Reinforcement Learning with Code 【Code 2. Tabular Sarsa】
Reinforcement Learning with Code 【Code 2. Tabular Sarsa】
This note records how the author begin to learn RL. Both theoretical understanding and code practice are presented. Many material are referenced such as ZhaoShiyu’s Mathematical Foundation of Reinforcement Learning.
This code refers to Mofan’s reinforcement learning course.
文章目录
- Reinforcement Learning with Code 【Code 2. Tabular Sarsa】
- 2.1 Problem and result
- 2.2 Environment
- 2.3 Tabular Sarsa Algorithm
- 2.4 Run this main
- 2.5 Check the Q table
- Reference
2.1 Problem and result
Please consider the problem that a little mouse (denoted by red block) wants to avoid trap (denoted by black block) to get the cheese (denoted by yellow circle). As the figure shows.
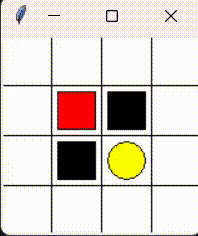
This chapter aims to realize tabular Sarsa algorithm sovle this problem.
2.2 Environment
We use the tkinter
package of python to build our environment to interact with agent.
import numpy as np
import time
import sys
import tkinter as tk
# if sys.version_info.major == 2: # 检查python版本是否是python2
# import Tkinter as tk
# else:
# import tkinter as tkUNIT = 40 # pixels
MAZE_H = 4 # grid height
MAZE_W = 4 # grid widthclass Maze(tk.Tk, object):def __init__(self):super(Maze, self).__init__()# Action Spaceself.action_space = ['up', 'down', 'right', 'left'] # action space self.n_actions = len(self.action_space)# 绘制GUIself.title('Maze env')self.geometry('{0}x{1}'.format(MAZE_W * UNIT, MAZE_H * UNIT)) # 指定窗口大小 "width x height"self._build_maze()def _build_maze(self):self.canvas = tk.Canvas(self, bg='white',height=MAZE_H * UNIT,width=MAZE_W * UNIT) # 创建背景画布# create gridsfor c in range(UNIT, MAZE_W * UNIT, UNIT): # 绘制列分隔线x0, y0, x1, y1 = c, 0, c, MAZE_H * UNITself.canvas.create_line(x0, y0, x1, y1)for r in range(UNIT, MAZE_H * UNIT, UNIT): # 绘制行分隔线x0, y0, x1, y1 = 0, r, MAZE_W * UNIT, rself.canvas.create_line(x0, y0, x1, y1)# create origin 第一个方格的中心,origin = np.array([UNIT/2, UNIT/2]) # hell1hell1_center = origin + np.array([UNIT * 2, UNIT])self.hell1 = self.canvas.create_rectangle(hell1_center[0] - (UNIT/2 - 5), hell1_center[1] - (UNIT/2 - 5),hell1_center[0] + (UNIT/2 - 5), hell1_center[1] + (UNIT/2 - 5),fill='black')# hell2hell2_center = origin + np.array([UNIT, UNIT * 2])self.hell2 = self.canvas.create_rectangle(hell2_center[0] - (UNIT/2 - 5), hell2_center[1] - (UNIT/2 - 5),hell2_center[0] + (UNIT/2 - 5), hell2_center[1] + (UNIT/2 - 5),fill='black')# create oval 绘制终点圆形oval_center = origin + np.array([UNIT*2, UNIT*2])self.oval = self.canvas.create_oval(oval_center[0] - (UNIT/2 - 5), oval_center[1] - (UNIT/2 - 5),oval_center[0] + (UNIT/2 - 5), oval_center[1] + (UNIT/2 - 5),fill='yellow')# create red rect 绘制agent红色方块,初始在方格左上角self.rect = self.canvas.create_rectangle(origin[0] - (UNIT/2 - 5), origin[1] - (UNIT/2 - 5),origin[0] + (UNIT/2 - 5), origin[1] + (UNIT/2 - 5),fill='red')# pack all 显示所有canvasself.canvas.pack()def get_state(self, rect):# convert the coordinate observation to state tuple# use the uniformed center as the state such as # |(1,1)|(2,1)|(3,1)|...# |(1,2)|(2,2)|(3,2)|...# |(1,3)|(2,3)|(3,3)|...# |....x0,y0,x1,y1 = self.canvas.coords(rect)x_center = (x0+x1)/2y_center = (y0+y1)/2state = ((x_center-(UNIT/2))/UNIT + 1, (y_center-(UNIT/2))/UNIT + 1)return statedef reset(self):self.update()self.after(500) # delay 500msself.canvas.delete(self.rect) # delete origin rectangleorigin = np.array([UNIT/2, UNIT/2])self.rect = self.canvas.create_rectangle(origin[0] - (UNIT/2 - 5), origin[1] - (UNIT/2 - 5),origin[0] + (UNIT/2 - 5), origin[1] + (UNIT/2 - 5),fill='red')# return observation return self.get_state(self.rect) def step(self, action):# agent和环境进行一次交互s = self.get_state(self.rect) # 获得智能体的坐标base_action = np.array([0, 0])reach_boundary = Falseif action == self.action_space[0]: # upif s[1] > 1:base_action[1] -= UNITelse: # 触碰到边界reward=-1并停留在原地reach_boundary = Trueelif action == self.action_space[1]: # downif s[1] < MAZE_H:base_action[1] += UNITelse:reach_boundary = True elif action == self.action_space[2]: # rightif s[0] < MAZE_W:base_action[0] += UNITelse:reach_boundary = Trueelif action == self.action_space[3]: # leftif s[0] > 1:base_action[0] -= UNITelse:reach_boundary = Trueself.canvas.move(self.rect, base_action[0], base_action[1]) # move agents_ = self.get_state(self.rect) # next state# reward functionif s_ == self.get_state(self.oval): # reach the terminalreward = 1done = Trues_ = 'success'elif s_ == self.get_state(self.hell1): # reach the blockreward = -1s_ = 'block_1'done = Falseelif s_ == self.get_state(self.hell2):reward = -1s_ = 'block_2'done = Falseelse:reward = 0done = Falseif reach_boundary:reward = -1return s_, reward, donedef render(self):time.sleep(0.15)self.update()if __name__ == '__main__':def test():for t in range(10):s = env.reset()print(s)while True:env.render()a = 'right's, r, done = env.step(a)print(s)if done:breakenv = Maze()env.after(100, test) # 在延迟100ms后调用函数testenv.mainloop()
This part is important that the reward function design is include, which is as follows
reward = { 1 , if reach the cheese − 1 , if reach the trap or reach the boundary 0 , others \text{reward} = \left \{ \begin{aligned} & 1, \quad \text{if reach the cheese} \\ & -1, \quad \text{if reach the trap or reach the boundary} \\ & 0, \quad \text{others} \end{aligned} \right. reward=⎩ ⎨ ⎧1,if reach the cheese−1,if reach the trap or reach the boundary0,others
We need to explan some function of the class Maze
.
- First, the function
_build_maze
creates the inital maze location.
In this example we use the left up coordination of each grid as the state of each block. - Second, the function
get_state
converts the coordination of each grid to numerical representation such as ( 1 , 1 ) , ( 1 , 2 ) , ⋯ (1,1),(1,2),\cdots (1,1),(1,2),⋯. - Third, the function
reset
renew the state which means placing the mouse in the original grid. - Then, the function
step
we let the agent interact with envrionment for one step, ang get the reward after the action. - Then, the function
render
controls updating the window.
2.3 Tabular Sarsa Algorithm
import numpy as np
import pandas as pdclass RL():def __init__(self, actions, learning_rate=0.01, reward_decay=0.9, e_greedy=0.9):self.actions = actions # action listself.lr = learning_rateself.gamma = reward_decayself.epsilon = e_greedy # epsilon greedy update policyself.q_table = pd.DataFrame(columns=self.actions, dtype=np.float64)def check_state_exist(self, state):if state not in self.q_table.index:# append new state to q table, use the coordinate as the observation# self.q_table = self.q_table.append( # DataFrame.append is invalid# pd.Series(# [0]*len(self.actions),# index=self.q_table.columns,# name=state,# )# )self.q_table = pd.concat([self.q_table,pd.DataFrame(data=np.zeros((1,len(self.actions))),columns = self.q_table.columns,index = [state])])def choose_action(self, observation):"""Use the epsilon-greedy method to update policy"""self.check_state_exist(observation)# action selection# epsilon greedy algorithmif np.random.uniform() < self.epsilon:state_action = self.q_table.loc[observation, :]# some actions may have the same value, randomly choose on in these actions# state_action == np.max(state_action) generate bool mask# choose best actionaction = np.random.choice(state_action[state_action == np.max(state_action)].index)else:# choose random actionaction = np.random.choice(self.actions)return actiondef learn(self, s, a, r, s_):passclass SarsaTable(RL):"""Implement Sarsa algorithm which is on-policy"""def __init__(self, actions, learning_rate=0.01, reward_decay=0.9, e_greedy=0.9):super(SarsaTable,self).__init__(actions, learning_rate, reward_decay, e_greedy)def learn(self, s, a, r, s_, a_):self.check_state_exist(s_)q_predict = self.q_table.loc[s, a]if s_ != 'success' :q_target = r + self.gamma * self.q_table.loc[s_, a_] # next state is not terminalelse:q_target = r # next state is terminalself.q_table.loc[s, a] += self.lr * (q_target - q_predict) # update
We store the Q-table as a DataFrame
of pandas
. The explanation of the functions are as follows.
- First, the function
check_state_exist
check the existence of one state, if not we append it to the Q-table. This is because once the state-action pair is visited, then we update it into the Q-table. - Second, the function
choose_action
is following the ϵ \epsilon ϵ-greedy algorithm
π ( a ∣ s ) = { 1 − ϵ ∣ A ( s ) ∣ ( ∣ A ( s ) ∣ − 1 ) , for the geedy action ϵ ∣ A ( s ) ∣ , for the other ∣ A ( s ) ∣ − 1 actions \pi(a|s) = \left \{ \begin{aligned} 1 - \frac{\epsilon}{|\mathcal{A}(s)|}(|\mathcal{A(s)}|-1), & \quad \text{for the geedy action} \\ \frac{\epsilon}{|\mathcal{A}(s)|}, & \quad \text{for the other } |\mathcal{A}(s)|-1 \text{ actions} \end{aligned} \right. π(a∣s)=⎩ ⎨ ⎧1−∣A(s)∣ϵ(∣A(s)∣−1),∣A(s)∣ϵ,for the geedy actionfor the other ∣A(s)∣−1 actions
- Third, the function
learn
is update the q value as Q-learning algorithm purposed, which relays on the sample ( s t , a t , r t + 1 , s t + 1 , a t + 1 ) \textcolor{red}{(s_t,a_t,r_{t+1},s_{t+1},a_{t+1})} (st,at,rt+1,st+1,at+1). The sample denotes current state, current action, immediate reward, next state and next action respectively.
Sarsa : { q t + 1 ( s t , a t ) = q t ( s t , a t ) − α t ( s t , a t ) [ q t ( s t , a t ) − ( r t + 1 + γ q t ( s t + 1 , a t + 1 ) ) ] q t + 1 ( s , a ) = q t ( s , a ) , for all ( s , a ) ≠ ( s t , a t ) \text{Sarsa} : \left \{ \begin{aligned} \textcolor{red}{q_{t+1}(s_t,a_t)} & \textcolor{red}{= q_t(s_t,a_t) - \alpha_t(s_t,a_t) \Big[q_t(s_t,a_t) - (r_{t+1}+ \gamma \ q_t(s_{t+1},a_{t+1})) \Big]} \\ \textcolor{red}{q_{t+1}(s,a)} & \textcolor{red}{= q_t(s,a)}, \quad \text{for all } (s,a) \ne (s_t,a_t) \end{aligned} \right. Sarsa:⎩ ⎨ ⎧qt+1(st,at)qt+1(s,a)=qt(st,at)−αt(st,at)[qt(st,at)−(rt+1+γ qt(st+1,at+1))]=qt(s,a),for all (s,a)=(st,at)
2.4 Run this main
Run this main script that we can run the all codes.
from maze_env_custom import Maze
from RL_brain import SarsaTableMAX_EPISODE = 30def update():for episode in range(MAX_EPISODE):# initial observation, observation is the rect's coordiante# observation is [x0,y0, x1,y1]observation = env.reset() # RL choose action based on observation ['up', 'down', 'right', 'left']action = RL.choose_action(str(observation))while True:# fresh envenv.render()# RL take action and get next observation and rewardobservation_, reward, done = env.step(action)action_ = RL.choose_action(str(observation_))# RL learn from this transitionRL.learn(str(observation), action, reward, str(observation_), action_)# swap observationobservation = observation_action = action_# break while loop when end of this episodeif done:break# show q_tableprint(RL.q_table)print('\n')# end of gameprint('game over')env.destroy()if __name__ == "__main__":env = Maze()RL = SarsaTable(env.action_space)env.after(100, update)env.mainloop()
2.5 Check the Q table
After a long run we can check the q-table to judge wheter the learning is reasonable. The q-table is as follows:
up down right left
(1.0, 1.0) -6.837352e-02 -0.000135 -0.000266 -2.970185e-02
(2.0, 1.0) -4.901299e-02 -0.000334 -0.000484 -6.039572e-04
(2.0, 2.0) -3.988164e-04 -0.049010 -0.038785 -2.737623e-04
block_1 0.000000e+00 0.049010 0.000000 0.000000e+00
(4.0, 2.0) -2.646359e-04 0.001314 -0.019900 -1.000000e-02
(4.0, 1.0) -4.900994e-02 0.000014 -0.010000 -3.128178e-06
(3.0, 1.0) -2.970450e-02 -0.029433 -0.000516 -2.078845e-04
(1.0, 2.0) -4.933690e-04 -0.000374 -0.000951 -3.940947e-02
block_2 -1.979099e-07 0.000000 0.010000 -1.531800e-07
(1.0, 3.0) -3.525635e-04 -0.000056 -0.010000 -3.940439e-02
(1.0, 4.0) -7.194310e-07 -0.010000 0.000591 -1.990000e-02
(2.0, 4.0) -1.000000e-02 -0.019900 0.012381 0.000000e+00
(3.0, 4.0) 1.654862e-01 0.000000 0.000000 0.000000e+00
(4.0, 4.0) 0.000000e+00 0.000000 -0.010000 0.000000e+00
(4.0, 3.0) 0.000000e+00 0.000000 0.000000 5.851985e-02
success 0.000000e+00 0.000000 0.000000 0.000000e+00
For example, when at the original place if the mouse wants to move up or move left it will reach the boundary and get reward − 1 -1 −1. Hence the state value in q-table is minus.
Reference
赵世钰老师的课程
莫烦ReinforcementLearning course
相关文章:
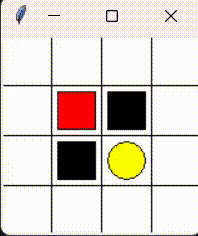
Reinforcement Learning with Code 【Code 2. Tabular Sarsa】
Reinforcement Learning with Code 【Code 2. Tabular Sarsa】 This note records how the author begin to learn RL. Both theoretical understanding and code practice are presented. Many material are referenced such as ZhaoShiyu’s Mathematical Foundation of Rei…...
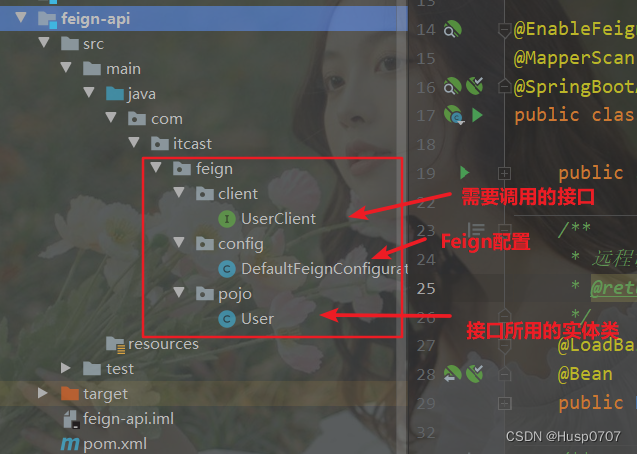
服务调用---------Ribbon和Feign
目录 1、Ribbon 1.1 Ribbon简介 1.2 Ribbon负载均衡 负载均衡原理 负载均衡策略 Ribbon和Nginx的区别 1.3 服务调用和Ribbon负载均衡实现 2、Feign&openFeign 3、Feign支持的配置 日志功能 连接池 feign-api远程包 1、Ribbon 1.1 Ribbon简介 Ribb…...
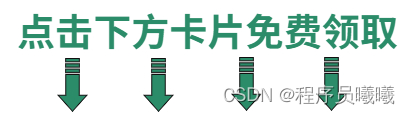
app自动化测试之Appium问题分析及定位
使用 Appium 进行测试时,会产生大量日志,一旦运行过程中遇到报错,可以通过 Appium 服务端的日志以及客户端的日志分析排查问题。 Appium Server日志-开启服务 通过命令行的方式启动 Appium Server,下面来分析一下启动日志&#…...
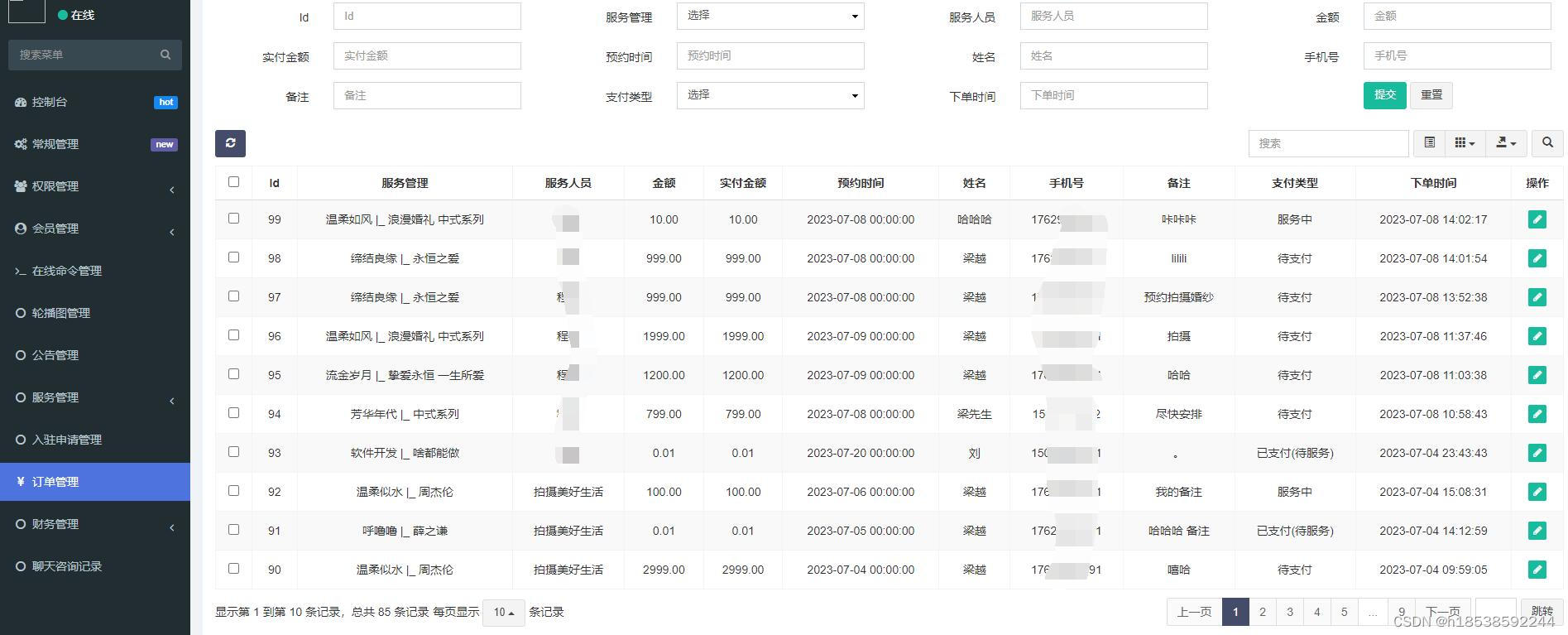
婚庆服务小程序app开发方案详解
开发一款婚庆行业服务小程序有哪些功能呢? 1、选择分类 选择婚庆、婚车、婚宴、司仪、彩妆、婚庆用品、跟拍、摄影等,筛选出对应的商家 2、选择商家 选择分类后,可以选择商家,查看各个商家的详细介绍情况。 3、选择服务套餐 各…...
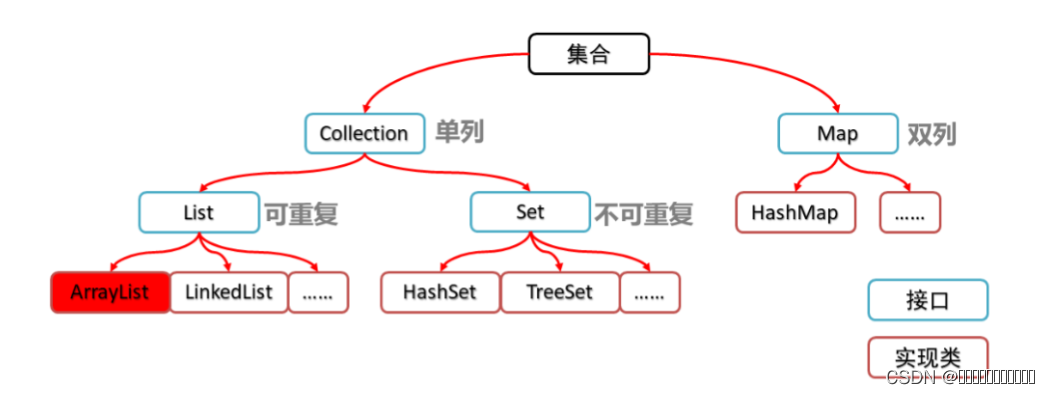
集合简述
集合ListArrayListLinkedList SetHashSetTreeSet MapHashMapTreeMap 集合与数组的区别 集合 集合是java中的一个容器,可以在里面存放数据,容量可以发生改变 从集合框架结构可以分析得知: 1、集合主要分为Collection和Map两个接口 2、Collecti…...

常见的软件测试面试题汇总
一、 你们的测试流程是怎么样的? 答:1.项目开始阶段,BA(需求分析师)从用户方收集需求并将需求转化为规格说明书,接 下来在项目组领导会组织需求评审。 2.需求评审通过后,BA 会组织项目经理…...
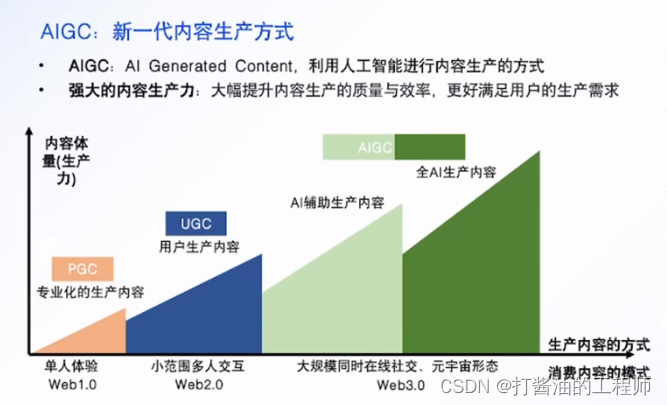
学习笔记|大模型优质Prompt开发与应用课(二)|第二节:超高产文本生成机,传媒营销人必备神器
文章目录 01 文字写作技能的革新,各行各业新机遇四大类常见文字工作新闻记者的一天新闻记者的一天–写策划prompt 新闻记者的一天–排采访prompt生成结果prompt生成结果 大模型加持,文字写作我们如何提效营销创作营销创作-使用预置法为不同平台生成文案p…...

Linux基础-4
1、linux高阶命令 1.1、find 在linux文件系统中,用来查找一个文件放在哪里了。 //举例 find /etc -name "interfaces" //总结: //(1)什么时候用find? //当你知道你要找的文件名,但是你忘记了它被放在哪个目录下&…...

oracle-创建函数
oracle自定义函数 核心提示:函数用于返回特定数据。执行时得找一个变量接收函数的返回值; 语法如下: create or replace function function_name ( argu1 [mode1]datatype1, argu2 [mode2] datatype2, … ) return datatype is begin end; 执行 var v1 varchar2(1…...
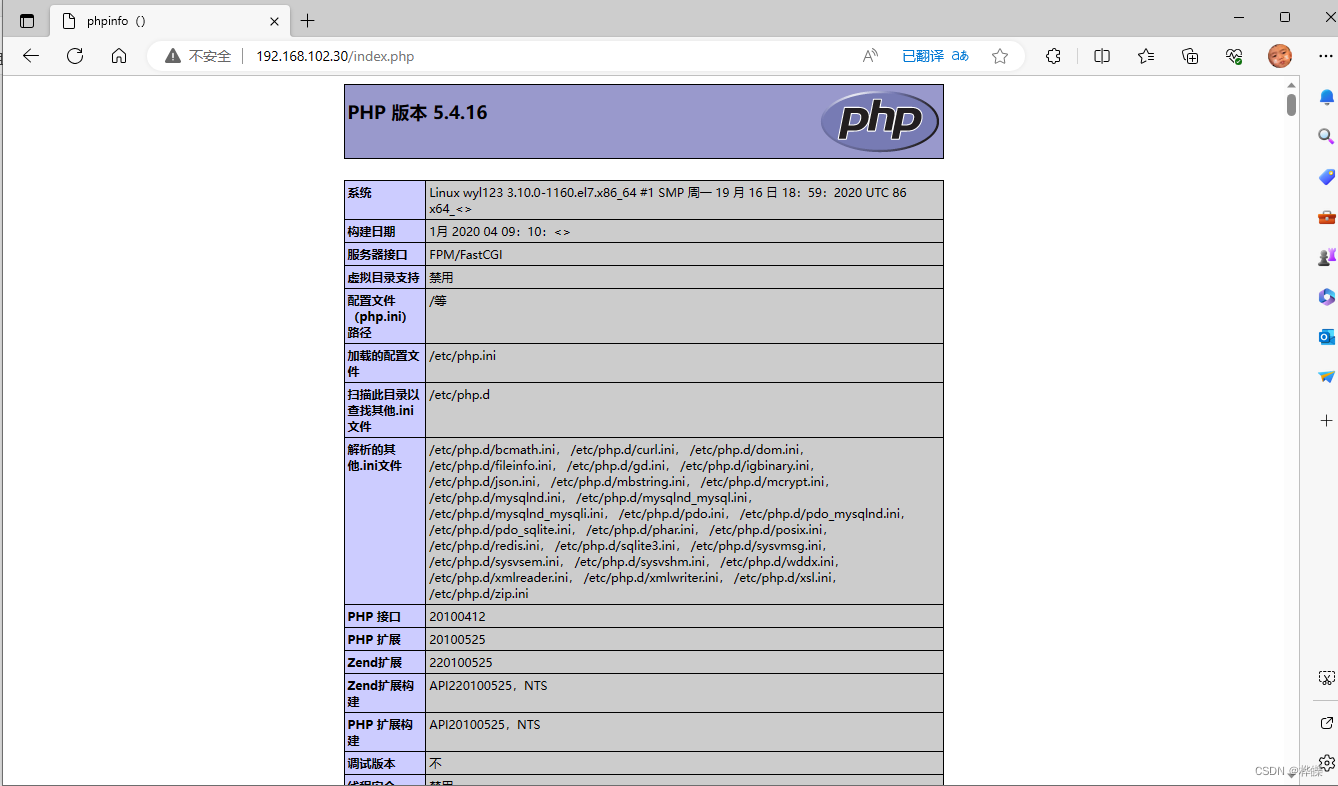
【Ansible 的脚本 --- playbook 剧本】
目录 一、playbook 剧本介绍二、示例1、运行playbook2、定义、引用变量 三、使用playbook部署lnmp集群 一、playbook 剧本介绍 playbooks 本身由以下各部分组成 (1)Tasks:任务,即通过 task 调用 ansible 的模板将多个操作组织在…...

ubuntu释放缓存
sudo sysctl vm.drop_caches1 sudo sysctl vm.drop_caches2 sudo sysctl vm.drop_caches3释放页面缓存: $ sudo sysctl vm.drop_caches1释放目录项和索引节点缓存: $ sudo sysctl vm.drop_caches2释放页面缓存、目录项和索引节点缓存: $ sudo…...
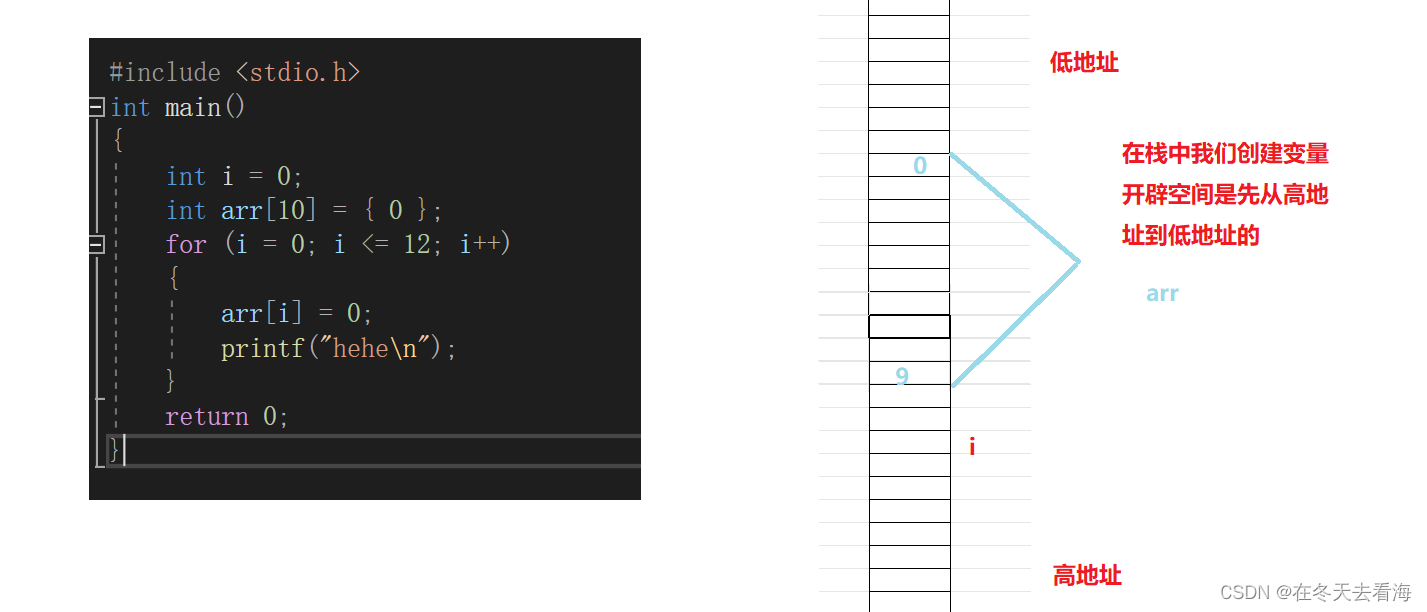
实用调试技巧(1)
什么是bug?调试是什么?有多重要?debug和release的介绍。windows环境调试介绍。一些调试的实例。如何写出好(易于调试)的代码。编程常见的错误。 什么是Bug 我们在写代码的时候遇到的一些问题而导致程序出问题的就是Bu…...
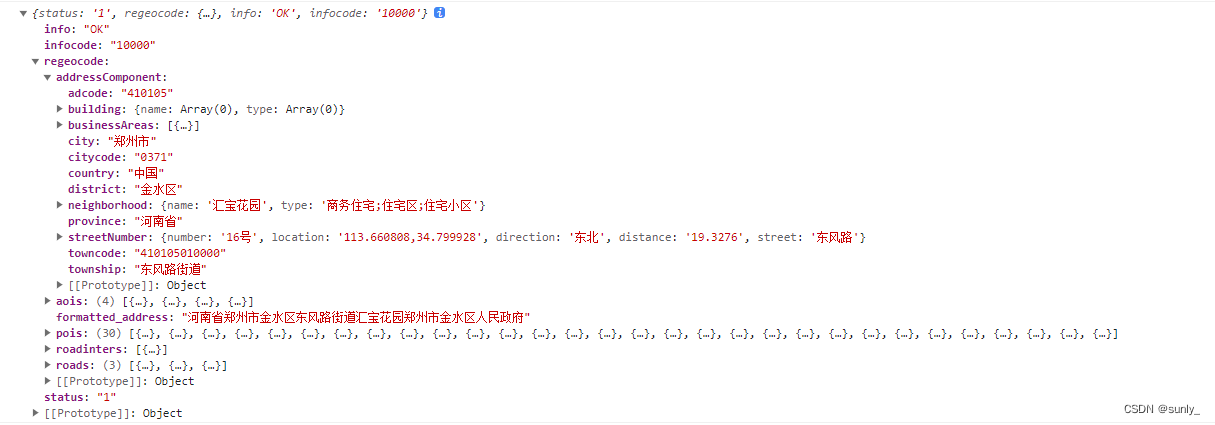
uniapp:H5定位当前省市区街道信息
高德地图api,H5定位省市区街道信息。 由于uniapp的uni.getLocation在H5不能获取到省市区街道信息,所以这里使用高德的逆地理编码接口地址接口,通过传key和当前经纬度,获取到省市区街道数据。 这里需要注意的是:**高德…...

自然语言处理从入门到应用——LangChain:提示(Prompts)-[提示模板:部分填充的提示模板和提示合成]
分类目录:《自然语言处理从入门到应用》总目录 部分填充的提示模板 提示模板是一个具有.format方法的类,它接受一个键值映射并返回一个字符串(一个提示),以传递给语言模型。与其他方法一样,将提示模板进行…...
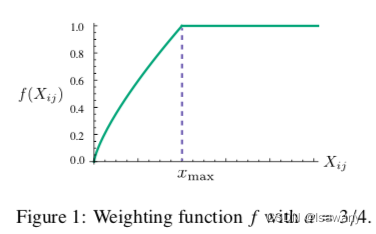
论文笔记--GloVe: Global Vectors for Word Representation
论文笔记--GloVe: Global Vectors for Word Representation 1. 文章简介2. 文章概括3 文章重点技术3.1 两种常用的单词向量训练方法3.2 GloVe3.3 模型的复杂度 4. 文章亮点5. 原文传送门6. References 1. 文章简介 标题:GloVe: Global Vectors for Word Representa…...
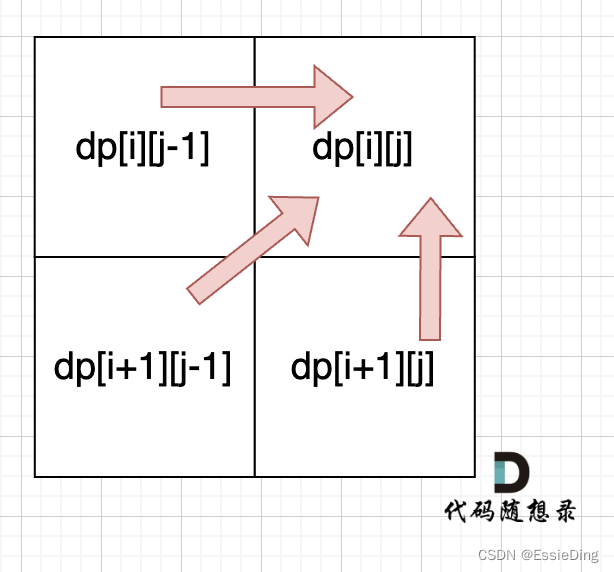
day57|● 647. 回文子串 ● 516.最长回文子序列
647. 回文子串 https://leetcode.cn/problems/palindromic-substrings/solution/by-lfool-2mvg/ Given a string s, return the number of palindromic substrings in it. A string is a palindrome when it reads the same backward as forward. A substring is a contiguous…...

docker compose.yml学习
docker compose 安装docker-compose sudo curl -L "https://github.com/docker/compose/releases/download/v2.2.2/docker-compose-$(uname -s)-$(uname -m)" -o /usr/local/bin/docker-composechmod x /usr/local/bin/docker-composeln -s /usr/local/bin/docker-…...
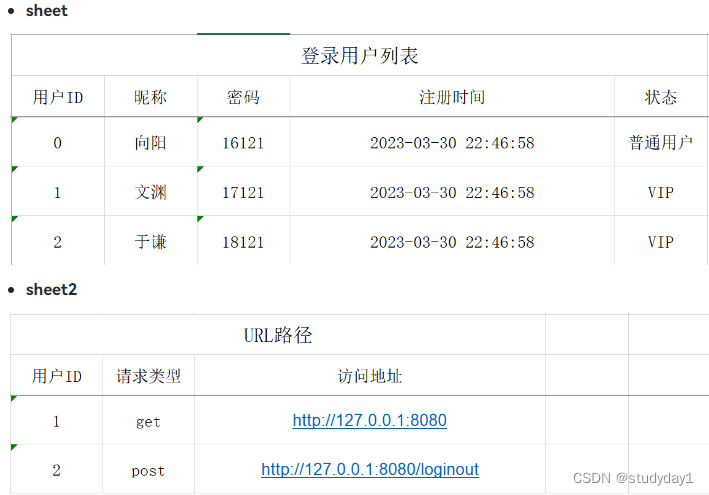
【业务功能篇55】Springboot+easyPOI 导入导出
Apache POI是Apache软件基金会的开源项目,POI提供API给Java程序对Microsoft Office格式档案读和写的功能。 Apache POI 代码实现复杂,学习成本较高。 Easypoi 功能如同名字easy,主打的功能就是容易,让一个没见接触过poi的人员 就可以方便的写出Excel导出…...
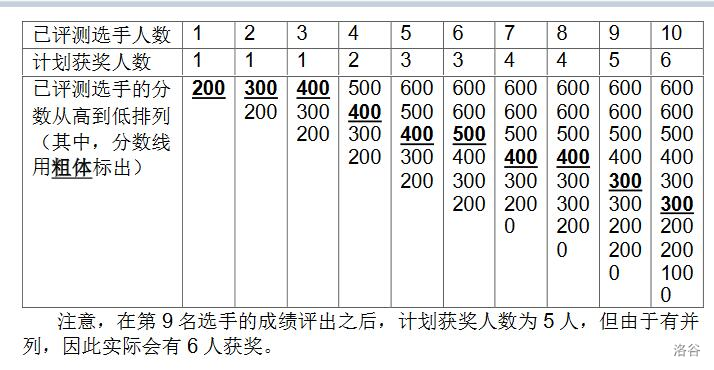
对顶堆算法
对顶堆可以动态维护一个序列上的第k大的数,由一个大根堆和一个小根堆组成, 小根堆维护前k大的数(包含第k个)大根堆维护比第k个数小的数 [CSP-J2020] 直播获奖 题目描述 NOI2130 即将举行。为了增加观赏性,CCF 决定逐一评出每个选手的成绩&a…...
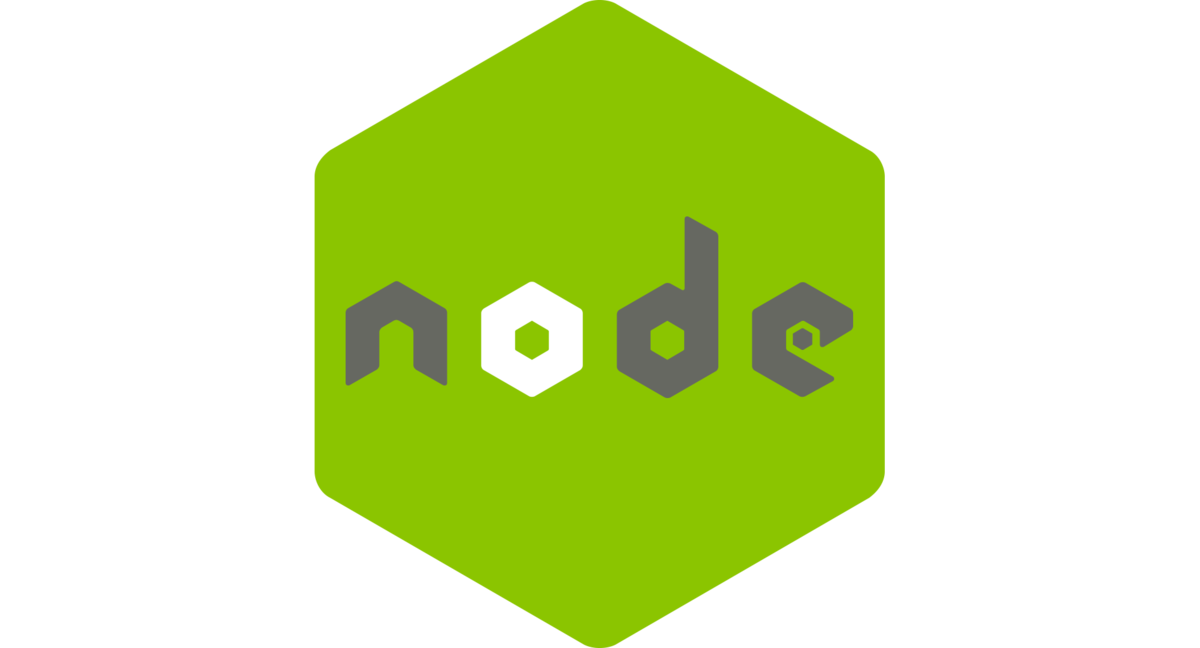
node.js的优点
提示:node.js的优点 文章目录 一、什么是node.js二、node.js的特性 一、什么是node.js 提示:什么是node.js? Node.js发布于2009年5月,由Ryan Dahl开发,是一个基于ChromeV8引擎的JavaScript运行环境,使用了一个事件驱…...

golang编译跨平台
golang可以在windows上编译出linux、MacOS等系统上的程序。 go编译器windows下可变翼linux程序,例如,GOARCHamd64 和 GOOSlinux 可以用于编译 64 位的 Linux 平台上的可执行文件。: set GOARCHamd64 set GOOSlinux go build main.go通过设置…...

关于Spring的bean的相关注解以及其简单使用方法
一、前置工作 第一步:创建一个maven项目 第二步:在resource中创建一个名字叫做spring-config.xml的文件,并把以下代码复制粘贴 <?xml version"1.0" encoding"UTF-8"?> <beans xmlns"http://www.sprin…...
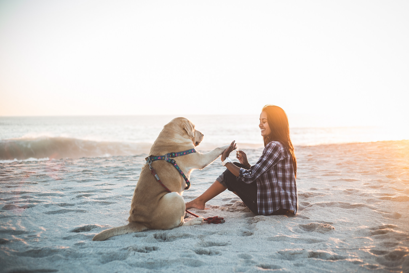
【计算机视觉】BLIP:源代码示例demo(含源代码)
文章目录 一、Image Captioning二、VQA三、Feature Extraction四、Image-Text Matching 一、Image Captioning 首先配置代码: import sys if google.colab in sys.modules:print(Running in Colab.)!pip3 install transformers4.15.0 timm0.4.12 fairscale0.4.4!g…...

TWILIGHT靶场详解
TWILIGHT靶场详解 下载地址:https://download.vulnhub.com/sunset/twilight.7z 这是一个比较简单的靶场,拿到IP后我们扫描发现开启了超级多的端口 其实这些端口一点用都没有,在我的方法中 但是也有不同的方法可以拿权限,就需要…...

【案例】--GPT衍生应用案例
目录 一、前言二、GPT实现智能问答架构2.1、基本的GPT实现智能问答架构2.2、可应用的GPT实现智能问答架构1、语义转换2、相似度关键字矩阵3、ES中搜索相似度关键字矩阵三、后续一、前言 GPT,全称Generative Pre-trained Transformer ,中文名可译作生成式预训练Transformer。…...
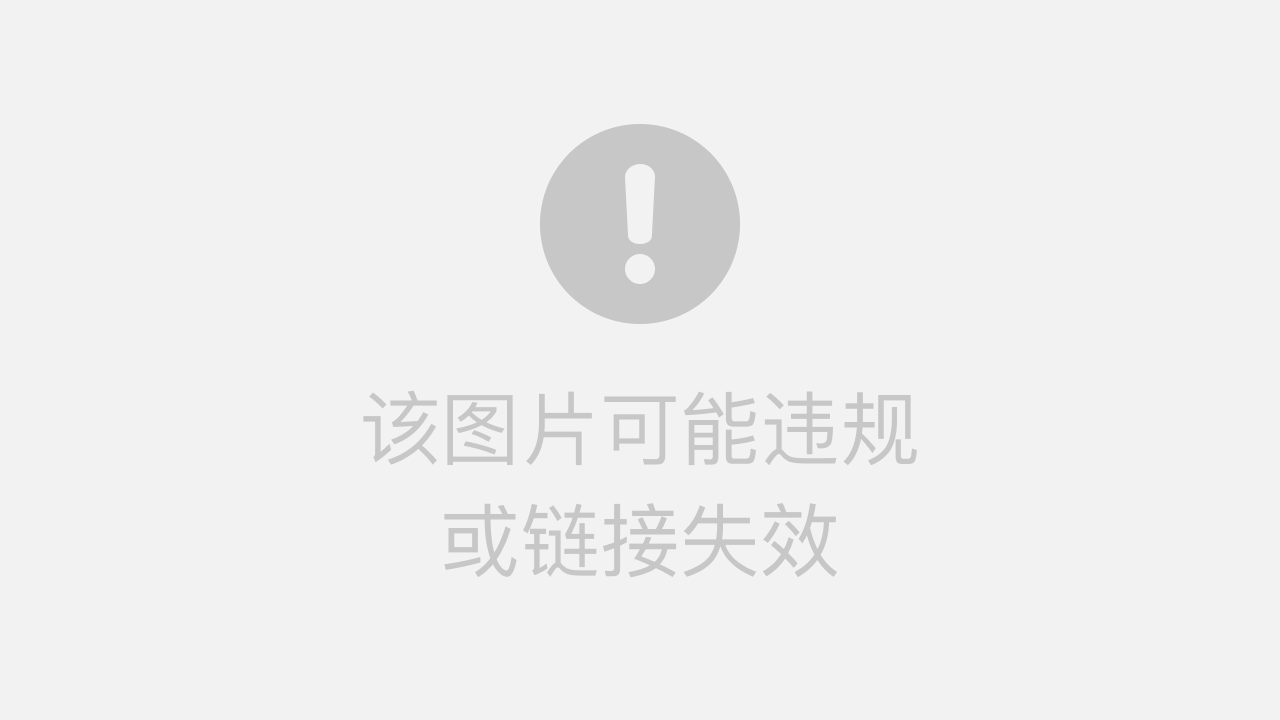
Sip网络音频对讲广播模块, sip网络寻呼话筒音频模块
Sip网络音频对讲广播模块, sip网络寻呼话筒音频模块 一、模块介绍 SV-2101VP和 SV-2103VP网络音频对讲广播模块 是一款通用的独立SIP音频功能模块,可以轻松地嵌入到OEM产品中。该模块对来自网络的SIP协议及RTP音频流进行编解码。 该模块支持多种网络协议…...

leetcode1219. 黄金矿工(java)
黄金矿工 leetcode1219. 黄金矿工题目描述回溯算法代码 回溯算法 leetcode1219. 黄金矿工 难度: 中等 eetcode 1219 黄金矿工 题目描述 你要开发一座金矿,地质勘测学家已经探明了这座金矿中的资源分布,并用大小为 m * n 的网格 grid 进行了标注。每个单元…...

Svelte框架入门
关键词 前端框架、编译器、响应式、模板 介绍 Svelte /svelt/ adj. 苗条的;线条清晰的;和蔼的 Svelte是一个前端组件框架,就像它的英文名字一样,Svelte的目标是打造一个更高性能的响应性前端框架。 Svelte类似于React和Vue框架&am…...
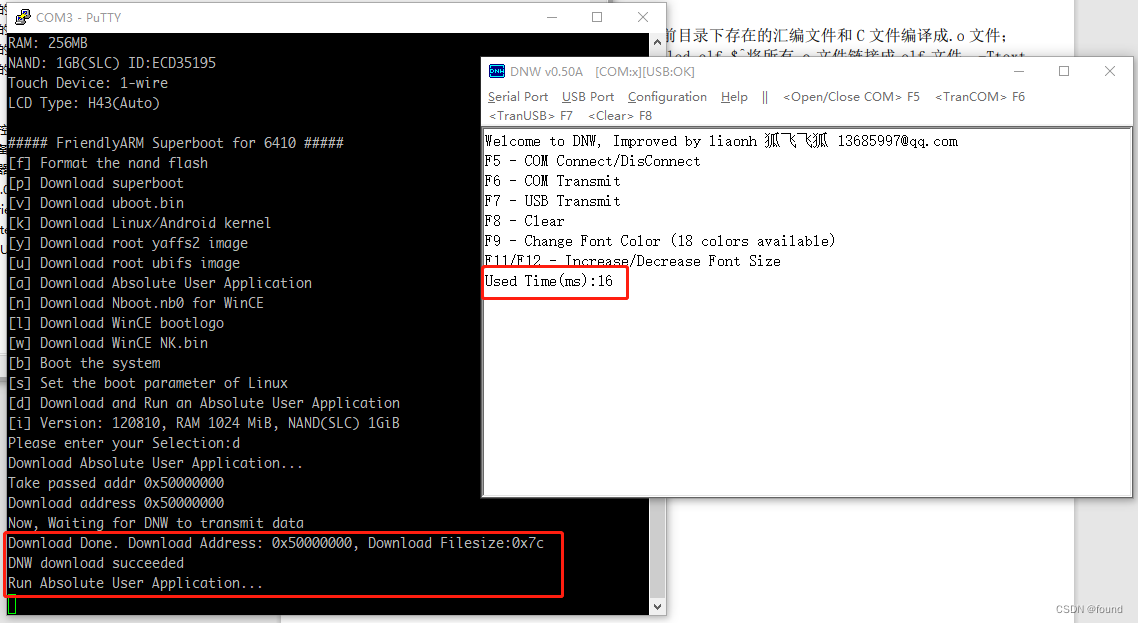
在linux中进行arm交叉编译体验tiny6410裸机程序开发流程
在某鱼上找了一个友善之臂的Tiny6410开发板用来体验一下嵌入式开发。这次先体验一下裸机程序的开发流程,由于这个开发板比较老旧了,官方文档有很多过期的内容,所以记录一下整个过程。 1. 交叉编译器安装 按照光盘A中的文档《04- Tiny6410 L…...
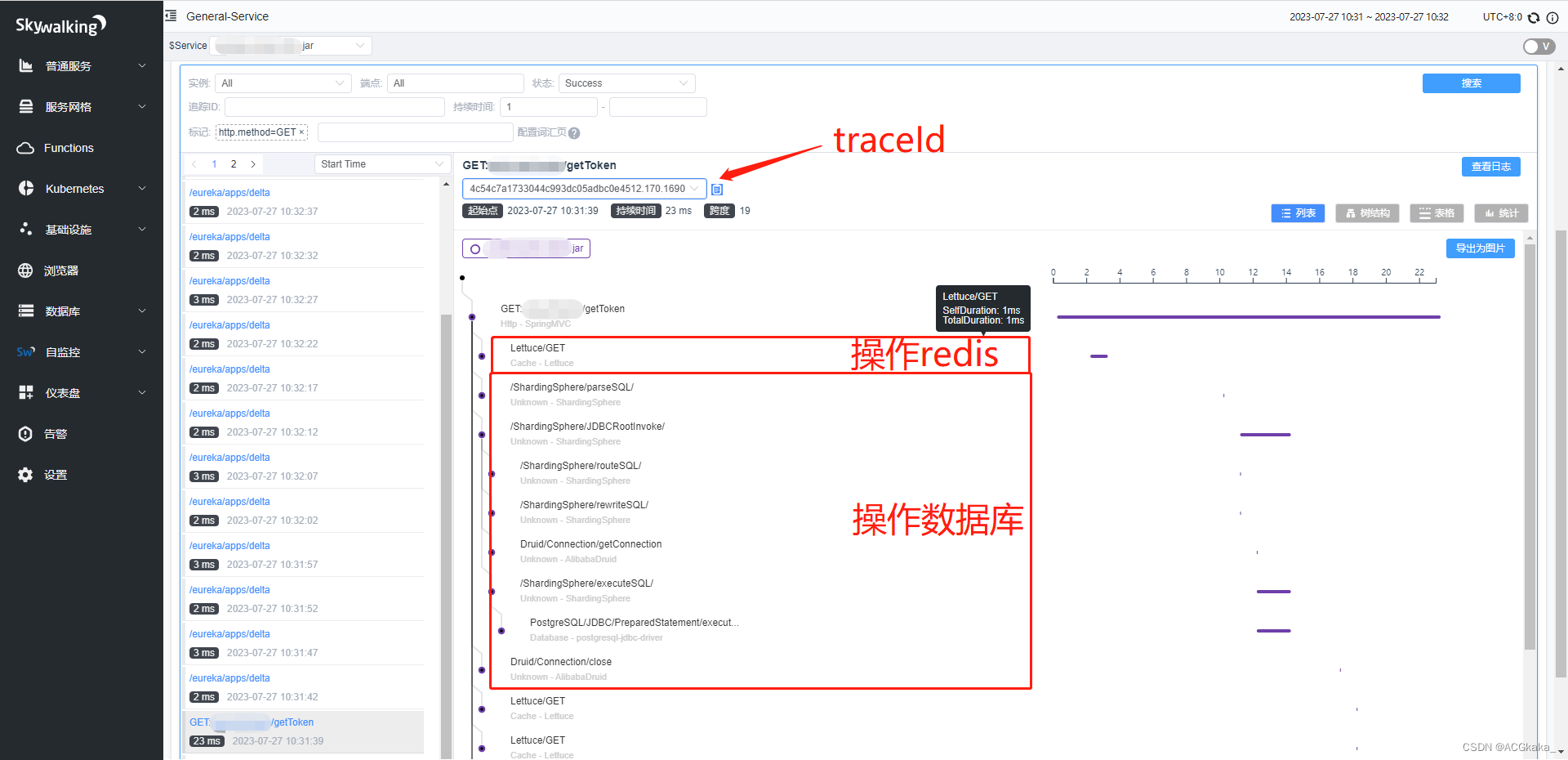
SpringBoot实战(二十三)集成 SkyWalking
目录 一、简介二、拉取镜像并部署1.拉取镜像2.运行skywalking-oap容器3.运行skywalking-ui容器4.访问页面 三、下载解压 agent1.下载2.解压 四、创建 skywalking-demo 项目1.Maven依赖2.application.yml3.DemoController.java 五、构建启动脚本1.startup.bat2.执行启动脚本3.发…...