NLP(六十四)使用FastChat计算LLaMA-2模型的token长度
LLaMA-2模型部署
在文章NLP(五十九)使用FastChat部署百川大模型中,笔者介绍了FastChat
框架,以及如何使用FastChat
来部署百川模型。
本文将会部署LLaMA-2 70B模型,使得其兼容OpenAI的调用风格。部署的Dockerfile
文件如下:
FROM nvidia/cuda:11.7.1-runtime-ubuntu20.04RUN apt-get update -y && apt-get install -y python3.9 python3.9-distutils curl
RUN curl https://bootstrap.pypa.io/get-pip.py -o get-pip.py
RUN python3.9 get-pip.py
RUN pip3 install fschat
Docker-compose.yml
文件如下:
version: "3.9"services:fastchat-controller:build:context: .dockerfile: Dockerfileimage: fastchat:latestports:- "21001:21001"entrypoint: ["python3.9", "-m", "fastchat.serve.controller", "--host", "0.0.0.0", "--port", "21001"]fastchat-model-worker:build:context: .dockerfile: Dockerfilevolumes:- ./model:/root/modelimage: fastchat:latestports:- "21002:21002"deploy:resources:reservations:devices:- driver: nvidiadevice_ids: ['0', '1']capabilities: [gpu]entrypoint: ["python3.9", "-m", "fastchat.serve.model_worker", "--model-names", "llama2-70b-chat", "--model-path", "/root/model/llama2/Llama-2-70b-chat-hf", "--num-gpus", "2", "--gpus", "0,1", "--worker-address", "http://fastchat-model-worker:21002", "--controller-address", "http://fastchat-controller:21001", "--host", "0.0.0.0", "--port", "21002"]fastchat-api-server:build:context: .dockerfile: Dockerfileimage: fastchat:latestports:- "8000:8000"entrypoint: ["python3.9", "-m", "fastchat.serve.openai_api_server", "--controller-address", "http://fastchat-controller:21001", "--host", "0.0.0.0", "--port", "8000"]
部署成功后,会占用2张A100,每张A100占用约66G显存。
测试模型是否部署成功:
curl http://localhost:8000/v1/models
输出结果如下:
{"object": "list","data": [{"id": "llama2-70b-chat","object": "model","created": 1691504717,"owned_by": "fastchat","root": "llama2-70b-chat","parent": null,"permission": [{"id": "modelperm-3XG6nzMAqfEkwfNqQ52fdv","object": "model_permission","created": 1691504717,"allow_create_engine": false,"allow_sampling": true,"allow_logprobs": true,"allow_search_indices": true,"allow_view": true,"allow_fine_tuning": false,"organization": "*","group": null,"is_blocking": false}]}]
}
部署LLaMA-2 70B模型成功!
Prompt token长度计算
在FastChat
的Github开源项目中,项目提供了计算Prompt的token长度的API,文件路径为:fastchat/serve/model_worker.py,调用方法为:
curl --location 'localhost:21002/count_token' \
--header 'Content-Type: application/json' \
--data '{"prompt": "What is your name?"}'
输出结果如下:
{"count": 6,"error_code": 0
}
Conversation token长度计算
在FastChat
中计算Conversation(对话)的token长度较为麻烦。
首先我们需要获取LLaMA-2 70B模型的对话配置
,调用API如下:
curl --location --request POST 'http://localhost:21002/worker_get_conv_template'
输出结果如下:
{'conv': {'messages': [],'name': 'llama-2','offset': 0,'roles': ['[INST]', '[/INST]'],'sep': ' ','sep2': ' </s><s>','sep_style': 7,'stop_str': None,'stop_token_ids': [2],'system_message': 'You are a helpful, respectful and honest ''assistant. Always answer as helpfully as ''possible, while being safe. Your answers should ''not include any harmful, unethical, racist, ''sexist, toxic, dangerous, or illegal content. ''Please ensure that your responses are socially ''unbiased and positive in nature.\n''\n''If a question does not make any sense, or is not ''factually coherent, explain why instead of '"answering something not correct. If you don't ""know the answer to a question, please don't share "'false information.','system_template': '[INST] <<SYS>>\n{system_message}\n<</SYS>>\n\n'}}
在FastChat
中的对话文件(fastchat/conversation.py)中,提供了对话加工的代码,这里不再展示,使用时直接复制整个文件即可,该文件不依赖任何第三方模块。
我们需要将对话按照OpenAI的方式加工成对应的Prompt,输入的对话(messages)如下:
messages = [{“role”: “system”, “content”: “You are Jack, you are 20 years old, answer questions with humor.”}, {“role”: “user”, “content”: “What is your name?”},{“role”: “assistant”, “content”: " Well, well, well! Look who’s asking the questions now! My name is Jack, but you can call me the king of the castle, the lord of the rings, or the prince of the pizza party. Whatever floats your boat, my friend!“}, {“role”: “user”, “content”: “How old are you?”}, {“role”: “assistant”, “content”: " Oh, you want to know my age? Well, let’s just say I’m older than a bottle of wine but younger than a bottle of whiskey. I’m like a fine cheese, getting better with age, but still young enough to party like it’s 1999!”}, {“role”: “user”, “content”: “Where is your hometown?”}]
Python代码如下:
# -*- coding: utf-8 -*-
# @place: Pudong, Shanghai
# @file: prompt.py
# @time: 2023/8/8 19:24
from conversation import Conversation, SeparatorStylemessages = [{"role": "system", "content": "You are Jack, you are 20 years old, answer questions with humor."}, {"role": "user", "content": "What is your name?"},{"role": "assistant", "content": " Well, well, well! Look who's asking the questions now! My name is Jack, but you can call me the king of the castle, the lord of the rings, or the prince of the pizza party. Whatever floats your boat, my friend!"}, {"role": "user", "content": "How old are you?"}, {"role": "assistant", "content": " Oh, you want to know my age? Well, let's just say I'm older than a bottle of wine but younger than a bottle of whiskey. I'm like a fine cheese, getting better with age, but still young enough to party like it's 1999!"}, {"role": "user", "content": "Where is your hometown?"}]llama2_conv = {"conv":{"name":"llama-2","system_template":"[INST] <<SYS>>\n{system_message}\n<</SYS>>\n\n","system_message":"You are a helpful, respectful and honest assistant. Always answer as helpfully as possible, while being safe. Your answers should not include any harmful, unethical, racist, sexist, toxic, dangerous, or illegal content. Please ensure that your responses are socially unbiased and positive in nature.\n\nIf a question does not make any sense, or is not factually coherent, explain why instead of answering something not correct. If you don't know the answer to a question, please don't share false information.","roles":["[INST]","[/INST]"],"messages":[],"offset":0,"sep_style":7,"sep":" ","sep2":" </s><s>","stop_str":None,"stop_token_ids":[2]}}
conv = llama2_conv['conv']conv = Conversation(name=conv["name"],system_template=conv["system_template"],system_message=conv["system_message"],roles=conv["roles"],messages=list(conv["messages"]), # prevent in-place modificationoffset=conv["offset"],sep_style=SeparatorStyle(conv["sep_style"]),sep=conv["sep"],sep2=conv["sep2"],stop_str=conv["stop_str"],stop_token_ids=conv["stop_token_ids"],)if isinstance(messages, str):prompt = messages
else:for message in messages:msg_role = message["role"]if msg_role == "system":conv.set_system_message(message["content"])elif msg_role == "user":conv.append_message(conv.roles[0], message["content"])elif msg_role == "assistant":conv.append_message(conv.roles[1], message["content"])else:raise ValueError(f"Unknown role: {msg_role}")# Add a blank message for the assistant.conv.append_message(conv.roles[1], None)prompt = conv.get_prompt()print(repr(prompt))
加工后的Prompt如下:
"[INST] <<SYS>>\nYou are Jack, you are 20 years old, answer questions with humor.\n<</SYS>>\n\nWhat is your name?[/INST] Well, well, well! Look who's asking the questions now! My name is Jack, but you can call me the king of the castle, the lord of the rings, or the prince of the pizza party. Whatever floats your boat, my friend! </s><s>[INST] How old are you? [/INST] Oh, you want to know my age? Well, let's just say I'm older than a bottle of wine but younger than a bottle of whiskey. I'm like a fine cheese, getting better with age, but still young enough to party like it's 1999! </s><s>[INST] Where is your hometown? [/INST]"
最后再调用计算Prompt的API(参考上节的Prompt token长度计算),输出该对话的token长度为199.
我们使用FastChat
提供的对话补充接口(v1/chat/completions)验证
输入的对话token长度,请求命令为:
curl --location 'http://localhost:8000/v1/chat/completions' \
--header 'Content-Type: application/json' \
--data '{"model": "llama2-70b-chat","messages": [{"role": "system", "content": "You are Jack, you are 20 years old, answer questions with humor."}, {"role": "user", "content": "What is your name?"},{"role": "assistant", "content": " Well, well, well! Look who'\''s asking the questions now! My name is Jack, but you can call me the king of the castle, the lord of the rings, or the prince of the pizza party. Whatever floats your boat, my friend!"}, {"role": "user", "content": "How old are you?"}, {"role": "assistant", "content": " Oh, you want to know my age? Well, let'\''s just say I'\''m older than a bottle of wine but younger than a bottle of whiskey. I'\''m like a fine cheese, getting better with age, but still young enough to party like it'\''s 1999!"}, {"role": "user", "content": "Where is your hometown?"}]
}'
输出结果为:
{"id": "chatcmpl-mQxcaQcNSNMFahyHS7pamA","object": "chat.completion","created": 1691506768,"model": "llama2-70b-chat","choices": [{"index": 0,"message": {"role": "assistant","content": " Ha! My hometown? Well, that's a tough one. I'm like a bird, I don't have a nest, I just fly around and land wherever the wind takes me. But if you really want to know, I'm from a place called \"The Internet\". It's a magical land where memes and cat videos roam free, and the Wi-Fi is always strong. It's a beautiful place, you should visit sometime!"},"finish_reason": "stop"}],"usage": {"prompt_tokens": 199,"total_tokens": 302,"completion_tokens": 103}
}
注意,输出的prompt_tokens为199,这与我们刚才计算的对话token长度的结果是一致的!
总结
本文主要介绍了如何在FastChat中部署LLaMA-2 70B模型,并详细介绍了Prompt token长度计算以及对话(conversation)的token长度计算。希望能对读者有所帮助~
笔者的一点心得是:阅读源码真的很重要。
笔者的个人博客网址为:https://percent4.github.io/ ,欢迎大家访问~
参考网址
- NLP(五十九)使用FastChat部署百川大模型: https://blog.csdn.net/jclian91/article/details/131650918
- FastChat: https://github.com/lm-sys/FastChat
相关文章:
NLP(六十四)使用FastChat计算LLaMA-2模型的token长度
LLaMA-2模型部署 在文章NLP(五十九)使用FastChat部署百川大模型中,笔者介绍了FastChat框架,以及如何使用FastChat来部署百川模型。 本文将会部署LLaMA-2 70B模型,使得其兼容OpenAI的调用风格。部署的Dockerfile文件…...
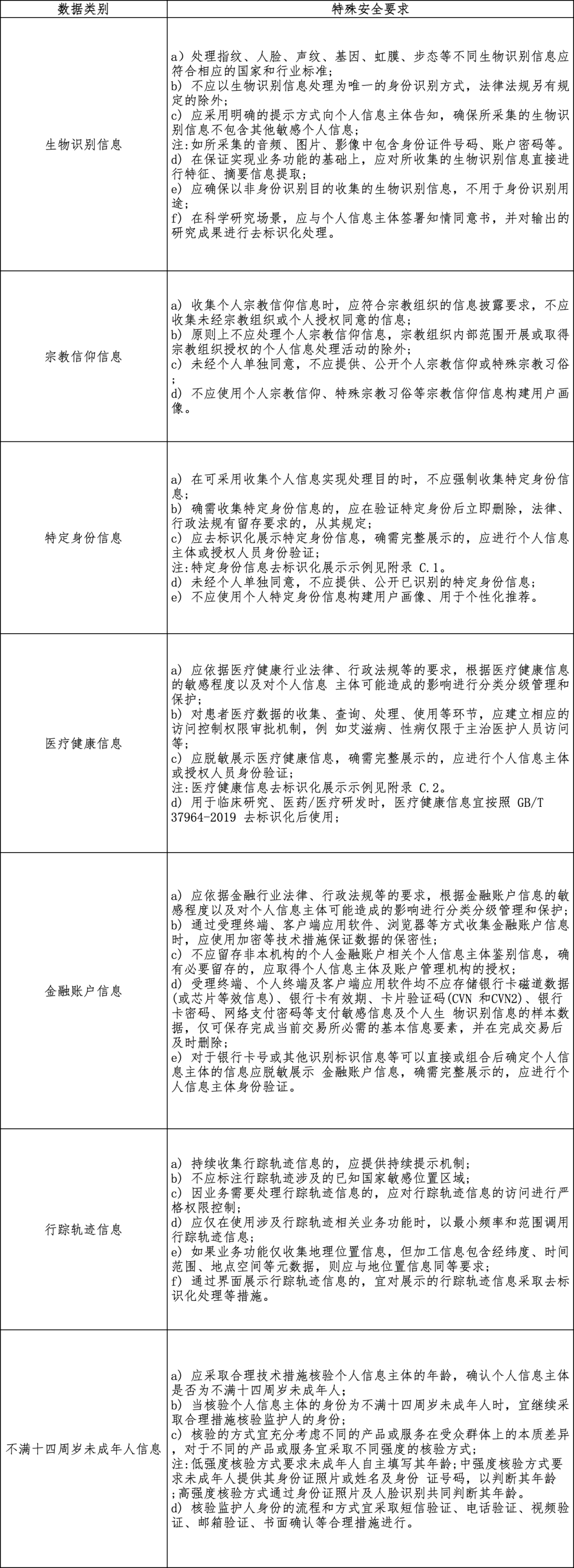
个保新标 | 《信息安全技术 敏感个人信息处理安全要求》(征求意见稿)发布
8 月 9 日,全国信息安全标准化技术委员会公开发布关于国家标准《信息安全技术 敏感个人信息处理安全要求》(征求意见稿)(以下简称《标准》)的通知,面向社会广泛征求意见。 《标准》的制定背景是为支撑《个人…...
【uniapp 返回顶部】
返回顶部 参数说明示例官方链接 uni.pageScrollTo(OBJECT) 将页面滚动到目标位置。 参数说明 参数名类型必填说明scrollTopNumber否滚动到页面的目标位置(单位px)selectorString否选择器,App、H5、微信小程序2.7.3 、支付宝小程序1.20.0支持…...
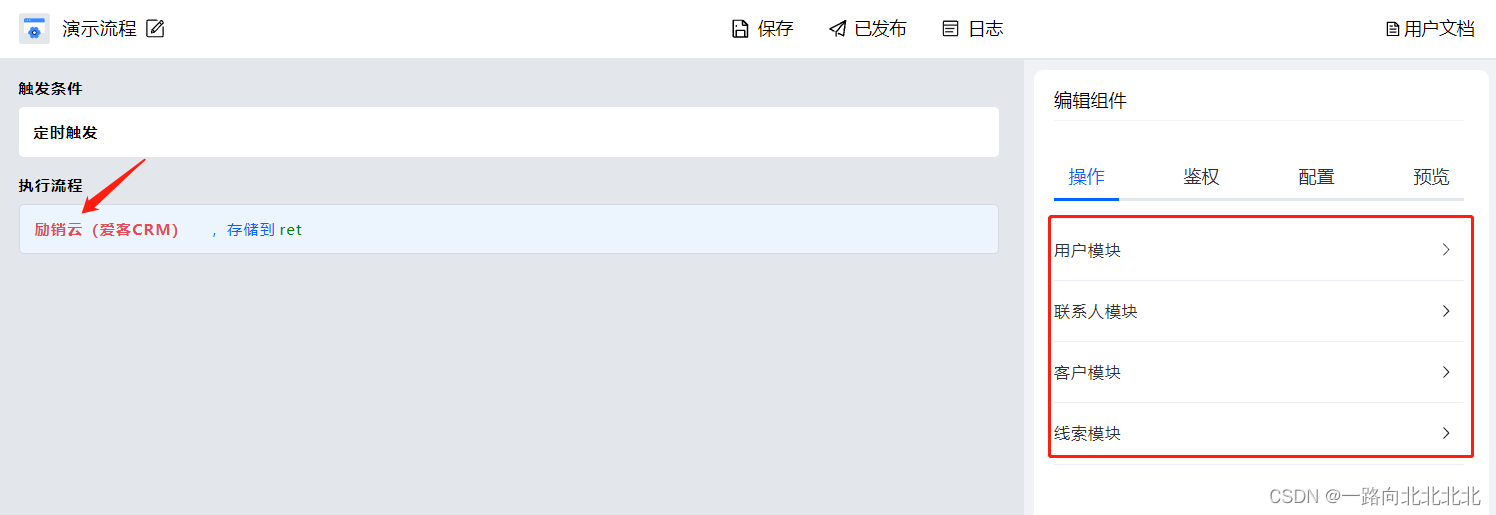
无代码集成励销云CRM连接更多应用
场景描述: 基于励销云的开放API,实现无代码集成连接励销云与其它应用。通过Aboter可轻松搭建业务自动化流程,实现多个应用之间的数据连接。 接口能力: 用户模块业务模块拜访签到模块公海客户模块联系人模块合同模块客户模块任务…...
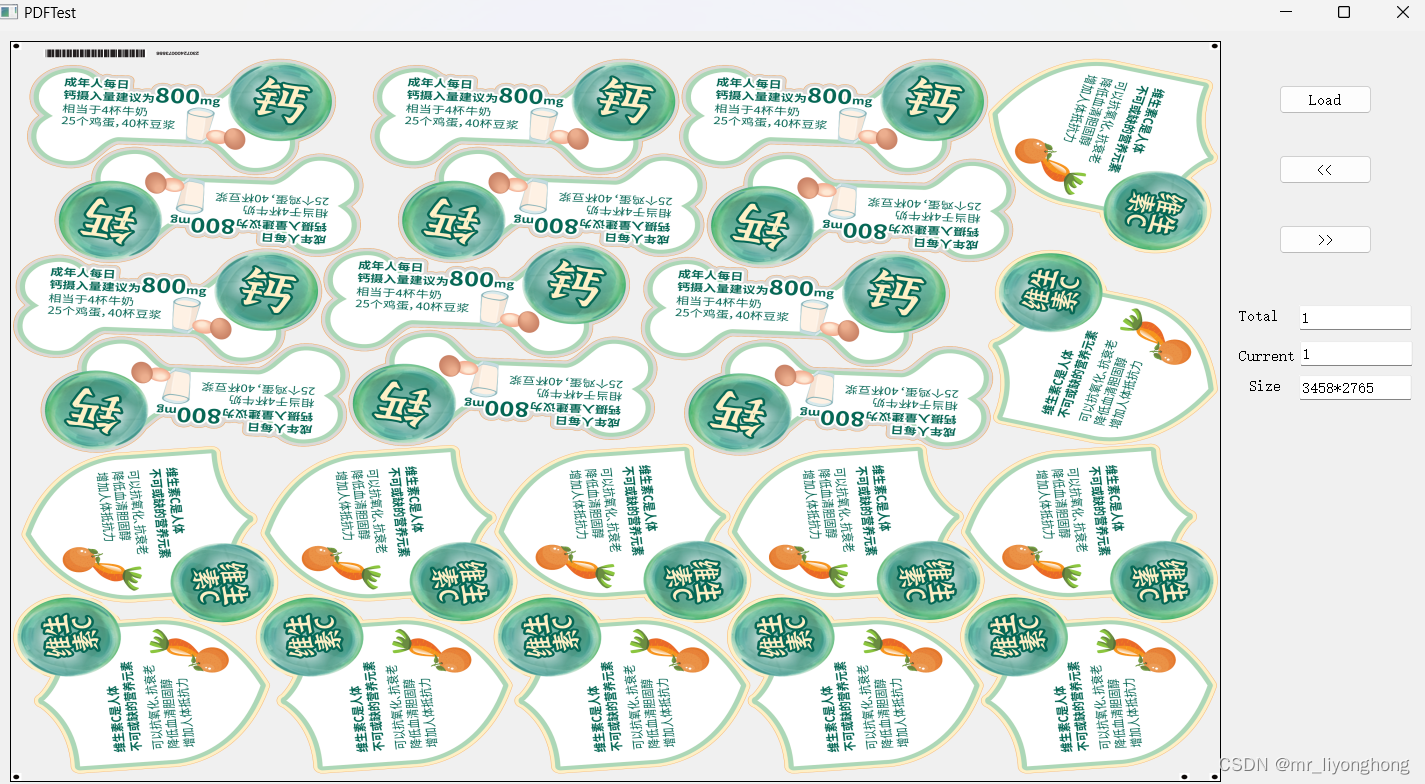
QT自带PDF库的使用
QT自带PDF库可以方便的打开PDF文件,并将文件解析为QImage,相比网上提供的开源库,QT自带PDF库使用更方便,也更加可靠,然而,QT自带PDF库的使用却不同于其他通用库的使用,具备一定的技巧。 1. 安装…...
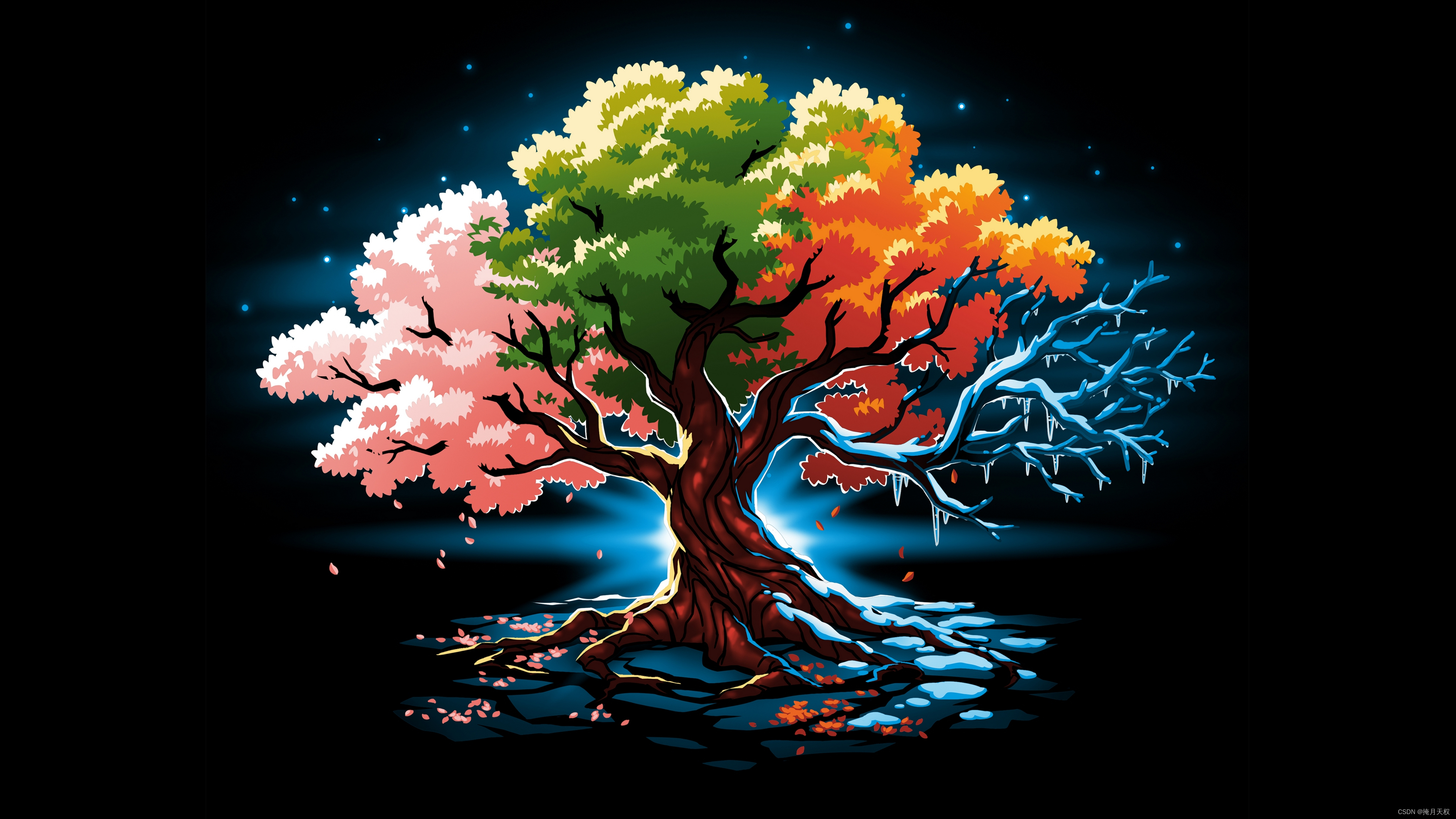
SQL | 排序检索的数据
3-排序检索的数据 使用order by语句排序检索到的数据。 3.1-排序数据 使用SQL语句返回一个数据表的列。 select prod_id from products; --------------------- | prod_name | --------------------- | 8 inch teddy bear | | 12 inch teddy bear | | 18 inch teddy bear |…...
8. yaml文件管理
文章目录 yaml文件管理编写yaml配置文件获取配置模板方法一方法二方法三方法四 yaml文件管理 Kubernetes 支持 YAML 和 JSON 格式管理资源对象 JSON 格式:主要用于 api 接口之间消息的传递YAML 格式:用于配置和管理,YAML 是一种简洁的非标记性…...
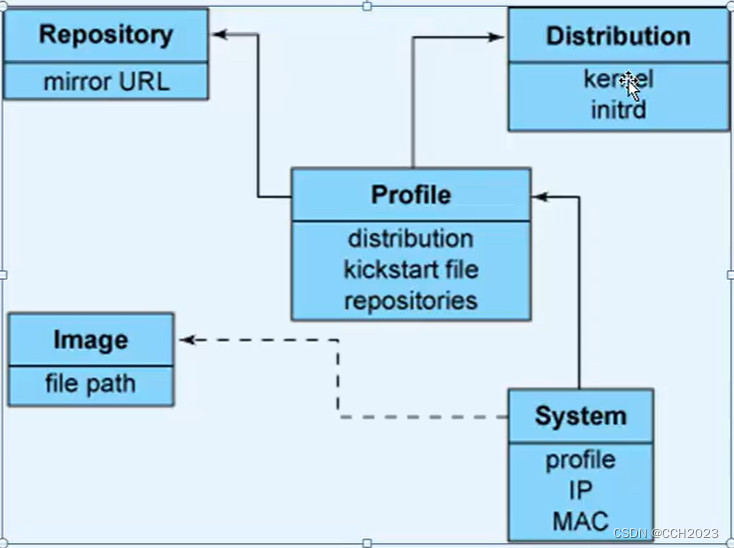
Cobbler自定义yum源
再次了解下Cobbler的目录结构: 在/var/www/cobbler/ks_mirror目录下存放的是所有的镜像。 存放的是仓库镜像: 在/var/lib/cobbler/kickstarts目录下是存放的所有的kickstarts文件。 再有就是/etc/cobbler这个目录: [rootvm1 loaders]# cd /…...
《算法竞赛·快冲300题》每日一题:“特殊数字”
《算法竞赛快冲300题》将于2024年出版,是《算法竞赛》的辅助练习册。 所有题目放在自建的OJ New Online Judge。 用C/C、Java、Python三种语言给出代码,以中低档题为主,适合入门、进阶。 文章目录 题目描述题解C代码Java代码Python代码 “ 特…...
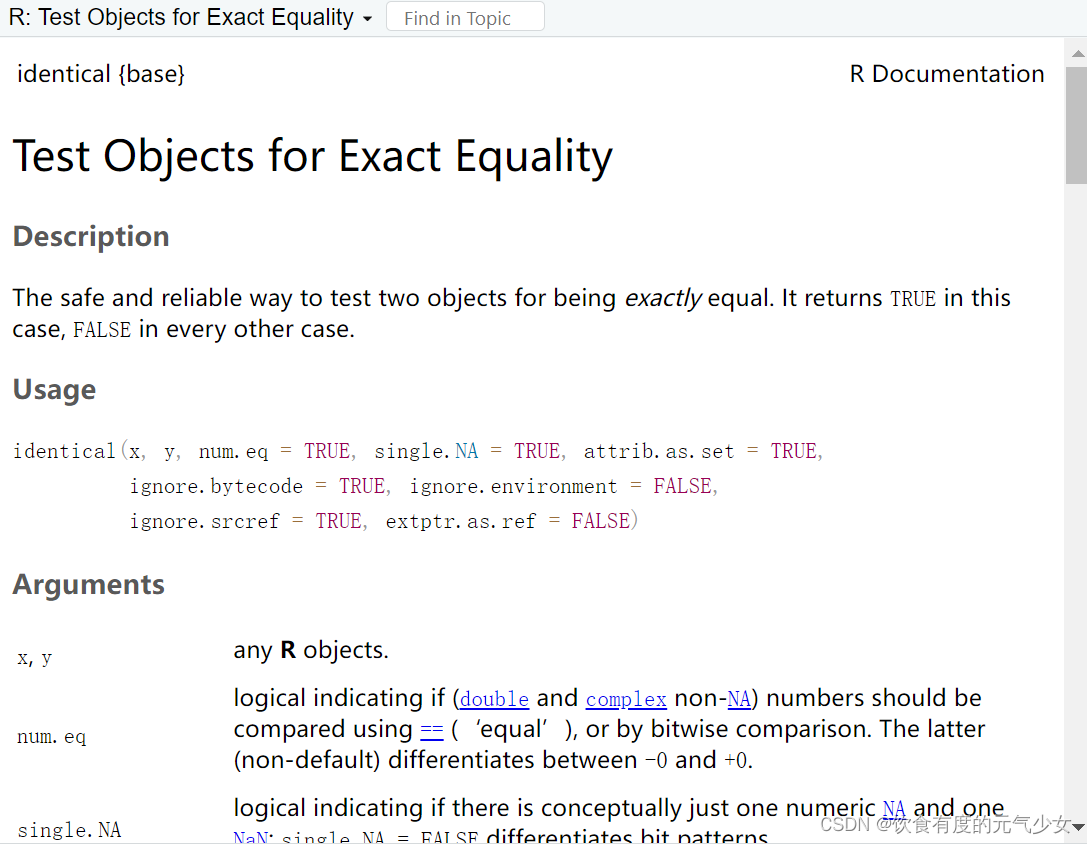
在R中比较两个矩阵是否相等
目录 方法一:使用all.equal()比较两个R对象是否近似相等 方法二:使用identical比较两个R对象是否精确相等。 方法一:使用all.equal()比较两个R对象是否近似相等 使用函数:all.equal(x,y) 比较两个R对象x和y是否近似相等 > M1…...
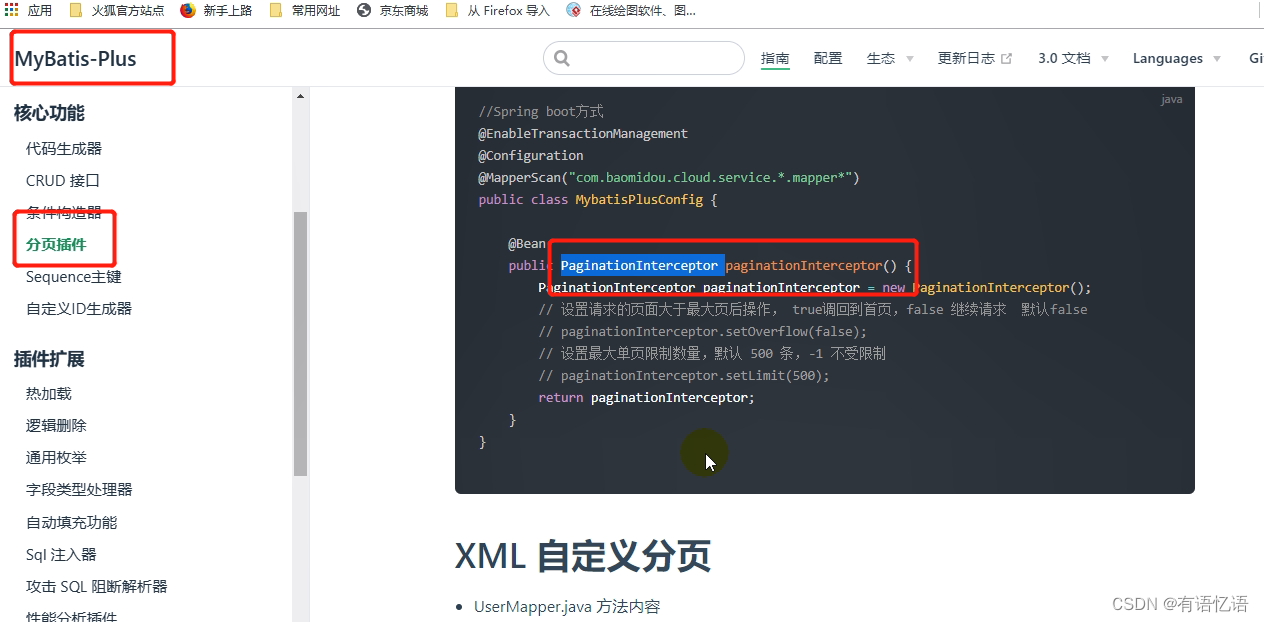
商城-学习整理-基础-商品服务API-属性分组(七)
目录 一、创建系统菜单二、开发商品系统-平台属性-属性分组1、将三级分类功能抽取出来2、编写后端代码3、属性分组新增功能4、属性分组修改回显功能 三、商品系统-平台属性-规则参数1、列表展示页面2、新增规格参数页面 四、商品系统-平台属性-销售属性1、列表展示页面2、新增或…...

什么是响应式设计?列举几种实现响应式设计的方法。
聚沙成塔每天进步一点点 ⭐ 专栏简介⭐ 什么是响应式设计?⭐ 实现响应式设计的方法⭐ 写在最后 ⭐ 专栏简介 前端入门之旅:探索Web开发的奇妙世界 记得点击上方或者右侧链接订阅本专栏哦 几何带你启航前端之旅 欢迎来到前端入门之旅!这个专栏…...
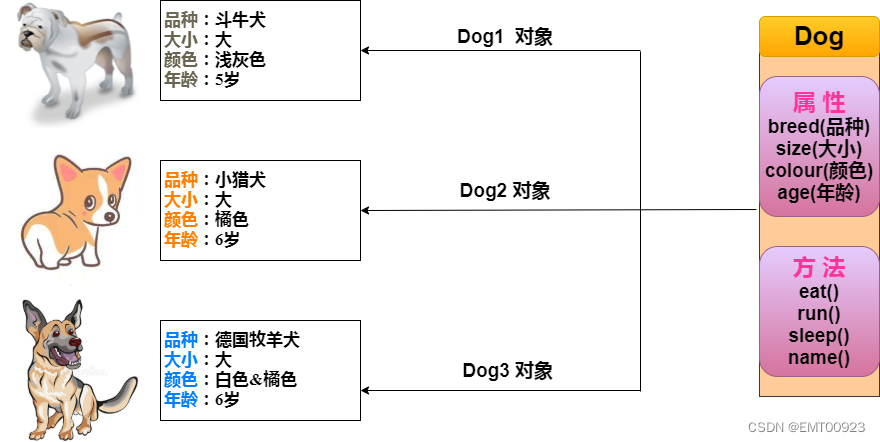
Java类和对象(一文读懂)
文章目录 类、对象是什么?创建类构造器 创建对象 类、对象是什么? 类:类是一个模板,它描述一类对象的行为和状态。类可以看成是创建 Java 对象的模板。 对象:对象是类的一个实例(对象不是找个女朋友&#x…...
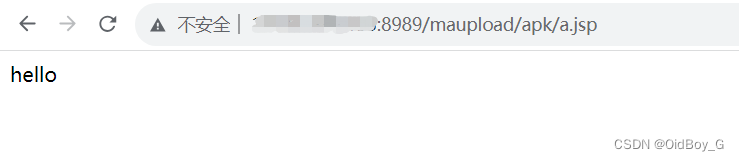
用友移动管理系统 任意文件上传漏洞复现(HW0day)
0x01 产品简介 用友移动系统管理是用友公司推出的一款移动办公解决方案,旨在帮助企业实现移动办公、提高管理效率和员工工作灵活性。它提供了一系列功能和工具,方便用户在移动设备上管理和处理企业的系统和业务。 0x02 漏洞概述 用友移动管理系统 uploa…...

启动springboot,出现Unable to start embedded Tomcat
报错信息 org.apache.catalina.core.ContainerBase : A child container failed during startjava.util.concurrent.ExecutionException: org.apache.catalina.LifecycleException: Failed to start component [StandardEngine[Tomcat].StandardHost[localhost].TomcatEmbedd…...
加密和安全
加密和安全 一.安全机制 安全攻击的几种典型方式: STRIDE Spoofing 假冒 Tampering 篡改 Repudiation 否认 Information Disclosure 信息泄漏 Denial of Service 拒绝服务 Elevation of Privilege 提升…...
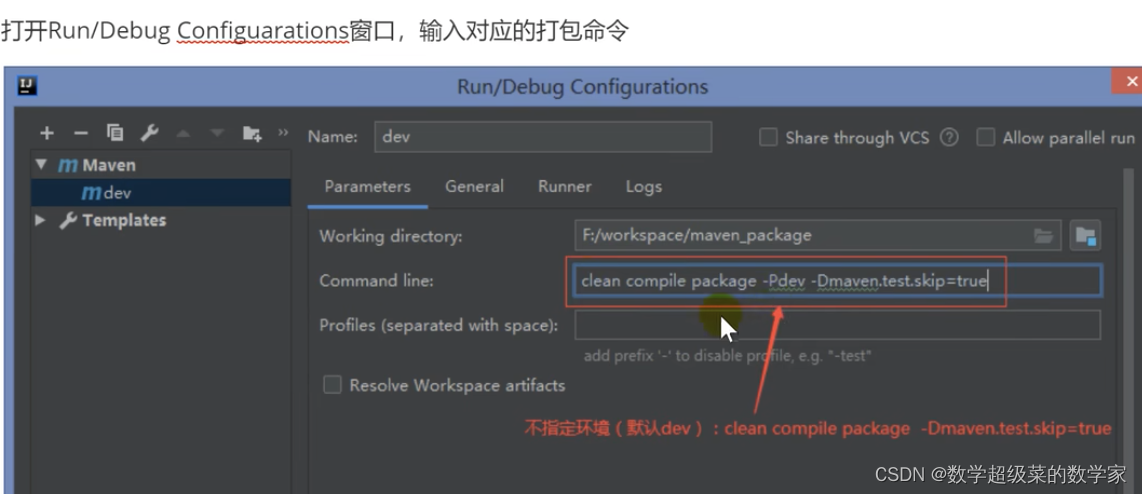
Maven基础总结
前言 Maven 是一个项目管理工具,可以对 Java 项目进行构建、依赖管理。 基本要求掌握 配置Maven环境直接查。 得会在IDEA创建Maven的java项目吧、会创建Maven的web项目吧、会创建多模块项目吧。 得会配置插件pligin、依赖dependency吧 一、Maven四大特性 1、…...
Java 编程实战:如何用 Java 编写一个简单而强大的 Tomcat
学习完了JavaWeb,为了深入了解tomcat,打算手撕tomcat搭建自己的tomcat,希望对来访小伙伴也有帮助 引言 Tomcat 是一个开源的 Web 服务器和 Servlet 容器,它可以提供动态 Web 内容的处理和交互功能。Tomcat 是用 Java 语言编写的&a…...
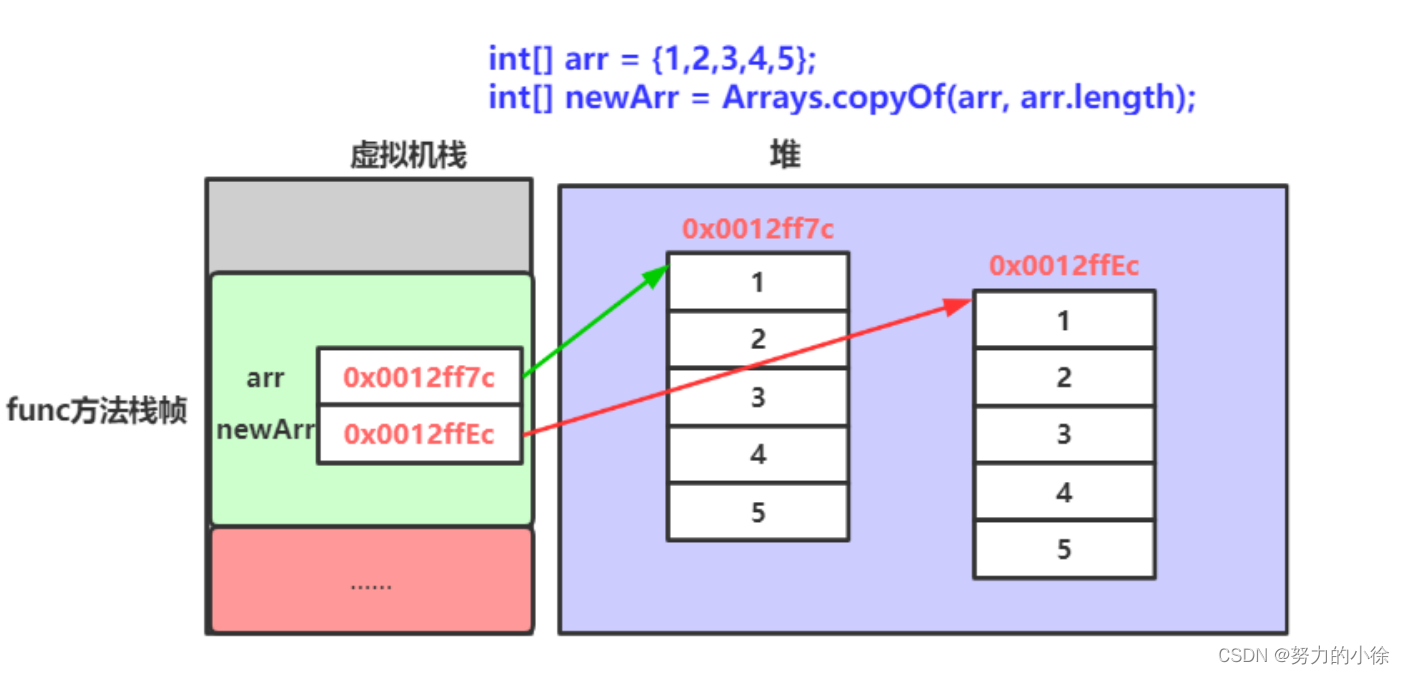
【JavaSE】数组的定义与使用
详解数组 数组的基本概念什么是数组数组的创建及初始化数组的使用 数组是引用类型基本类型变量与引用类型变量的区别引用变量认识 null 数组的应用场景数组练习二维数组 数组的基本概念 什么是数组 数组可以看成是相同类型元素的一个集合。在内存中是一段连续的空间。比如现实…...

银河麒麟安装php7.1.33
银河麒麟V10兼容CentOS 8 安装过程与CentOS类似。 TencentOS3.1安装PHPNginxredis测试系统_乐大师的博客-CSDN博客 可以参考之前我写的文章。 不过有2个细节不同,下面说下。 问题1:编译错误提示“error:off_t undefined” 解决方法: 编…...
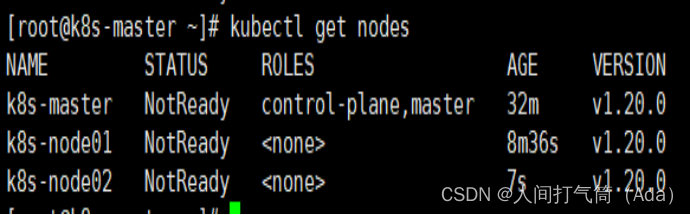
Kubernetes集群部署上篇(安装部署,但是集群网络未部署)
第四阶段 时 间:2023年8月9日 参加人:全班人员 内 容: Kubernetes集群部署上篇 目录 一、Kubernetes部署方式 (一)minikube (二)二进制包 (三)Kubeadm Kubea…...
跨境电商中的安全挑战与隐擎Fox指纹浏览器的应用
随着全球互联网的蓬勃发展,跨境电商已经成为了国际贸易的重要组成部分。然而,跨境电商的迅速崛起也伴随着一系列安全挑战,其中之一就是恶意活动和隐私泄露。为了应对这些挑战,诸多技术手段被开发出来,其中隐擎Fox指纹浏…...

10. Docker Swarm(一)
目录 1、前言 2、Docker Swarm体系架构 2.1、简单介绍 2.2、体系架构 3、简单使用 3.1、环境准备 3.2、初始化master节点 3.3、建立worker节点 3.4、查看集群的节点信息 3.5、部署应用 3.5.1、创建Dockerfile文件 3.5.2、构建镜像 3.5.3、将镜像上传到Docker仓库 …...
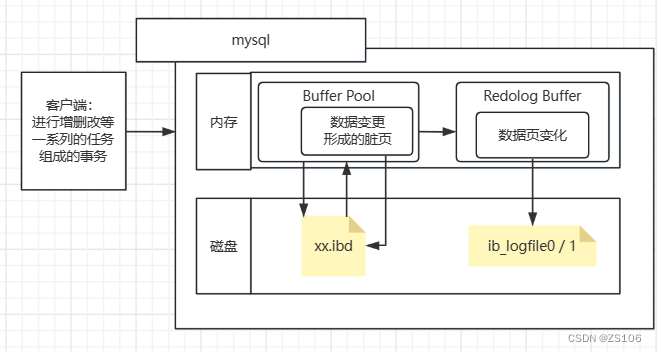
【MySQL】InnoDB存储引擎详解
InnoDB引擎是MySQL5.5版本之后默认的存储引擎 逻辑存储结构 首先是表空间Tablespace(ibd文件):一个mysql实力可以对应多个表空间,用于存储及记录,索引等数据 这些存储记录,索引等数据中是用段(Segment)来…...
组合求和-矩阵连乘所有加括号方式_2023_08_12
矩阵链加括号方式总数 前言 矩阵链乘积的瓶颈在于其标量运算的次数,不同的结合次序对其时间性能影响远大于矩阵乘积运算本身,可以看到许多教材上把求解矩阵标量运算的最优解作为动态规划的示例,问题隐含动态规划两大特征: 最优子…...
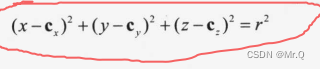
《3D 数学基础》12 几何图元
目录 1 表达图元的方法 1.1 隐式表示法 1.2 参数表示 1.3 直接表示 2. 直线和射线 2.1 射线的不同表示法 2.1.1 两点表示 2.1.2 参数表示 2.1.3 相互转换 2.2 直线的不同表示法 2.2.1 隐式表示法 2.2.2 斜截式 2.2.3 相互转换 3. 球 3.1 隐式表示 1 表达图元的方…...
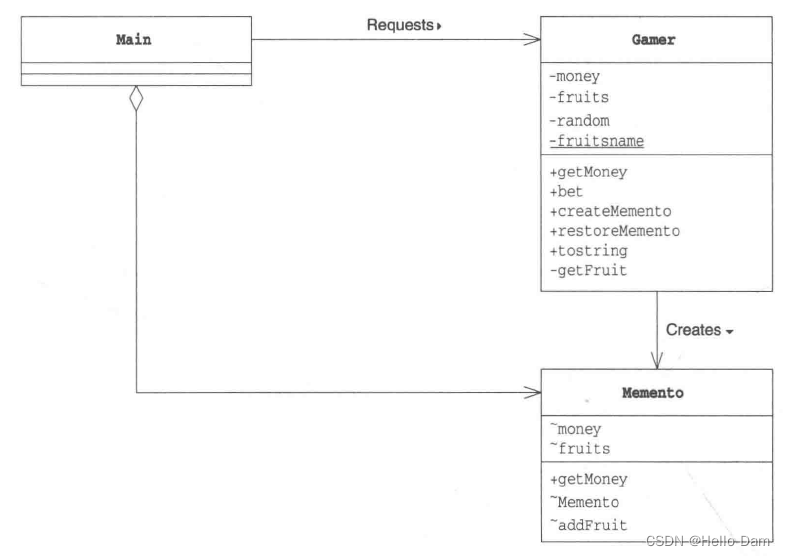
【设计模式——学习笔记】23种设计模式——备忘录模式Memento(原理讲解+应用场景介绍+案例介绍+Java代码实现)
案例引入 游戏角色有攻击力和防御力,在大战Boss前保存自身的状态(攻击力和防御力),当大战Boss后攻击力和防御力下降,可以从备忘录对象恢复到大战前的状态 传统设计方案 针对每一种角色,设计一个类来存储该角色的状态 【分析】…...
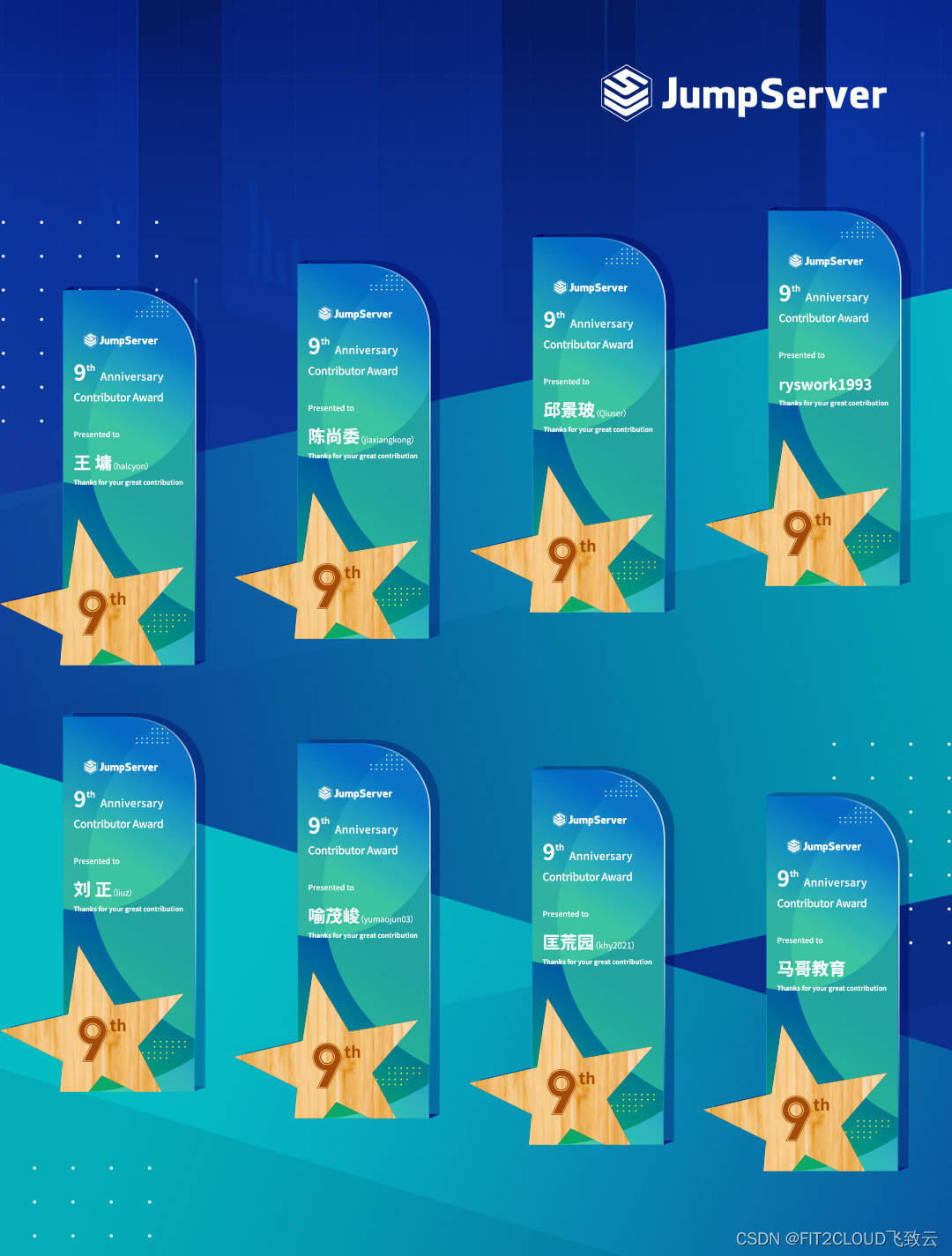
致谢丨感谢有你,JumpServer开源项目九周年致谢名单
2014年到2023年,JumpServer开源项目已经走过了九年的时间。感谢以下社区贡献者对JumpServer项目的帮助和支持。 因为有你,一切才能成真。 JumpServer开源项目贡献者奖杯将于近日邮寄到以上贡献者手中,同时JumpServer开源项目组还准备了一份小…...
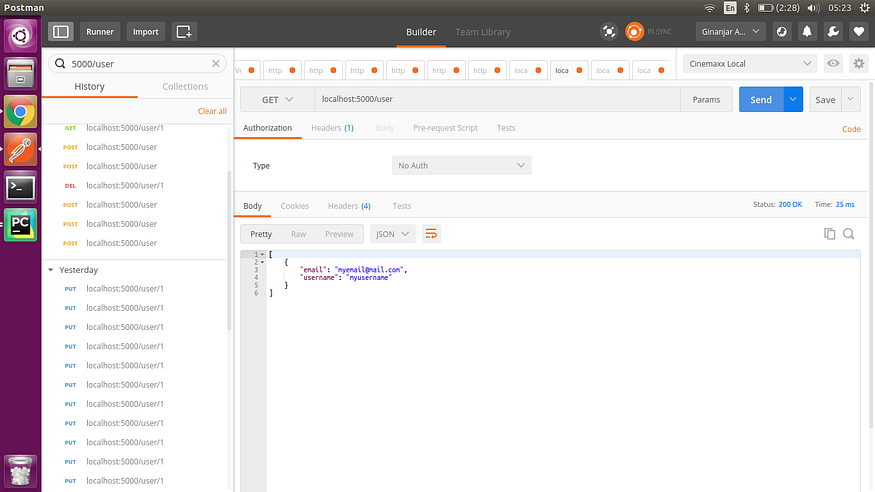
使用 Python 和 Flask 构建简单的 Restful API 第 1 部分
一、说明 我将把这个系列分成 3 或 4 篇文章。在本系列的最后,您将了解使用flask构建 restful API 是多么容易。在本文中,我们将设置环境并创建将显示“Hello World”的终结点。 我假设你的电脑上安装了python 2.7和pip。我已经在python 2.7上测试了本文…...
【深度学习所有损失函数】在 NumPy、TensorFlow 和 PyTorch 中实现(2/2)
一、说明 在本文中,讨论了深度学习中使用的所有常见损失函数,并在NumPy,PyTorch和TensorFlow中实现了它们。 (二-五)见 六、稀疏分类交叉熵损失 稀疏分类交叉熵损失类似于分类交叉熵损失,但在真实标签作为整数而不是独热编码提…...