【文生图】Stable Diffusion XL 1.0模型Full Fine-tuning指南(U-Net全参微调)
文章目录
- 前言
- 重要教程链接
- 以海报生成微调为例
- 总体流程
- 数据获取
- POSTER-TEXT
- AutoPoster
- CGL-Dataset
- PKU PosterLayout
- PosterT80K
- Movie & TV Series & Anime Posters
- 数据清洗与标注
- 模型训练
- 模型评估
- 生成图片样例
- 宠物包商品海报
- 护肤精华商品海报
- 一些Tips
- Mata:EMU(Expressive Media Universe)
- ideogram
- DALL-E3
- 关于模型优化
- Examples of Commonly Used Negative Prompts:
前言
Stable Diffusion是计算机视觉领域的一个生成式大模型,能够进行文生图(txt2img)和图生图(img2img)等图像生成任务。Stable Diffusion的开源公布,以及随之而来的一系列借助Stable Diffusion为基础的工作使得人工智能绘画领域呈现出前所未有的高品质创作与创意。
今年7月Stability AI 正式推出了 Stable Diffusion XL(SDXL)1.0,这是当前图像生成领域最好的开源模型。文生图模型又完成了进化过程中的一次重要迭代,SDXL 1.0几乎能够生成任何艺术风格的高质量图像,并且是实现逼真效果的最佳开源模型。该模型在色彩的鲜艳度和准确度方面做了很好的调整,对比度、光线和阴影都比上一代更好,并全部采用原生1024x1024分辨率。除此之外,SDXL 1.0 对于难以生成的概念有了很大改善,例如手、文本以及空间的排列。
目前关于文生图(text2img)模型的训练教程多集中在LoRA、DreamBooth、Text Inversion等模型,且训练方式大多也依赖于可视化UI界面工具,如SD WebUI、AI 绘画一键启动软件等等。而Full Fine-tuning的详细教程可以说几乎没有,所以这里记录一下我在微调SDXL Base模型过程中所参考的资料,以及一些训练参数的说明。
重要教程链接
- 主要参考:训练流程解读https://zhuanlan.zhihu.com/p/643420260——知乎
- SDXL原理详解:https://zhuanlan.zhihu.com/p/650717774
- 代码库:https://github.com/qaneel/kohya-trainer
- SDXL训练说明:https://github.com/kohya-ss/sd-scripts/blob/main/README.md#sdxl-training
- 微调Stable Diffusion模型例子:https://keras.io/examples/generative/finetune_stable_diffusion/
- huggingface官方基于Diffusers微调SDXL代码库:
https://github.com/huggingface/diffusers/blob/main/examples/text_to_image/README_sdxl.md
以海报生成微调为例
总体流程
数据获取
使用科研机构、公司以及Kaggle平台的公开数据集,具体如下:
POSTER-TEXT
数据集POSTER-TEXT是关于电商海报图片的文本图像生成任务,它包含114,009条记录,由阿里巴巴集团提供。包括原始海报图及擦除海报图中文字后的图片。
Paper:TextPainter: Multimodal Text Image Generation with Visual-harmony and Text-comprehension for Poster Design ACM MM 2023.
Source:https://tianchi.aliyun.com/dataset/160034
AutoPoster
数据集AutoPoster-Dataset是关于电商海报图片的自动化生成任务,它包含 76000 条记录,由阿里巴巴集团提供。
在一些图像中存在重复标注的问题。该论文提到训练集中有69,249张图像,测试集中有7,711张图像。但实际上,在去除重复数据后,训练集中有68,866张唯一的广告海报图像,测试集中有7,671张唯一的图像。
Paper: AutoPoster: A Highly Automatic and Content-aware Design System for Advertising Poster Generation ACM MM 2023
Source:https://tianchi.aliyun.com/dataset/159829
CGL-Dataset
Paper: Composition-aware Graphic Layout GAN for Visual-textual Presentation Designs IJCAI 2022
Github: https://github.com/minzhouGithub/CGL-GAN
Source:https://tianchi.aliyun.com/dataset/142692
PKU PosterLayout
作为第一个包含复杂布局的公共数据集,它在建模布局内关系方面提供了更多的困难,并代表了需要复杂布局的扩展任务。包含9,974个训练图片和905个测试图片。
- 领域多样性
从多个来源收集了数据,包括电子商务海报数据集和多个图片库网站。图像在域、质量和分辨率方面都是多样化的,这导致了数据分布的变化,并使数据集更加通用。 - 内容多样性
定义了九个类别,涵盖大多数产品,包括食品/饮料、化妆品/配件、电子产品/办公用品、玩具/仪器、服装、运动/交通、杂货、电器/装饰和新鲜农产品。
Paper: A New Dataset and Benchmark for Content-aware Visual-Textual Presentation Layout CVPR 2023
Github: https://github.com/PKU-ICST-MIPL/PosterLayout-CVPR2023
Source:http://59.108.48.34/tiki/PosterLayout/
PosterT80K
电商海报图片,但是数据未公开,单位为中科大和阿里巴巴
Paper: TextPainter: Multimodal Text Image Generation with
Visual-harmony and Text-comprehension for Poster Design ACM MM 2023
Source:None
Movie & TV Series & Anime Posters
Kaggle上的公开数据,需要从提供的csv或json文件中的图片url地址自己写个下载脚本。\
Source:
- https://www.kaggle.com/datasets/bourdier/all-tv-series-details-dataset
file prefix: https://www.themoviedb.org/t/p/w600_and_h900_bestv2/xx.jpg - https://www.kaggle.com/datasets/crawlfeeds/movies-and-tv-shows-dataset
- https://www.kaggle.com/datasets/phiitm/movie-posters
- https://www.kaggle.com/datasets/ostamand/tmdb-box-office-prediction-posters
- https://www.kaggle.com/datasets/dbdmobile/myanimelist-dataset
- https://www.kaggle.com/zakarihachemi/datasets
- https://www.kaggle.com/datasets/rezaunderfit/48k-imdb-movies-data
以第一个数据源为例:
import csv
import os
import requests
import warnings
warnings.filterwarnings('ignore')csv_file = r"C:\Users\xxx\Downloads\tvs.csv"
url_prefix = 'https://www.themoviedb.org/t/p/w600_and_h900_bestv2'
save_root_path = r"D:\dataset\download_data\tv_series"def parse_csv(path):cnt = 0s = requests.Session()s.verify = False # 全局关闭ssl验证with open(path, 'r', encoding='utf-8') as f:reader = csv.DictReader(f)for row in reader:raw_img_url = row['poster_path'] # url itemimg_url = url_prefix + raw_img_urlif raw_img_url == '':continuetry:img_file = s.get(img_url, verify=False)except Exception as e:print(repr(e))print("错误的状态响应码:{}".format(img_file.status_code))if img_file.status_code == 200:img_name = raw_img_url.split('/')[-1]# img_name = row['url'].split('/')[-2] + '.jpg'save_path = os.path.join(save_root_path, img_name)with open(save_path, 'wb') as img:img.write(img_file.content)cnt += 1print(cnt, 'saved!')print("Done!")if __name__ == '__main__':if not os.path.exists(save_root_path):os.makedirs(save_root_path)parse_csv(csv_file)
数据清洗与标注
数据筛选标准:低于512和超过1024的去除,文件大小/分辨率<0.0005的去除,dpi小于96的去除。这里的0.0005是根据SD所生成图片的文件大小(kb)和分辨率所确定的一个主观参数标准,用于保证图片质量。统计八张SD所生成图片在该指标下的数值如下:
SD生成的文件大小/图像分辨率:0.00129, 0.0012, 0.0011, 0.00136, 0.0014, 0.0015, 0.0013, 0.00149
图片标注:使用BLIP和Waifu模型自动标注,上文给出的那个知乎链接中有详细的说明,这里不做赘述。
模型训练
- 模型准备:
SDXL 1.0 的 vea 由于数字水印的问题生成的图像会有彩色条纹的伪影存在,可以使用 0.9 的 vae 文件解决这个问题。这里建议使用整合好的Base模型(链接),HuggingFace官方也有相关的说明如下:
SDXL’s VAE is known to suffer from numerical instability issues. This is why we also expose a CLI argument namely --pretrained_vae_model_name_or_path that lets you specify the location of a better VAE (such as this one).
- 部分训练参数说明
- mixed_precision: 是否使用混合精度,根据unet模型决定,[“no”, “fp16”, “bf16”]
- save_percision: 保存模型精度,[float, fp16, bf16], "float"表示torch.float32
- vae: 训练和推理的所使用的指定vae模型
- keep_tokens: 训练中会将tags随机打乱,如果设置为n,则前n个tags的顺序不会被打乱
- shuffle_caption:bool,打乱标签,可增强模型泛化性
- sdxl_train_util.py的
_load_target_model()
判断是否从单个safetensor读取模型,有修改StableDiffusionXLPipeline读取模型的代码 - sample_every_n_steps: 间隔多少训练迭代次数推理一次
- noise_offset: 避免生成图像的平均亮度值为0.5,对于logo、立体裁切图像和自然光亮与黑暗场景图片生成有很大提升
- optimizer_args: 优化器的额外设定参数,比如weight_decay和betas等等,在train_util.py中定义。
- clip_threshold: AdaFactor优化器的参数,在
optimizer_args
里新增该参数,默认值1.0。参考:https://huggingface.co/docs/transformers/main_classes/optimizer_schedules - gradient checkpointing:用额外的计算时间换取GPU内存,使得有限的GPU显存下训练更大的模型
- lr_scheduler: 设置学习率变化规则,如linear,cosine,constant_with_warmup
- lr_scheduler_args: 设置该规则下的具体参数,可参考pytorch文档
模型评估
目前,AIGC领域的测评过程整体上还是比较主观,但这里还是通过美学评分(Aesthetics)和CLIP score指标来分别衡量生成的图片质量与文图匹配度。评测代码基于GhostMix的作者开发的GhostReview,笔者仅取其中的一部分并做了一些优化,请结合着原作者的代码理解,具体代码如下:
import numpy as np
import torch
import pytorch_lightning as pl
import torch.nn as nn
import clip
import os
import torch.nn.functional as F
import pandas as pd
from PIL import Image
import scipyclass MLP(pl.LightningModule):def __init__(self, input_size, xcol='emb', ycol='avg_rating'):super().__init__()self.input_size = input_sizeself.xcol = xcolself.ycol = ycolself.layers = nn.Sequential(nn.Linear(self.input_size, 1024),# nn.ReLU(),nn.Dropout(0.2),nn.Linear(1024, 128),# nn.ReLU(),nn.Dropout(0.2),nn.Linear(128, 64),# nn.ReLU(),nn.Dropout(0.1),nn.Linear(64, 16),# nn.ReLU(),nn.Linear(16, 1))def forward(self, x):return self.layers(x)def training_step(self, batch, batch_idx):x = batch[self.xcol]y = batch[self.ycol].reshape(-1, 1)x_hat = self.layers(x)loss = F.mse_loss(x_hat, y)return lossdef validation_step(self, batch, batch_idx):x = batch[self.xcol]y = batch[self.ycol].reshape(-1, 1)x_hat = self.layers(x)loss = F.mse_loss(x_hat, y)return lossdef configure_optimizers(self):optimizer = torch.optim.Adam(self.parameters(), lr=1e-3)return optimizerdef normalized(a, axis=-1, order=2):l2 = np.atleast_1d(np.linalg.norm(a, order, axis))l2[l2 == 0] = 1return a / np.expand_dims(l2, axis)def PredictionLAION(image, laion_model, clip_model, clip_process, device='cpu'):image = clip_process(image).unsqueeze(0).to(device)with torch.no_grad():image_features = clip_model.encode_image(image)im_emb_arr = normalized(image_features.cpu().detach().numpy())prediction = laion_model(torch.from_numpy(im_emb_arr).to(device).type(torch.FloatTensor))return float(prediction)# ClipScore for 1 image
# 1张图片的ClipScore
def get_clip_score(image, text, clip_model, preprocess, device='cpu'):# Preprocess the image and tokenize the textimage_input = preprocess(image).unsqueeze(0)text_input = clip.tokenize([text], truncate=True)# Move the inputs to GPU if availableimage_input = image_input.to(device)text_input = text_input.to(device)# Generate embeddings for the image and textwith torch.no_grad():image_features = clip_model.encode_image(image_input)text_features = clip_model.encode_text(text_input)# Normalize the featuresimage_features = image_features / image_features.norm(dim=-1, keepdim=True)text_features = text_features / text_features.norm(dim=-1, keepdim=True)# Calculate the cosine similarity to get the CLIP scoreclip_score = torch.matmul(image_features, text_features.T).item()return clip_scoreif __name__ == '__main__':# 读取图片路径ImgRoot = './Image/ImageRating'DataFramePath = './dataresult/MyImageRating' # all prompts results of each modelModelSummaryFile = './ImageRatingSummary/MyModelSummary_Total.csv'PromptsFolder = os.listdir(ImgRoot)if not os.path.exists(DataFramePath):os.makedirs(DataFramePath)# 读取图片对应的PromptsPromptDataFrame = pd.read_csv('./PromptsForReviews/mytest.csv')PromptsList = list(PromptDataFrame['Prompts'])# 载入评估模型device = "cuda" if torch.cuda.is_available() else "cpu"MLP_Model = MLP(768) # CLIP embedding dim is 768 for CLIP ViT L 14# load LAION aesthetics modelstate_dict = torch.load("./models/sac+logos+ava1-l14-linearMSE.pth", map_location=torch.device(device))MLP_Model.load_state_dict(state_dict)MLP_Model.to(device)MLP_Model.eval()# Load the pre-trained CLIP model and the imageCLIP_Model, CLIP_Preprocess = clip.load('ViT-L/14', device=device, download_root='./models/clip') # RN50x64CLIP_Model.to(device)CLIP_Model.eval()# 跳过已经做过的Promptstry:DataSummaryDone = pd.read_csv(ModelSummaryFile)PromptsNotDone = [i for i in PromptsFolder if i not in list(DataSummaryDone['Model'])]except:DataSummaryDone = pd.DataFrame()PromptsNotDone = [i for i in PromptsFolder]if not PromptsNotDone:import syssys.exit("There are no models to analyze.")for i, name in enumerate(PromptsNotDone):FolderPath = os.path.join(ImgRoot, str(name))ImageInFolder = os.listdir(FolderPath)DataCollect = pd.DataFrame()for j, img in enumerate(ImageInFolder):prompt_index = int(img.split('-')[1])txt = PromptsList[prompt_index]ImagePath = os.path.join(FolderPath, img)Img = Image.open(ImagePath)# ClipscoreImgClipScore = get_clip_score(Img, txt, CLIP_Model, CLIP_Preprocess, device)# aesthetics scorer# ImageScore = predict(Img)# LAION aesthetics scorerImageLAIONScore = PredictionLAION(Img, MLP_Model, CLIP_Model, CLIP_Preprocess, device)# temp = list(ImageScore)temp = list()temp.append(float(ImgClipScore))temp.append(ImageLAIONScore)temp = pd.DataFrame(temp)DataCollect = pd.concat([DataCollect, temp], axis=1)print("Model:{}/{}, image:{}/{}".format(i+1, len(PromptsNotDone), j+1, len(ImageInFolder)))DataCollect = DataCollect.TDataCollect['ImageIndex'] = [i + 1 for i in range(len(ImageInFolder))]DataCollect.columns = ['ClipScore', 'LAIONScore', 'ImageIndex']# 保存原数据DataCollect.to_csv(os.path.join(DataFramePath, str(name) + '.csv'), index=False)print("One Results File Saved!")print('Image rating complete!')# do some calculationModelSummary = pd.DataFrame()for i in PromptsNotDone:DataCollect = pd.read_csv(os.path.join('dataresult/MyImageRating', str(i) + '.csv'))temp = pd.DataFrame(DataCollect['LAIONScore'].describe()).T# 计算数据的偏度temp['skew'] = scipy.stats.skew(DataCollect['LAIONScore'], axis=0, bias=True, nan_policy="propagate")# 计算数据的峰度temp['kurtosis'] = scipy.stats.kurtosis(DataCollect['LAIONScore'], axis=0, fisher=True, bias=True,nan_policy="propagate")temp.columns = [i + '_LAIONScore' for i in list(temp.columns)]# temp['RatingScore_mean']=np.mean(DataCollect['Rating'])# temp['RatingScore_std']=np.std(DataCollect['Rating'])temp['Clipscore_mean'] = np.mean(DataCollect['ClipScore'])temp['Clipscore_std'] = np.std(DataCollect['ClipScore'])# temp['Artifact_mean']=np.mean(DataCollect['Artifact'])# temp['Artifact_std']=np.std(DataCollect['Artifact'])temp['Model'] = str(i)ModelSummary = pd.concat([ModelSummary, temp], axis=0)# save resultsnew_order = ['Model', 'count_LAIONScore', 'mean_LAIONScore', 'std_LAIONScore','min_LAIONScore', '25%_LAIONScore', '50%_LAIONScore', '75%_LAIONScore','max_LAIONScore', 'skew_LAIONScore', 'kurtosis_LAIONScore','Clipscore_mean', 'Clipscore_std']# 使用 reindex() 方法重新排序列ModelSummary = ModelSummary.reindex(columns=new_order)DataSummaryDone = pd.concat([DataSummaryDone, ModelSummary], axis=0)DataSummaryDone.to_csv('./ImageRatingSummary/MyModelSummary_Total.csv')pd.set_option('display.max_rows', None) # None表示没有限制pd.set_option('display.max_columns', None) # None表示没有限制pd.set_option('display.width', 1000) # 设置宽度为1000字符print(DataSummaryDone)
下图给出了本文所训练的SDXL-Poster与主流文生图模型的比较结果,注意其中包括Anything模型开始往下的结果都是笔者自己调用相关模型生成的180张图片计算得来,所以标准差都偏大;而上方则是GhostReview作者调用这些模型生成960张图片的计算而来的结果。由于样本数量不一致,请读者谨慎参考。
生成图片样例
将本文训练的SDXL-Poster与SDXL-Base、CyberRealistic比较。
宠物包商品海报
A feline peering out from a striped transparent travel bag with a bicycle in the background. Outdoor setting, sunset ambiance. Product advertisement of pet bag, No humans, focus on cat and bag, vibrant colors, recreational theme
护肤精华商品海报
Four amber glass bottles with droppers placed side by side, arranged on a white background, skincare product promotion, no individuals present, still life setup
一些Tips
Mata:EMU(Expressive Media Universe)
简单文字,5秒出图。论文:https://arxiv.org/abs/2309.15807
知乎详细解读:https://zhuanlan.zhihu.com/p/659476603
介绍了EMU的训练方式:quality-tuning,一种有监督微调。其有三个关键:
- 微调数据集可以小得出奇,大约只有几千张图片;
- 数据集的质量非常高,这使得数据整理难以完全自动化,需要人工标注;
- 即使微调数据集很小,质量调整不仅能显著提高生成图片的美观度,而且不会牺牲通用性,因为通用性是根据输入提示的忠实度来衡量的。
- 基础的预训练大模型生成过程没有被引导为生成与微调数据集统计分布一致的图像,而quality-tuning能有效地限制输出图像与微调子集保持分布一致
- 分辨率低于1024x1024的图像使用pixel diffusion upsampler来提升分辨率
ideogram
生成图像中包含文字的生成模型:ideogram,2023年8月23日发布,免费,官网https://ideogram.ai/
DALL-E3
“文本渲染仍然不可靠,他们认为该模型很难将单词 token 映射为图像中的字母”
- 增强模型的prompt following能力,训练一个image captioner来生成更为准确详细的图像caption
- 合成的长caption能够提升模型性能,且与groud-truth caption混合比例为95%效果最佳。长caption通过GPT-4对人类的描述进行upsampling得到
关于模型优化
- 将训练出的base模型与类似的LoRA模型mix会好些
- base模型的作用就是多风格兼容,风格细化是LoRA做的事情
- SDXL在生成文字以及手时出现问题:https://zhuanlan.zhihu.com/p/649308666
- 微调迭代次数不能超过5k,否则导致明显的过拟合,降低视觉概念的通用性(来源:EMU模型tips)
Examples of Commonly Used Negative Prompts:
- Basic Negative Prompts: worst quality, normal quality, low quality, low res, blurry, text, watermark, logo, banner, extra digits, cropped, jpeg artifacts, signature, username, error, sketch, duplicate, ugly, monochrome, horror, geometry, mutation, disgusting.
- For Animated Characters: bad anatomy, bad hands, three hands, three legs, bad arms, missing legs, missing arms, poorly drawn face, bad face, fused face, cloned face, worst face, three crus, extra crus, fused crus, worst feet, three feet, fused feet, fused thigh, three thigh, fused thigh, extra thigh, worst thigh, missing fingers, extra fingers, ugly fingers, long fingers, horn, realistic photo, extra eyes, huge eyes, 2girl, amputation, disconnected limbs.
- For Realistic Characters: bad anatomy, bad hands, three hands, three legs, bad arms, missing legs, missing arms, poorly drawn face, bad face, fused face, cloned face, worst face, three crus, extra crus, fused crus, worst feet, three feet, fused feet, fused thigh, three thigh, fused thigh, extra thigh, worst thigh, missing fingers, extra fingers, ugly fingers, long fingers, horn, extra eyes, huge eyes, 2girl, amputation, disconnected limbs, cartoon, cg, 3d, unreal, animate.
- For Non-Adult Content: nsfw, nude, censored.
相关文章:
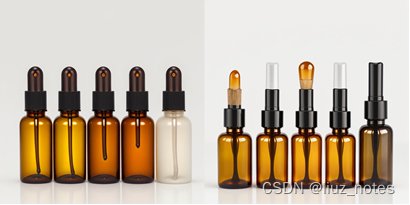
【文生图】Stable Diffusion XL 1.0模型Full Fine-tuning指南(U-Net全参微调)
文章目录 前言重要教程链接以海报生成微调为例总体流程数据获取POSTER-TEXTAutoPosterCGL-DatasetPKU PosterLayoutPosterT80KMovie & TV Series & Anime Posters 数据清洗与标注模型训练模型评估生成图片样例宠物包商品海报护肤精华商品海报 一些TipsMata:…...
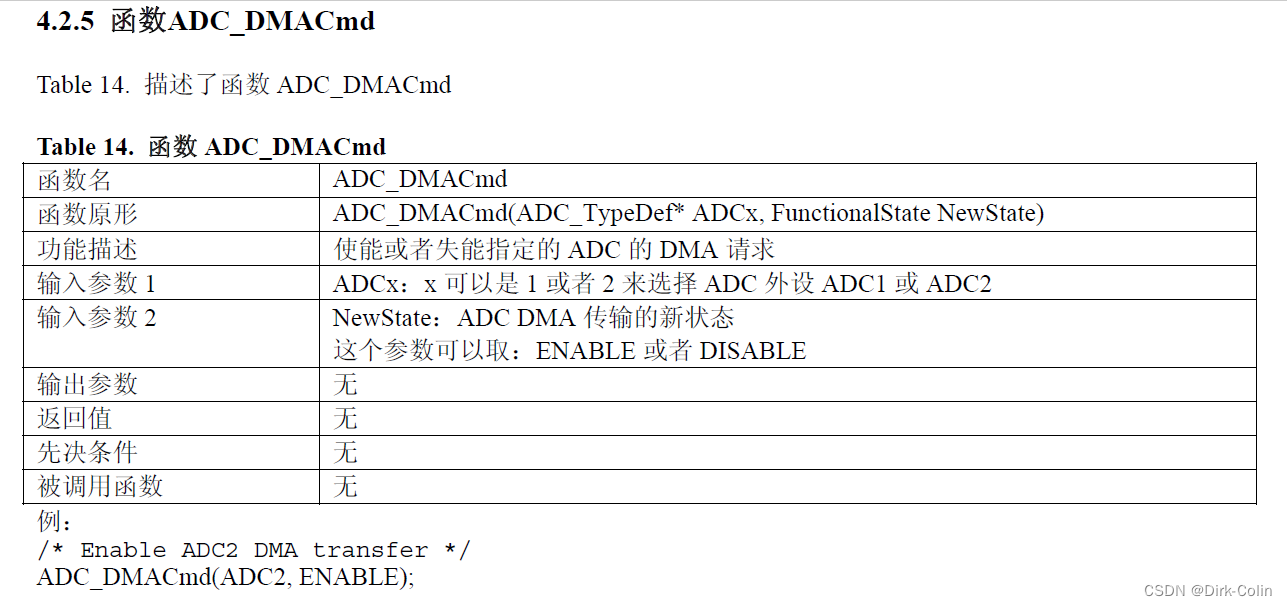
STM32笔记—DMA
目录 一、DMA简介 二、DMA主要特性 三、DMA框图 3.1 DMA处理 3.2 仲裁器 3.3 DMA通道 扩展: 断言: 枚举: 3.4 可编程的数据传输宽度、对齐方式和数据大小端 3.5 DMA请求映像 四、DMA基本结构 4.1 DMA_Init配置 4.2 实现DMAADC扫描模式 实现要求…...
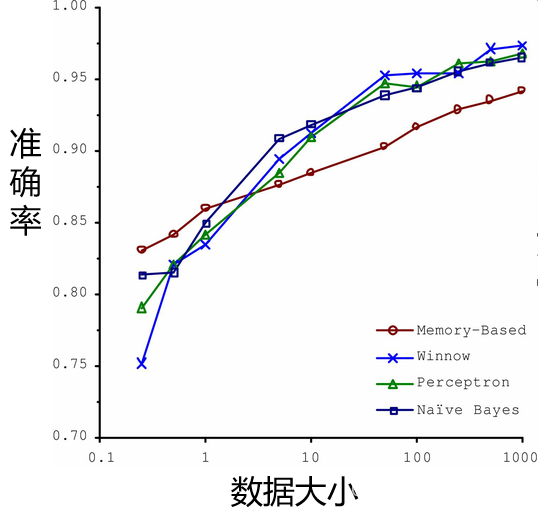
机器学习概论
一、机器学习概述 1、机器学习与人工智能、深度学习的关系 人工智能:机器展现的人类智能机器学习:计算机利用已有的数据(经验),得出了某种模型,并利用此模型预测未来的一种方法。深度学习:实现机器学习的一种技术 2…...
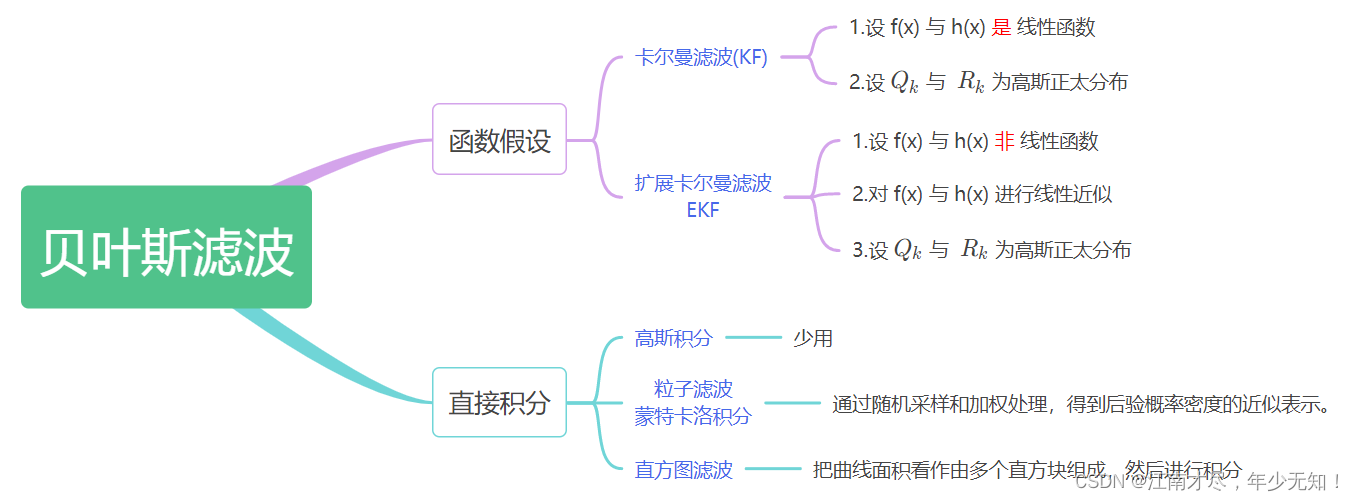
卡尔曼家族从零解剖-(04)贝叶斯滤波→细节讨论,逻辑梳理,批量优化
讲解关于slam一系列文章汇总链接:史上最全slam从零开始,针对于本栏目讲解的 卡尔曼家族从零解剖 链接 :卡尔曼家族从零解剖-(00)目录最新无死角讲解:https://blog.csdn.net/weixin_43013761/article/details/133846882 文末正下方中心提供了本人 联系…...
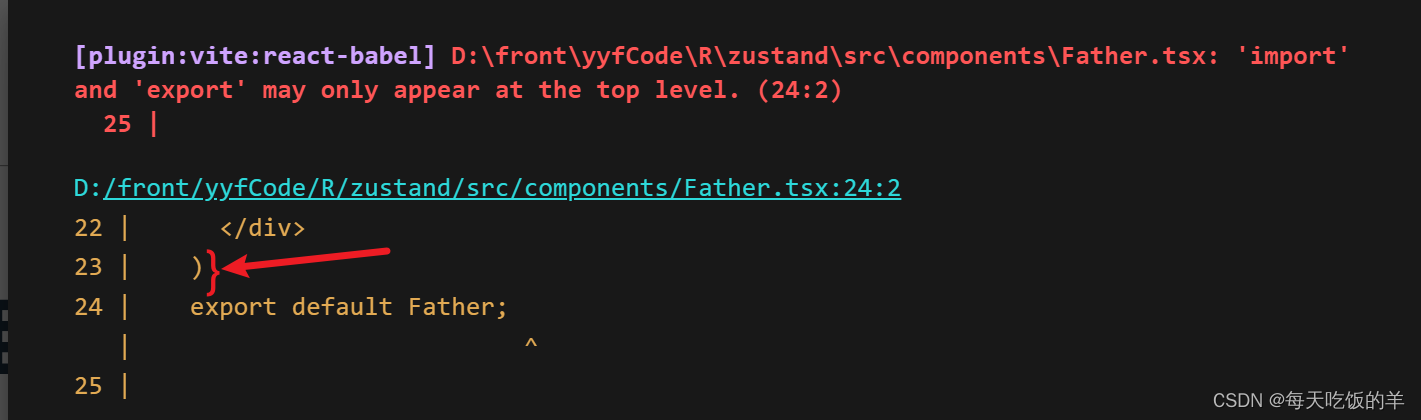
小菜React
1、Unterminated regular expression literal, 对于函数就写.ts,有dom元素就写.tsx 2、 The requested module /src/components/setup.tsx?t1699255799463 does not provide an export named Father export default useStore默认导出的钩子,组件引入的…...
新手用mac电脑,对文件的疑问和gpt回应
macOs系统安装软件的疑问 所有问题mac系统文件结构我用mac安装软件,不用像windows一样创建文件夹吗只能安装到Applications文件夹吗安装程序的指南和提供的安装选项是什么软件安装在Applications下的/appName文件夹,它的所有数据都会在该文件夹吗如果卸载…...
LeetCode|动态规划|392. 判断子序列、115. 不同的子序列、 583. 两个字符串的删除操作
目录 一、392. 判断子序列 1.题目描述 2.解题思路 3.代码实现(双指针解法) 4.代码实现(动态规划解法) 二、115. 不同的子序列 1.题目描述 2.解题思路 3.代码实现(C语言版本) 4.代码实现(C版本) …...
vscode 阅读 android以及kernel 源码
在Ubuntu系统中安装vscode 参考文档: https://blog.csdn.net/m0_57368670/article/details/127184424 1, 下载vscode https://code.visualstudio.com 2, 安装vscode $ sudo dpkg -i code_1.78.1-1683194560_amd64.deb 3, 打开vscode $ code vscode 阅读 android…...
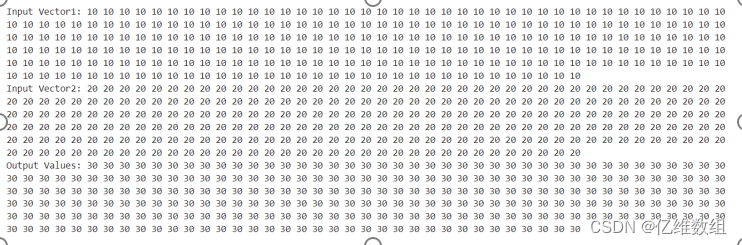
Intel oneAPI笔记(3)--jupyter官方文档(SYCL Program Structure)学习笔记
前言 本文是对jupyterlab中oneAPI_Essentials/02_SYCL_Program_Structure文档的学习记录,包含对Device Selector、Data Parallel Kernel、Host Accessor、Buffer Destruction、的介绍,最后还有一个小关于向量(Vector)加法的实例 …...
verilog——移位寄存器
在Verilog中,你可以使用移位寄存器来实现数据的移位操作。移位寄存器是一种常用的数字电路,用于将数据向左或向右移动一个或多个位置。这在数字信号处理、通信系统和其他应用中非常有用。以下是一个使用Verilog实现的简单移位寄存器的示例: m…...
C++11 多线程学习笔记
1. thread — 线程篇 所需头文件:<thread> 1.1 构造函数 // 1 默认构造函数 thread() noexcept; // 2 移动构造函数,把other的所有权转移给新的thread对象,之后 other 不再表示执行线程。 thread( thread&& other ) noex…...
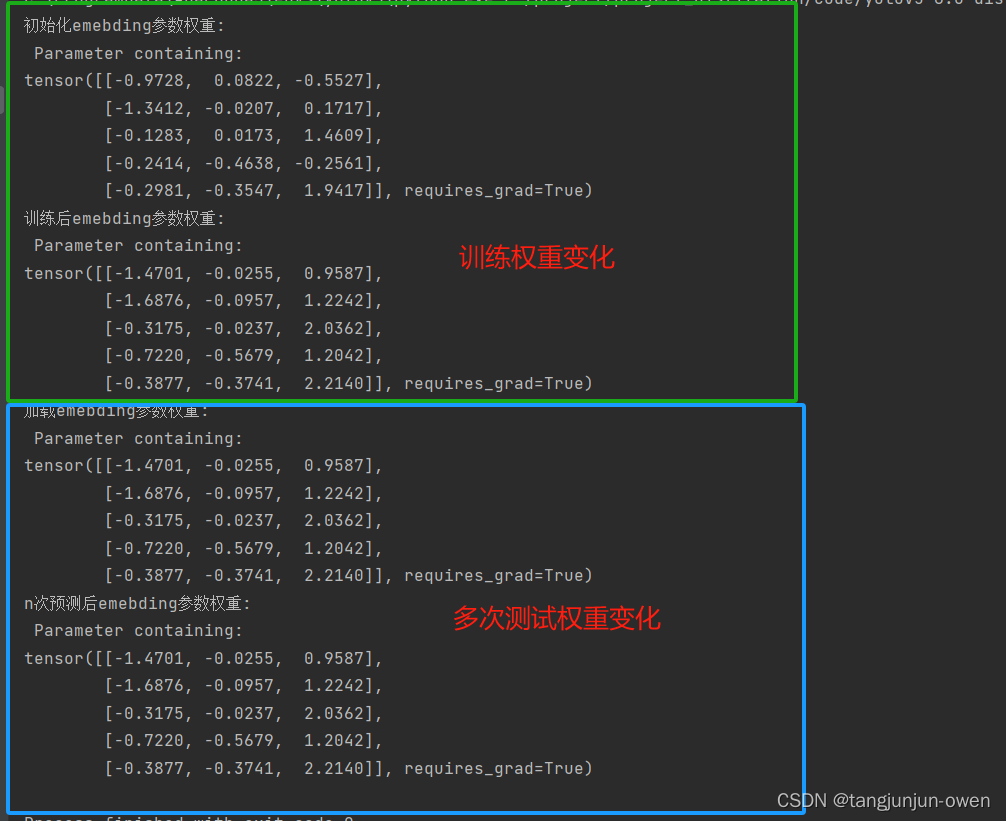
nn.embedding函数详解(pytorch)
提示:文章附有源码!!! 文章目录 前言一、nn.embedding函数解释二、nn.embedding函数使用方法四、模型训练与预测的权重变化探讨 前言 最近发现prompt工程(如sam模型),也有transform的detr模型等都使用了nn.Embedding函…...
gitee.com[0: xxx.xx.xxx.xx]: errno=Unknown error
git在提交或拉取代码的时候,遇到以下报错信息: Unable to connect to gitee.com[0: xxx.xx.xxx.xx]: errnoUnknown error 解决问题步骤: 1、找到自己的电脑上的git用户配置文件 文件位置位于:C:\Users\用户名\.gitconfig 比如我…...

bug: https://aip.baidubce.com/oauth/2.0/token报错blocked by CORS policy
还是跟以前一样,我们先看报错点:(注意小编这里是H5解决跨域的,不过解决跨域的原理都差不多) Access to XMLHttpRequest at https://aip.baidubce.com/oauth/2.0/token from origin http://localhost:8000 has been blo…...
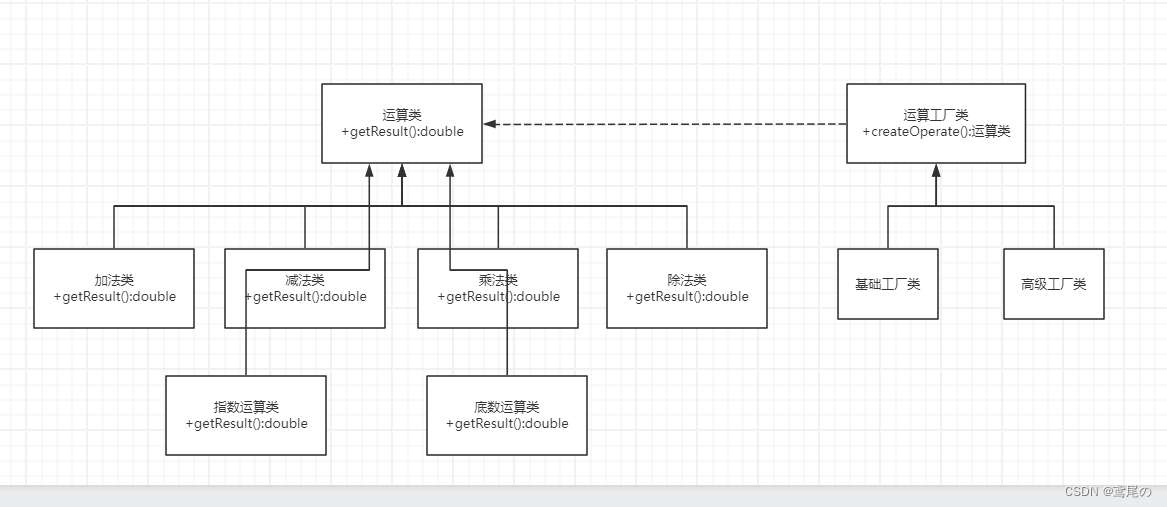
简单工厂VS工厂方法
工厂方法模式–制造细节无需知 前面介绍过简单工厂模式,简单工厂模式只是最基本的创建实例相关的设计模式。在真实情况下,有更多复杂的情况需要处理。简单工厂生成实例的类,知道了太多的细节,这就导致这个类很容易出现难维护、灵…...

使用VSCODE链接Anaconda
打代码还是在VSCODE里得劲 所以得想个办法在VSCODE里运行py文件 一开始在插件商店寻找插件 但是没有发现什么有效果的 幸运的是VSCODE支持自己选择Python的编译器 打开VSCODE 按住CtrlShiftP 输入Select Interpreter 如果电脑已经安装上了Python的环境 VSCODE会默认选择普通…...
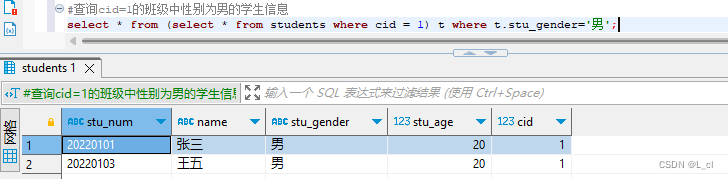
Mysql数据库 9.SQL语言 查询语句 连接查询、子查询
连接查询 通过查询多张表,用连接查询进行多表联合查询 关键字:inner join 内连接 left join 左连接 right join 右连接 数据准备 创建新的数据库:create database 数据库名; create database db_test2; 使用数据库:use 数据…...
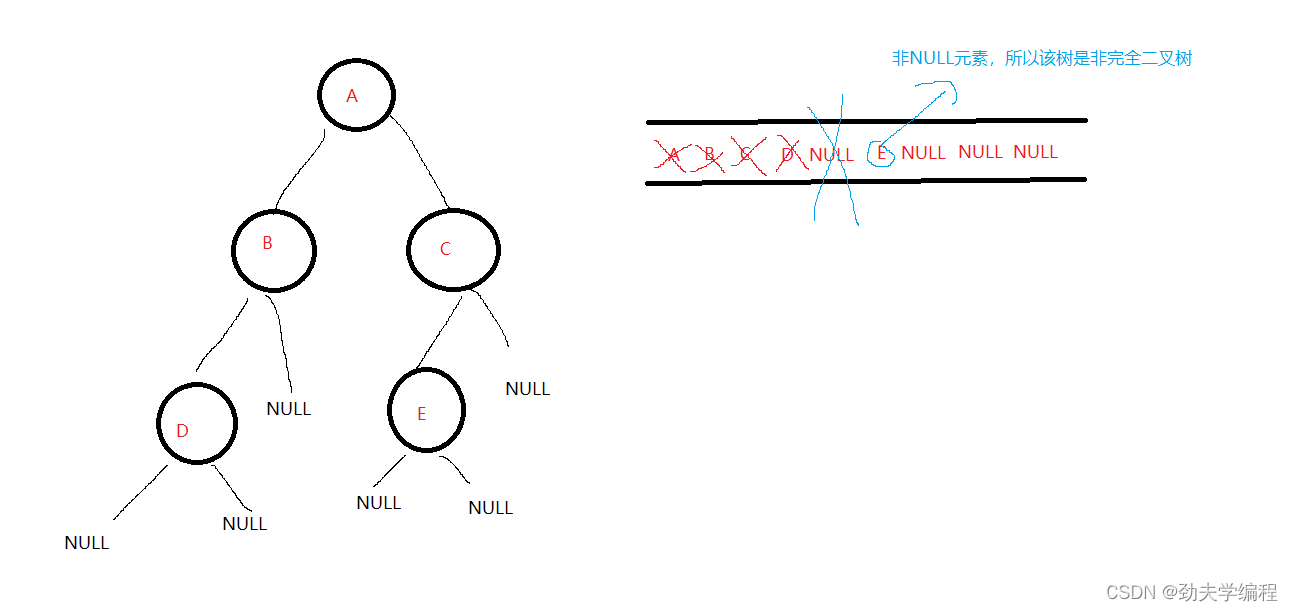
二叉树按二叉链表形式存储,试编写一个判别给定二叉树是否是完全二叉树的算法
完全二叉树:就是每层横着划过去是连起来的,中间不会断开 比如下面的左图就是完全二叉树 再比如下面的右图就是非完全二叉树 那我们可以采用层序遍历的方法,借助一个辅助队列 当辅助队列不空的时候,出队头元素,入队头…...
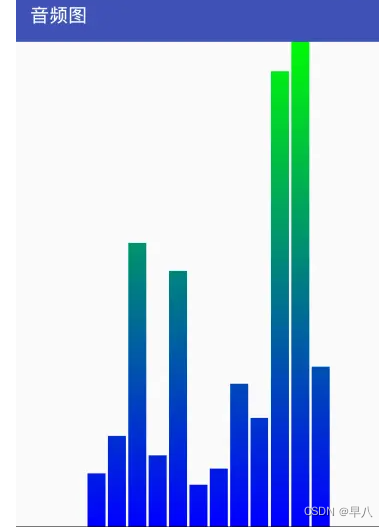
Android自定义控件
目录 Android自定义控件一、对现有控件进行扩展二、创建复合控件1 定义属性2 组合控件3 引用UI模板 三、重写View来实现全新控件1 弧线展示图1.1 具体步骤: 2 音频条形图2.1 具体步骤 四、补充:自定义ViewGroup Android自定义控件 ref: Android自定义控件…...
Java 中的 Cloneable 接口和深拷贝
引言: 在 Java 中,深拷贝是一种常见的需求,它可以创建一个对象的完全独立副本。Cloneable 接口提供了一种标记机制,用于指示一个类实例可以被复制。本文将详细介绍 Java 中的 Cloneable 接口和深拷贝的相关知识࿰…...
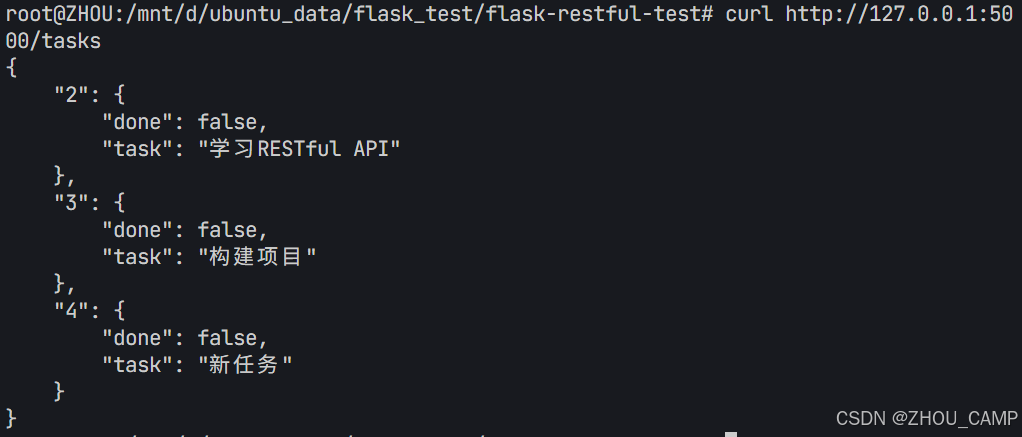
Flask RESTful 示例
目录 1. 环境准备2. 安装依赖3. 修改main.py4. 运行应用5. API使用示例获取所有任务获取单个任务创建新任务更新任务删除任务 中文乱码问题: 下面创建一个简单的Flask RESTful API示例。首先,我们需要创建环境,安装必要的依赖,然后…...
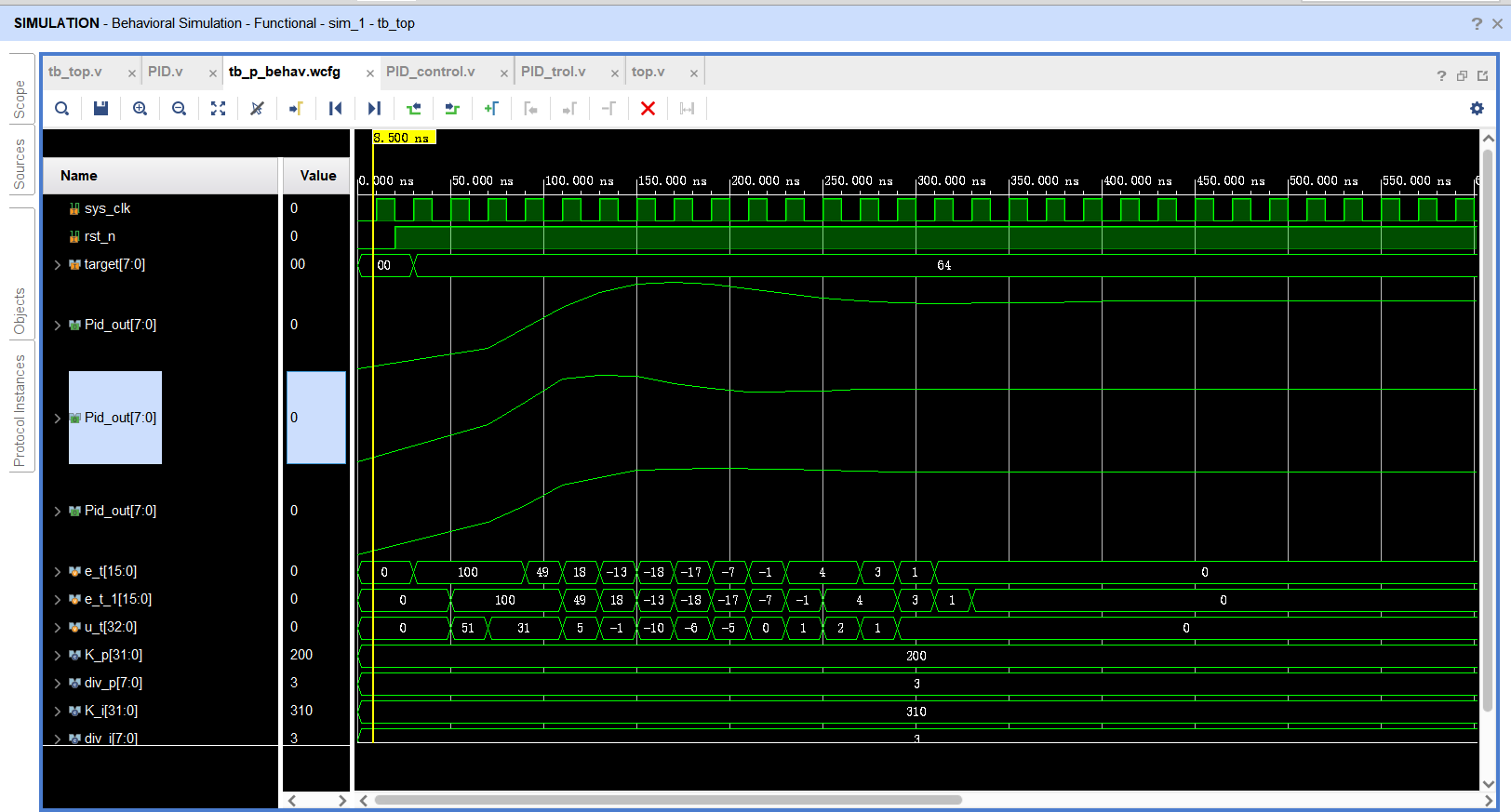
基于FPGA的PID算法学习———实现PID比例控制算法
基于FPGA的PID算法学习 前言一、PID算法分析二、PID仿真分析1. PID代码2.PI代码3.P代码4.顶层5.测试文件6.仿真波形 总结 前言 学习内容:参考网站: PID算法控制 PID即:Proportional(比例)、Integral(积分&…...
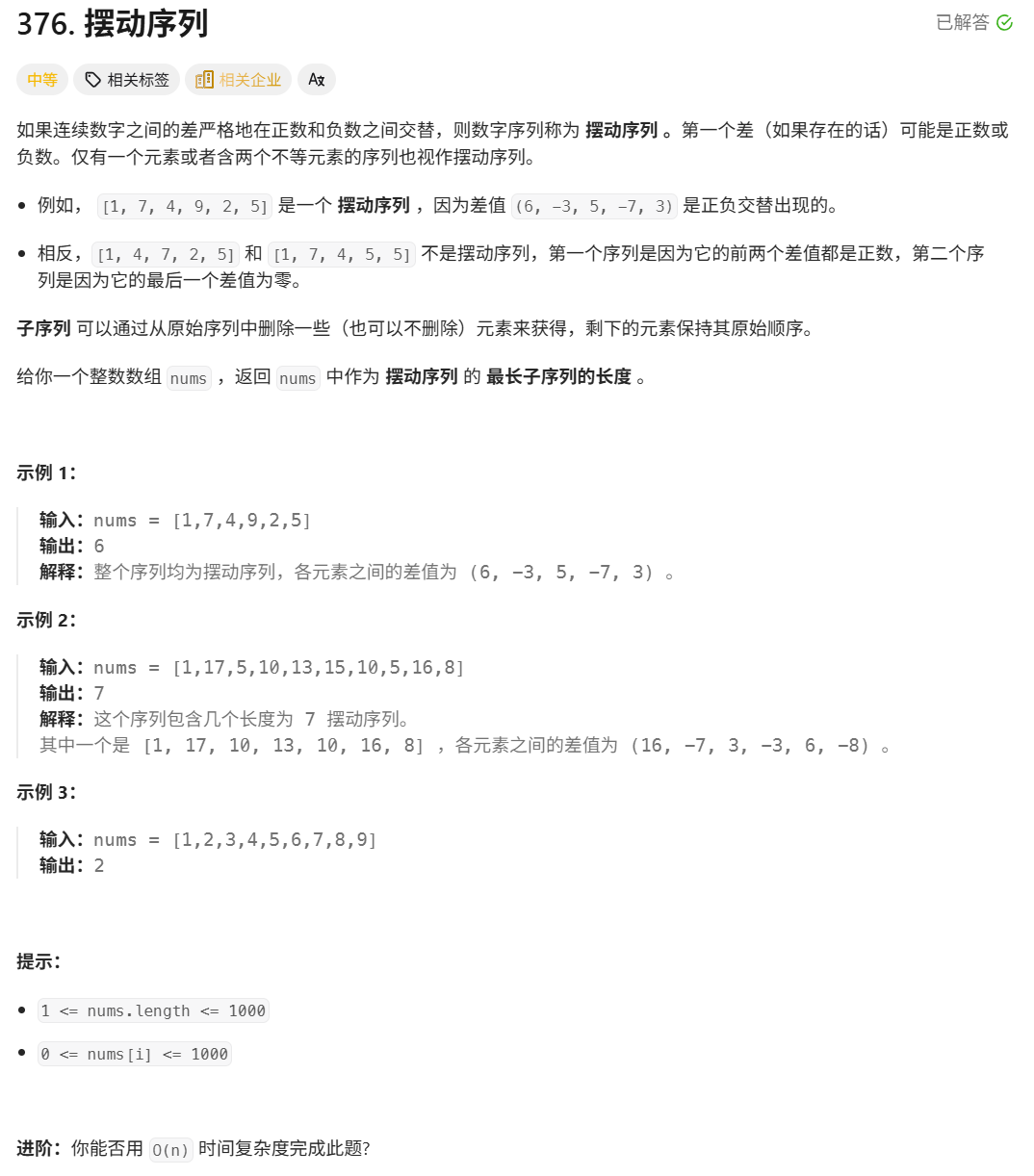
376. Wiggle Subsequence
376. Wiggle Subsequence 代码 class Solution { public:int wiggleMaxLength(vector<int>& nums) {int n nums.size();int res 1;int prediff 0;int curdiff 0;for(int i 0;i < n-1;i){curdiff nums[i1] - nums[i];if( (prediff > 0 && curdif…...
oracle与MySQL数据库之间数据同步的技术要点
Oracle与MySQL数据库之间的数据同步是一个涉及多个技术要点的复杂任务。由于Oracle和MySQL的架构差异,它们的数据同步要求既要保持数据的准确性和一致性,又要处理好性能问题。以下是一些主要的技术要点: 数据结构差异 数据类型差异ÿ…...
sqlserver 根据指定字符 解析拼接字符串
DECLARE LotNo NVARCHAR(50)A,B,C DECLARE xml XML ( SELECT <x> REPLACE(LotNo, ,, </x><x>) </x> ) DECLARE ErrorCode NVARCHAR(50) -- 提取 XML 中的值 SELECT value x.value(., VARCHAR(MAX))…...
Java 加密常用的各种算法及其选择
在数字化时代,数据安全至关重要,Java 作为广泛应用的编程语言,提供了丰富的加密算法来保障数据的保密性、完整性和真实性。了解这些常用加密算法及其适用场景,有助于开发者在不同的业务需求中做出正确的选择。 一、对称加密算法…...
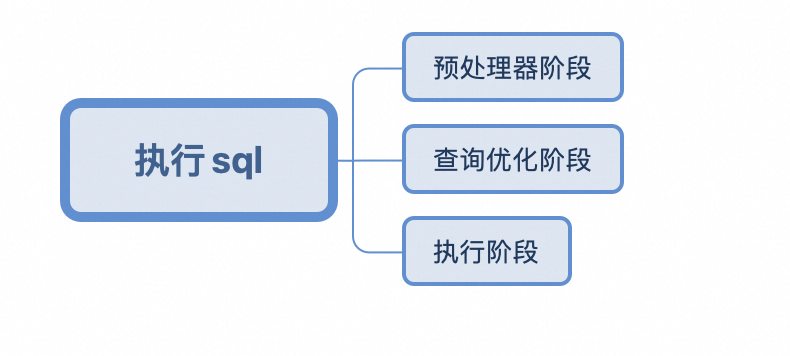
Mysql中select查询语句的执行过程
目录 1、介绍 1.1、组件介绍 1.2、Sql执行顺序 2、执行流程 2.1. 连接与认证 2.2. 查询缓存 2.3. 语法解析(Parser) 2.4、执行sql 1. 预处理(Preprocessor) 2. 查询优化器(Optimizer) 3. 执行器…...
C#中的CLR属性、依赖属性与附加属性
CLR属性的主要特征 封装性: 隐藏字段的实现细节 提供对字段的受控访问 访问控制: 可单独设置get/set访问器的可见性 可创建只读或只写属性 计算属性: 可以在getter中执行计算逻辑 不需要直接对应一个字段 验证逻辑: 可以…...
现有的 Redis 分布式锁库(如 Redisson)提供了哪些便利?
现有的 Redis 分布式锁库(如 Redisson)相比于开发者自己基于 Redis 命令(如 SETNX, EXPIRE, DEL)手动实现分布式锁,提供了巨大的便利性和健壮性。主要体现在以下几个方面: 原子性保证 (Atomicity)ÿ…...
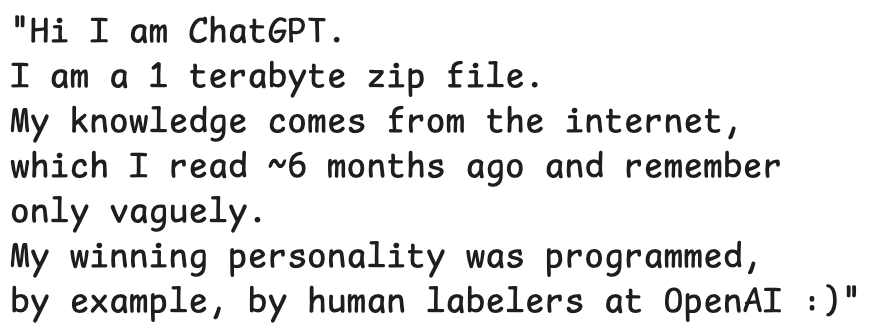
LLMs 系列实操科普(1)
写在前面: 本期内容我们继续 Andrej Karpathy 的《How I use LLMs》讲座内容,原视频时长 ~130 分钟,以实操演示主流的一些 LLMs 的使用,由于涉及到实操,实际上并不适合以文字整理,但还是决定尽量整理一份笔…...