【机器学习】Linear Regression
Model Representation
- 1、问题描述
- 2、表示说明
- 3、数据绘图
- 4、模型函数
- 5、预测
- 总结
- 附录
1、问题描述
一套 1000 平方英尺 (sqft) 的房屋售价为300,000美元,一套 2000 平方英尺的房屋售价为500,000美元。这两点将构成我们的数据或训练集。面积单位为 1000 平方英尺,价格单位为 1000 美元。
Size (1000 sqft) | Price (1000s of dollars) |
---|---|
1.0 | 300 |
2.0 | 500 |
希望通过这两个点拟合线性回归模型,以便可以预测其他房屋的价格。例如,面积为 1200 平方英尺的房屋价格是多少。
首先导入所需要的库
import numpy as np
import matplotlib.pyplot as plt
plt.style.use('./deeplearning.mplstyle')
以下代码来创建x_train和y_train变量。数据存储在一维 NumPy 数组中。
# x_train is the input variable (size in 1000 square feet)
# y_train is the target (price in 1000s of dollars)
x_train = np.array([1.0, 2.0])
y_train = np.array([300.0, 500.0])
print(f"x_train = {x_train}")
print(f"y_train = {y_train}")
2、表示说明
使用 m 来表示训练样本的数量。 (x ( i ) ^{(i)} (i), y ( i ) ^{(i)} (i)) 表示第 i 个训练样本。由于 Python 是零索引的,(x ( 0 ) ^{(0)} (0), y ( 0 ) ^{(0)} (0)) 是 (1.0, 300.0) , (x ( 1 ) ^{(1)} (1), y ( 1 ) ^{(1)} (1)) 是 (2.0, 500.0).
3、数据绘图
使用 matplotlib
库中的scatter()
函数绘制这两个点。 其中,函数参数marker
和 c
将点显示为红叉(默认为蓝点)。使用matplotlib
库中的其他函数来设置要显示的标题和标签。
# Plot the data points
plt.scatter(x_train, y_train, marker='x', c='r')
# Set the title
plt.title("Housing Prices")
# Set the y-axis label
plt.ylabel('Price (in 1000s of dollars)')
# Set the x-axis label
plt.xlabel('Size (1000 sqft)')
plt.show()
4、模型函数
线性回归的模型函数(这是一个从 x
映射到 y
的函数)可以表示为 f w , b ( x ( i ) ) = w x ( i ) + b (1) f_{w,b}(x^{(i)}) = wx^{(i)} + b \tag{1} fw,b(x(i))=wx(i)+b(1)
计算 f w , b ( x ( i ) ) f_{w,b}(x^{(i)}) fw,b(x(i)) 的值,可以将每个数据点显示地写为:
对于 x ( 0 ) x^{(0)} x(0), f_wb = w * x[0] + b
对于 x ( 1 ) x^{(1)} x(1), f_wb = w * x[1] + b
对于大量的数据点,这可能会变得笨拙且重复。 因此,可以在for
循环中计算输出,如下面的函数compute_model_output
所示。
def compute_model_output(x, w, b):"""Computes the prediction of a linear modelArgs:x (ndarray (m,)): Data, m examples w,b (scalar) : model parameters Returnsy (ndarray (m,)): target values"""m = x.shape[0]f_wb = np.zeros(m)for i in range(m):f_wb[i] = w * x[i] + breturn f_wb
调用 compute_model_output
函数并绘制输出
w = 100
b = 100tmp_f_wb = compute_model_output(x_train, w, b,)# Plot our model prediction
plt.plot(x_train, tmp_f_wb, c='b',label='Our Prediction')# Plot the data points
plt.scatter(x_train, y_train, marker='x', c='r',label='Actual Values')# Set the title
plt.title("Housing Prices")
# Set the y-axis label
plt.ylabel('Price (in 1000s of dollars)')
# Set the x-axis label
plt.xlabel('Size (1000 sqft)')
plt.legend()
plt.show()
很明显, w = 100 w = 100 w=100 和 b = 100 b = 100 b=100 不会产生适合数据的直线。
根据学过的数学知识,可以容易求出 w = 200 w = 200 w=200 和 b = 100 b = 100 b=100
5、预测
现在我们已经有了一个模型,可以用它来做出房屋价格的预测。来预测一下 1200 平方英尺的房子的价格。由于面积单位为 1000 平方英尺,所以 x x x 是1.2。
w = 200
b = 100
x_i = 1.2
cost_1200sqft = w * x_i + b print(f"${cost_1200sqft:.0f} thousand dollars")
输出的结果是:$340 thousand dollars
总结
- 线性回归建立一个特征和目标之间关系的模型
- 在上面的例子中,特征是房屋面积,目标是房价。
- 对于简单线性回归,模型有两个参数 w w w 和 b b b ,其值使用训练数据进行拟合。
- 一旦确定了模型的参数,该模型就可以用于对新数据进行预测。
附录
deeplearning.mplstyle 源码:
# see https://matplotlib.org/stable/tutorials/introductory/customizing.html
lines.linewidth: 4
lines.solid_capstyle: buttlegend.fancybox: true# Verdana" for non-math text,
# Cambria Math#Blue (Crayon-Aqua) 0096FF
#Dark Red C00000
#Orange (Apple Orange) FF9300
#Black 000000
#Magenta FF40FF
#Purple 7030A0axes.prop_cycle: cycler('color', ['0096FF', 'FF9300', 'FF40FF', '7030A0', 'C00000'])
#axes.facecolor: f0f0f0 # grey
axes.facecolor: ffffff # white
axes.labelsize: large
axes.axisbelow: true
axes.grid: False
axes.edgecolor: f0f0f0
axes.linewidth: 3.0
axes.titlesize: x-largepatch.edgecolor: f0f0f0
patch.linewidth: 0.5svg.fonttype: pathgrid.linestyle: -
grid.linewidth: 1.0
grid.color: cbcbcbxtick.major.size: 0
xtick.minor.size: 0
ytick.major.size: 0
ytick.minor.size: 0savefig.edgecolor: f0f0f0
savefig.facecolor: f0f0f0#figure.subplot.left: 0.08
#figure.subplot.right: 0.95
#figure.subplot.bottom: 0.07#figure.facecolor: f0f0f0 # grey
figure.facecolor: ffffff # white## ***************************************************************************
## * FONT *
## ***************************************************************************
## The font properties used by `text.Text`.
## See https://matplotlib.org/api/font_manager_api.html for more information
## on font properties. The 6 font properties used for font matching are
## given below with their default values.
##
## The font.family property can take either a concrete font name (not supported
## when rendering text with usetex), or one of the following five generic
## values:
## - 'serif' (e.g., Times),
## - 'sans-serif' (e.g., Helvetica),
## - 'cursive' (e.g., Zapf-Chancery),
## - 'fantasy' (e.g., Western), and
## - 'monospace' (e.g., Courier).
## Each of these values has a corresponding default list of font names
## (font.serif, etc.); the first available font in the list is used. Note that
## for font.serif, font.sans-serif, and font.monospace, the first element of
## the list (a DejaVu font) will always be used because DejaVu is shipped with
## Matplotlib and is thus guaranteed to be available; the other entries are
## left as examples of other possible values.
##
## The font.style property has three values: normal (or roman), italic
## or oblique. The oblique style will be used for italic, if it is not
## present.
##
## The font.variant property has two values: normal or small-caps. For
## TrueType fonts, which are scalable fonts, small-caps is equivalent
## to using a font size of 'smaller', or about 83%% of the current font
## size.
##
## The font.weight property has effectively 13 values: normal, bold,
## bolder, lighter, 100, 200, 300, ..., 900. Normal is the same as
## 400, and bold is 700. bolder and lighter are relative values with
## respect to the current weight.
##
## The font.stretch property has 11 values: ultra-condensed,
## extra-condensed, condensed, semi-condensed, normal, semi-expanded,
## expanded, extra-expanded, ultra-expanded, wider, and narrower. This
## property is not currently implemented.
##
## The font.size property is the default font size for text, given in points.
## 10 pt is the standard value.
##
## Note that font.size controls default text sizes. To configure
## special text sizes tick labels, axes, labels, title, etc., see the rc
## settings for axes and ticks. Special text sizes can be defined
## relative to font.size, using the following values: xx-small, x-small,
## small, medium, large, x-large, xx-large, larger, or smallerfont.family: sans-serif
font.style: normal
font.variant: normal
font.weight: normal
font.stretch: normal
font.size: 8.0font.serif: DejaVu Serif, Bitstream Vera Serif, Computer Modern Roman, New Century Schoolbook, Century Schoolbook L, Utopia, ITC Bookman, Bookman, Nimbus Roman No9 L, Times New Roman, Times, Palatino, Charter, serif
font.sans-serif: Verdana, DejaVu Sans, Bitstream Vera Sans, Computer Modern Sans Serif, Lucida Grande, Geneva, Lucid, Arial, Helvetica, Avant Garde, sans-serif
font.cursive: Apple Chancery, Textile, Zapf Chancery, Sand, Script MT, Felipa, Comic Neue, Comic Sans MS, cursive
font.fantasy: Chicago, Charcoal, Impact, Western, Humor Sans, xkcd, fantasy
font.monospace: DejaVu Sans Mono, Bitstream Vera Sans Mono, Computer Modern Typewriter, Andale Mono, Nimbus Mono L, Courier New, Courier, Fixed, Terminal, monospace## ***************************************************************************
## * TEXT *
## ***************************************************************************
## The text properties used by `text.Text`.
## See https://matplotlib.org/api/artist_api.html#module-matplotlib.text
## for more information on text properties
#text.color: black
相关文章:
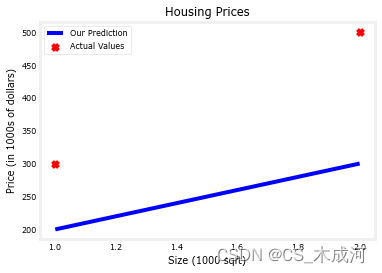
【机器学习】Linear Regression
Model Representation 1、问题描述2、表示说明3、数据绘图4、模型函数5、预测总结附录 1、问题描述 一套 1000 平方英尺 (sqft) 的房屋售价为300,000美元,一套 2000 平方英尺的房屋售价为500,000美元。这两点将构成我们的数据或训练集。面积单位为 1000 平方英尺&a…...

STM32 中断优先级管理(二)
NVIC中断管理相关函数主要在HAL库关键文件stm32f1xx_hal_cortex.c中定义。 中断优先级分组函数 void HAL_NVIC_SetPriorityGrouping(uint32_t PriorityGroup);这个函数的作用是对中断的优先级进行分组,这个函数在系统中只需要被调用一次。 void HAL_NVIC_SetPrio…...

17-汽水瓶
题目 某商店规定:三个空汽水瓶可以换一瓶汽水,允许向老板借空汽水瓶(但是必须要归还)。 小张手上有n个空汽水瓶,她想知道自己最多可以喝到多少瓶汽水。 数据范围:输入的正整数满足 1≤n≤100 注意&…...
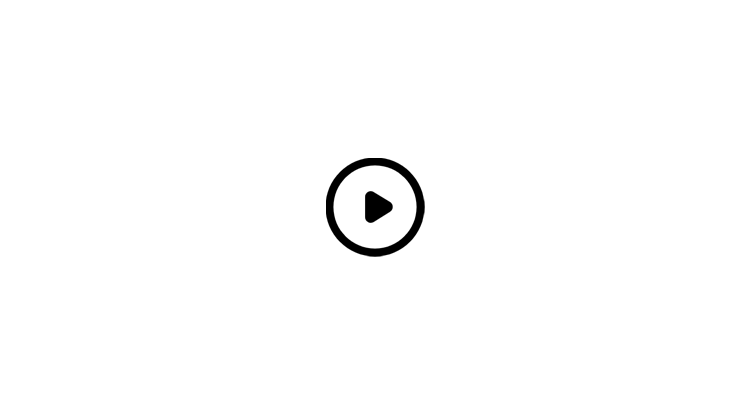
Mindar.JS——实现AR图像追踪插入图片或视频
Mindar.JS使用方式 注意:此篇文章需要启动https才可调用相机权限 图像追踪示例 需要用到两个js库 <script src"./js/aframe.min.js"></script><script src"./js/mindar-image-aframe.prod.js"></script>下面看一下标签…...
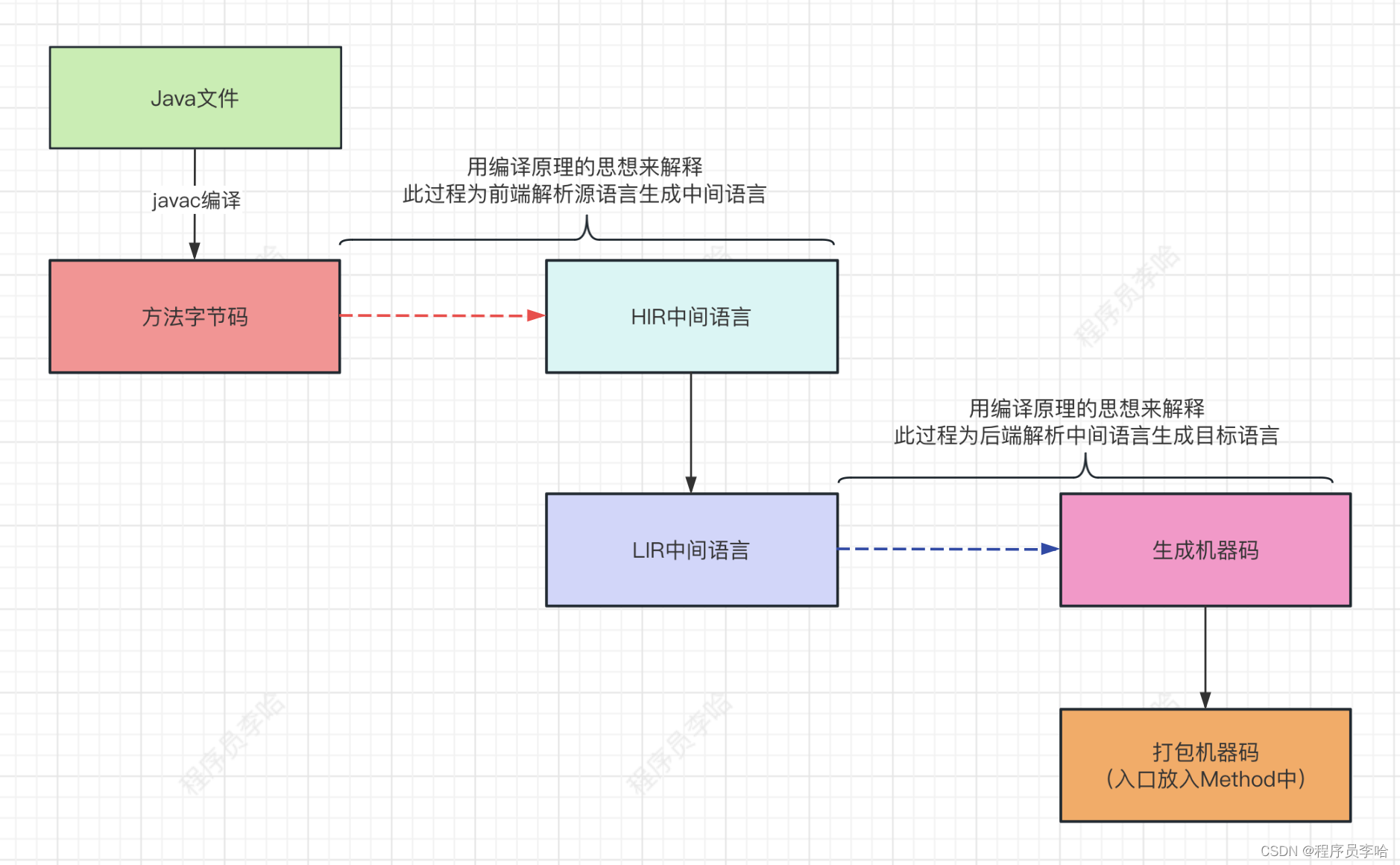
JVM源码剖析之JIT工作流程
版本信息: jdk版本:jdk8u40思想至上 Hotspot中执行引擎分为解释器、JIT及时编译器,上篇文章描述到解释器过度到JIT的条件。JVM源码剖析之达到什么条件进行JIT优化 这篇文章大致讲述JIT的编译过程。在JDK中javac和JIT两部分跟编译原理挂钩&a…...
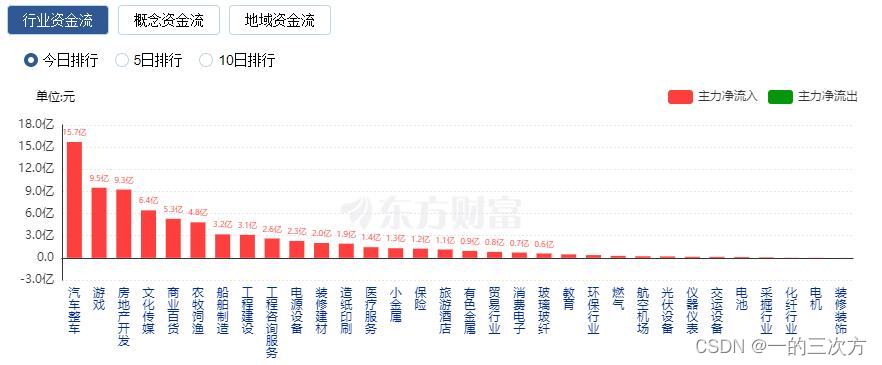
【投资笔记】(23/7/31)下半年消费复苏的机会来了?
本文为本人投资逻辑验证,不作为任何建议; 政策面 汽车:(一)优化汽车购买使用管理(二)扩大新能源汽车消费,重点在于新能源汽车;房地产:(三&#x…...

MySQL二进制日志(binlog)配置、二进制日志binlog查看、mysqlbinlog查看二进制日志、二进制日志binlog清理等详解
提示:MySQL 中的日志比较重要的有 binlog(归档日志)、redo log(重做日志)以及 undo log,那么跟我们本文相关的主要是 binlog,另外两个日志松哥将来有空了再和大家详细介绍。 文章目录 1、二进制…...

Python内存管理解析:高效利用资源的关键
推荐阅读 AI文本 OCR识别最佳实践 AI Gamma一键生成PPT工具直达链接 玩转cloud Studio 在线编码神器 玩转 GPU AI绘画、AI讲话、翻译,GPU点亮AI想象空间 引言 在当今互联网时代,Python已经成为最受欢迎的编程语言之一。它的简洁、灵活和强大的生态系统使其成为…...
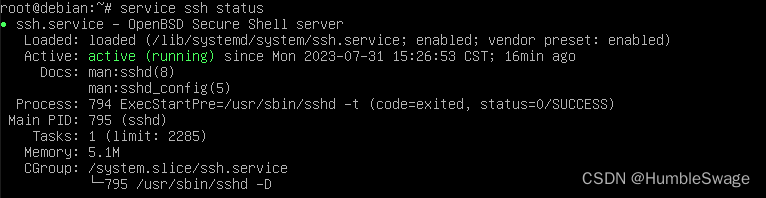
解决Debian10乱码以及远程连接ssh的问题
文章目录 解决Debian10乱码Debian10配置ssh 解决Debian10乱码 下载locales apt-get install locales配置语言 dpkg-reconfigure locales输入上述命令后会进入到以下页面【空格为选中,回车下一个页面】 在这个页面里我们按空格选中如图的选项,然后回…...

C# 泛型(Generic)
方法重载:方法名称相同,参数个数和参数类型不同; 优势:可以节约方法名称 劣势:方法过多 语法:public void writeContent(T t) 原理:普通的C#代码他是运行在前端进行编译,所有的类型需…...

Golang之路---02 基础语法——流程控制(if-else , switch-case , for-range , defer)
流程控制 条件语句——if-else if 条件 1 {分支 1 } else if 条件 2 {分支 2 } else if 条件 ... {分支 ... } else {分支 else }注: Golang编译器,对于 { 和 } 的位置有严格的要求,它要求 else if (或 else)和 两边…...
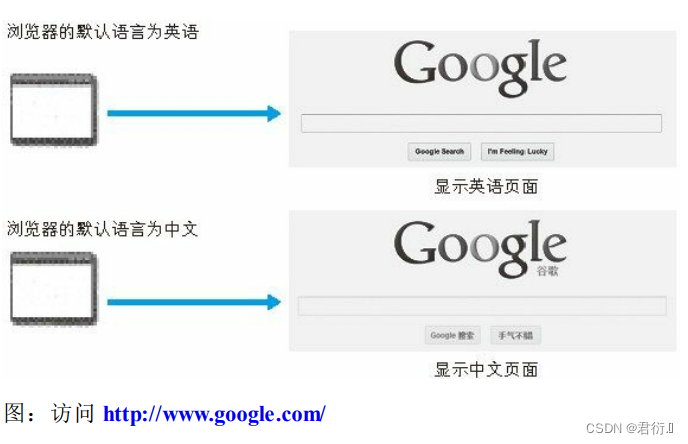
HTTP——HTTP报文内的HTTP信息
HTTP 通信过程包括从客户端发往服务器端的请求及从服务器端返回客户端的响应。本章就让我们来了解一下请求和响应是怎样运作的。 HTTP 一、HTTP报文二、请求报文及响应报文的结构三、编码提升传输速率1、报文主体和实体主题的差异2、压缩传输的内容编码3、分割发送的分块传输编…...
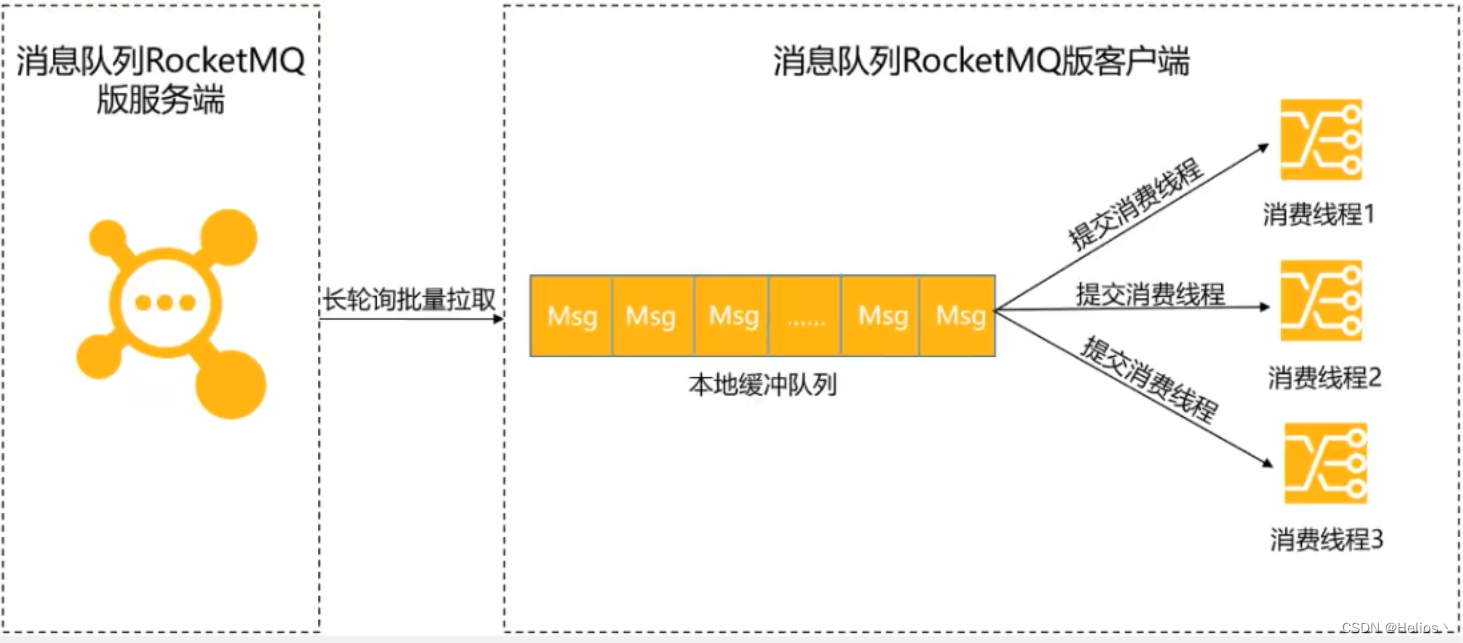
RocketMQ工作原理
文章目录 三.RocketMQ工作原理1.消息的生产消息的生产过程Queue选择算法 2.消息的存储1.commitlog文件目录与文件消息单元 2.consumequeue目录与文件索引条目 3.对文件的读写消息写入消息拉取性能提升 3.indexFile1.索引条目结构2.文件名的作用3.查询流程 4.消息的消费1.推拉消…...
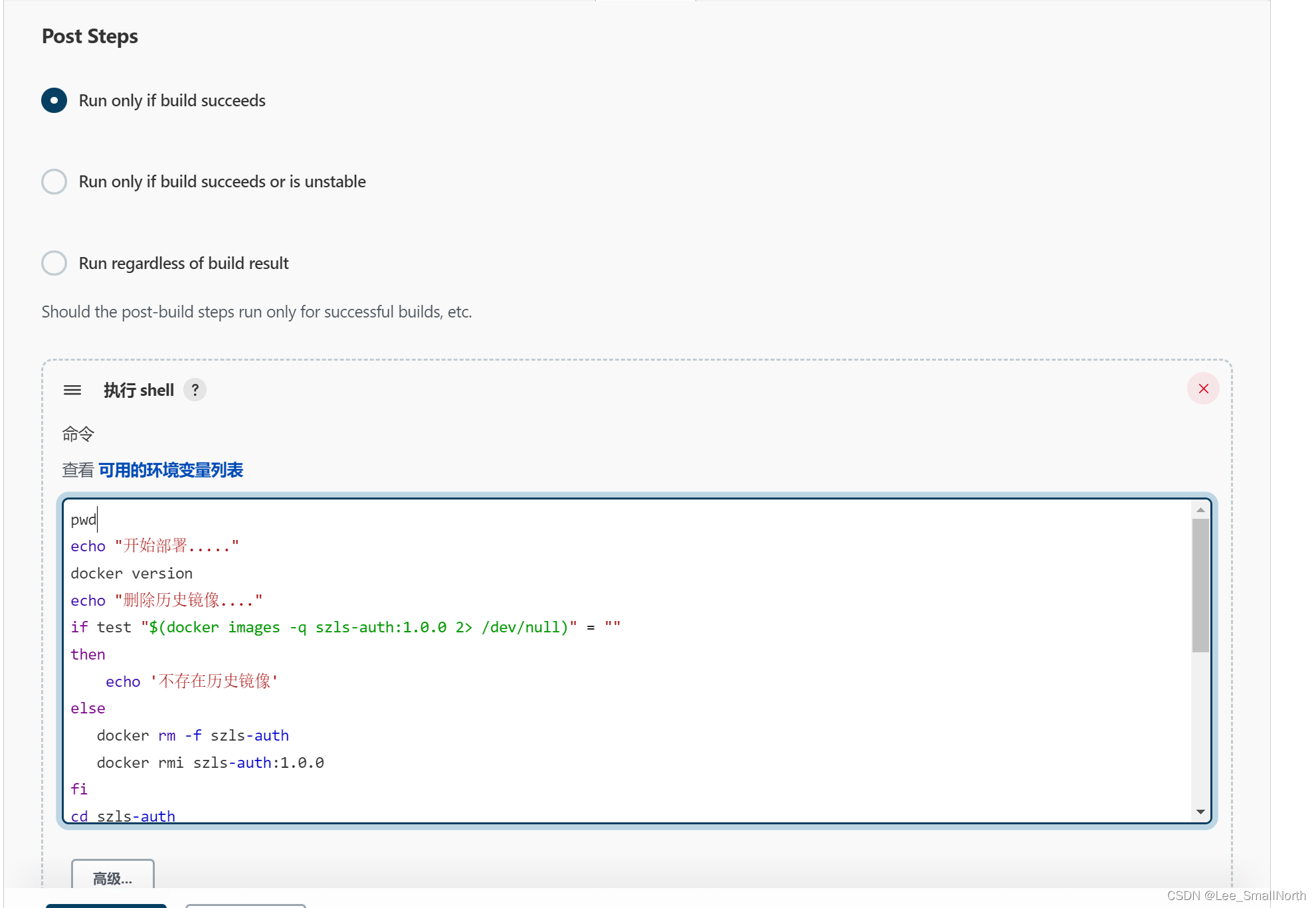
Jenkins+Docker+Docker-Compose自动部署,SpringCloud架构公共包一个任务配置
前言 Jenkins和docker的安装,随便百度吧,实际场景中我们很多微服务的架构,都是有公共包,肯定是希望一个任务能够把公共包的配置加进去,一并构建,ok,直接上干货。 Jenkins 全局环境安装 pwd e…...
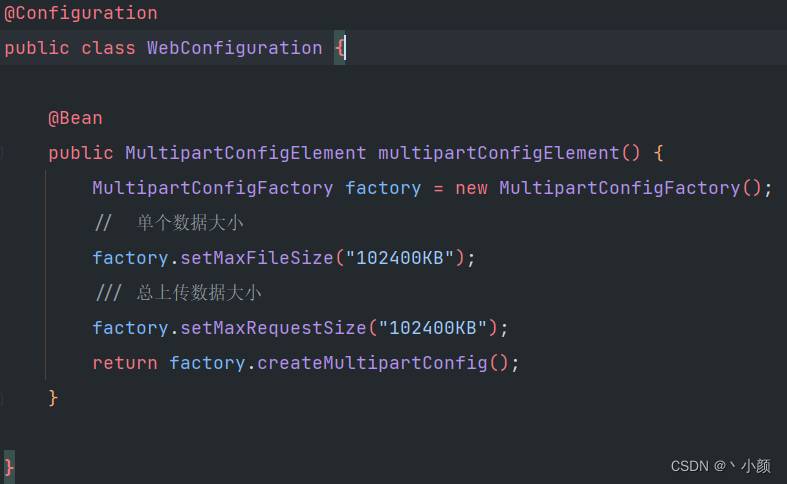
spring boot 2 配置上传文件大小限制
一、起因:系统页面上传一个文件超过日志提示的文件最大100M的限制,需要更改配置文件 二、经过: 1、在本地代码中找到配置文件,修改相应数值后交给运维更新生产环境配置,但是运维说生产环境没有这行配置,遂…...
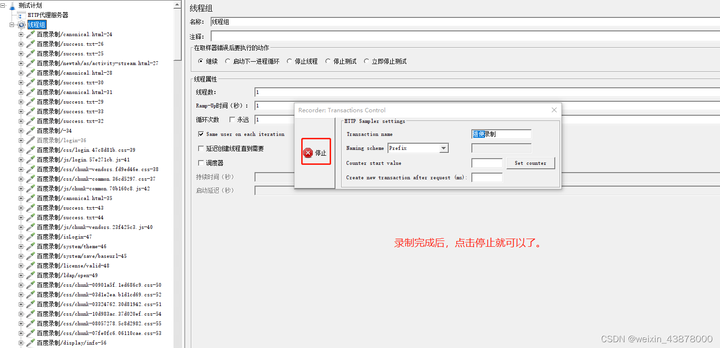
Jmeter —— 录制脚本
1. 第一步:添加http代理服务器,在测试计划--》添加--》非测试元件--》http代理服务器 2. 第二步:添加线程组(这个线程组是用来放录制的脚本,不添加也可以,就直接放在代理服务器下) 测试计划--》…...
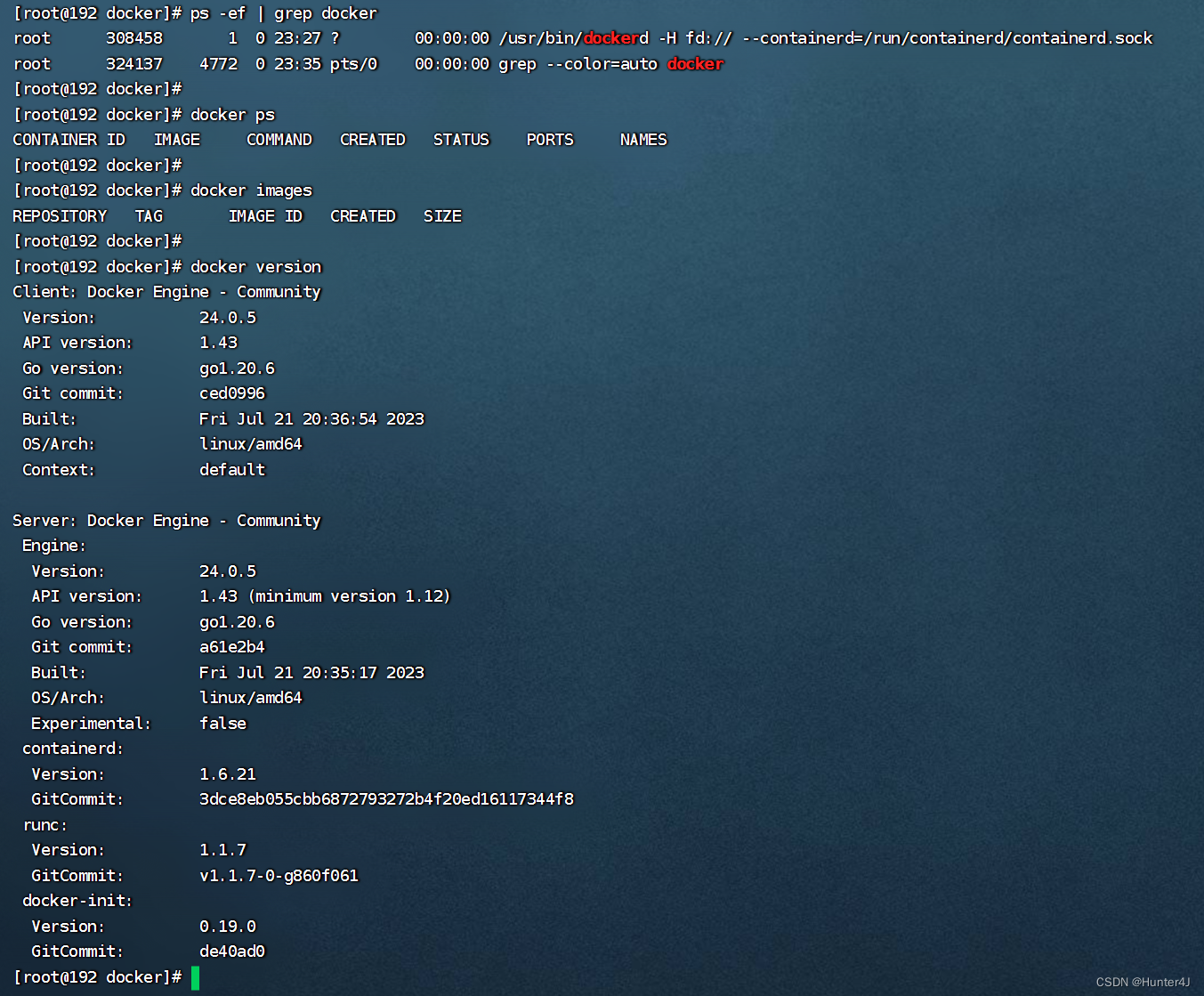
从零开始学Docker(一):Docker的安装部署
前述:本次学习与整理来至B站【Python开发_老6哥】老师分享的课程,有兴趣的小伙伴可以去加油啦,附链接 宿主机环境:RockyLinux 9 版本管理 Docker引擎主要有两个版本:企业版(EE)和社区版&#…...

【ROS 02】ROS通信机制
机器人是一种高度复杂的系统性实现,在机器人上可能集成各种传感器(雷达、摄像头、GPS...)以及运动控制实现,为了解耦合,在ROS中每一个功能点都是一个单独的进程,每一个进程都是独立运行的。更确切的讲,ROS是进程&#…...
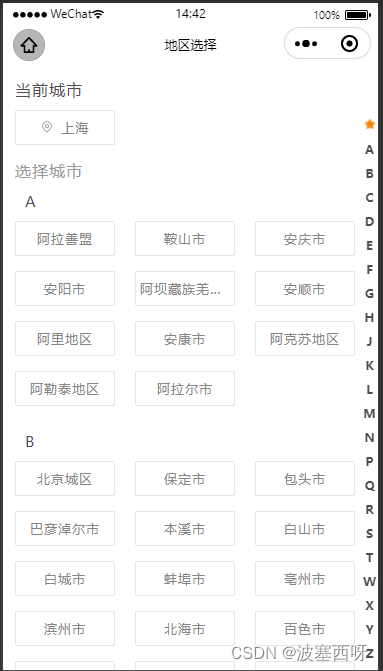
uniapp 选择城市定位 根据城市首字母分类排序
获取城市首字母排序,按字母顺序排序 <template><view class"address-wrap" id"address"><!-- 搜索输入框-end --><template v-if"!isSearch"><!-- 城市列表-start --><view class"address-sc…...
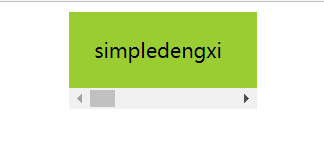
flex盒子 center排布,有滚动条时,拖动滚动条无法完整显示内容
文章目录 问题示例代码解决问题改进后的效果 问题 最近在开发项目的过程中,发现了一个有趣的事情,与flex盒子有关,不知道算不算是一个bug,不过对于开发者来说,确实有些不方便,感兴趣的同学不妨也去试试。 …...

Workbox使用分享
一、简要介绍 1.1 什么是Workbox 官方文档原文: At this point, service workers may seem tricky. There’s lots of complex interactions that are hard to get right. Network requests! Caching strategies! Cache management! Precaching! It’s a lot to r…...

秋招算法备战第32天 | 122.买卖股票的最佳时机II、55. 跳跃游戏、45.跳跃游戏II
122. 买卖股票的最佳时机 II - 力扣(LeetCode) 通过做差可以得到利润序列,然后只要利润需求的非负数求和就可以,因为这里没有手续费,某天买入之后买出可以等价为这几天连续买入卖出 class Solution:def maxProfit(se…...

Python状态模式介绍、使用
一、Python状态模式介绍 Python状态模式(State Pattern)是一种行为型设计模式,它允许对象在不同的状态下表现不同的行为,从而避免在代码中使用多重条件语句。该模式将状态封装在独立的对象中,并根据当前状态选择不同的…...
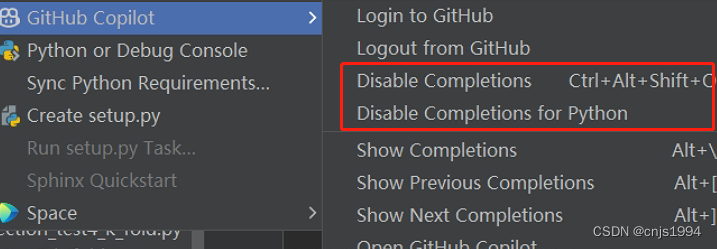
Github-Copilot初体验-Pycharm插件的安装与测试
引言: 80%代码秒生成!AI神器Copilot大升级 最近copilot又在众多独角兽公司的合力下,取得了重大升级。GitHub Copilot发布还不到两年, 就已经为100多万的开发者,编写了46%的代码,并提高了55%的编码速度。 …...

Spring AOP API详解
上一章介绍了Spring对AOP的支持,包括AspectJ和基于schema的切面定义。在这一章中,我们将讨论低级别的Spring AOP API。对于普通的应用,我们推荐使用前一章中描述的带有AspectJ pointcuts 的Spring AOP。 6.1. Spring 中的 Pointcut API 这一…...

分治法 Divide and Conquer
1.分治法 分治法(Divide and Conquer)是一种常见的算法设计思想,它将一个大问题分解成若干个子问题,递归地解决每个子问题,最后将子问题的解合并起来得到整个问题的解。分治法通常包含三个步骤: 1. Divid…...

super(Module_ModuleList, self).__init__()的作用是什么?
class Module_ModuleList(nn.Module):def __init__(self):super(Module_ModuleList, self).__init__()self.linears nn.ModuleList([nn.Linear(10, 10)])在这段代码中,super(Module_ModuleList, self).__init__() 的作用是调用父类 nn.Module 的 __init__ 方法&…...
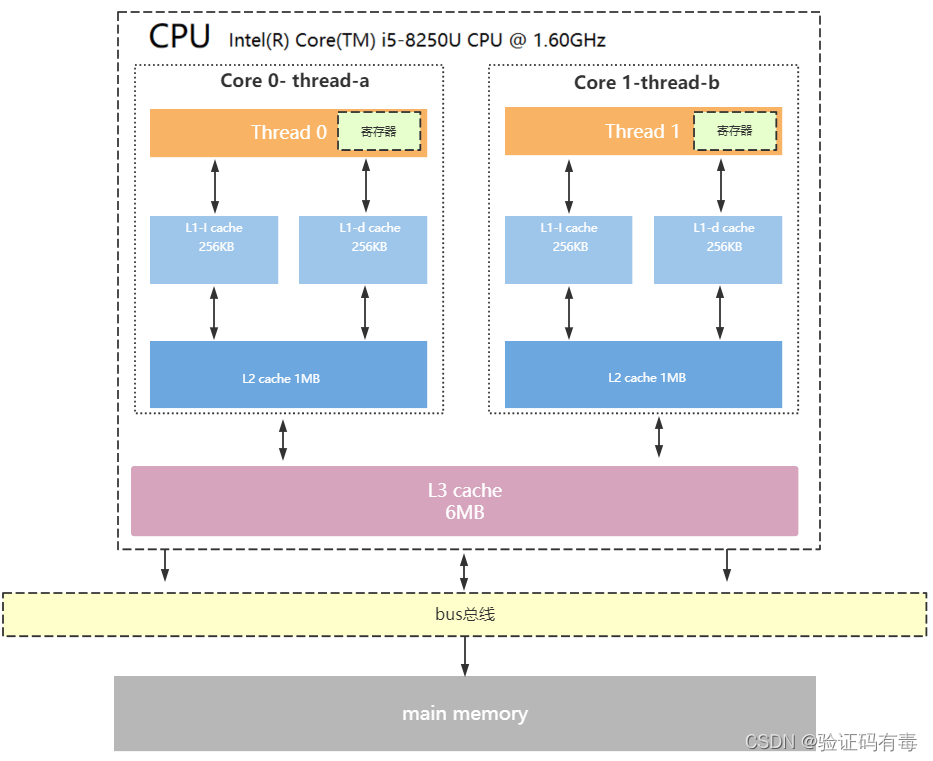
【并发专题】操作系统模型及三级缓存架构
目录 课程内容一、冯诺依曼计算机模型详解1.计算机五大核心组成部分2.CPU内部结构3.CPU缓存结构4.CPU读取存储器数据过程5.CPU为何要有高速缓存 学习总结 课程内容 一、冯诺依曼计算机模型详解 现代计算机模型是基于-冯诺依曼计算机模型 计算机在运行时,先从内存中…...

java基础复习(第二日)
java基础复习(二) 1.抽象的(abstract)方法是否可同时是静态的(static),是否可同时是本地方法(native),是否可同时被 synchronized修饰? 都不能。 抽象方法需要子类重写…...
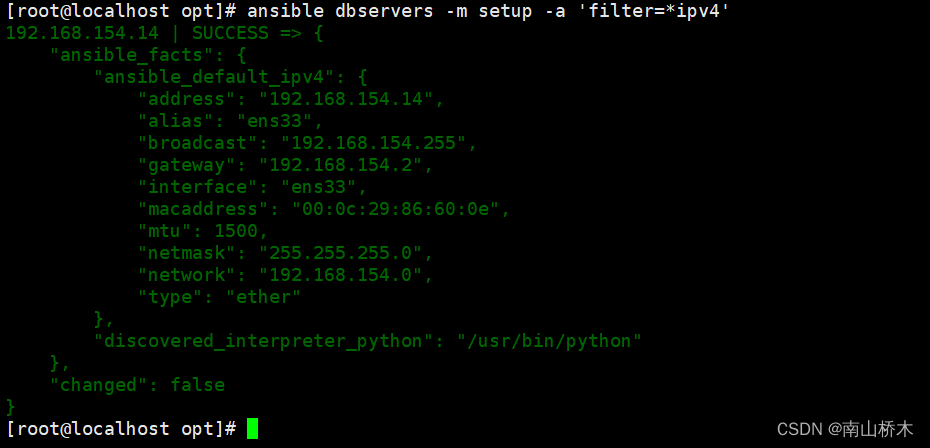
Ansible自动化运维工具
Ansible自动化运维工具 一、ansible介绍二、ansible环境安装部署三、ansible命令行模块1、command模块2、shell模块3、cron模块4、user模块5、group模块6、copy模块7、file模块8、hostname模块9、ping模块10、yum模块11、service/systemd模块12、script模块13、mount模块14、ar…...