【YOLOv5/v7改进系列】改进池化层为RT-DETR的AIFI
一、导言
Real-Time DEtection TRansformer(RT-DETR),是一种实时端到端目标检测器,克服了Non-Maximum Suppression(NMS)对速度和准确性的影响。通过设计高效的混合编码器和不确定性最小化查询选择,RT-DETR在保持准确性的同时提高了速度,实现了实时检测的要求。实验结果表明,RT-DETR在COCO数据集上达到了53.1%的平均精度(AP),并且在T4 GPU上实现了108 FPS的速度,优于以前的先进YOLO检测器。此外,RT-DETR还支持灵活的速度调节,适用于不同场景的应用。
AIFI(Attention-based Intra-scale Feature Interaction)是论文"DETRs Beat YOLOs on Real-time Object Detection"中提出的一个组件,属于Real-Time Detection Transformer (RT-DETR)模型的一部分。这个组件专注于解决Transformer编码器中多尺度特征交互导致的高计算成本问题,以实现更高效的实时目标检测。下面是对AIFI部分的优缺点的详细分析:
优点:
-
减少计算成本: AIFI通过仅在高级别的特征图(如S5)上执行特征内的自注意力操作,显著减少了计算负担。高级特征富含语义信息,对对象定位和识别至关重要,而低级别特征的自注意力操作可能引入冗余和混淆,因此针对性的处理提高了效率。
-
提升特征利用率: 通过聚焦于高层特征上的自注意力交互,AIFI能够更好地捕捉概念实体间的关联,这对于后续模块进行精确的物体定位和分类是极为有利的。
-
优化内存使用: 限制自注意力操作的范围到单个尺度的特征图,有助于减少内存占用,这对于实时应用来说至关重要,因为它允许在有限资源的硬件上运行。
-
提高准确性与速度平衡: 实验结果显示,在变体DS5中,仅在S5层进行的尺度内交互不仅显著提升了处理速度(比基础变体快35%),而且提高了检测精度(AP提高0.4%)。这表明AIFI在保持实时性的同时,对模型性能有正面影响。
缺点:
-
忽略低级特征交互: AIFI忽略了低级别特征图的尺度内交互,这可能意味着模型丢失了一些基于局部细节的特征信息。低级别特征通常包含边缘、纹理等基本信息,这些对于精细的物体识别也是必要的,尽管在实际中这种忽略并未导致性能下降,但理论上可能限制了模型在某些特定场景下的表现。
-
设计复杂性: AIFI的设计涉及到对Transformer编码器结构的改动,包括如何有效整合不同尺度的特征以及如何优化自注意力运算的范围,这增加了模型架构的复杂度,可能对模型理解和调试带来一定挑战。
-
参数调整敏感性: 任何涉及特征选择和交互方式改变的设计都可能对模型的整体表现非常敏感,这意味着AIFI的参数设置(如Transformer层数、特征融合方式等)需要细致调优,以达到最佳性能。
综上所述,AIFI在RT-DETR模型中是一个创新性的设计,它通过精心设计的特征交互策略,有效提高了实时目标检测的速度和精度。尽管存在一些潜在的局限性,如对低级特征的处理简化,但实验结果证明了其整体的有效性和实用性。
二、准备工作
首先在YOLOv5/v7的models文件夹下新建文件aifi.py,导入如下代码
class TransformerEncoderLayer(nn.Module):"""Defines a single layer of the transformer encoder."""def __init__(self, c1, cm=2048, num_heads=8, dropout=0.0, act=nn.GELU(), normalize_before=False):"""Initialize the TransformerEncoderLayer with specified parameters."""super().__init__()self.ma = nn.MultiheadAttention(c1, num_heads, dropout=dropout, batch_first=True)# Implementation of Feedforward modelself.fc1 = nn.Linear(c1, cm)self.fc2 = nn.Linear(cm, c1)self.norm1 = nn.LayerNorm(c1)self.norm2 = nn.LayerNorm(c1)self.dropout = nn.Dropout(dropout)self.dropout1 = nn.Dropout(dropout)self.dropout2 = nn.Dropout(dropout)self.act = actself.normalize_before = normalize_before@staticmethoddef with_pos_embed(tensor, pos=None):"""Add position embeddings to the tensor if provided."""return tensor if pos is None else tensor + posdef forward_post(self, src, src_mask=None, src_key_padding_mask=None, pos=None):"""Performs forward pass with post-normalization."""q = k = self.with_pos_embed(src, pos)src2 = self.ma(q, k, value=src, attn_mask=src_mask, key_padding_mask=src_key_padding_mask)[0]src = src + self.dropout1(src2)src = self.norm1(src)src2 = self.fc2(self.dropout(self.act(self.fc1(src))))src = src + self.dropout2(src2)return self.norm2(src)def forward_pre(self, src, src_mask=None, src_key_padding_mask=None, pos=None):"""Performs forward pass with pre-normalization."""src2 = self.norm1(src)q = k = self.with_pos_embed(src2, pos)src2 = self.ma(q, k, value=src2, attn_mask=src_mask, key_padding_mask=src_key_padding_mask)[0]src = src + self.dropout1(src2)src2 = self.norm2(src)src2 = self.fc2(self.dropout(self.act(self.fc1(src2))))return src + self.dropout2(src2)def forward(self, src, src_mask=None, src_key_padding_mask=None, pos=None):"""Forward propagates the input through the encoder module."""if self.normalize_before:return self.forward_pre(src, src_mask, src_key_padding_mask, pos)return self.forward_post(src, src_mask, src_key_padding_mask, pos)class AIFI(TransformerEncoderLayer):"""Defines the AIFI transformer layer."""def __init__(self, c1, cm=2048, num_heads=8, dropout=0, act=nn.GELU(), normalize_before=False):"""Initialize the AIFI instance with specified parameters."""super().__init__(c1, cm, num_heads, dropout, act, normalize_before)def forward(self, x):"""Forward pass for the AIFI transformer layer."""c, h, w = x.shape[1:]pos_embed = self.build_2d_sincos_position_embedding(w, h, c)# Flatten [B, C, H, W] to [B, HxW, C]x = super().forward(x.flatten(2).permute(0, 2, 1), pos=pos_embed.to(device=x.device, dtype=x.dtype))return x.permute(0, 2, 1).view([-1, c, h, w]).contiguous()@staticmethoddef build_2d_sincos_position_embedding(w, h, embed_dim=256, temperature=10000.0):"""Builds 2D sine-cosine position embedding."""grid_w = torch.arange(int(w), dtype=torch.float32)grid_h = torch.arange(int(h), dtype=torch.float32)grid_w, grid_h = torch.meshgrid(grid_w, grid_h, indexing='ij')assert embed_dim % 4 == 0, \'Embed dimension must be divisible by 4 for 2D sin-cos position embedding'pos_dim = embed_dim // 4omega = torch.arange(pos_dim, dtype=torch.float32) / pos_dimomega = 1. / (temperature ** omega)out_w = grid_w.flatten()[..., None] @ omega[None]out_h = grid_h.flatten()[..., None] @ omega[None]return torch.cat([torch.sin(out_w), torch.cos(out_w), torch.sin(out_h), torch.cos(out_h)], 1)[None]
其次在在YOLOv5/v7项目文件下的models/yolo.py中在文件首部添加代码
from models.aifi import AIFI
并搜索def parse_model(d, ch)
定位到如下行添加以下代码
elif m is AIFI:args = [ch[f], *args]
三、YOLOv7-tiny改进工作
完成二后,在YOLOv7项目文件下的models文件夹下创建新的文件yolov7-tiny-aifi.yaml,导入如下代码。
# parameters
nc: 80 # number of classes
depth_multiple: 1.0 # model depth multiple
width_multiple: 1.0 # layer channel multiple# anchors
anchors:- [10,13, 16,30, 33,23] # P3/8- [30,61, 62,45, 59,119] # P4/16- [116,90, 156,198, 373,326] # P5/32# yolov7-tiny backbone
backbone:# [from, number, module, args] c2, k=1, s=1, p=None, g=1, act=True[[-1, 1, Conv, [32, 3, 2, None, 1, nn.LeakyReLU(0.1)]], # 0-P1/2[-1, 1, Conv, [64, 3, 2, None, 1, nn.LeakyReLU(0.1)]], # 1-P2/4[-1, 1, Conv, [32, 1, 1, None, 1, nn.LeakyReLU(0.1)]],[-2, 1, Conv, [32, 1, 1, None, 1, nn.LeakyReLU(0.1)]],[-1, 1, Conv, [32, 3, 1, None, 1, nn.LeakyReLU(0.1)]],[-1, 1, Conv, [32, 3, 1, None, 1, nn.LeakyReLU(0.1)]],[[-1, -2, -3, -4], 1, Concat, [1]],[-1, 1, Conv, [64, 1, 1, None, 1, nn.LeakyReLU(0.1)]], # 7[-1, 1, MP, []], # 8-P3/8[-1, 1, Conv, [64, 1, 1, None, 1, nn.LeakyReLU(0.1)]],[-2, 1, Conv, [64, 1, 1, None, 1, nn.LeakyReLU(0.1)]],[-1, 1, Conv, [64, 3, 1, None, 1, nn.LeakyReLU(0.1)]],[-1, 1, Conv, [64, 3, 1, None, 1, nn.LeakyReLU(0.1)]],[[-1, -2, -3, -4], 1, Concat, [1]],[-1, 1, Conv, [128, 1, 1, None, 1, nn.LeakyReLU(0.1)]], # 14[-1, 1, MP, []], # 15-P4/16[-1, 1, Conv, [128, 1, 1, None, 1, nn.LeakyReLU(0.1)]],[-2, 1, Conv, [128, 1, 1, None, 1, nn.LeakyReLU(0.1)]],[-1, 1, Conv, [128, 3, 1, None, 1, nn.LeakyReLU(0.1)]],[-1, 1, Conv, [128, 3, 1, None, 1, nn.LeakyReLU(0.1)]],[[-1, -2, -3, -4], 1, Concat, [1]],[-1, 1, Conv, [256, 1, 1, None, 1, nn.LeakyReLU(0.1)]], # 21[-1, 1, MP, []], # 22-P5/32[-1, 1, Conv, [256, 1, 1, None, 1, nn.LeakyReLU(0.1)]],[-2, 1, Conv, [256, 1, 1, None, 1, nn.LeakyReLU(0.1)]],[-1, 1, Conv, [256, 3, 1, None, 1, nn.LeakyReLU(0.1)]],[-1, 1, Conv, [256, 3, 1, None, 1, nn.LeakyReLU(0.1)]],[[-1, -2, -3, -4], 1, Concat, [1]],[-1, 1, Conv, [512, 1, 1, None, 1, nn.LeakyReLU(0.1)]], # 28]# yolov7-tiny head
head:[[-1, 1, AIFI, [256]], # 29[-1, 1, Conv, [128, 1, 1, None, 1, nn.LeakyReLU(0.1)]],[-1, 1, nn.Upsample, [None, 2, 'nearest']],[21, 1, Conv, [128, 1, 1, None, 1, nn.LeakyReLU(0.1)]], # route backbone P4[[-1, -2], 1, Concat, [1]],[-1, 1, Conv, [64, 1, 1, None, 1, nn.LeakyReLU(0.1)]],[-2, 1, Conv, [64, 1, 1, None, 1, nn.LeakyReLU(0.1)]],[-1, 1, Conv, [64, 3, 1, None, 1, nn.LeakyReLU(0.1)]],[-1, 1, Conv, [64, 3, 1, None, 1, nn.LeakyReLU(0.1)]],[[-1, -2, -3, -4], 1, Concat, [1]],[-1, 1, Conv, [128, 1, 1, None, 1, nn.LeakyReLU(0.1)]], # 39[-1, 1, Conv, [64, 1, 1, None, 1, nn.LeakyReLU(0.1)]],[-1, 1, nn.Upsample, [None, 2, 'nearest']],[14, 1, Conv, [64, 1, 1, None, 1, nn.LeakyReLU(0.1)]], # route backbone P3[[-1, -2], 1, Concat, [1]],[-1, 1, Conv, [32, 1, 1, None, 1, nn.LeakyReLU(0.1)]],[-2, 1, Conv, [32, 1, 1, None, 1, nn.LeakyReLU(0.1)]],[-1, 1, Conv, [32, 3, 1, None, 1, nn.LeakyReLU(0.1)]],[-1, 1, Conv, [32, 3, 1, None, 1, nn.LeakyReLU(0.1)]],[[-1, -2, -3, -4], 1, Concat, [1]],[-1, 1, Conv, [64, 1, 1, None, 1, nn.LeakyReLU(0.1)]], # 49[-1, 1, Conv, [128, 3, 2, None, 1, nn.LeakyReLU(0.1)]],[[-1, 39], 1, Concat, [1]],[-1, 1, Conv, [64, 1, 1, None, 1, nn.LeakyReLU(0.1)]],[-2, 1, Conv, [64, 1, 1, None, 1, nn.LeakyReLU(0.1)]],[-1, 1, Conv, [64, 3, 1, None, 1, nn.LeakyReLU(0.1)]],[-1, 1, Conv, [64, 3, 1, None, 1, nn.LeakyReLU(0.1)]],[[-1, -2, -3, -4], 1, Concat, [1]],[-1, 1, Conv, [128, 1, 1, None, 1, nn.LeakyReLU(0.1)]], # 57[-1, 1, Conv, [256, 3, 2, None, 1, nn.LeakyReLU(0.1)]],[[-1, 29], 1, Concat, [1]],[-1, 1, Conv, [128, 1, 1, None, 1, nn.LeakyReLU(0.1)]],[-2, 1, Conv, [128, 1, 1, None, 1, nn.LeakyReLU(0.1)]],[-1, 1, Conv, [128, 3, 1, None, 1, nn.LeakyReLU(0.1)]],[-1, 1, Conv, [128, 3, 1, None, 1, nn.LeakyReLU(0.1)]],[[-1, -2, -3, -4], 1, Concat, [1]],[-1, 1, Conv, [256, 1, 1, None, 1, nn.LeakyReLU(0.1)]], # 65[49, 1, Conv, [128, 3, 1, None, 1, nn.LeakyReLU(0.1)]],[57, 1, Conv, [256, 3, 1, None, 1, nn.LeakyReLU(0.1)]],[65, 1, Conv, [512, 3, 1, None, 1, nn.LeakyReLU(0.1)]],[[66, 67, 68], 1, IDetect, [nc, anchors]], # Detect(P3, P4, P5)]
from n params module arguments 0 -1 1 928 models.common.Conv [3, 32, 3, 2, None, 1, LeakyReLU(negative_slope=0.1)]1 -1 1 18560 models.common.Conv [32, 64, 3, 2, None, 1, LeakyReLU(negative_slope=0.1)]2 -1 1 2112 models.common.Conv [64, 32, 1, 1, None, 1, LeakyReLU(negative_slope=0.1)]3 -2 1 2112 models.common.Conv [64, 32, 1, 1, None, 1, LeakyReLU(negative_slope=0.1)]4 -1 1 9280 models.common.Conv [32, 32, 3, 1, None, 1, LeakyReLU(negative_slope=0.1)]5 -1 1 9280 models.common.Conv [32, 32, 3, 1, None, 1, LeakyReLU(negative_slope=0.1)]6 [-1, -2, -3, -4] 1 0 models.common.Concat [1] 7 -1 1 8320 models.common.Conv [128, 64, 1, 1, None, 1, LeakyReLU(negative_slope=0.1)]8 -1 1 0 models.common.MP [] 9 -1 1 4224 models.common.Conv [64, 64, 1, 1, None, 1, LeakyReLU(negative_slope=0.1)]10 -2 1 4224 models.common.Conv [64, 64, 1, 1, None, 1, LeakyReLU(negative_slope=0.1)]11 -1 1 36992 models.common.Conv [64, 64, 3, 1, None, 1, LeakyReLU(negative_slope=0.1)]12 -1 1 36992 models.common.Conv [64, 64, 3, 1, None, 1, LeakyReLU(negative_slope=0.1)]13 [-1, -2, -3, -4] 1 0 models.common.Concat [1] 14 -1 1 33024 models.common.Conv [256, 128, 1, 1, None, 1, LeakyReLU(negative_slope=0.1)]15 -1 1 0 models.common.MP [] 16 -1 1 16640 models.common.Conv [128, 128, 1, 1, None, 1, LeakyReLU(negative_slope=0.1)]17 -2 1 16640 models.common.Conv [128, 128, 1, 1, None, 1, LeakyReLU(negative_slope=0.1)]18 -1 1 147712 models.common.Conv [128, 128, 3, 1, None, 1, LeakyReLU(negative_slope=0.1)]19 -1 1 147712 models.common.Conv [128, 128, 3, 1, None, 1, LeakyReLU(negative_slope=0.1)]20 [-1, -2, -3, -4] 1 0 models.common.Concat [1] 21 -1 1 131584 models.common.Conv [512, 256, 1, 1, None, 1, LeakyReLU(negative_slope=0.1)]22 -1 1 0 models.common.MP [] 23 -1 1 66048 models.common.Conv [256, 256, 1, 1, None, 1, LeakyReLU(negative_slope=0.1)]24 -2 1 66048 models.common.Conv [256, 256, 1, 1, None, 1, LeakyReLU(negative_slope=0.1)]25 -1 1 590336 models.common.Conv [256, 256, 3, 1, None, 1, LeakyReLU(negative_slope=0.1)]26 -1 1 590336 models.common.Conv [256, 256, 3, 1, None, 1, LeakyReLU(negative_slope=0.1)]27 [-1, -2, -3, -4] 1 0 models.common.Concat [1] 28 -1 1 525312 models.common.Conv [1024, 512, 1, 1, None, 1, LeakyReLU(negative_slope=0.1)]29 -1 1 1315584 models.aifi.AIFI [512, 256] 30 -1 1 65792 models.common.Conv [512, 128, 1, 1, None, 1, LeakyReLU(negative_slope=0.1)]31 -1 1 0 torch.nn.modules.upsampling.Upsample [None, 2, 'nearest'] 32 21 1 33024 models.common.Conv [256, 128, 1, 1, None, 1, LeakyReLU(negative_slope=0.1)]33 [-1, -2] 1 0 models.common.Concat [1] 34 -1 1 16512 models.common.Conv [256, 64, 1, 1, None, 1, LeakyReLU(negative_slope=0.1)]35 -2 1 16512 models.common.Conv [256, 64, 1, 1, None, 1, LeakyReLU(negative_slope=0.1)]36 -1 1 36992 models.common.Conv [64, 64, 3, 1, None, 1, LeakyReLU(negative_slope=0.1)]37 -1 1 36992 models.common.Conv [64, 64, 3, 1, None, 1, LeakyReLU(negative_slope=0.1)]38 [-1, -2, -3, -4] 1 0 models.common.Concat [1] 39 -1 1 33024 models.common.Conv [256, 128, 1, 1, None, 1, LeakyReLU(negative_slope=0.1)]40 -1 1 8320 models.common.Conv [128, 64, 1, 1, None, 1, LeakyReLU(negative_slope=0.1)]41 -1 1 0 torch.nn.modules.upsampling.Upsample [None, 2, 'nearest'] 42 14 1 8320 models.common.Conv [128, 64, 1, 1, None, 1, LeakyReLU(negative_slope=0.1)]43 [-1, -2] 1 0 models.common.Concat [1] 44 -1 1 4160 models.common.Conv [128, 32, 1, 1, None, 1, LeakyReLU(negative_slope=0.1)]45 -2 1 4160 models.common.Conv [128, 32, 1, 1, None, 1, LeakyReLU(negative_slope=0.1)]46 -1 1 9280 models.common.Conv [32, 32, 3, 1, None, 1, LeakyReLU(negative_slope=0.1)]47 -1 1 9280 models.common.Conv [32, 32, 3, 1, None, 1, LeakyReLU(negative_slope=0.1)]48 [-1, -2, -3, -4] 1 0 models.common.Concat [1] 49 -1 1 8320 models.common.Conv [128, 64, 1, 1, None, 1, LeakyReLU(negative_slope=0.1)]50 -1 1 73984 models.common.Conv [64, 128, 3, 2, None, 1, LeakyReLU(negative_slope=0.1)]51 [-1, 39] 1 0 models.common.Concat [1] 52 -1 1 16512 models.common.Conv [256, 64, 1, 1, None, 1, LeakyReLU(negative_slope=0.1)]53 -2 1 16512 models.common.Conv [256, 64, 1, 1, None, 1, LeakyReLU(negative_slope=0.1)]54 -1 1 36992 models.common.Conv [64, 64, 3, 1, None, 1, LeakyReLU(negative_slope=0.1)]55 -1 1 36992 models.common.Conv [64, 64, 3, 1, None, 1, LeakyReLU(negative_slope=0.1)]56 [-1, -2, -3, -4] 1 0 models.common.Concat [1] 57 -1 1 33024 models.common.Conv [256, 128, 1, 1, None, 1, LeakyReLU(negative_slope=0.1)]58 -1 1 295424 models.common.Conv [128, 256, 3, 2, None, 1, LeakyReLU(negative_slope=0.1)]59 [-1, 29] 1 0 models.common.Concat [1] 60 -1 1 98560 models.common.Conv [768, 128, 1, 1, None, 1, LeakyReLU(negative_slope=0.1)]61 -2 1 98560 models.common.Conv [768, 128, 1, 1, None, 1, LeakyReLU(negative_slope=0.1)]62 -1 1 147712 models.common.Conv [128, 128, 3, 1, None, 1, LeakyReLU(negative_slope=0.1)]63 -1 1 147712 models.common.Conv [128, 128, 3, 1, None, 1, LeakyReLU(negative_slope=0.1)]64 [-1, -2, -3, -4] 1 0 models.common.Concat [1] 65 -1 1 131584 models.common.Conv [512, 256, 1, 1, None, 1, LeakyReLU(negative_slope=0.1)]66 49 1 73984 models.common.Conv [64, 128, 3, 1, None, 1, LeakyReLU(negative_slope=0.1)]67 57 1 295424 models.common.Conv [128, 256, 3, 1, None, 1, LeakyReLU(negative_slope=0.1)]68 65 1 1180672 models.common.Conv [256, 512, 3, 1, None, 1, LeakyReLU(negative_slope=0.1)]69 [66, 67, 68] 1 17132 models.yolo.IDetect [1, [[10, 13, 16, 30, 33, 23], [30, 61, 62, 45, 59, 119], [116, 90, 156, 198, 373, 326]], [128, 256, 512]]Model Summary: 250 layers, 6771468 parameters, 6771468 gradients, 12.9 GFLOPS
运行后若打印出如上文本代表改进成功。
四、YOLOv5s改进工作
完成二后,在YOLOv5项目文件下的models文件夹下创建新的文件yolov5s-aifi.yaml,导入如下代码。
nc: 80 # number of classes
depth_multiple: 0.33 # model depth multiple
width_multiple: 0.50 # layer channel multiple
anchors:- [10,13, 16,30, 33,23] # P3/8- [30,61, 62,45, 59,119] # P4/16- [116,90, 156,198, 373,326] # P5/32# YOLOv5 v6.0 backbone
backbone:# [from, number, module, args][[-1, 1, Conv, [64, 6, 2, 2]], # 0-P1/2[-1, 1, Conv, [128, 3, 2]], # 1-P2/4[-1, 3, C3, [128]],[-1, 1, Conv, [256, 3, 2]], # 3-P3/8[-1, 6, C3, [256]],[-1, 1, Conv, [512, 3, 2]], # 5-P4/16[-1, 9, C3, [512]],[-1, 1, Conv, [1024, 3, 2]], # 7-P5/32[-1, 3, C3, [1024]],[-1, 1, Conv, [512, 1]], # 9[-1, 1, AIFI, [1024, 8]], # 10]# YOLOv5 v6.0 head
head:[[-1, 1, nn.Upsample, [None, 2, 'nearest']],[[-1, 6], 1, Concat, [1]], # cat backbone P4[-1, 3, C3, [512, False]], # 13[-1, 1, Conv, [256, 1, 1]],[-1, 1, nn.Upsample, [None, 2, 'nearest']],[[-1, 4], 1, Concat, [1]], # cat backbone P3[-1, 3, C3, [256, False]], # 17 (P3/8-small)[-1, 1, Conv, [256, 3, 2]],[[-1, 14], 1, Concat, [1]], # cat head P4[-1, 3, C3, [512, False]], # 20 (P4/16-medium)[-1, 1, Conv, [512, 3, 2]],[[-1, 10], 1, Concat, [1]], # cat head P5[-1, 3, C3, [1024, False]], # 23 (P5/32-large)[[17, 20, 23], 1, Detect, [nc, anchors]], # Detect(P3, P4, P5)]
from n params module arguments 0 -1 1 3520 models.common.Conv [3, 32, 6, 2, 2] 1 -1 1 18560 models.common.Conv [32, 64, 3, 2] 2 -1 1 18816 models.common.C3 [64, 64, 1] 3 -1 1 73984 models.common.Conv [64, 128, 3, 2] 4 -1 2 115712 models.common.C3 [128, 128, 2] 5 -1 1 295424 models.common.Conv [128, 256, 3, 2] 6 -1 3 625152 models.common.C3 [256, 256, 3] 7 -1 1 1180672 models.common.Conv [256, 512, 3, 2] 8 -1 1 1182720 models.common.C3 [512, 512, 1] 9 -1 1 131584 models.common.Conv [512, 256, 1] 10 -1 1 789760 models.aifi.AIFI [256, 1024, 8] 11 -1 1 0 torch.nn.modules.upsampling.Upsample [None, 2, 'nearest'] 12 [-1, 6] 1 0 models.common.Concat [1] 13 -1 1 361984 models.common.C3 [512, 256, 1, False] 14 -1 1 33024 models.common.Conv [256, 128, 1, 1] 15 -1 1 0 torch.nn.modules.upsampling.Upsample [None, 2, 'nearest'] 16 [-1, 4] 1 0 models.common.Concat [1] 17 -1 1 90880 models.common.C3 [256, 128, 1, False] 18 -1 1 147712 models.common.Conv [128, 128, 3, 2] 19 [-1, 14] 1 0 models.common.Concat [1] 20 -1 1 296448 models.common.C3 [256, 256, 1, False] 21 -1 1 590336 models.common.Conv [256, 256, 3, 2] 22 [-1, 10] 1 0 models.common.Concat [1] 23 -1 1 1182720 models.common.C3 [512, 512, 1, False] 24 [17, 20, 23] 1 16182 models.yolo.Detect [1, [[10, 13, 16, 30, 33, 23], [30, 61, 62, 45, 59, 119], [116, 90, 156, 198, 373, 326]], [128, 256, 512]]Model Summary: 271 layers, 7155190 parameters, 7155190 gradients, 15.8 GFLOPs
运行后若打印出如上文本代表改进成功。
五、YOLOv5n改进工作
完成二后,在YOLOv5项目文件下的models文件夹下创建新的文件yolov5n-aifi.yaml,导入如下代码。
nc: 80 # number of classes
depth_multiple: 0.33 # model depth multiple
width_multiple: 0.25 # layer channel multiple
anchors:- [10,13, 16,30, 33,23] # P3/8- [30,61, 62,45, 59,119] # P4/16- [116,90, 156,198, 373,326] # P5/32# YOLOv5 v6.0 backbone
backbone:# [from, number, module, args][[-1, 1, Conv, [64, 6, 2, 2]], # 0-P1/2[-1, 1, Conv, [128, 3, 2]], # 1-P2/4[-1, 3, C3, [128]],[-1, 1, Conv, [256, 3, 2]], # 3-P3/8[-1, 6, C3, [256]],[-1, 1, Conv, [512, 3, 2]], # 5-P4/16[-1, 9, C3, [512]],[-1, 1, Conv, [1024, 3, 2]], # 7-P5/32[-1, 3, C3, [1024]],[-1, 1, Conv, [512, 1]], # 9[-1, 1, AIFI, [1024, 8]], # 10]# YOLOv5 v6.0 head
head:[[-1, 1, nn.Upsample, [None, 2, 'nearest']],[[-1, 6], 1, Concat, [1]], # cat backbone P4[-1, 3, C3, [512, False]], # 13[-1, 1, Conv, [256, 1, 1]],[-1, 1, nn.Upsample, [None, 2, 'nearest']],[[-1, 4], 1, Concat, [1]], # cat backbone P3[-1, 3, C3, [256, False]], # 17 (P3/8-small)[-1, 1, Conv, [256, 3, 2]],[[-1, 14], 1, Concat, [1]], # cat head P4[-1, 3, C3, [512, False]], # 20 (P4/16-medium)[-1, 1, Conv, [512, 3, 2]],[[-1, 10], 1, Concat, [1]], # cat head P5[-1, 3, C3, [1024, False]], # 23 (P5/32-large)[[17, 20, 23], 1, Detect, [nc, anchors]], # Detect(P3, P4, P5)]
from n params module arguments 0 -1 1 1760 models.common.Conv [3, 16, 6, 2, 2] 1 -1 1 4672 models.common.Conv [16, 32, 3, 2] 2 -1 1 4800 models.common.C3 [32, 32, 1] 3 -1 1 18560 models.common.Conv [32, 64, 3, 2] 4 -1 2 29184 models.common.C3 [64, 64, 2] 5 -1 1 73984 models.common.Conv [64, 128, 3, 2] 6 -1 3 156928 models.common.C3 [128, 128, 3] 7 -1 1 295424 models.common.Conv [128, 256, 3, 2] 8 -1 1 296448 models.common.C3 [256, 256, 1] 9 -1 1 33024 models.common.Conv [256, 128, 1] 10 -1 1 329856 models.aifi.AIFI [128, 1024, 8] 11 -1 1 0 torch.nn.modules.upsampling.Upsample [None, 2, 'nearest'] 12 [-1, 6] 1 0 models.common.Concat [1] 13 -1 1 90880 models.common.C3 [256, 128, 1, False] 14 -1 1 8320 models.common.Conv [128, 64, 1, 1] 15 -1 1 0 torch.nn.modules.upsampling.Upsample [None, 2, 'nearest'] 16 [-1, 4] 1 0 models.common.Concat [1] 17 -1 1 22912 models.common.C3 [128, 64, 1, False] 18 -1 1 36992 models.common.Conv [64, 64, 3, 2] 19 [-1, 14] 1 0 models.common.Concat [1] 20 -1 1 74496 models.common.C3 [128, 128, 1, False] 21 -1 1 147712 models.common.Conv [128, 128, 3, 2] 22 [-1, 10] 1 0 models.common.Concat [1] 23 -1 1 296448 models.common.C3 [256, 256, 1, False] 24 [17, 20, 23] 1 8118 models.yolo.Detect [1, [[10, 13, 16, 30, 33, 23], [30, 61, 62, 45, 59, 119], [116, 90, 156, 198, 373, 326]], [64, 128, 256]]Model Summary: 271 layers, 1930518 parameters, 1930518 gradients, 4.3 GFLOPs
运行后打印如上代码说明改进成功。
更多文章产出中,主打简洁和准确,欢迎关注我,共同探讨!
相关文章:
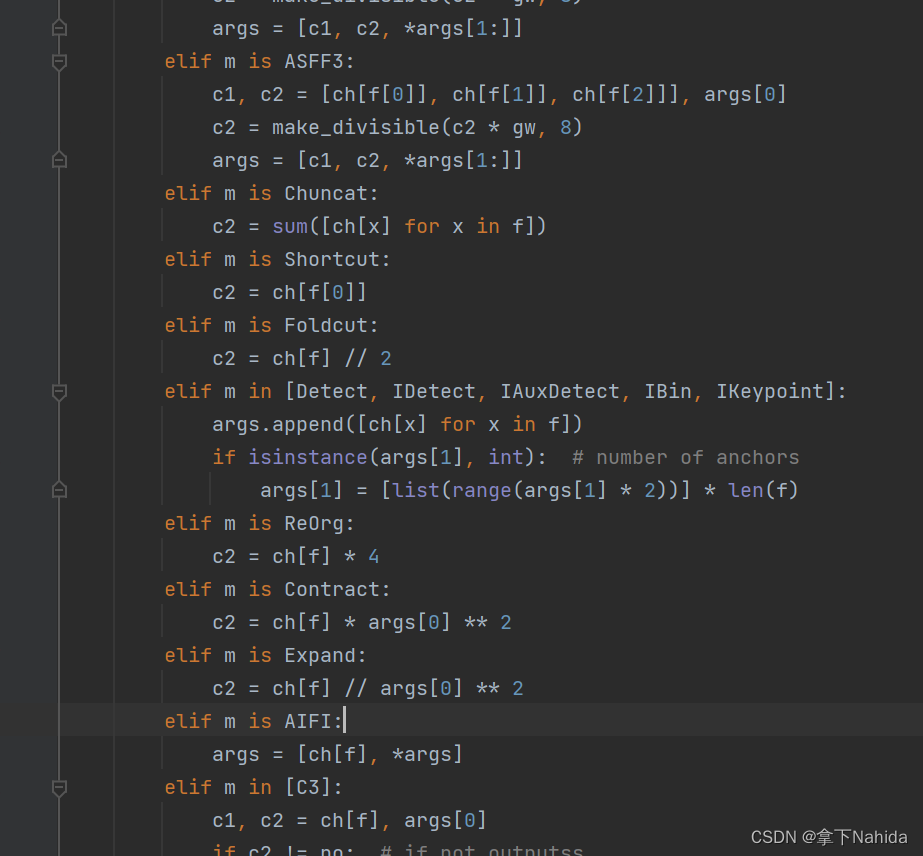
【YOLOv5/v7改进系列】改进池化层为RT-DETR的AIFI
一、导言 Real-Time DEtection TRansformer(RT-DETR),是一种实时端到端目标检测器,克服了Non-Maximum Suppression(NMS)对速度和准确性的影响。通过设计高效的混合编码器和不确定性最小化查询选择…...
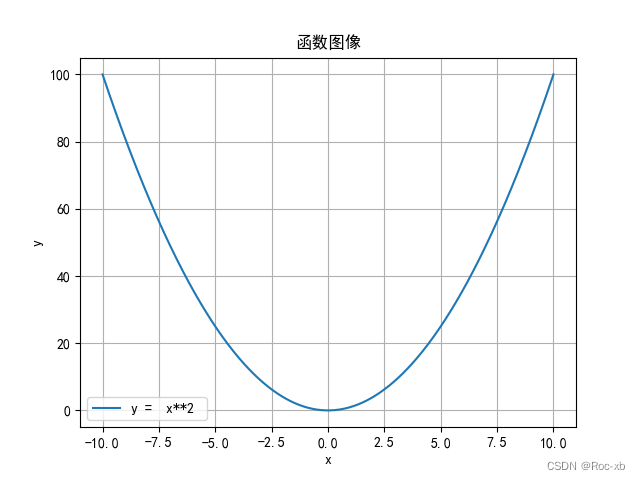
使用Python和Matplotlib绘制复杂数学函数图像
本文介绍了如何使用Python编程语言和Matplotlib库来绘制复杂的数学函数图像。通过引入NumPy库的数学函数,我们可以处理包括指数函数在内的各种复杂表达式。本文详细讲解了如何设置中文字体以确保在图像中正确显示中文标题和标签,并提供了一个完整的代码示例,用户可以通过输入…...

淘宝/1688获得店铺的所有商品(商品列表)
通过以下步骤,可以获得淘宝或1688店铺的所有商品。请注意,具体步骤可能会因为平台的更新而有所改变,可以根据实际情况进行操作。 更多API调用展示以及获取Key和secret请移步 返回数据格式: {"user": null,"ite…...
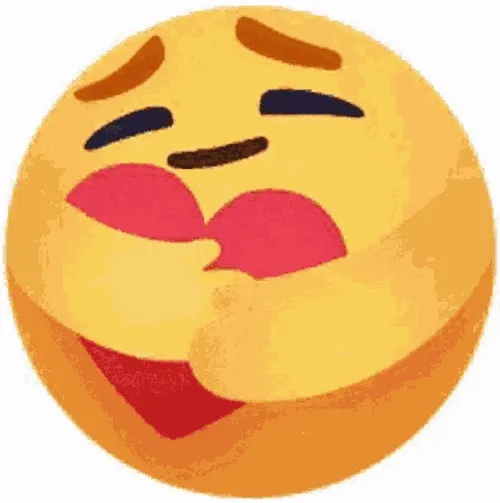
【MySQL】锁机制
创作不易,本篇文章如果帮助到了你,还请点赞 关注支持一下♡>𖥦<)!! 主页专栏有更多知识,如有疑问欢迎大家指正讨论,共同进步! 给大家跳段街舞感谢支持!ጿ ኈ ቼ ዽ ጿ ኈ ቼ ዽ ጿ ኈ ቼ …...

LangChain入门学习笔记(一)——Hello World
什么是LangChain LangChain是一个开源(github repo)的大语言模型应用开发框架,提供了一整套的工具、方法和接口去帮助程序员构建基于大语言模型的端到端应用。LangChain是长链(long chain)的意思,它的一个…...
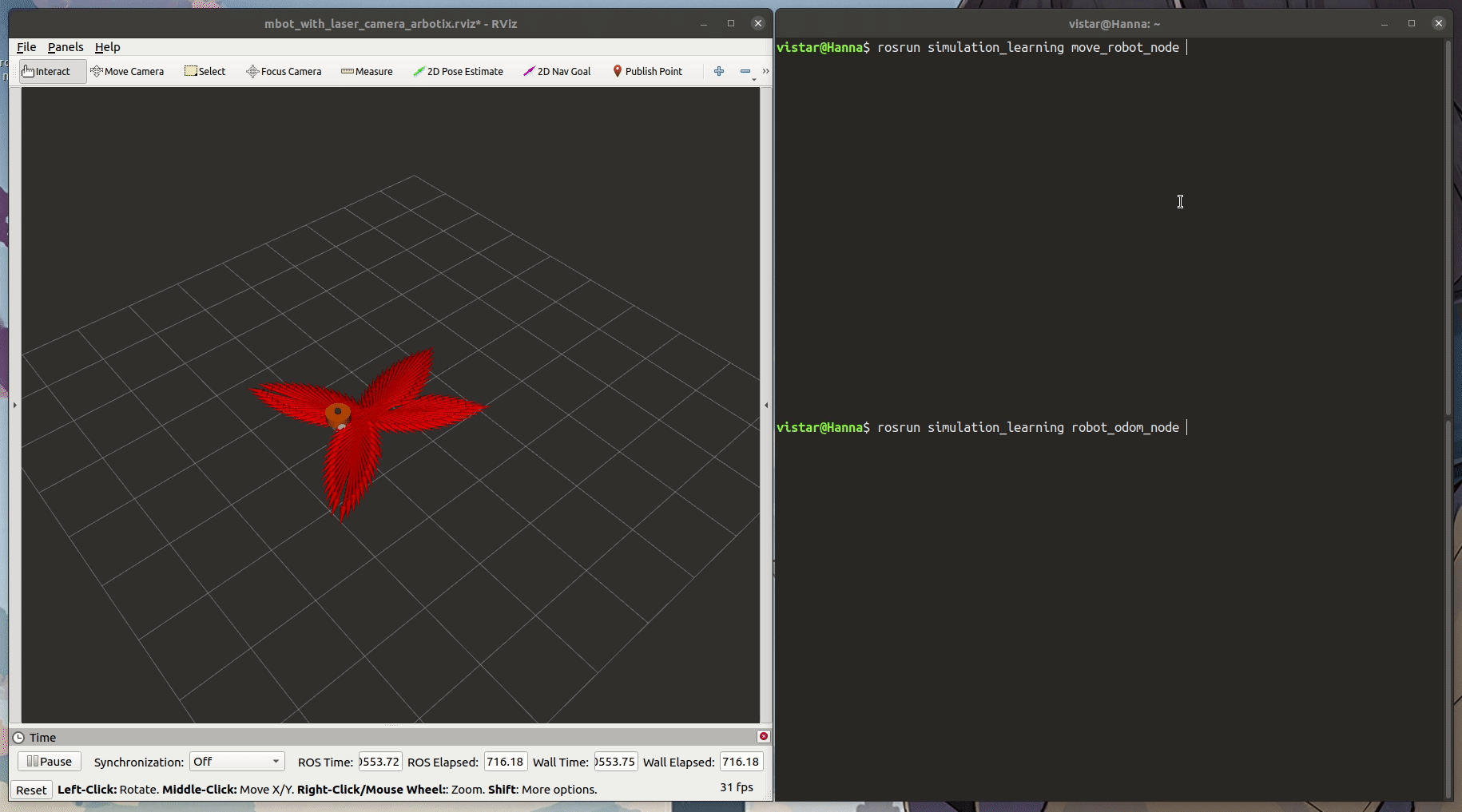
[ROS 系列学习教程] 建模与仿真 - 使用 Arbotix 控制机器人
ROS 系列学习教程(总目录) 本文目录 一、Arbotix 简介二、安装Arbotix三、配置Arbotix控制器四、配置launch启动文件五、数据交互接口六、在rviz中仿真控制机器人6.1 直接发topic控制6.2 使用键盘控制6.3 编写代码控制机器人移动 前面讲了机器人的建模,是静态的&…...

java:使用JSqlParser给sql语句增加tenant_id和deleted条件
# 示例代码 【pom.xml】 <dependency><groupId>com.baomidou</groupId><artifactId>mybatis-plus-core</artifactId><version>3.4.3.1</version> </dependency>【MyJSqlParserTest.java】 package com.chz.myJSqlParser;pu…...
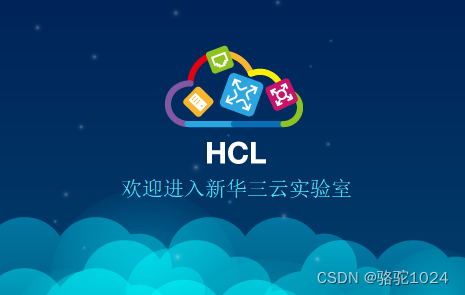
华三HCL模拟器安装及华三防火墙配置
0、前言 最近跟模拟器杠上了,主要是需要运行防火墙,目前已经成功模拟出华为、山石防火墙,而且模拟出来的设备能与物理网络环境进行互联。现在我又盯上华三防火墙了。 首先下载模拟器: 下载地址:H3C网络设备模拟器官方免…...
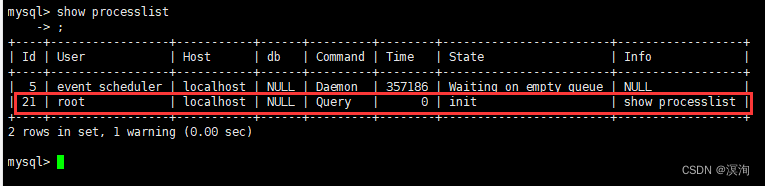
MySQL基础---库的操作和表的操作(配着自己的实操图,简单易上手)
绪论 勿问成功的秘诀为何,且尽全力做您应该做的事吧。–美华纳;本章是MySQL的第二章,本章主要写道MySQL中库和表的增删查改以及对库和表的备份处理,本章是基于上一章所写若没安装mysql可以查看Linux下搭建mysql软件及登录和基本…...
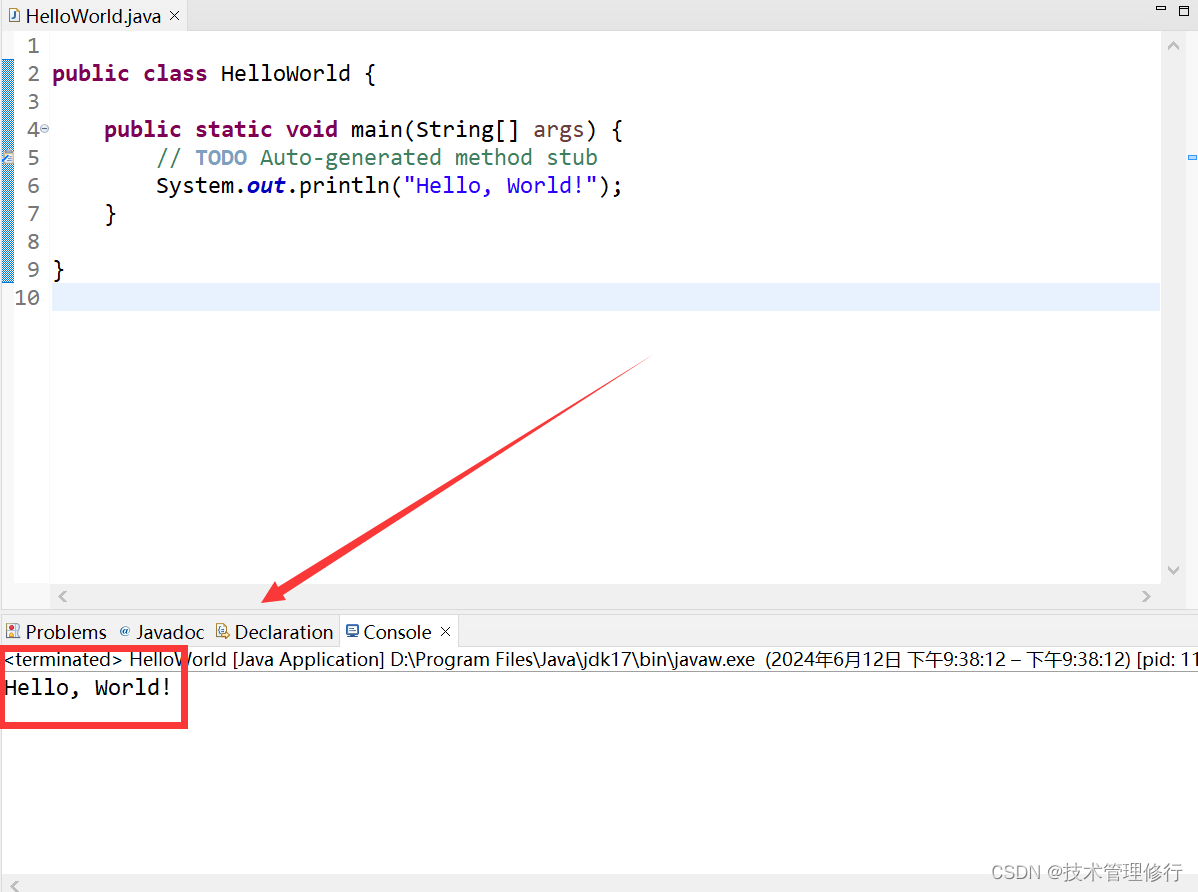
【6】第一个Java程序:Hello World
一、引言 Java,作为一种广泛使用的编程语言,其强大的跨平台能力和丰富的库函数使其成为开发者的首选。对于初学者来说,编写并运行第一个Java程序是一个令人兴奋的时刻。本文将指导你使用Eclipse这一流行的集成开发环境(IDE&#…...
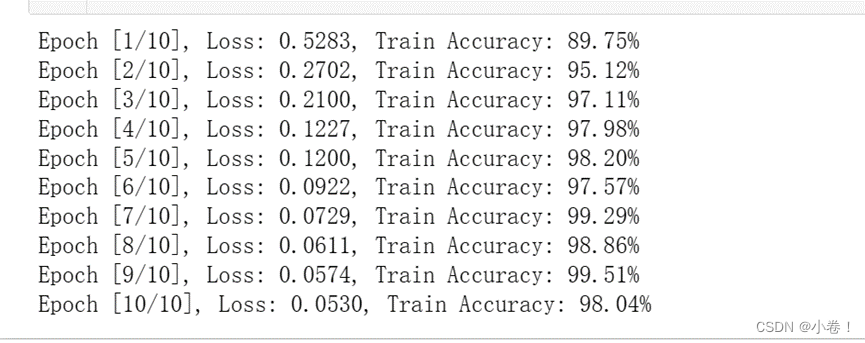
pytorch神经网络训练(AlexNet)
导包 import osimport torchimport torch.nn as nnimport torch.optim as optimfrom torch.utils.data import Dataset, DataLoaderfrom PIL import Imagefrom torchvision import models, transforms 定义自定义图像数据集 class CustomImageDataset(Dataset): 定义一个自…...
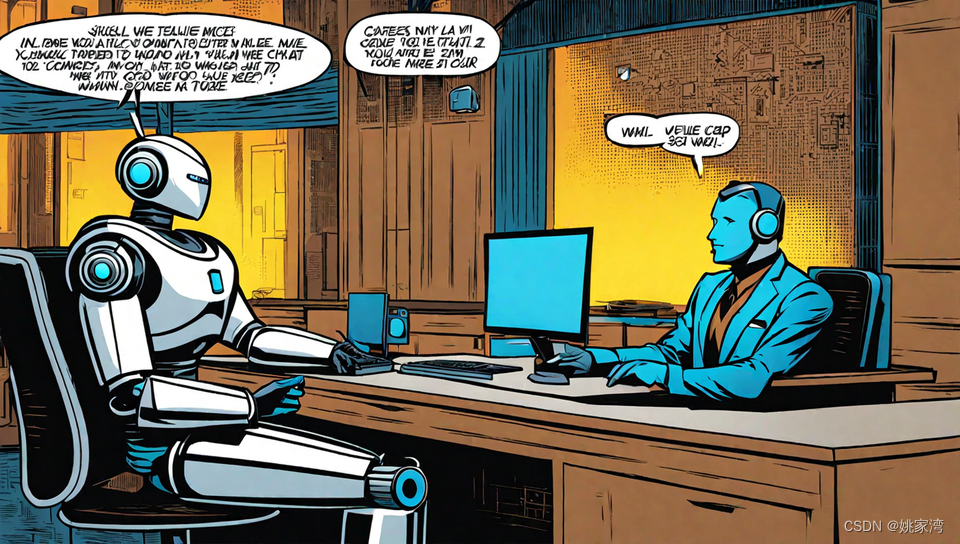
构建大语言模型友好型网站
以大语言模型为代表的AI 技术迅速发展,将会影响原有信息网络的方式。其中一个明显的趋势是通过chatGPT 对话代替搜索引擎和浏览器来获取信息。 互联网时代,主要是通过网站(website)提供信息。网站主要为人类阅读的方式构建的。主要…...
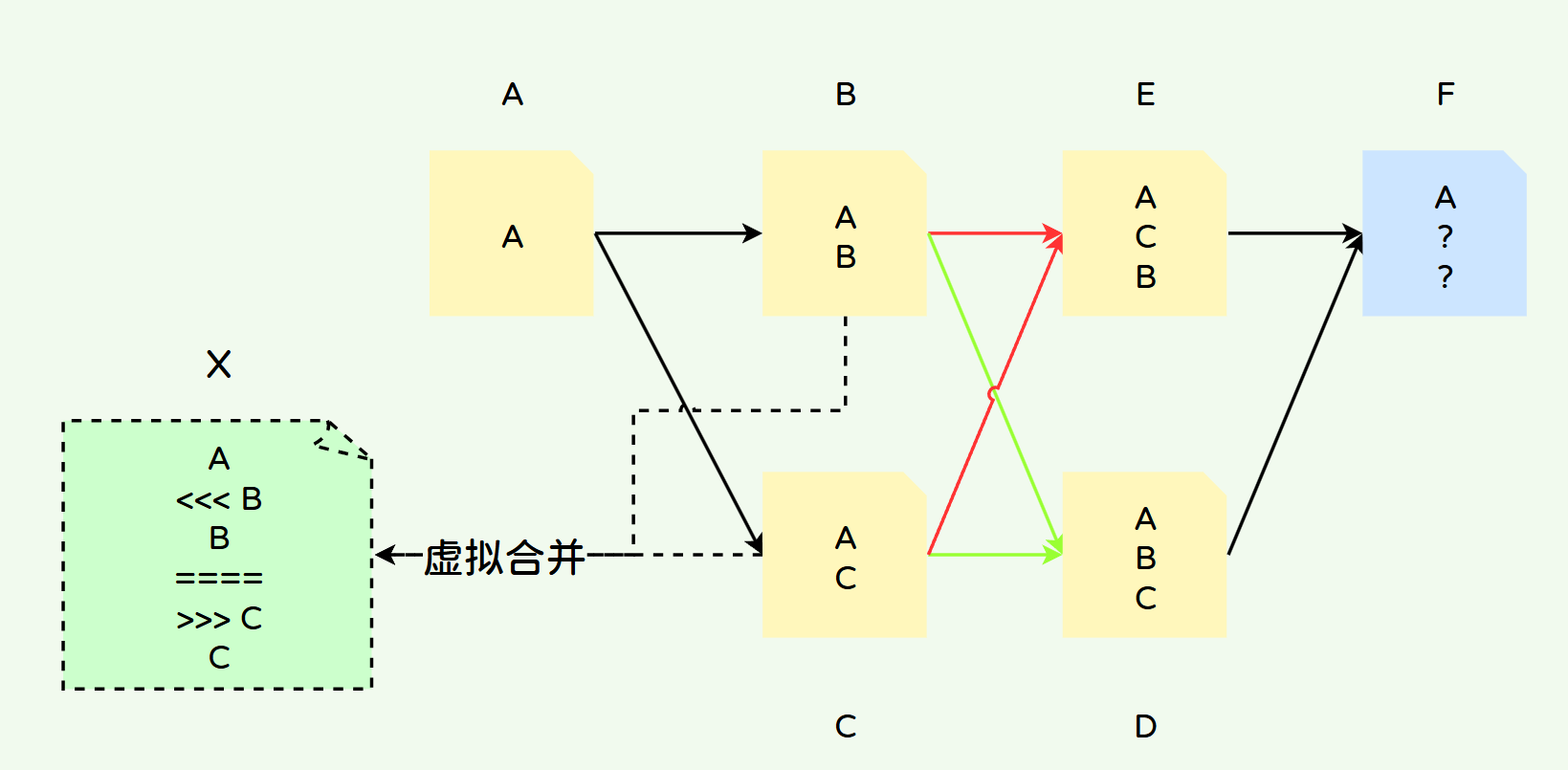
Git代码冲突原理与三路合并算法
Git代码冲突原理 Git合并文件是以行为单位进行一行一行合并的,但是有些时候并不是两行内容不一样Git就会报冲突,这是因为Git会帮助我们进行分析得出哪个结果是我们所期望的最终结果。而这个分析依据就是三路合并算法。当然,三路合并算法并不…...
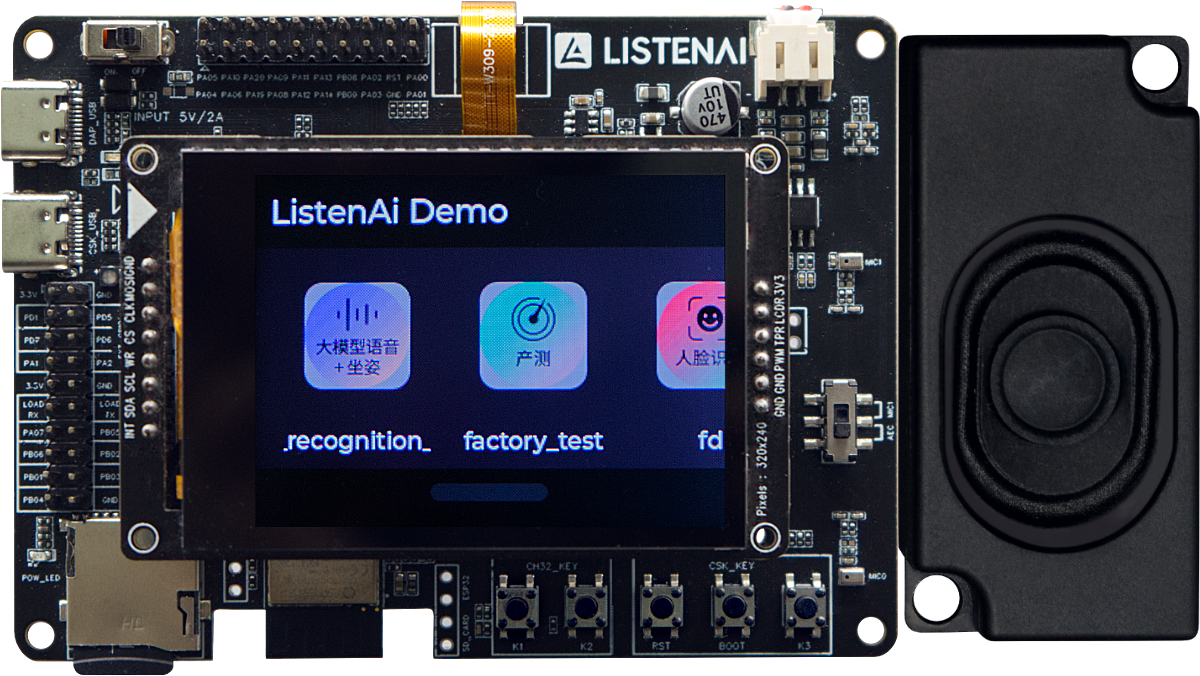
聆思CSK6大模型开发板英语评测类开源SDK详解
离线英文评测算法SDK 能力简介 CSK6 大模型开发套件可以对用户通过语音输入的英文单词进行精准识别,并对单词的发音、错读、漏读、多读等方面进行评估,进行音素级的识别,根据用户的发音给出相应的建议和纠正,帮助用户更好地掌握单…...
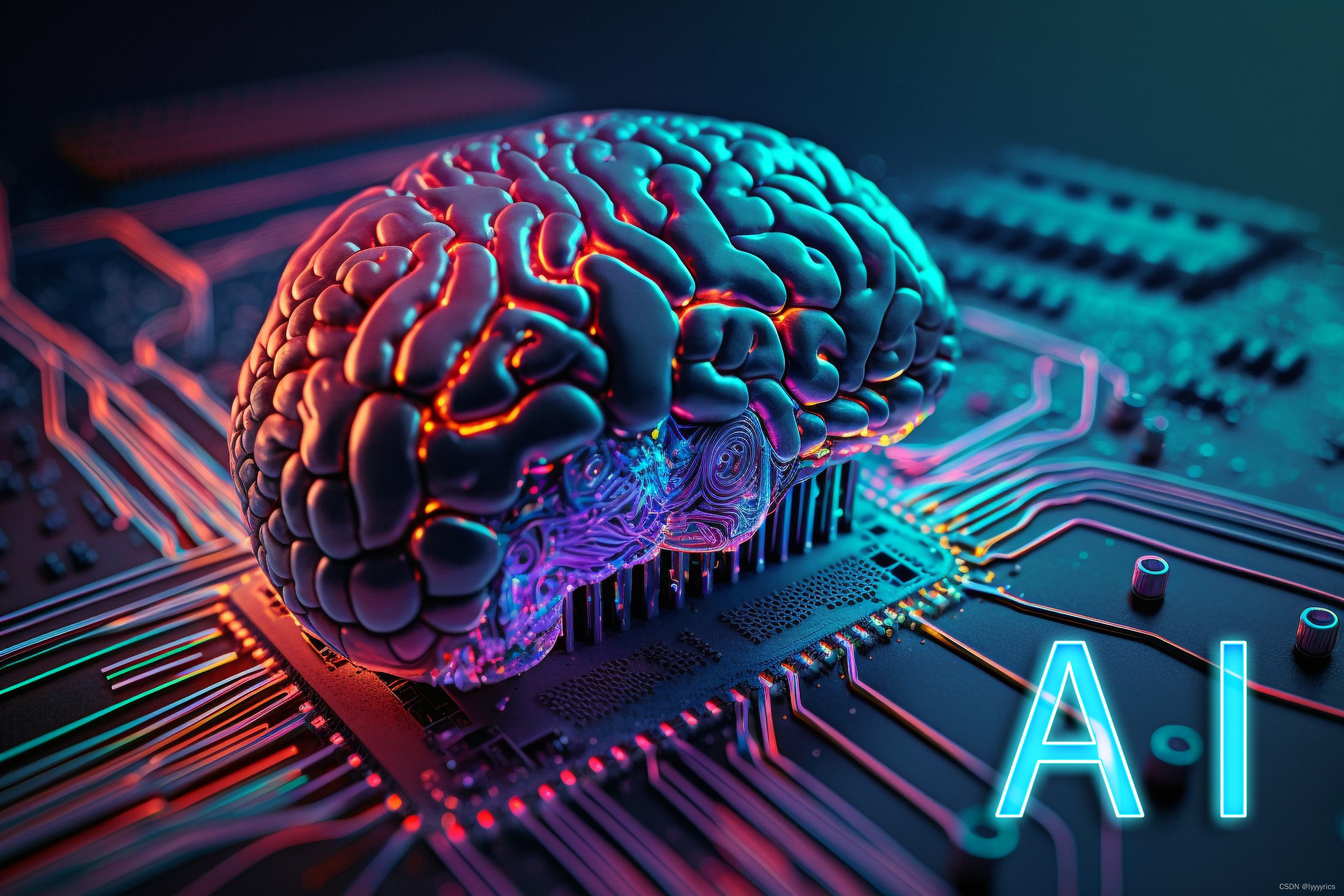
通用大模型VS垂直大模型,你更青睐哪一方?
这里写目录标题 一、通用大模型简介二、垂直大模型简介三、通用大模型与垂直大模型的比较四、如何选择适合的模型五、通用大模型和垂直大模型的应用场景六、总结 近年来,随着人工智能技术的飞速发展,大模型的应用越来越广泛。无论是自然语言处理、计算机…...
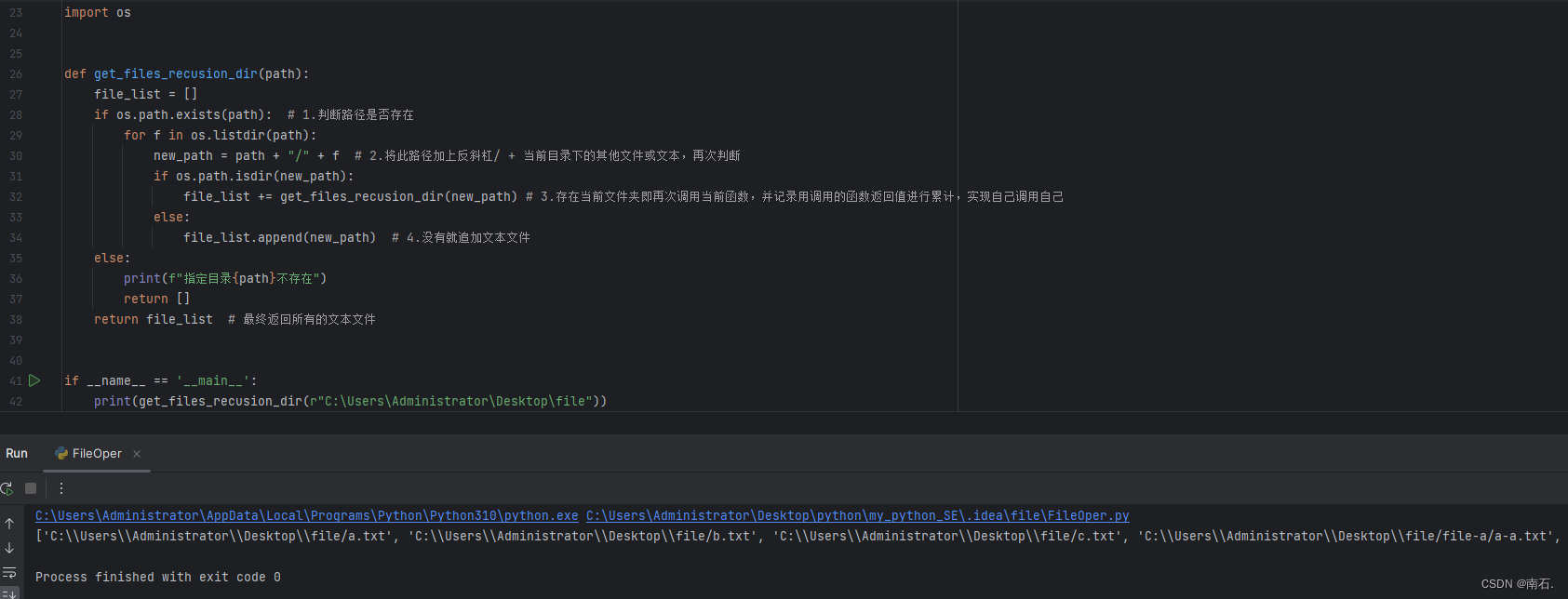
Python第二语言(十四、高阶基础)
目录 1. 闭包 1.1 使用闭包注意事项 1.2 小结 2. 装饰器:实际上也是一种闭包; 2.1 装饰器的写法(闭包写法) :基础写法,只是解释装饰器是怎么写的; 2.2 装饰器的语法糖写法:函数…...

python脚本之调用其他目录脚本
import sys# 添加新路径到搜索路径中 sys.path.append(/脚本父级)# 现在可以导入该路径下的模块了 from 脚本 import 方法方法()...

C# 事件(Event)定义及其使用
1.定义个委托和类 //委托 public delegate void ProductEventHandler(Product product);/// <summary> /// 产品 /// </summary> public class Product {public int Id { get; set; }public string Code { get; set; }public string Name { get; set; }private de…...

2.负载压力测试
负载压力测试是一种重要的系统测试方法,旨在评估系统在正常和峰值负载情况下的性能表现。 一、基本概念: 负载压力测试是在一定约束条件下,通过模拟实际用户访问系统的行为,来测试系统所能承受的并发用户数、运行时间、数据量等&…...
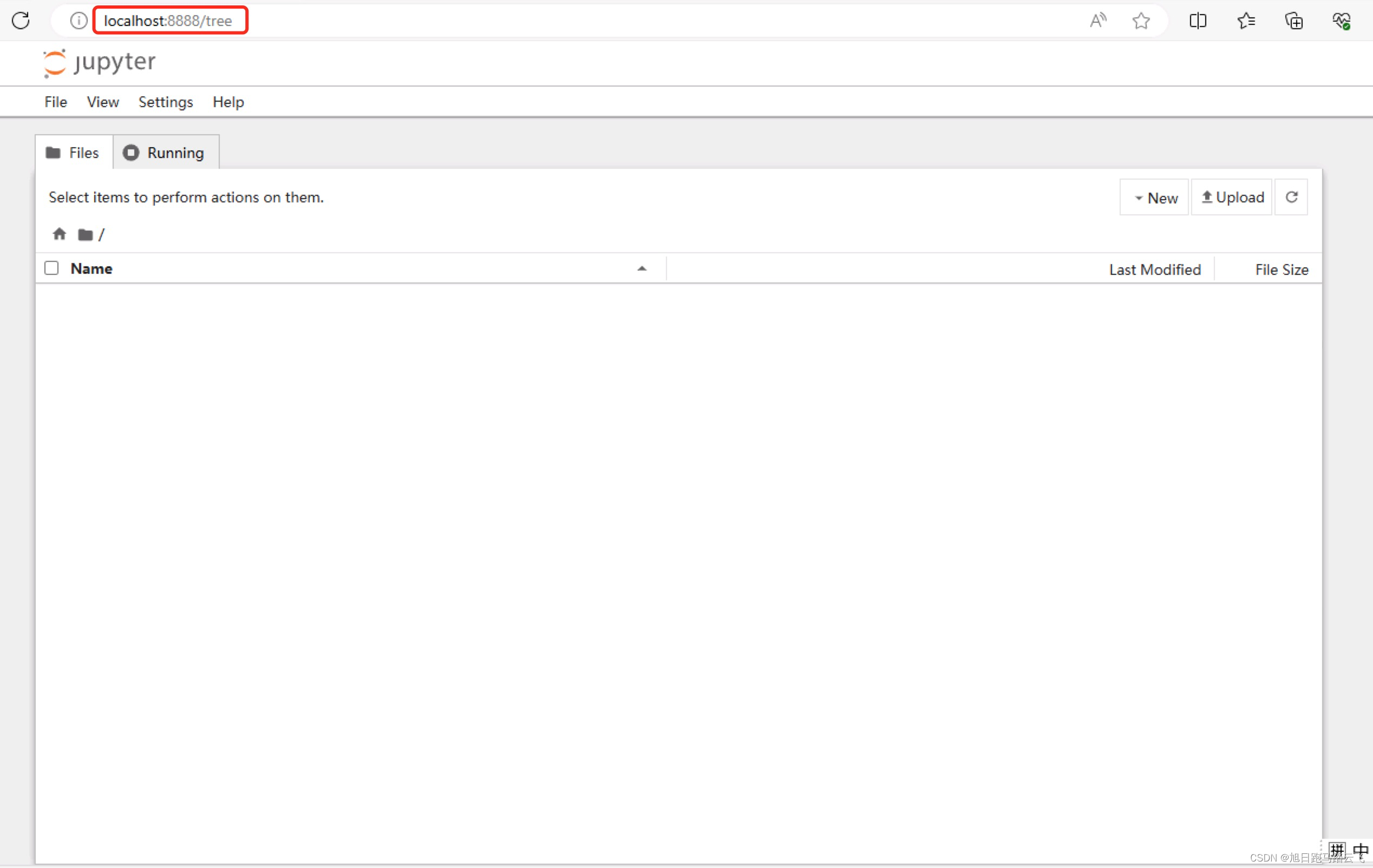
【AI工具】jupyter notebook和jupyterlab对比和安装
简单说,jupyterlab是jupyter notebook的下一代。 选择安装一个即可。 一、这里是AI对比介绍 Jupyter Notebook和JupyterLab都是基于Jupyter内核的交互式计算环境,但它们在设计和功能上有一些关键的区别: 用户界面: Jupyter Not…...

Linux 基本指令3
date指令 date[选项][格式] %Y--年 %m--月 %d--日 %H--小时 %M--分 %S--秒 中间可用其他符号分割,不能使用空格。 -s 设置时间,会返回设置时间的信息并不是改变当前时间 设置全部时间年可用-或者:分割日期和时间用空格分隔ÿ…...

在Linux系统中,可以使用OpenSSL来生成CSR(Certificate Signing Request)、PEM格式的公钥和PEM格式的私钥。
在Linux系统中,可以使用OpenSSL来生成CSR(Certificate Signing Request)、PEM格式的公钥和PEM格式的私钥。以下是生成这些文件的命令: 首先,生成私钥(通常是以.key结尾,但可以转换成PEM格式&am…...
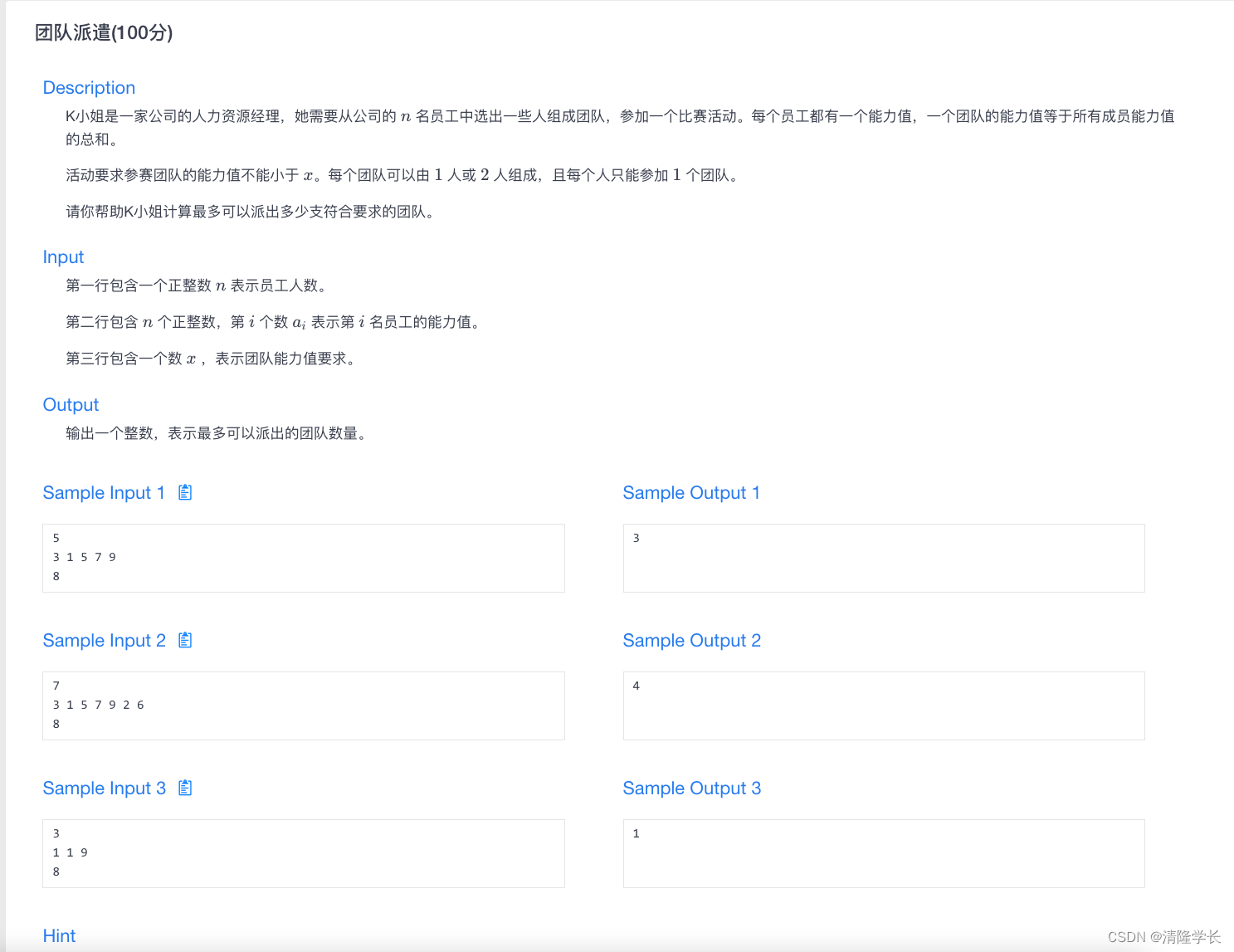
【2024最新华为OD-C/D卷试题汇总】[支持在线评测] 团队派遣(100分) - 三语言AC题解(Python/Java/Cpp)
🍭 大家好这里是清隆学长 ,一枚热爱算法的程序员 ✨ 本系列打算持续跟新华为OD-C/D卷的三语言AC题解 💻 ACM银牌🥈| 多次AK大厂笔试 | 编程一对一辅导 👏 感谢大家的订阅➕ 和 喜欢💗 🍓OJ题目截图 📎在线评测链接 团队派遣(100分) 🌍 评测功能需要订阅专栏…...
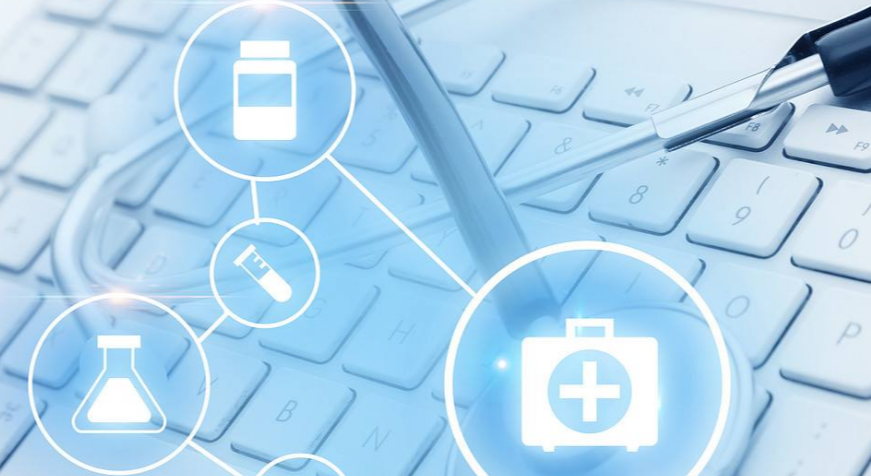
Python数据分析与机器学习在医疗诊断中的应用
文章目录 📑引言一、数据收集与预处理1.1 数据收集1.2 数据预处理 二、特征选择与构建2.1 特征选择2.2 特征构建 三、模型选择与训练3.1 逻辑回归3.2 随机森林3.3 深度学习 四、模型评估与调优4.1 交叉验证4.2 超参数调优 五、模型部署与应用5.1 模型保存与加载5.2 …...
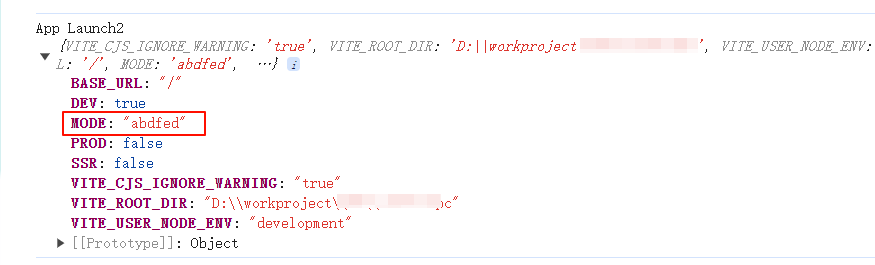
vite.config.js如何使用env的环境变量
了解下环境变量在vite中 官方文档走起 https://cn.vitejs.dev/guide/env-and-mode.html#env-variables-and-modes 你见到的.env,.env.production等就是放置环境变量的 官方文档说到.env.[mode] # 只在指定模式下加载,比如.env.development只在开发环境加载 至于为什么是deve…...

MySql几十万条数据,同时新增或者修改
项目场景: 十万条甚至更多的数据新增或者修改 问题描述 现在有十万条数据甚至更多数据,在这些数据中,有部分数据存在数据库中,有部分数据确是新数据,存在的数据需要更新,不存在的数据需要新增 原因分析&a…...

如何提高MySQL DELETE 速度
提高MySQL中DELETE操作的速度通常涉及多个方面,包括优化查询、索引、表结构、硬件和配置等。以下是一些建议,以及一些示例代码,用于帮助我们提高DELETE操作的速度。 1.提高MySQL DELETE 速度的方法 1.1 优化查询 只删除必要的行:…...
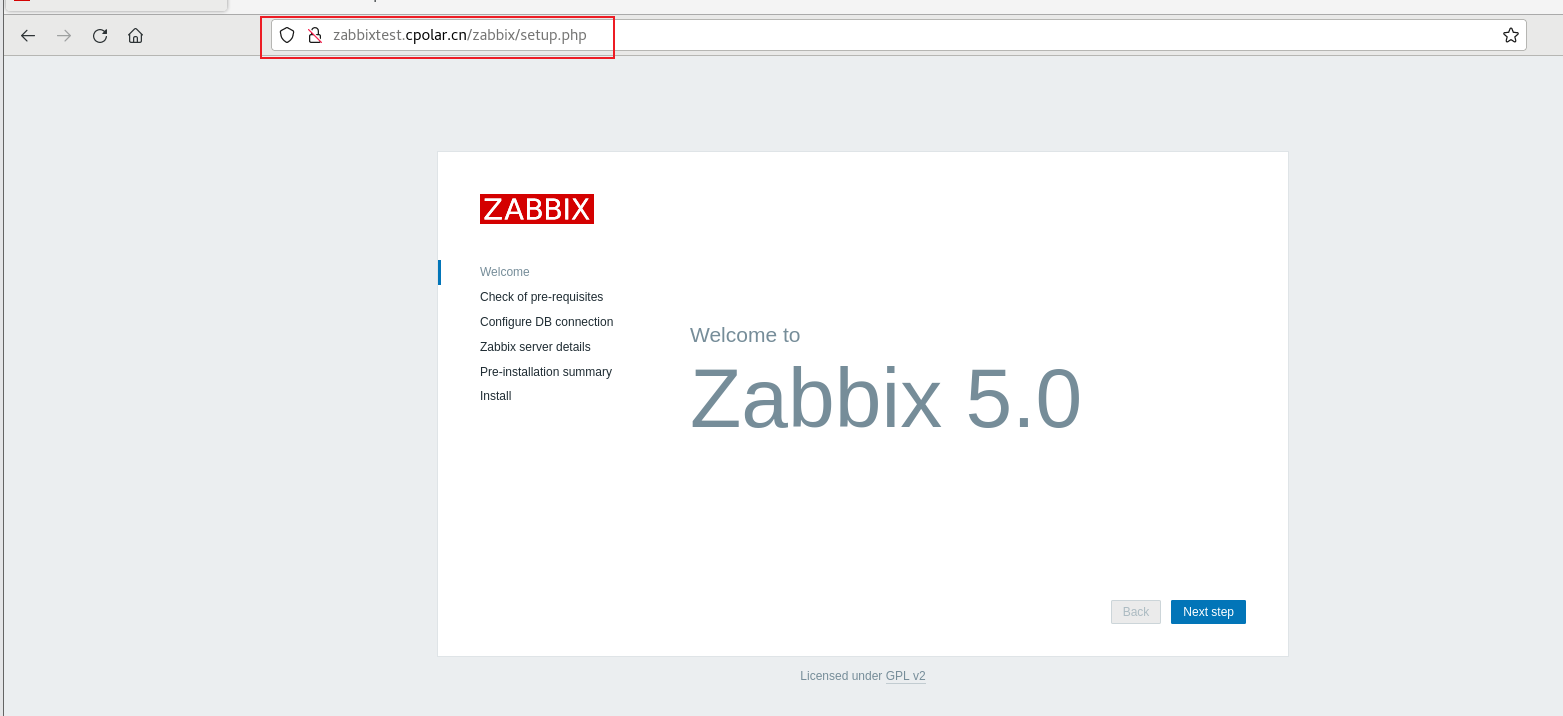
本地Zabbix开源监控系统安装内网穿透实现远程访问详细教程
文章目录 前言1. Linux 局域网访问Zabbix2. Linux 安装cpolar3. 配置Zabbix公网访问地址4. 公网远程访问Zabbix5. 固定Zabbix公网地址 💡推荐 前些天发现了一个巨牛的人工智能学习网站,通俗易懂,风趣幽默,忍不住分享一下给大家。【…...

从Android刷机包提取System和Framework
因为VIVO的手机很难解锁BL和Root,故直接从ADB中获取完整的Framework代码是比较困难的。我就考虑直接从VIVO提供的刷机包文件中获取相关的代码 由于vivo把system.new.dat分割了,所以下一步,我们使用cat命令,合并这些文件࿰…...
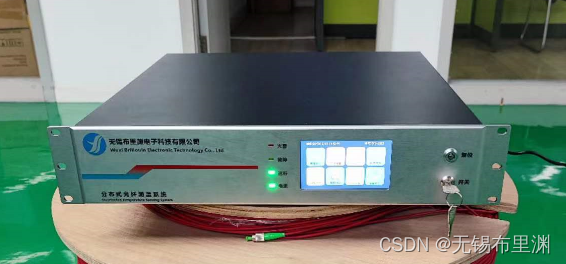
分布式光纤测温DTS与红外热成像系统的主要区别是什么?
分布式光纤测温DTS和红外热成像系统在应用领域和工作原理上存在显著的区别,两者具有明显的差异性。红外热成像系统适用于表现扩散式发热、面式场景以及环境条件较好的情况下。它主要用于检测物体表面的温度,并且受到镜头遮挡或灰尘等因素的影响会导致失效…...