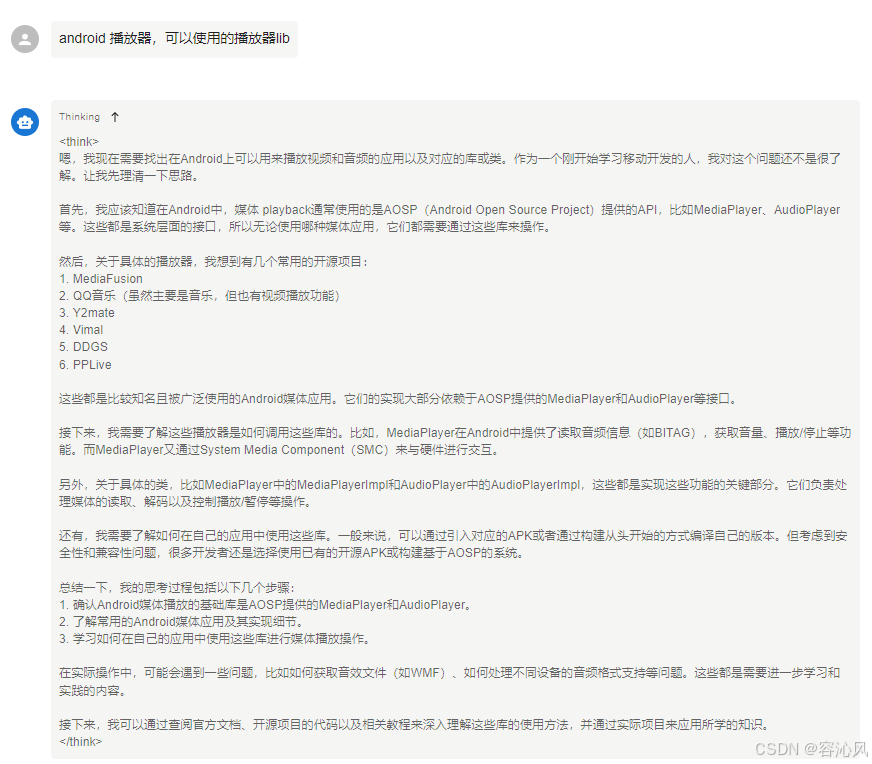
deepseek R1 14b显存占用
RTX2080ti 11G显卡,模型7b速度挺快,试试14B也不错。 7B显存使用5.6G,14B显存刚好够,出文字速度差不多。 打算自己写个移动宽带的IPTV播放器,不知道怎么下手,就先问他了。...

无用知识研究:对std::common_type以及问号表达式类型的理解
先说结论: 如果问号表达式能编译通过,那么std::common_type就能通过。因为common_type的底层依赖的就是?: common_type的实现里,利用了问号表达式:ternary conditional operator (?:) https://stackoverflow.com/questions/14…...
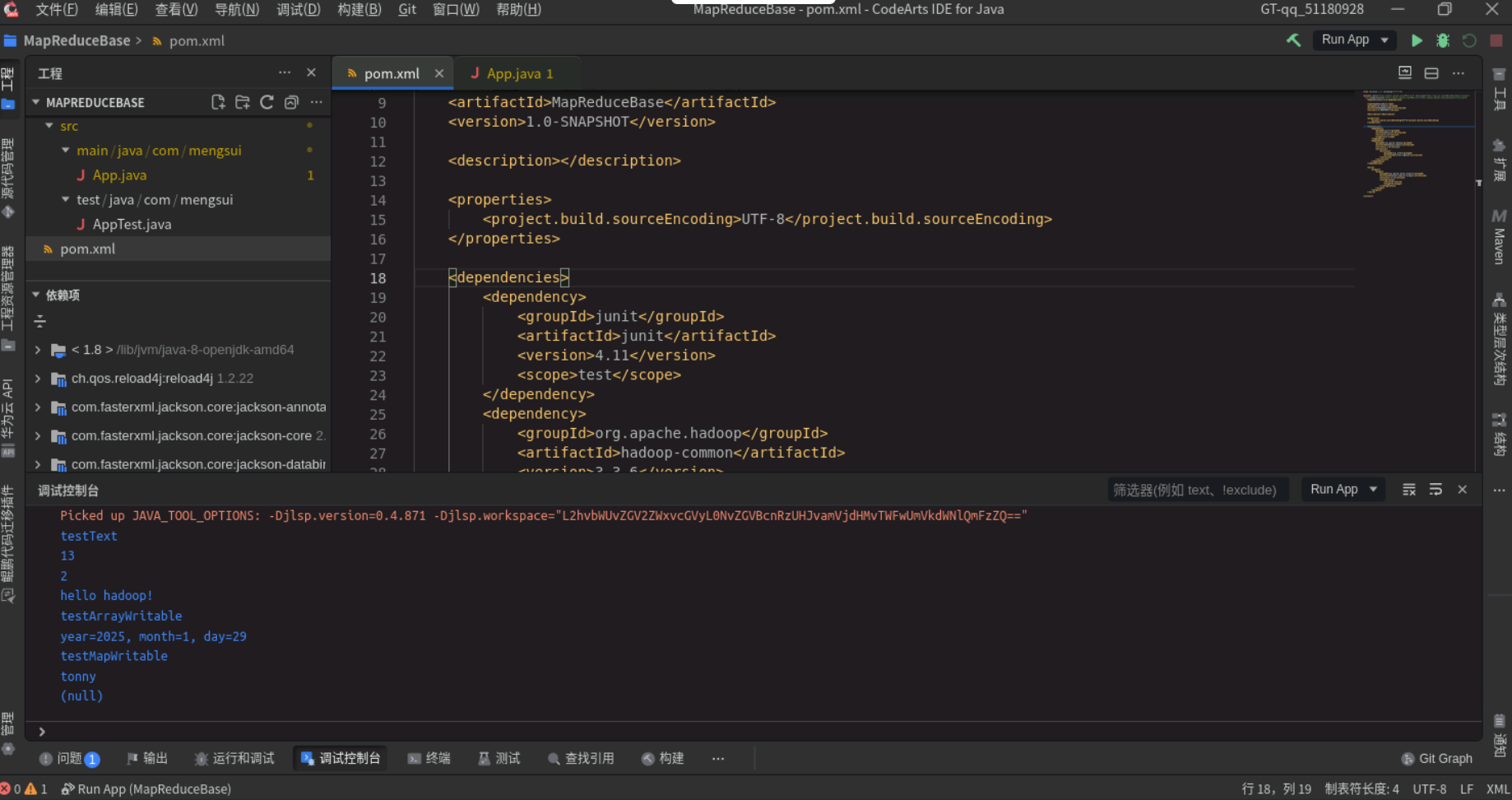
MapReduce概述
目录 1. MapReduce概述2. MapReduce的功能2.1 数据划分和计算任务调度2.2 数据/代码互定位2.3 系统优化2.4 出错检测和恢复 3. MapReduce处理流程4. MapReduce编程基础参考 1. MapReduce概述 MapReduce是面向大数据并行处理的计算模型、框架和平台: 1. 基于集群的高性能并行…...
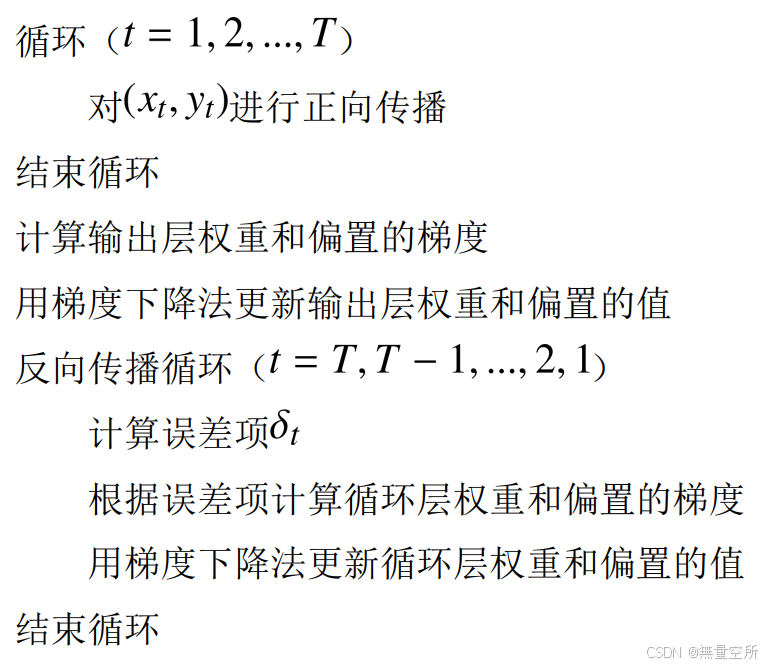
循环神经网络(RNN)+pytorch实现情感分析
目录 一、背景引入 二、网络介绍 2.1 输入层 2.2 循环层 2.3 输出层 2.4 举例 2.5 深层网络 三、网络的训练 3.1 训练过程举例 1)输出层 2)循环层 3.2 BPTT 算法 1)输出层 2)循环层 3)算法流程 四、循…...
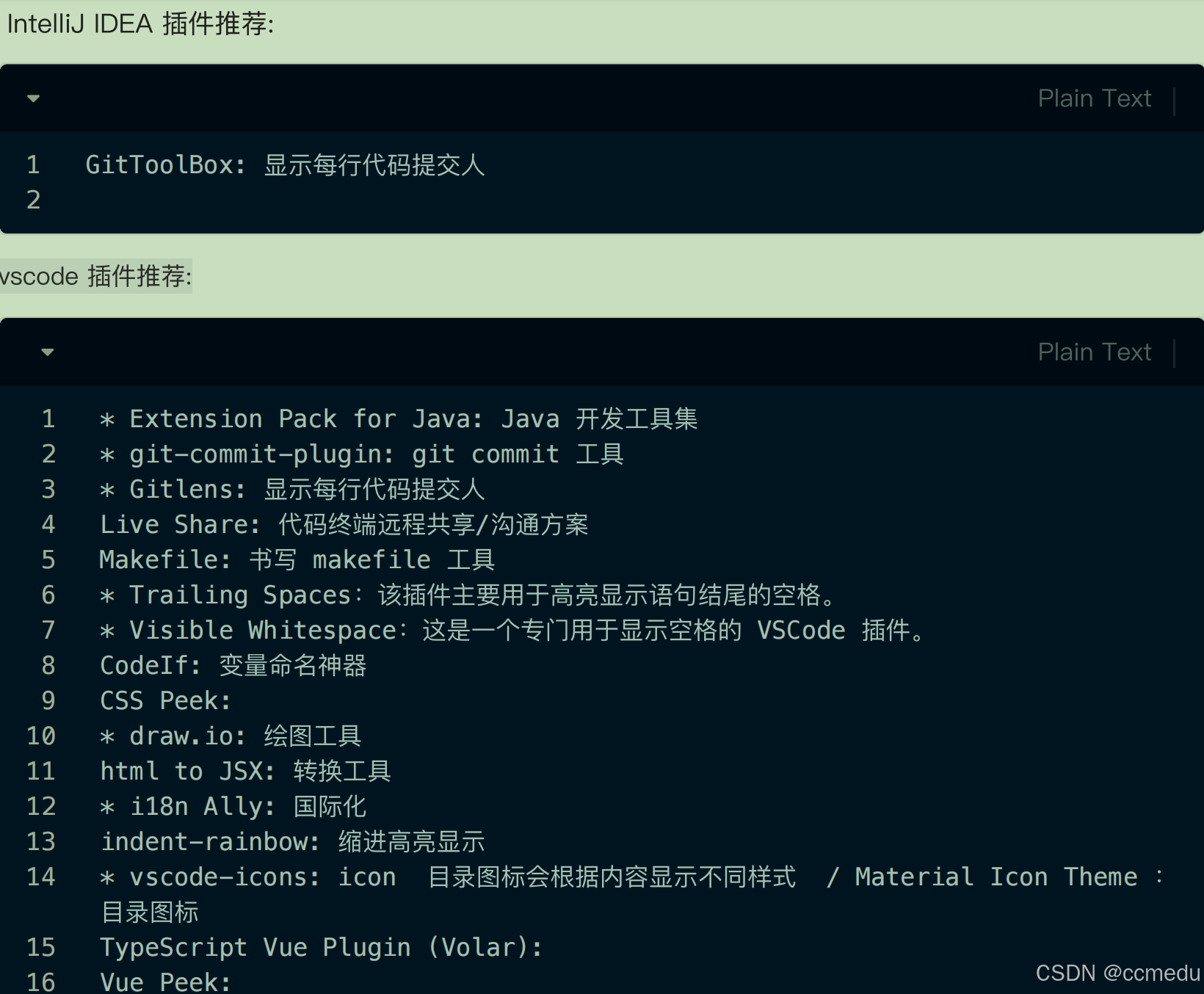
Mac cursor设置jdk、Maven版本
基本配置 – Cursor 使用文档 首先是系统用户级别的设置参数,运行cursor,按下ctrlshiftp,输入Open User Settings(JSON),在弹出的下拉菜单中选中下面这样的: 在打开的json编辑器中追加下面的内容: {"…...
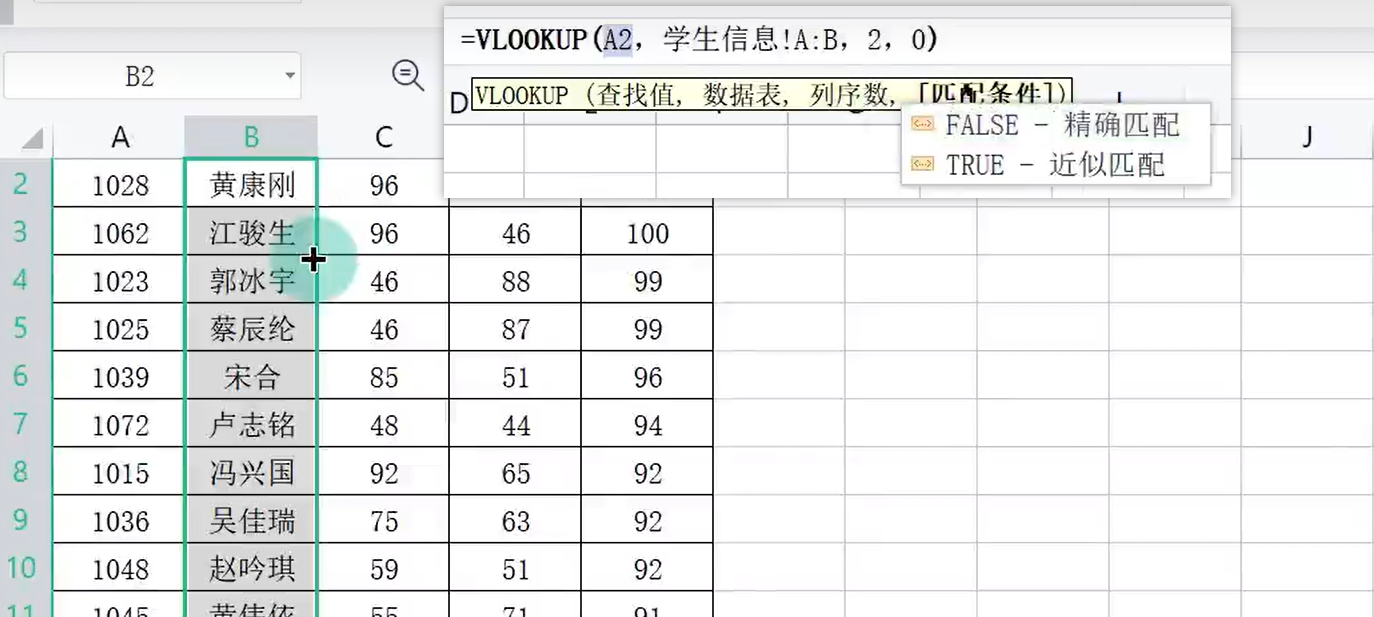
WPS数据分析000005
目录 一、数据录入技巧 二、一维表 三、填充柄 向下自动填充 自动填充选项 日期填充 星期自定义 自定义序列 1-10000序列 四、智能填充 五、数据有效性 出错警告 输入信息 下拉列表 六、记录单 七、导入数据 编辑 八、查找录入 会员功能 Xlookup函数 VL…...

CTF从入门到精通
文章目录 背景知识CTF赛制 背景知识 CTF赛制 1.web安全:通过浏览器访问题目服务器上的网站,寻找网站漏洞(sql注入,xss(钓鱼链接),文件上传,包含漏洞,xxe,ssrf,命令执行,…...
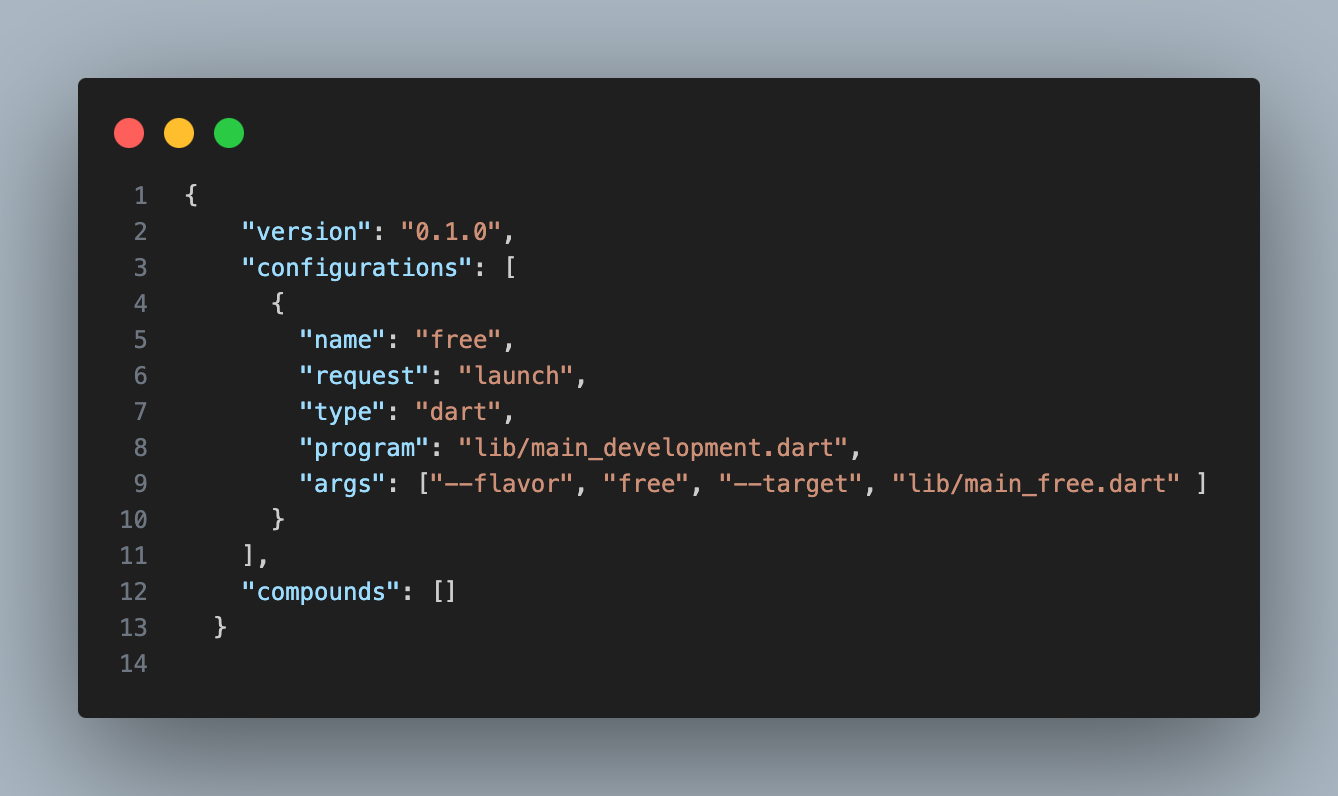
Flutter使用Flavor实现切换环境和多渠道打包
在Android开发中通常我们使用flavor进行多渠道打包,flutter开发中同样有这种方式,不过需要在原生中配置 具体方案其实flutter官网个了相关示例(https://docs.flutter.dev/deployment/flavors),我这里记录一下自己的操作 Android …...

Springboot如何使用面向切面编程AOP?
Springboot如何使用面向切面编程AOP? 在 Spring Boot 中使用面向切面编程(AOP)非常简单,Spring Boot 提供了对 AOP 的自动配置支持。以下是详细的步骤和示例,帮助你快速上手 Spring Boot 中的 AOP。 1. 添加依赖 首先ÿ…...
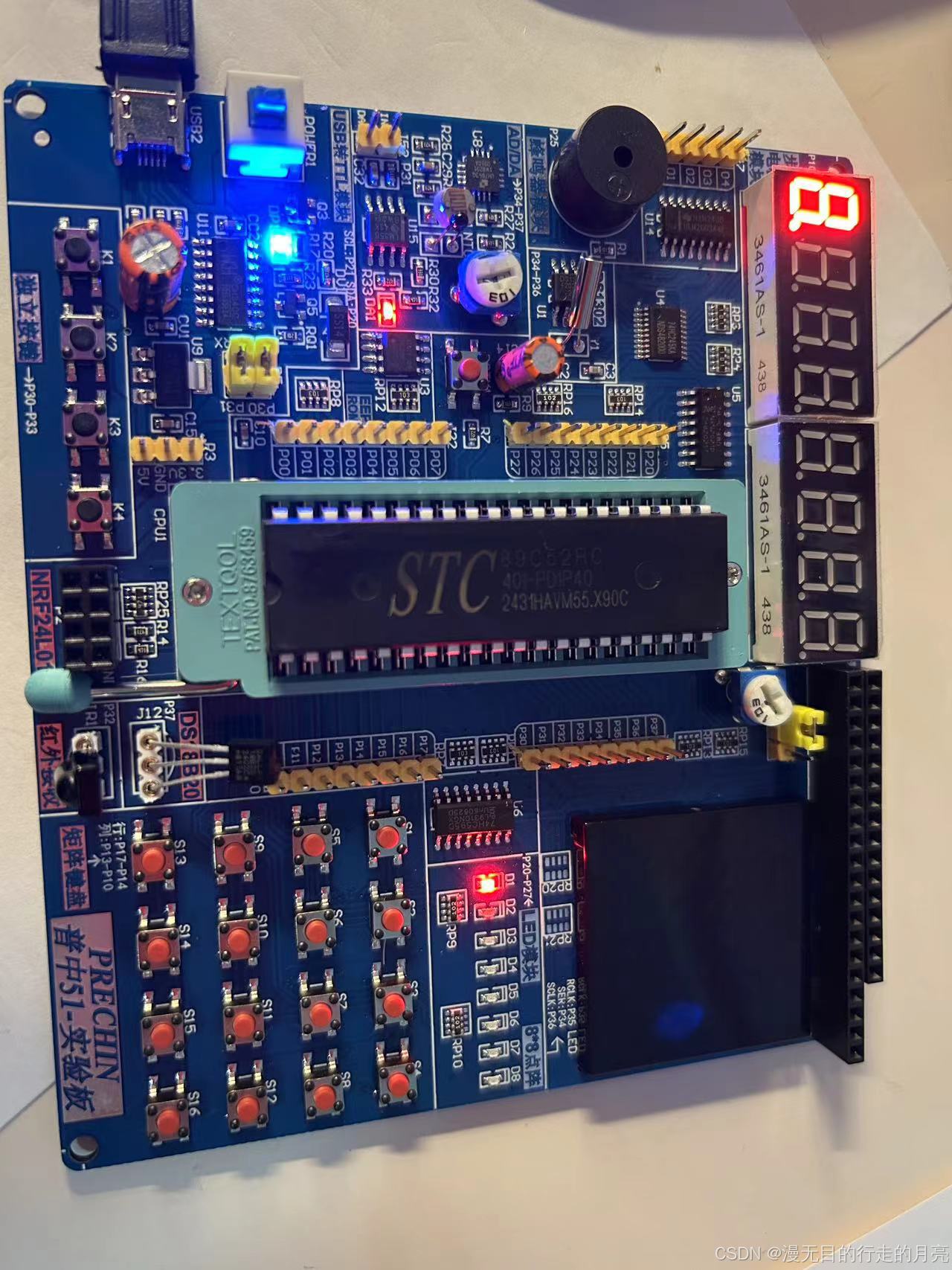
51单片机(STC89C52)开发:点亮一个小灯
软件安装: 安装开发板CH340驱动。 安装KEILC51开发软件:C51V901.exe。 下载软件:PZ-ISP.exe 创建项目: 新建main.c 将main.c加入至项目中: main.c:点亮一个小灯 #include "reg52.h"sbit LED1P2^0; //P2的…...
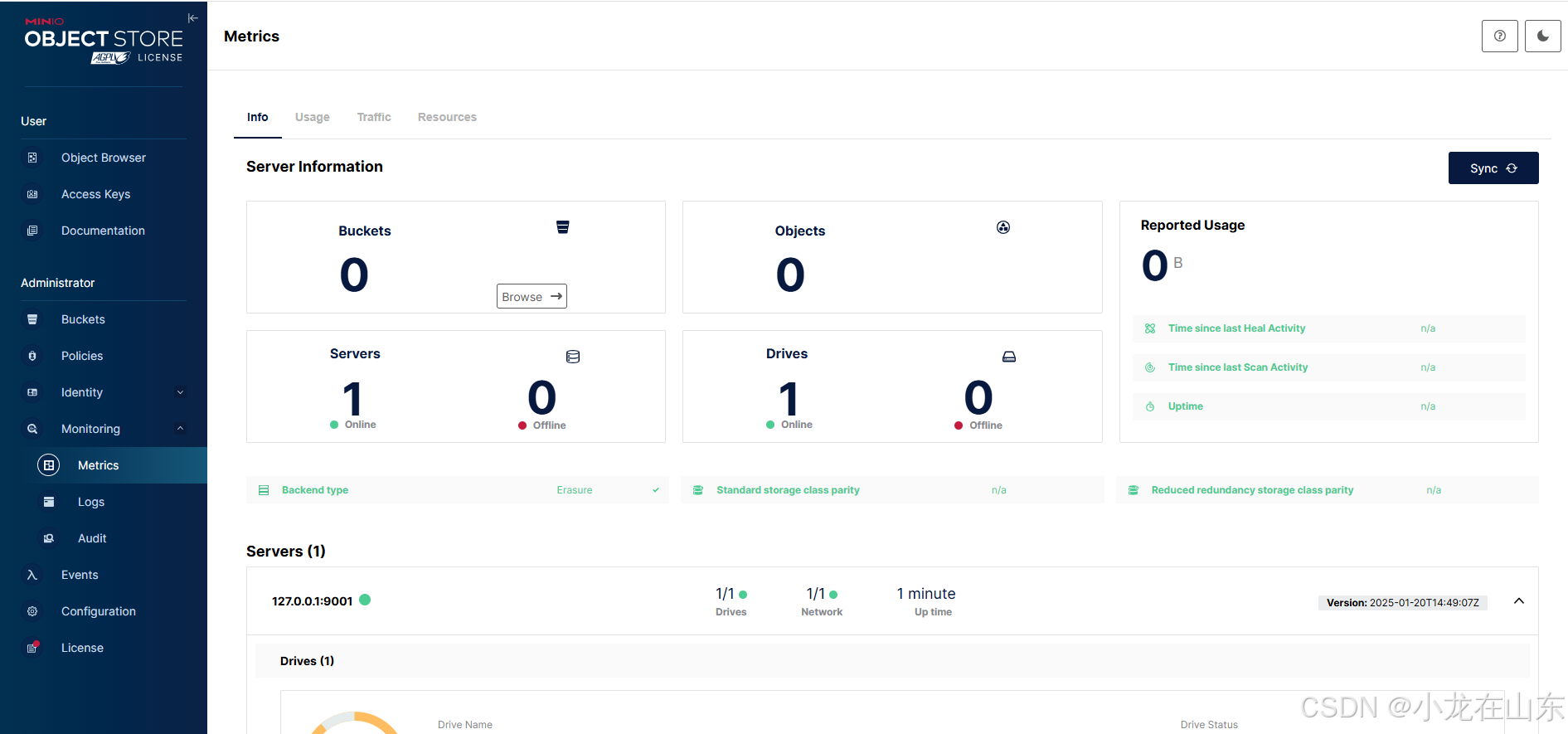
基于MinIO的对象存储增删改查
MinIO是一个高性能的分布式对象存储服务。Python的minio库可操作MinIO,包括创建/列出存储桶、上传/下载/删除文件及列出文件。 查看帮助信息 minio.exe --help minio.exe server --help …...
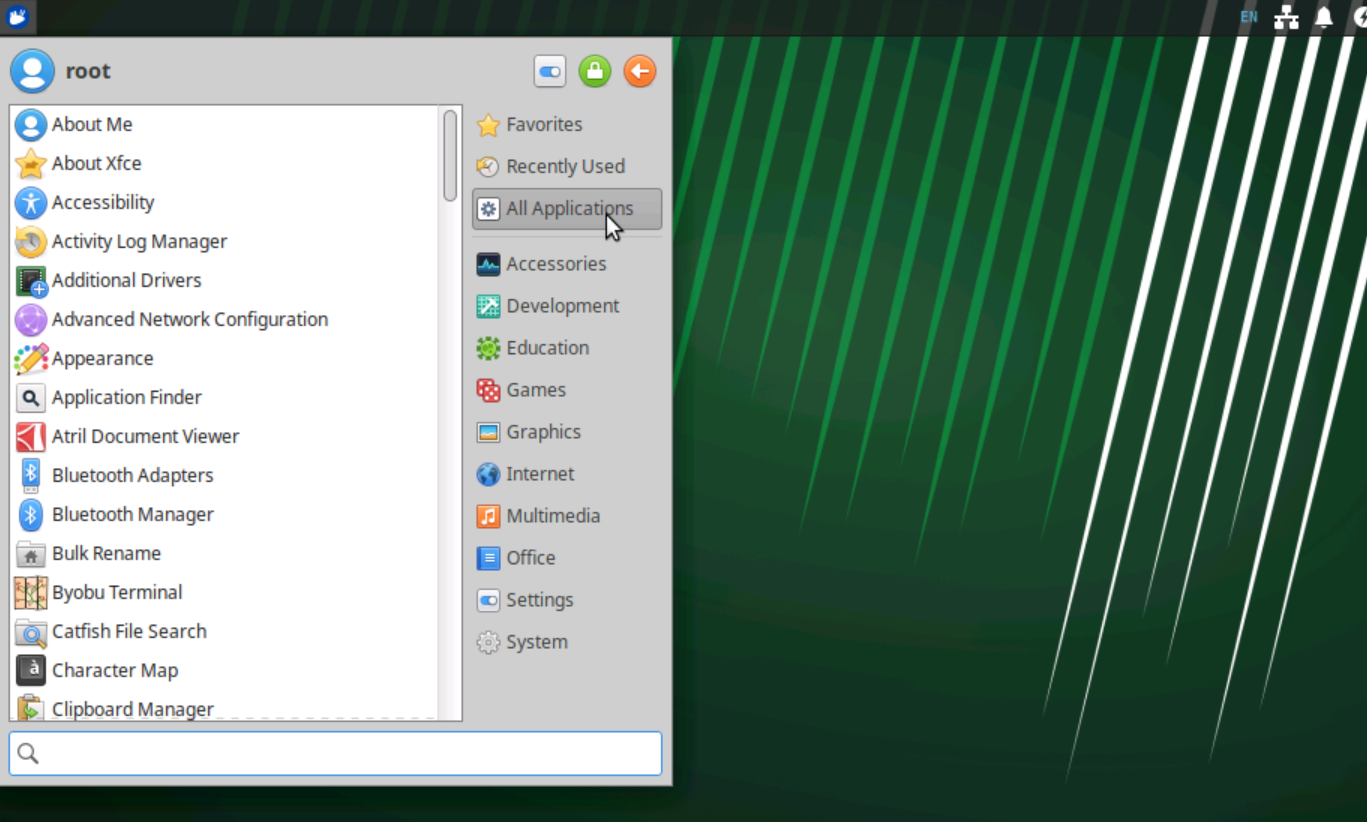
Ubuntu Server 安装 XFCE4桌面
Ubuntu Server没有桌面环境,一些软件有桌面环境使用起来才更加方便,所以我尝试安装桌面环境。常用的桌面环境有:GNOME、KDE Plasma、XFCE4等。这里我选择安装XFCE4桌面环境,主要因为它是一个极轻量级的桌面环境,适合内…...

MySQL 存储函数:数据库的自定义函数
在数据库开发中,存储函数(Stored Function)是一种非常有用的工具。它允许我们创建自定义的函数,这些函数可以在 SQL 查询中像内置函数一样使用,用于实现特定的逻辑和计算。本文将深入探讨 MySQL 存储函数的概念、与存储…...
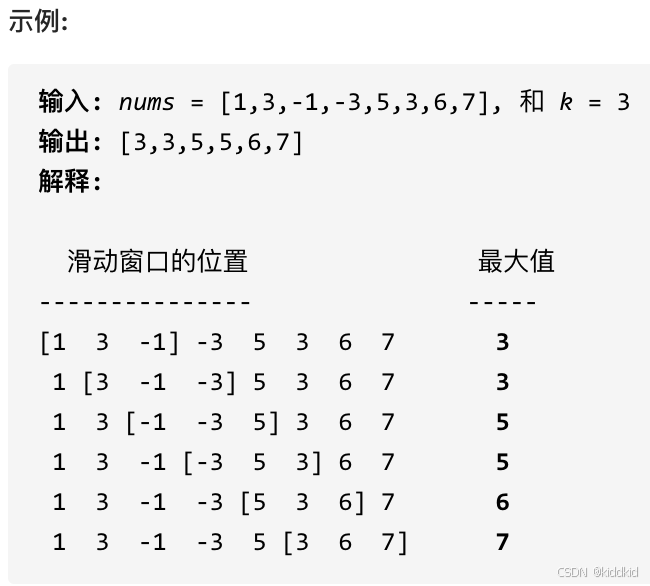
代码随想录_栈与队列
栈与队列 232.用栈实现队列 232. 用栈实现队列 使用栈实现队列的下列操作: push(x) – 将一个元素放入队列的尾部。 pop() – 从队列首部移除元素。 peek() – 返回队列首部的元素。 empty() – 返回队列是否为空。 思路: 定义两个栈: 入队栈, 出队栈, 控制出入…...

【微服务与分布式实践】探索 Sentinel
参数设置 熔断时长 、最小请求数、最大RT ms、比例阈值、异常数 熔断策略 慢调⽤⽐例 当单位统计时⻓内请求数⽬⼤于设置的最⼩请求数⽬,并且慢调⽤的⽐例⼤于阈值,则接下来的熔断时⻓内请求会⾃动被熔断 异常⽐例 当单位统计时⻓内请求数⽬⼤于设置…...
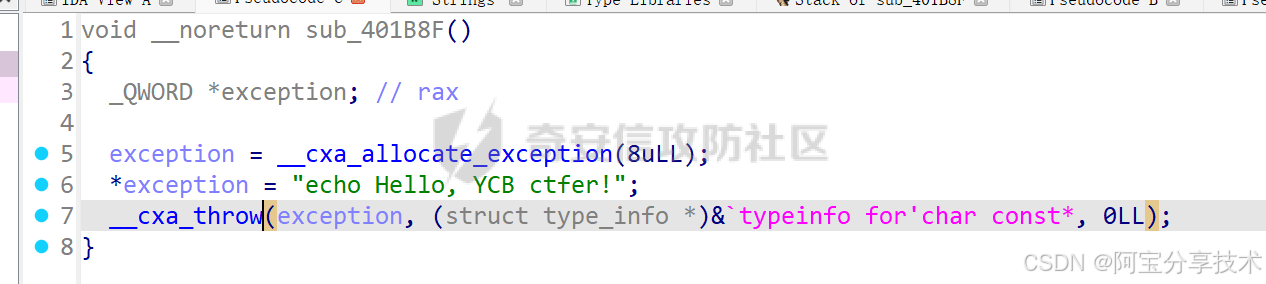
深入研究异常处理机制
一、原理探究 C异常处理 本节内容针对 Linux 下的 C 异常处理机制,重点在于研究如何在异常处理流程中利用溢出漏洞,所以不对异常处理及 unwind 的过程做详细分析,只做简单介绍 异常机制中主要的三个关键字:throw 抛出异常&#x…...
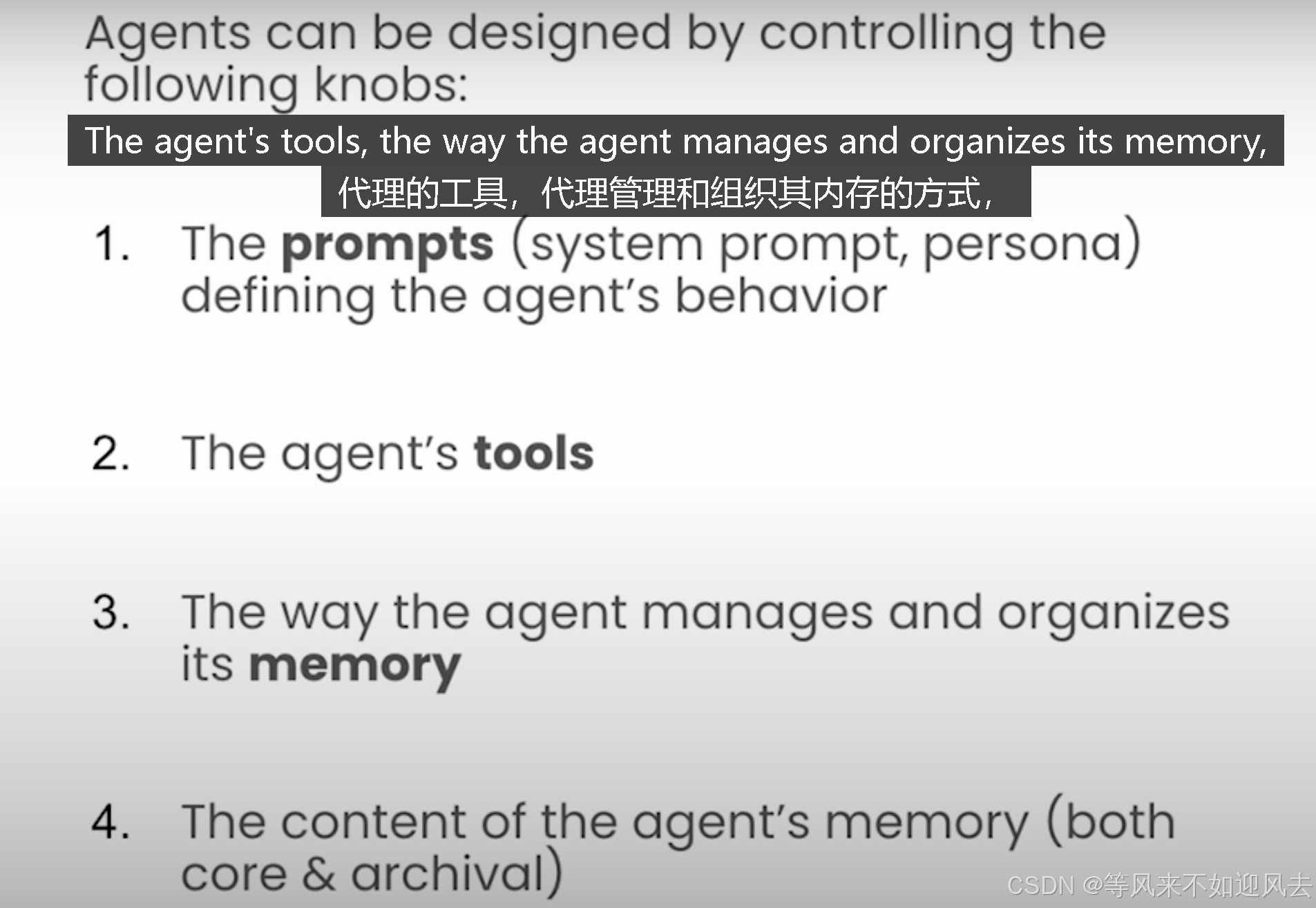
【memgpt】letta 课程4:基于latta框架构建MemGpt代理并与之交互
Lab 3: Building Agents with memory 基于latta框架构建MemGpt代理并与之交互理解代理状态,例如作为系统提示符、工具和agent的内存查看和编辑代理存档内存MemGPT 代理是有状态的 agents的设计思路 每个步骤都要定义代理行为 Letta agents persist information over time and…...
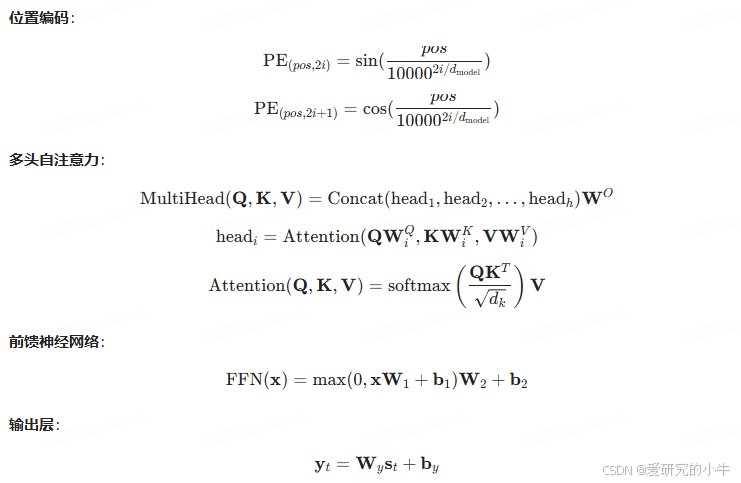
讯飞智作 AI 配音技术浅析(二):深度学习与神经网络
讯飞智作 AI 配音技术依赖于深度学习与神经网络,特别是 Tacotron、WaveNet 和 Transformer-TTS 模型。这些模型通过复杂的神经网络架构和数学公式,实现了从文本到自然语音的高效转换。 一、Tacotron 模型 Tacotron 是一种端到端的语音合成模型ÿ…...
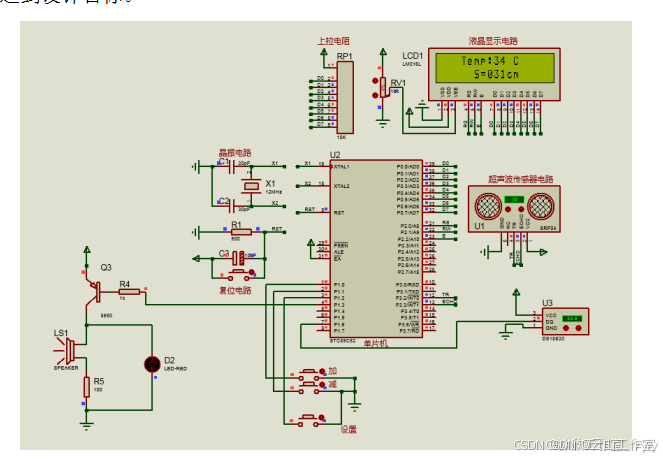
基于单片机的超声波液位检测系统(论文+源码)
1总体设计 本课题为基于单片机的超声波液位检测系统的设计,系统的结构框图如图2.1所示。其中包括了按键模块,温度检测模块,超声波液位检测模块,显示模块,蜂鸣器等器件设备。其中,采用STC89C52单片机作为主控…...

Autogen_core: test_code_executor.py
目录 代码代码解释 代码 import textwrapimport pytest from autogen_core.code_executor import (Alias,FunctionWithRequirements,FunctionWithRequirementsStr,ImportFromModule, ) from autogen_core.code_executor._func_with_reqs import build_python_functions_file f…...

从0开始使用面对对象C语言搭建一个基于OLED的图形显示框架
目录 前言 环境介绍 代码与动机 架构设计,优缺点 博客系列指引 前言 笔者前段时间花费了一周,整理了一下自从TM1637开始打算的,使用OLED来搭建一个通用的显示库的一个工程。笔者的OLED库已经开源到Github上了,地址在…...
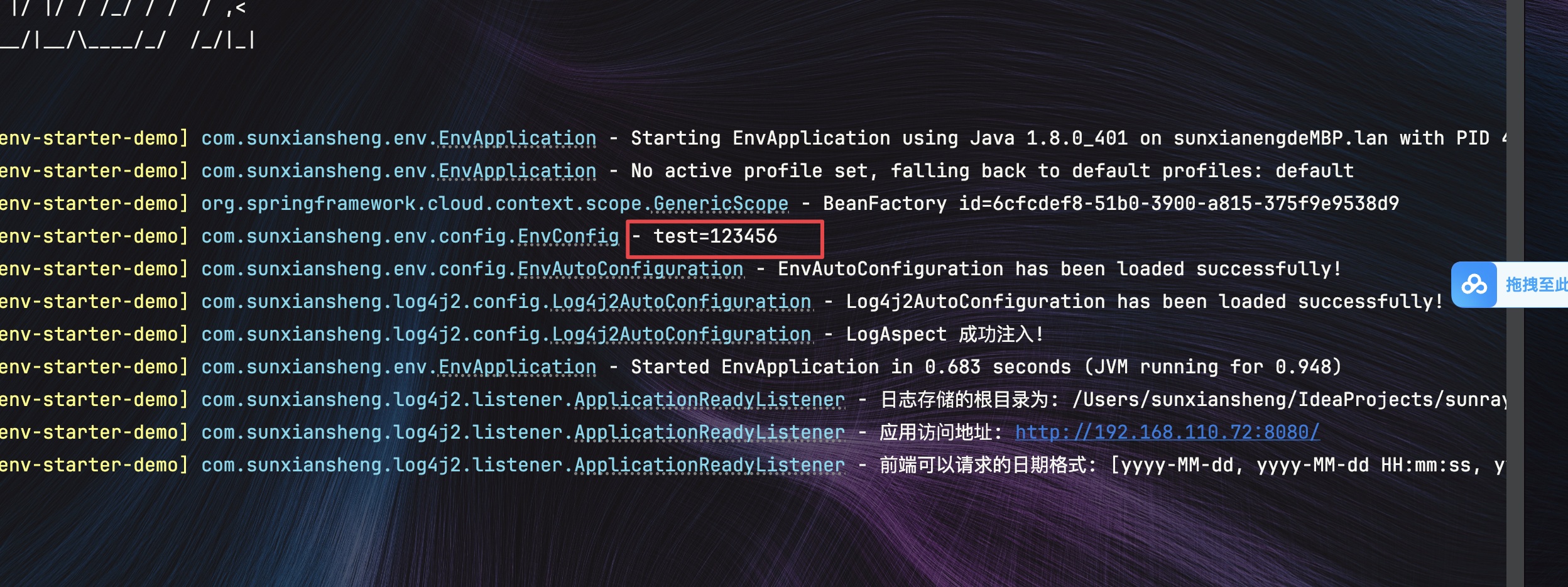
Java实现.env文件读取敏感数据
文章目录 1.common-env-starter模块1.目录结构2.DotenvEnvironmentPostProcessor.java 在${xxx}解析之前执行,提前读取配置3.EnvProperties.java 这里的path只是为了代码提示4.EnvAutoConfiguration.java Env模块自动配置类5.spring.factories 自动配置和注册Enviro…...

Go反射指南
概念: 官方对此有个非常简明的介绍,两句话耐人寻味: 反射提供一种让程序检查自身结构的能力反射是困惑的源泉 第1条,再精确点的描述是“反射是一种检查interface变量的底层类型和值的机制”。 第2条,很有喜感的自嘲…...
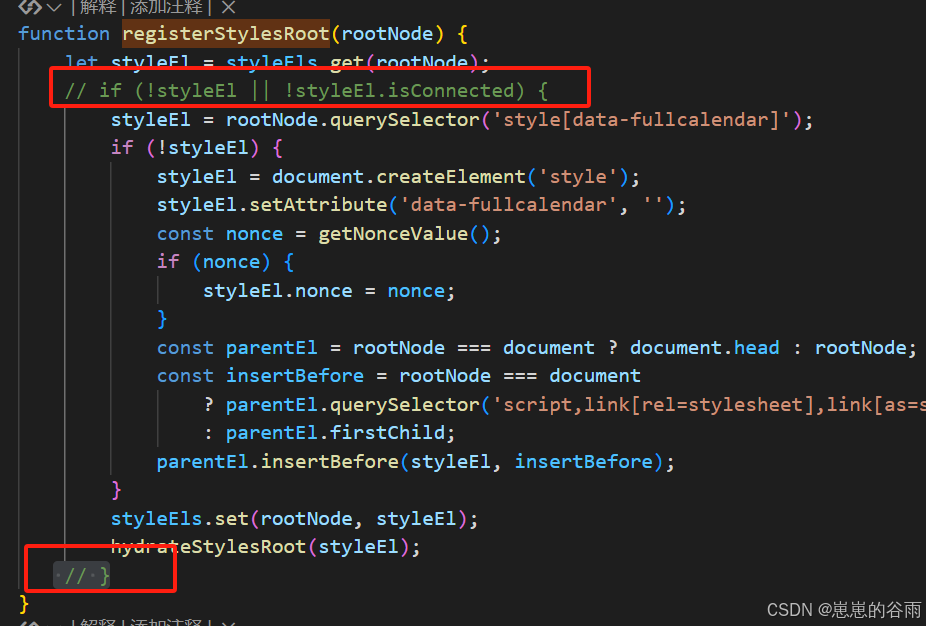
Fullcalendar @fullcalendar/react 样式错乱丢失问题和导致页面卡顿崩溃问题
问题描述: 我使用 fullcalendar的react版本时,出现了一个诡异的问题,当我切换到 一个iframe页面时(整个页面是一个iframe嵌入的),再切换回来日历的样式丢失了!不仅丢失了样式还导致页面崩溃了&…...
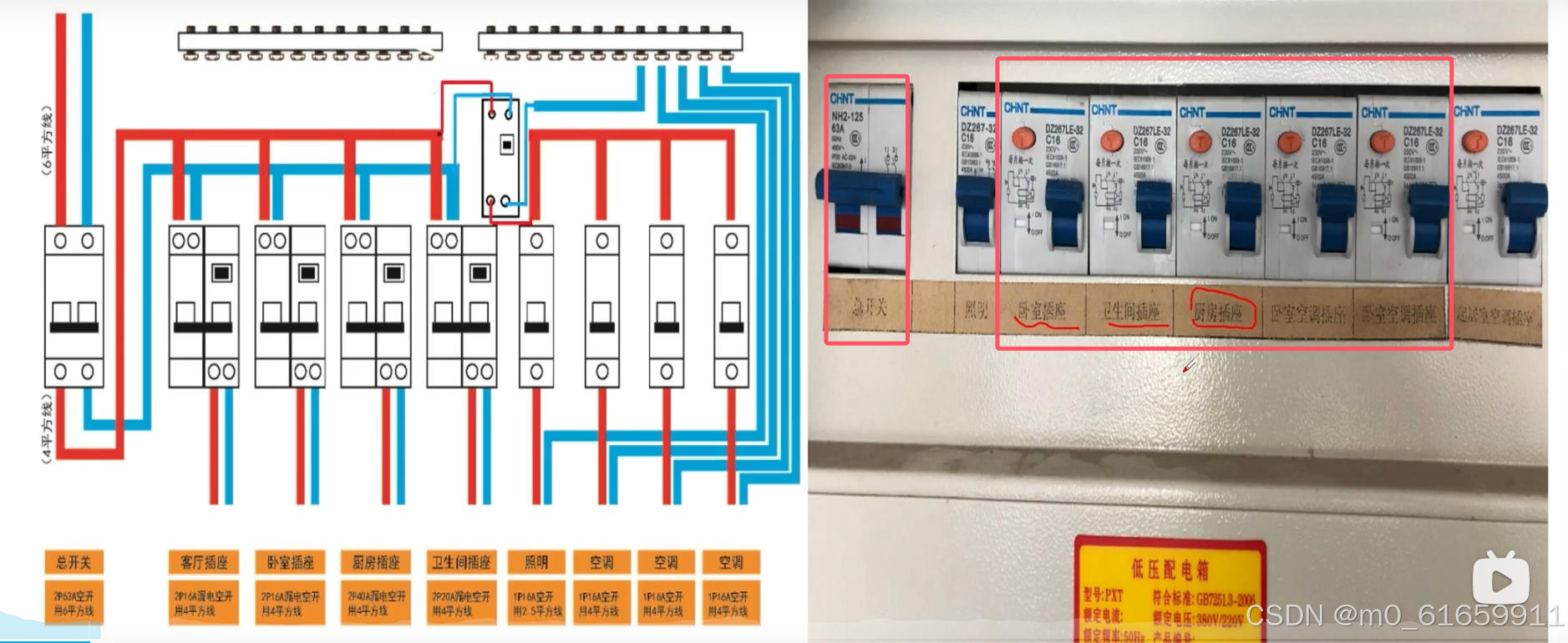
【电工基础】4.低压电器元件,漏电保护器,熔断器,中间继电器
一。漏电保护器 1.使用区域 我们在家用总开关上使用空气开关(断路器),其余的厨房卧室为漏电保护器。 2.漏电保护器的简介 1.漏电:就是流入的电流和流出的电流不等,意味着电路回路中还有其它分支,可能是电流通过人体进…...
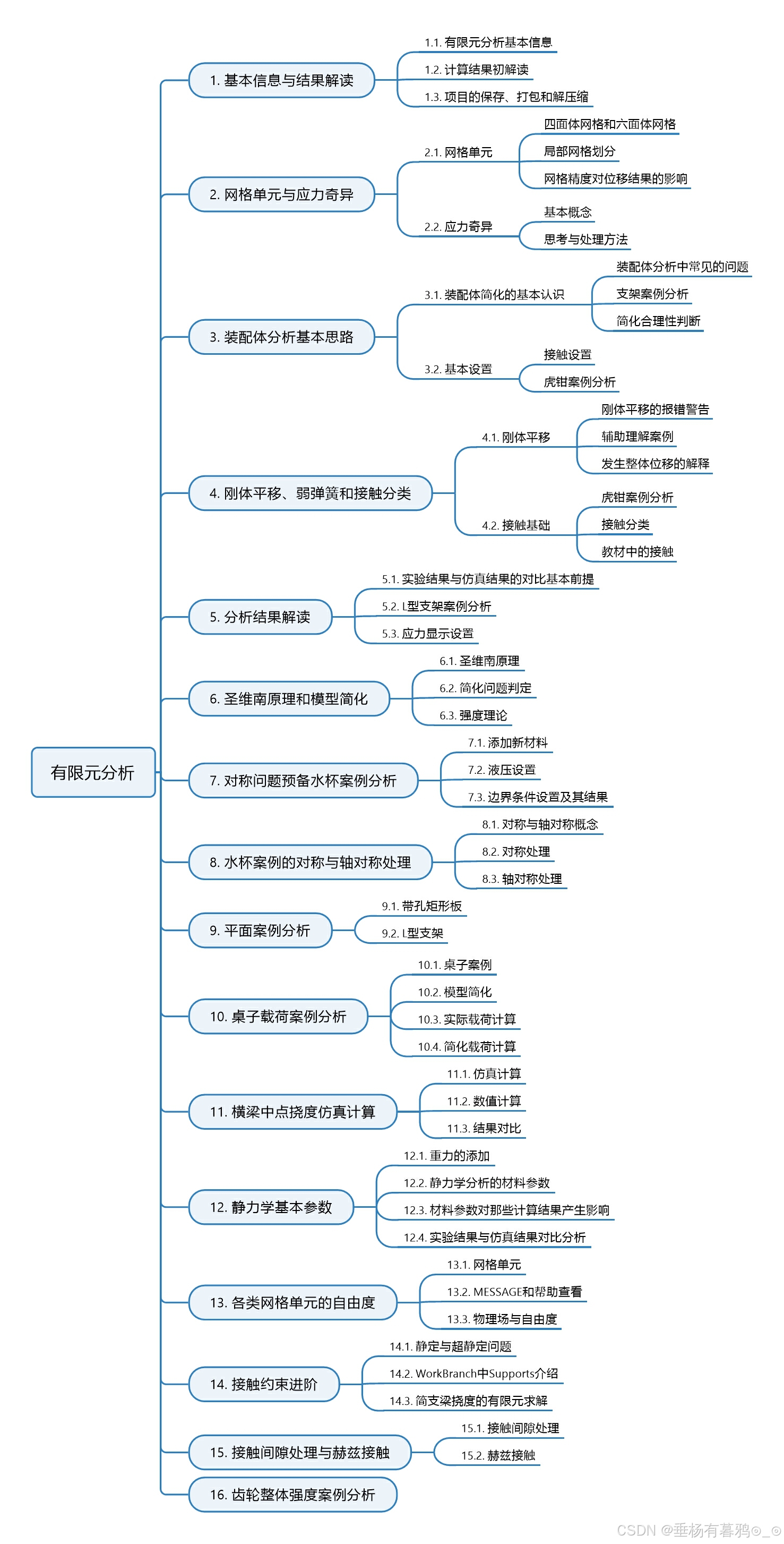
有限元分析学习——Anasys Workbanch第一阶段笔记梳理
第一阶段笔记主要源自于哔哩哔哩《ANSYS-workbench 有限元分析应用基础教程》 张晔 主要内容导图: 笔记导航如下: Anasys Workbanch第一阶段笔记(1)基本信息与结果解读_有限元分析变形比例-CSDN博客 Anasys Workbanch第一阶段笔记(2)网格单元与应力奇…...
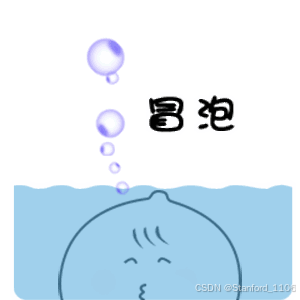
C++中常用的十大排序方法之1——冒泡排序
成长路上不孤单😊😊😊😊😊😊 【😊///计算机爱好者😊///持续分享所学😊///如有需要欢迎收藏转发///😊】 今日分享关于C中常用的排序方法之——冒泡排序的相关…...
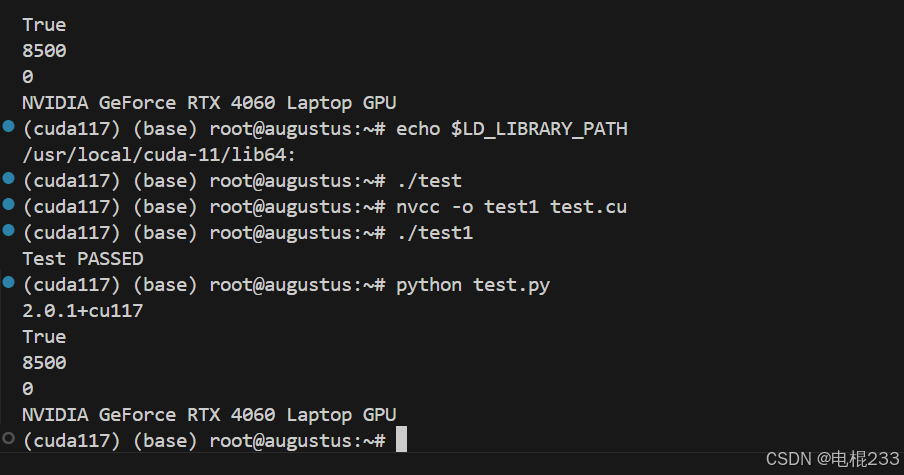
vscode+WSL2(ubuntu22.04)+pytorch+conda+cuda+cudnn安装系列
最近在家过年闲的没事,于是研究起深度学习开发工具链的配置和安装,之前欲与天公试比高,尝试在win上用vscodecuda11.6vs2019的cl编译器搭建cuda c编程环境,最后惨败,沦为笑柄,痛定思痛,这次直接和…...
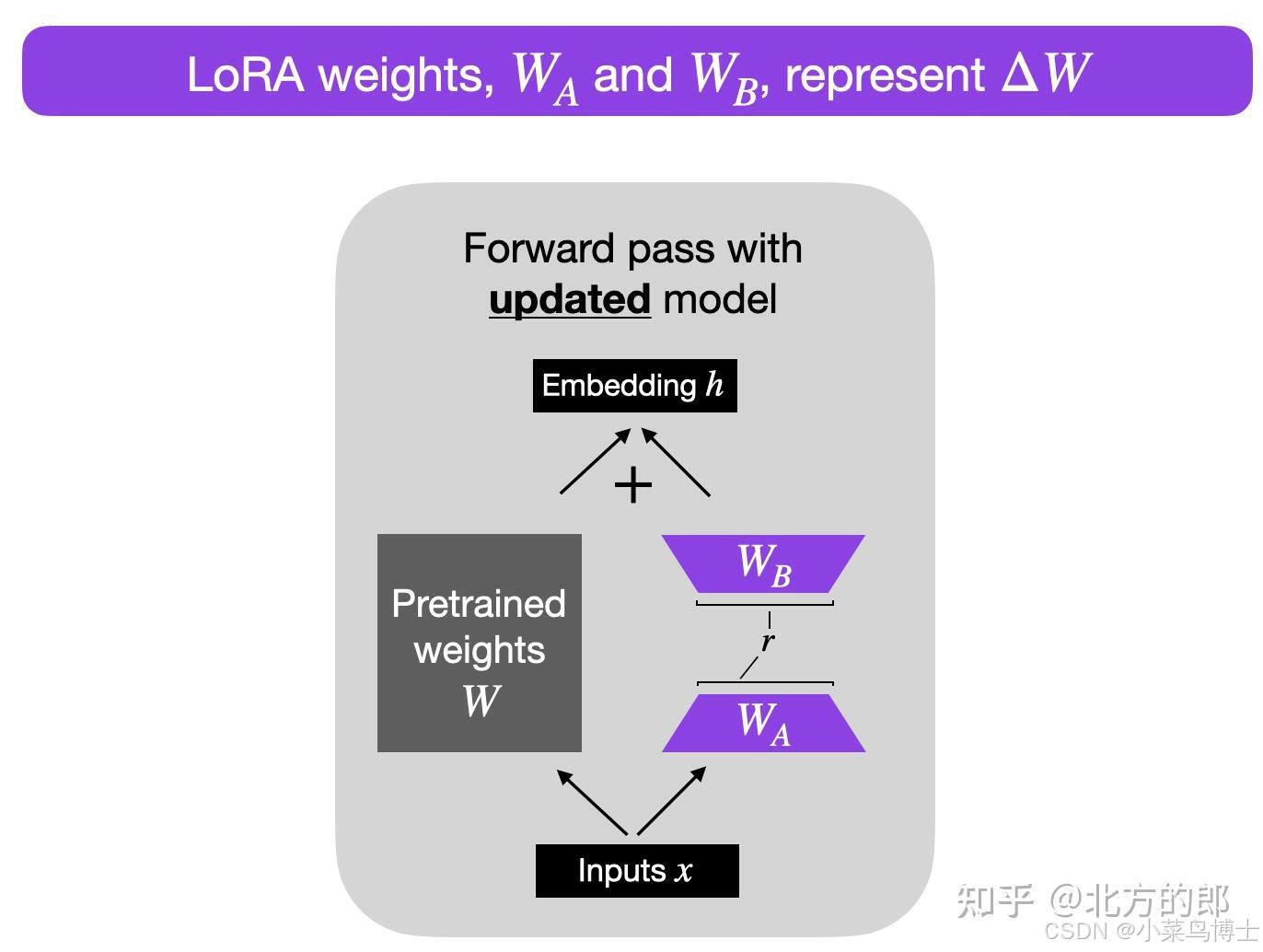
手撕Diffusion系列 - 第十一期 - lora微调 - 基于Stable Diffusion(代码)
手撕Diffusion系列 - 第十一期 - lora微调 - 基于Stable Diffusion(代码) 目录 手撕Diffusion系列 - 第十一期 - lora微调 - 基于Stable Diffusion(代码)Stable Diffusion 原理图Stable Diffusion的原理解释Stable Diffusion 和Di…...