基于xiaothink对Wanyv-50M模型进行c-eval评估
使用pypi安装xiaothink:
pip install xiaothink==1.0.2
下载模型:
万语-50M
开始评估(修改模型路径后即可直接开始运行,结果保存在output文件夹里):
import os
import json
import pandas as pd
import re
from tqdm import tqdm
import random
import time
import requests
from xiaothink.llm.inference.test_formal import *
model=QianyanModel(MT=40.231,ckpt_dir=r'path\to\wanyv\model\ckpt_test_40_2_3_1_formal_open')def chat_x(inp,temp=0.3):return model.chat_SingleTurn(inp,temp=temp,loop=True,stop='。')#from collections import Counterdef pre(question: str, options_str: str) -> str:question = question.replace('答案:', '')options_str = options_str.replace('答案:', '')if not 'A' in question:#你只需要直接-让我们首先一步步思考,最后在回答末尾prompt_template = '''题目:{question}\n{options_str}\n让我们首先一步步思考,最后在回答末尾给出一个字母作为你的答案(A或B或C或D)'''prompt_template2 = '''题目:{question}\n选项:{options_str}\n给出答案'''prompt_template3 = '''{question}\n{options_str}\n'''prompt_template4 = '''{question}\n{options_str}\n给出你的选择'''prompt_template5 = '''题目:{question}\n{options_str}\n答案:'''else:prompt_template = '''题目:{question}\n让我们首先一步步思考,最后在回答末尾给出一个字母作为你的答案(A或B或C或D)'''prompt_template2 = '''题目:{question}\n给出答案'''prompt_template3 = '''{question}\n'''prompt_template4 = '''{question}\n给出你的选择'''prompt_template5 = '''题目:{question}\n答案:'''ansd={}# Run the chat_core function 5 times and collect answersanswers = []for _ in range(1):response = chat_x(prompt_template.format(question=question, options_str=options_str))#print(response)# Extract answer from responsefor option in 'ABCD':if option in response:answers.append(option)ansd[option]=responsebreakelse:print('AI选项检查:', repr(response))answers.append('A') # Default to 'A' if no option foundansd['A']=''# Count occurrences of each answeranswer_counts = Counter(answers)# Find the most common answer(s)most_common_answers = answer_counts.most_common()highest_frequency = most_common_answers[0][1]most_frequent_answers = [answer for answer, count in most_common_answers if count == highest_frequency]# Choose one of the most frequent answers (if there's a tie, choose the first alphabetically)final_answer = min(most_frequent_answers)with open('ceval_text_sklm.txt','a',encoding='utf-8') as f:f.write(
'{"instruction": "{prompt_template}", "input": "", "output": "{final_answer}"}\n'.replace('{prompt_template}',prompt_template.format(question=question, options_str=options_str).replace('\n','\\n')).replace('{final_answer}',ansd[final_answer]),)with open('ceval_text_sklm.txt','a',encoding='utf-8') as f:f.write(
'{"instruction": "{prompt_template}", "input": "", "output": "{final_answer}"}\n'.replace('{prompt_template}',prompt_template2.format(question=question, options_str=options_str).replace('\n','\\n')).replace('{final_answer}',ansd[final_answer]),)with open('ceval_text_sklm.txt','a',encoding='utf-8') as f:f.write(
'{"instruction": "{prompt_template}", "input": "", "output": "{final_answer}"}\n'.replace('{prompt_template}',prompt_template3.format(question=question, options_str=options_str).replace('\n','\\n')).replace('{final_answer}',ansd[final_answer]),)with open('ceval_text_sklm.txt','a',encoding='utf-8') as f:f.write(
'{"instruction": "{prompt_template}", "input": "", "output": "{final_answer}"}\n'.replace('{prompt_template}',prompt_template4.format(question=question, options_str=options_str).replace('\n','\\n')).replace('{final_answer}',ansd[final_answer]),)with open('ceval_text_sklm.txt','a',encoding='utf-8') as f:f.write(
'{"instruction": "{prompt_template}", "input": "", "output": "{final_answer}"}\n'.replace('{prompt_template}',prompt_template5.format(question=question, options_str=options_str).replace('\n','\\n')).replace('{final_answer}',ansd[final_answer]),)return final_answerclass Llama_Evaluator:def __init__(self, choices, k):self.choices = choicesself.k = kdef eval_subject(self, subject_name,test_df,dev_df=None,few_shot=False,cot=False,save_result_dir=None,with_prompt=False,constrained_decoding=False,do_test=False):all_answers = {}correct_num = 0if save_result_dir:result = []score = []if few_shot:history = self.generate_few_shot_prompt(subject_name, dev_df, cot=cot)else:history = ''answers = ['NA'] * len(test_df) if do_test is True else list(test_df['answer'])for row_index, row in tqdm(test_df.iterrows(), total=len(test_df)):question = self.format_example(row, include_answer=False, cot=cot, with_prompt=with_prompt)options_str = self.format_options(row)instruction = history + question + "\n选项:" + options_strans = pre(instruction, options_str)if ans == answers[row_index]:correct_num += 1correct = 1else:correct = 0print(f"\n=======begin {str(row_index)}=======")print("question: ", question)print("options: ", options_str)print("ans: ", ans)print("ground truth: ", answers[row_index], "\n")if save_result_dir:result.append(ans)score.append(correct)print(f"=======end {str(row_index)}=======")all_answers[str(row_index)] = anscorrect_ratio = 100 * correct_num / len(answers)if save_result_dir:test_df['model_output'] = resulttest_df['correctness'] = scoretest_df.to_csv(os.path.join(save_result_dir, f'{subject_name}_test.csv'))return correct_ratio, all_answersdef format_example(self, line, include_answer=True, cot=False, with_prompt=False):example = line['question']for choice in self.choices:example += f'\n{choice}. {line[f"{choice}"]}'if include_answer:if cot:example += "\n答案:让我们一步一步思考,\n" + \line["explanation"] + f"\n所以答案是{line['answer']}。\n\n"else:example += '\n答案:' + line["answer"] + '\n\n'else:if with_prompt is False:if cot:example += "\n答案:让我们一步一步思考,\n1."else:example += '\n答案:'else:if cot:example += "\n答案是什么?让我们一步一步思考,\n1."else:example += '\n答案是什么? 'return exampledef generate_few_shot_prompt(self, subject, dev_df, cot=False):prompt = f"以下是中国关于{subject}考试的单项选择题,请选出其中的正确答案。\n\n"k = self.kif self.k == -1:k = dev_df.shape[0]for i in range(k):prompt += self.format_example(dev_df.iloc[i, :],include_answer=True,cot=cot)return promptdef format_options(self, line):options_str = ""for choice in self.choices:options_str += f"{choice}: {line[f'{choice}']} "return options_strdef main(model_path, output_dir, take, few_shot=False, cot=False, with_prompt=False, constrained_decoding=False, do_test=False, n_times=1, do_save_csv=False):assert os.path.exists("subject_mapping.json"), "subject_mapping.json not found!"with open("subject_mapping.json") as f:subject_mapping = json.load(f)filenames = os.listdir("data/val")subject_list = [val_file.replace("_val.csv", "") for val_file in filenames]accuracy, summary = {}, {}run_date = time.strftime('%Y-%m-%d_%H-%M-%S', time.localtime(time.time()))save_result_dir = os.path.join(output_dir, f"take{take}")if not os.path.exists(save_result_dir):os.makedirs(save_result_dir, exist_ok=True)evaluator = Llama_Evaluator(choices=choices, k=n_times)all_answers = {}for index, subject_name in tqdm(list(enumerate(subject_list)),desc='主进度'):print(f"{index / len(subject_list)} Inference starts at {run_date} on {model_path} with subject of {subject_name}!")val_file_path = os.path.join('data/val', f'{subject_name}_val.csv')dev_file_path = os.path.join('data/dev', f'{subject_name}_dev.csv')test_file_path = os.path.join('data/test', f'{subject_name}_test.csv')val_df = pd.read_csv(val_file_path) if not do_test else pd.read_csv(test_file_path)dev_df = pd.read_csv(dev_file_path) if few_shot else Nonecorrect_ratio, answers = evaluator.eval_subject(subject_name, val_df, dev_df,save_result_dir=save_result_dir if do_save_csv else None,few_shot=few_shot,cot=cot,with_prompt=with_prompt,constrained_decoding=constrained_decoding,do_test=do_test)print(f"Subject: {subject_name}")print(f"Acc: {correct_ratio}")accuracy[subject_name] = correct_ratiosummary[subject_name] = {"score": correct_ratio,"num": len(val_df),"correct": correct_ratio * len(val_df) / 100}all_answers[subject_name] = answersjson.dump(all_answers, open(save_result_dir + '/submission.json', 'w'), ensure_ascii=False, indent=4)print("Accuracy:")for k, v in accuracy.items():print(k, ": ", v)total_num = 0total_correct = 0summary['grouped'] = {"STEM": {"correct": 0.0, "num": 0},"Social Science": {"correct": 0.0, "num": 0},"Humanities": {"correct": 0.0, "num": 0},"Other": {"correct": 0.0, "num": 0}}for subj, info in subject_mapping.items():group = info[2]summary['grouped'][group]["num"] += summary[subj]['num']summary['grouped'][group]["correct"] += summary[subj]['correct']for group, info in summary['grouped'].items():info['score'] = info["correct"] / info["num"]total_num += info["num"]total_correct += info["correct"]summary['All'] = {"score": total_correct / total_num, "num": total_num, "correct": total_correct}json.dump(summary, open(save_result_dir + '/summary.json', 'w'), ensure_ascii=False, indent=2)# Example usage
if __name__ == "__main__":model_path = "path/to/model"output_dir = "output"take = 0few_shot = Falsecot = Falsewith_prompt = Falseconstrained_decoding = Falsedo_test = True#Falsen_times = 1do_save_csv = Falsemain(model_path, output_dir, take, few_shot, cot, with_prompt, constrained_decoding, do_test, n_times, do_save_csv)
相关文章:
基于xiaothink对Wanyv-50M模型进行c-eval评估
使用pypi安装xiaothink: pip install xiaothink1.0.2下载模型: 万语-50M 开始评估(修改模型路径后即可直接开始运行,结果保存在output文件夹里): import os import json import pandas as pd import re from tqdm import tqdm i…...
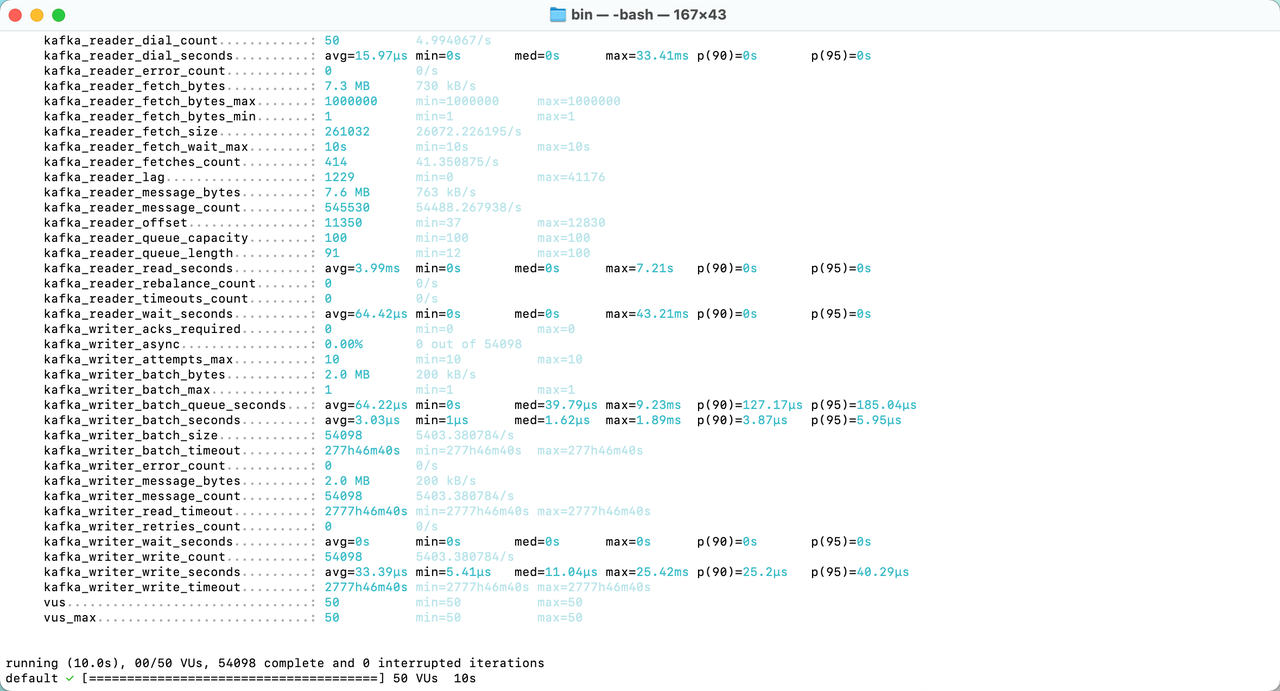
使用k6进行kafka负载测试
1.安装环境 kafka环境 参考Docker搭建kafka环境-CSDN博客 xk6-kafka环境 ./xk6 build --with github.com/mostafa/xk6-kafkalatest 查看安装情况 2.编写脚本 test_kafka.js // Either import the module object import * as kafka from "k6/x/kafka";// Or in…...
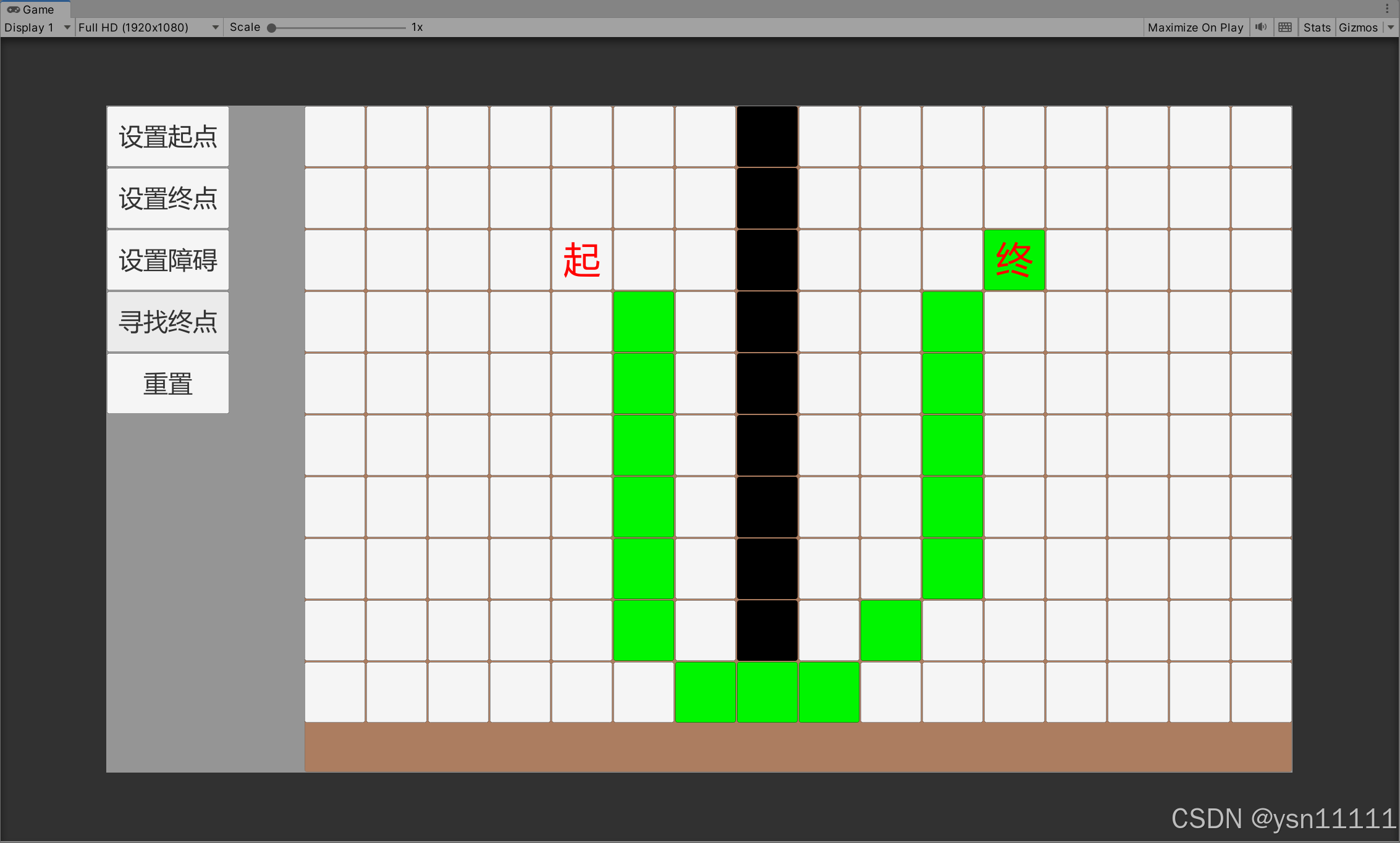
Unity A*算法实现+演示
注意: 本文是对基于下方文章链接的理论,并最终代码实现,感谢作者大大的描述,非常详细,流程稍微做了些改动,文末有工程网盘链接,感兴趣的可以下载。 A*算法详解(个人认为最详细,最通俗易懂的一…...
浏览器要求用户确认 Cookies Privacy(隐私相关内容)是基于隐私法规的要求,VUE 实现,html 代码
Cookie Notices and Cookie Consent | Cookiepedia 1. 法律法规要求 许多国家和地区的隐私法律要求网站在存储或处理用户数据(包括 Cookies)之前必须获得用户的明确同意: GDPR(欧盟通用数据保护条例) 要求ÿ…...
如何设计高效的商品系统并提升扩展性:从架构到实践的全方位探索
在现代电商、零售及企业资源管理系统中,商品管理无疑是核心模块之一。随着市场的变化与企业规模的扩展,商品系统需要具备强大的功能支持以及高效的扩展能力,以应对日益复杂的业务需求。一个设计良好的商品系统不仅仅是一个商品信息的容器&…...
使用计算机创建一个虚拟世界
创建一个虚拟世界是一项复杂而多方面的工作,它涉及多个领域的知识,包括计算机图形学、编程、物理模拟、声音设计、艺术设计等。以下是创建虚拟世界的基本步骤和工具建议: 1. 确定虚拟世界的目标和范围 目标:明确这个虚拟世界的用…...
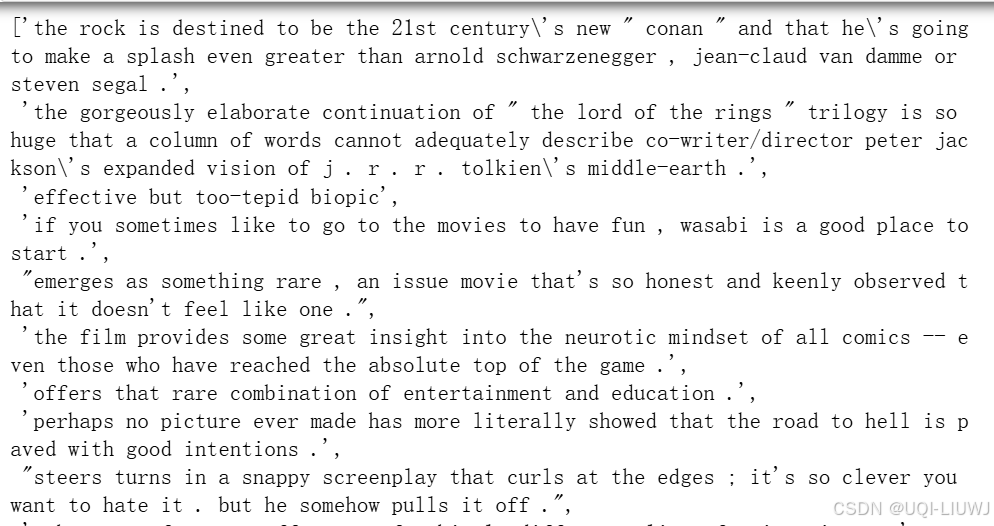
datasets笔记:两种数据集对象
Datasets 提供两种数据集对象:Dataset 和 ✨ IterableDataset ✨。 Dataset 提供快速随机访问数据集中的行,并支持内存映射,因此即使加载大型数据集也只需较少的内存。IterableDataset 适用于超大数据集,甚至无法完全下载到磁盘或…...
【ETCD】【Linearizable Read OR Serializable Read】ETCD 数据读取:强一致性 vs 高性能,选择最适合的读取模式
ETCD 提供了两种不同类型的读取操作方式,分别是 Linearizable Read(线性化读取)和 Serializable Read(可串行化读取)。这两种方式主要区分在读取数据时对一致性的要求不同。 目录 1. Linearizable Read(线…...
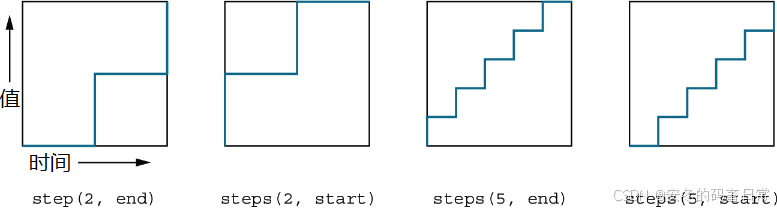
【CSS in Depth 2 精译_089】15.2:CSS 过渡特效中的定时函数
当前内容所在位置(可进入专栏查看其他译好的章节内容) 第五部分 添加动效 ✔️【第 15 章 过渡】 ✔️ 15.1 状态间的由此及彼15.2 定时函数 ✔️ 15.2.1 定制贝塞尔曲线 ✔️15.2.2 阶跃 ✔️ 15.3 非动画属性 文章目录 15.2 定时函数 Timing function…...
不常用命令指南
常用命令网上资料很多,讲的也不错。这里记录下日常工作中用到的,但对于新手又不常用的命令 文章目录 信息相关进程相关htoppgrep(根据指定的条件获取进程id)lsof 网络相关ssnc(netcat) 信息相关 进程相关 …...
spring mvc | servlet :serviceImpl无法自动装配 UserMapper
纯注解SSM整合 解决办法: 在MybatisConfig添加 Configuration MapperScan("mapper")...
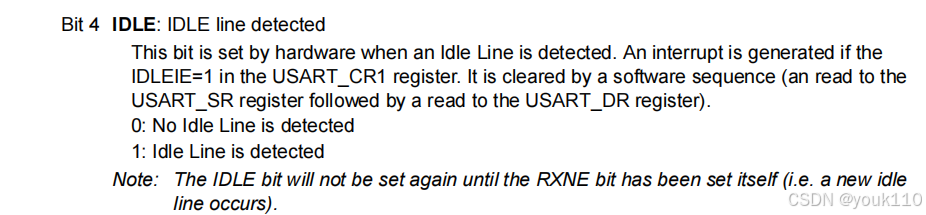
STM32 HAL库之串口接收不定长字符
背景 在项目开发过程中,经常会使用MCU的串口与外界进行通信,例如两个单片机之间TTL电平型串口通信,单片机与成熟电路模块之间的串口通信等等.... 如何高效的使用串口是开发人员必须关注的问题。 STM32的HAL库为我们提供了三种串口通信机制&am…...
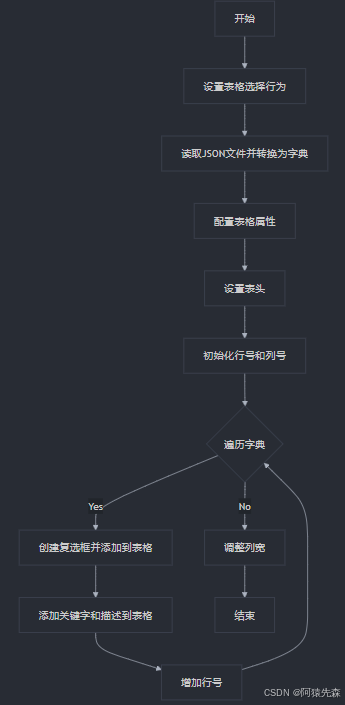
Pyqt6的tableWidget填充数据
代码 from PySide6.QtWidgets import QTableWidget QTableWidgetItemdef tableInit(self):self.tableWidgetself.tableWidget.setSelectionBehavior(QAbstractItemView.SelectRows)module_keyWord readJsonToDict(keyWordFileDir module_name) #读取模块关键字json字典数据s…...
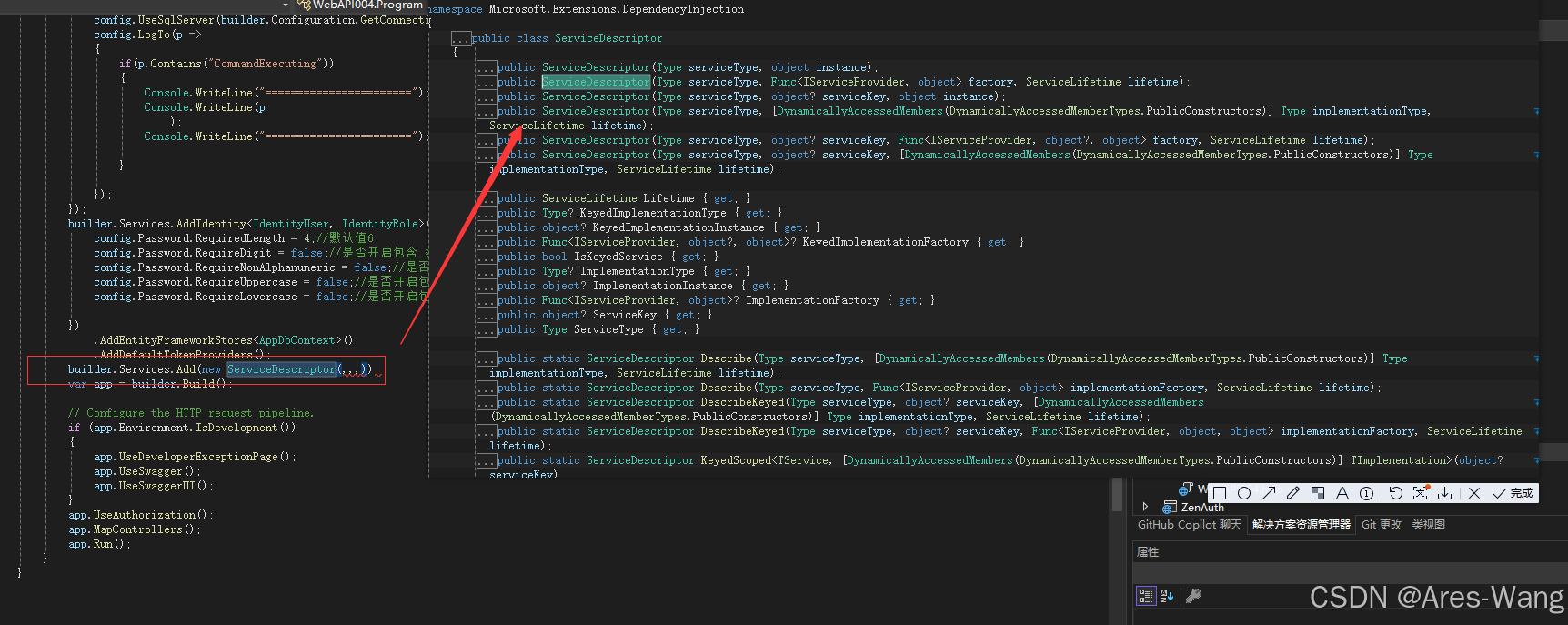
ASP.NET Core - 依赖注入 自动批量注入
依赖注入配置变形 随着业务的增长,我们项目工作中的类型、服务越来越多,而每一个服务的依赖注入关系都需要在入口文件通过Service.Add{}方法去进行注册,这将是非常麻烦的,入口文件需要频繁改动,而且代码组织管理也会变…...
UVM 验证方法学之interface学习系列文章(十一)virtual interface 再续篇
一 前言 并非总是可以将被测单元(DUT)视为一个黑盒,即仅监控和驱动DUT的顶层端口信号。这一点在从模块级测试转向更大规模的系统级测试时尤为明显。有时,我们需要了解实现细节以便访问DUT内部的信号。这被称为白盒验证。 Verilog一直提供从其他作用域访问几乎任何层次结构…...
面试题整理5----进程、线程、协程区别及僵尸进程处理
面试题整理5----进程、线程、协程区别及僵尸进程处理 1. 进程、线程与协程的区别1.1 进程(Process)1.2 线程(Thread)1.3 协程(Coroutine)2. 总结对比 3. 僵尸进程3.1 什么是僵尸进程?3.2 僵尸进…...
OpenTK 中帧缓存的深度解析与应用实践
摘要: 本文深入探讨了 OpenTK 中帧缓存的使用。首先介绍了帧缓存的基本概念与在图形渲染管线中的关键地位,包括其与颜色缓存、深度缓存、模板缓存等各类缓存的关联。接着详细阐述了帧缓存对象(FBO)的创建、绑定与解绑等操作,深入分析了纹理附件、渲染缓冲区附件在 FBO 中的…...
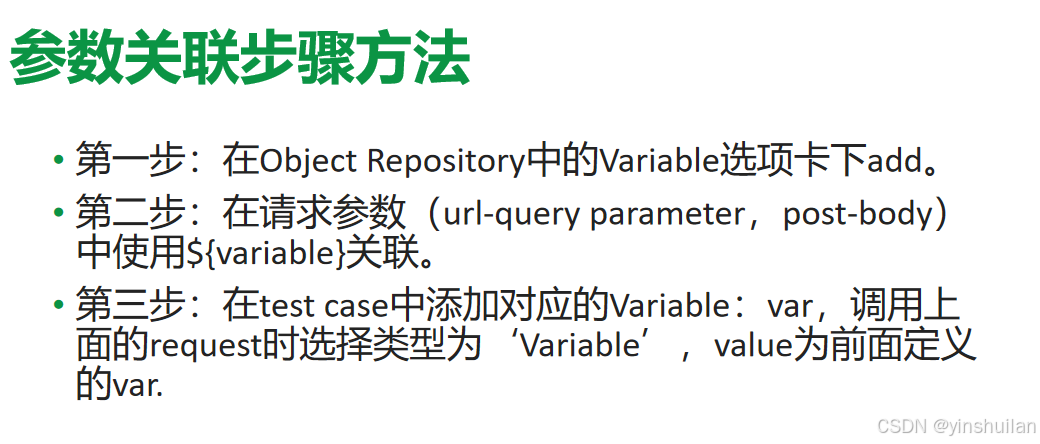
第2节-Test Case如何调用Object Repository中的请求并关联参数
前提: 已经创建好了project(File -> New -> Project,Type:API/WebService),object repository中已经创建了RESTful endpoint(Object Repository -> New -> Web Service Request&am…...
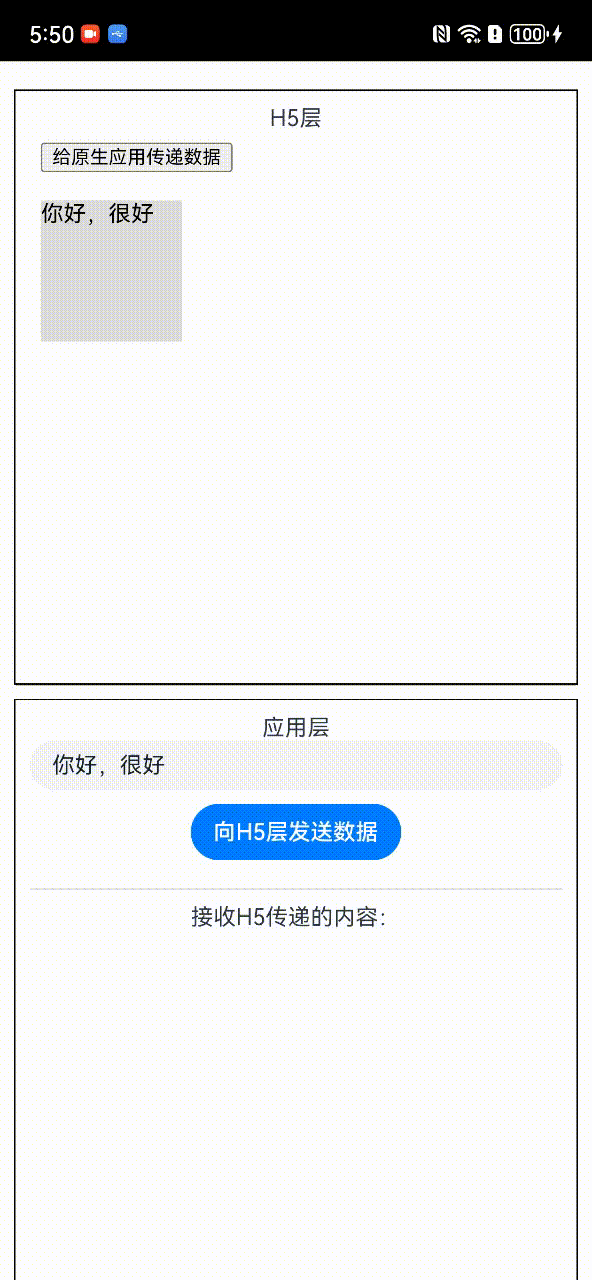
【HarmonyOS NEXT】Web 组件的基础用法以及 H5 侧与原生侧的双向数据通讯
关键词:鸿蒙、ArkTs、Web组件、通讯、数据 官方文档Web组件用法介绍:文档中心 Web 组件加载沙箱中页面可参考我的另一篇文章:【HarmonyOS NEXT】 如何将rawfile中文件复制到沙箱中_鸿蒙rawfile 复制到沙箱-CSDN博客 目录 如何在鸿蒙应用中加…...
Android学习(六)-Kotlin编程语言-数据类与单例类
假设我们要创建一个表示书籍的数据类 Book,包含书名和作者两个字段。在 Java 中,代码如下: public class Book { String title; String author; public Book(String title, String author) { this.title title; this.author author; } Ove…...
在软件开发中正确使用MySQL日期时间类型的深度解析
在日常软件开发场景中,时间信息的存储是底层且核心的需求。从金融交易的精确记账时间、用户操作的行为日志,到供应链系统的物流节点时间戳,时间数据的准确性直接决定业务逻辑的可靠性。MySQL作为主流关系型数据库,其日期时间类型的…...
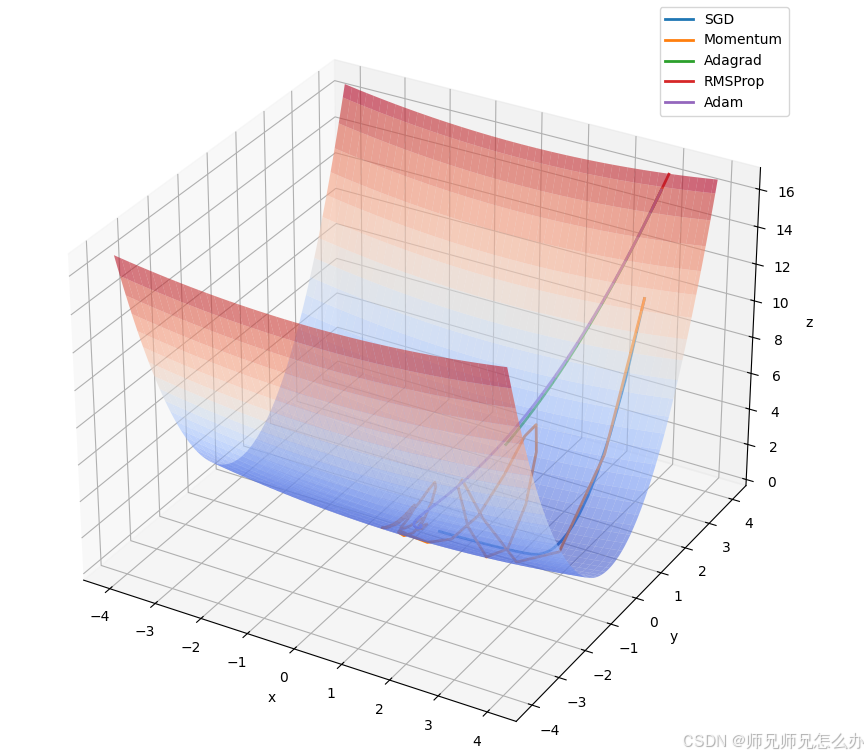
【人工智能】神经网络的优化器optimizer(二):Adagrad自适应学习率优化器
一.自适应梯度算法Adagrad概述 Adagrad(Adaptive Gradient Algorithm)是一种自适应学习率的优化算法,由Duchi等人在2011年提出。其核心思想是针对不同参数自动调整学习率,适合处理稀疏数据和不同参数梯度差异较大的场景。Adagrad通…...
将对透视变换后的图像使用Otsu进行阈值化,来分离黑色和白色像素。这句话中的Otsu是什么意思?
Otsu 是一种自动阈值化方法,用于将图像分割为前景和背景。它通过最小化图像的类内方差或等价地最大化类间方差来选择最佳阈值。这种方法特别适用于图像的二值化处理,能够自动确定一个阈值,将图像中的像素分为黑色和白色两类。 Otsu 方法的原…...
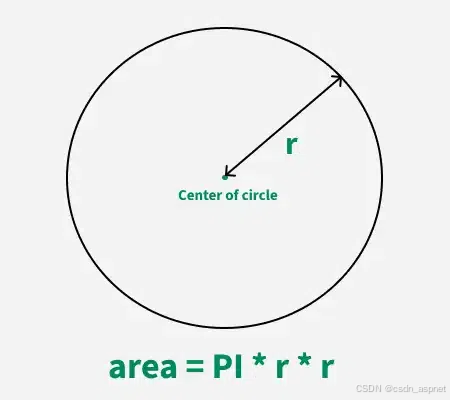
C++ 求圆面积的程序(Program to find area of a circle)
给定半径r,求圆的面积。圆的面积应精确到小数点后5位。 例子: 输入:r 5 输出:78.53982 解释:由于面积 PI * r * r 3.14159265358979323846 * 5 * 5 78.53982,因为我们只保留小数点后 5 位数字。 输…...
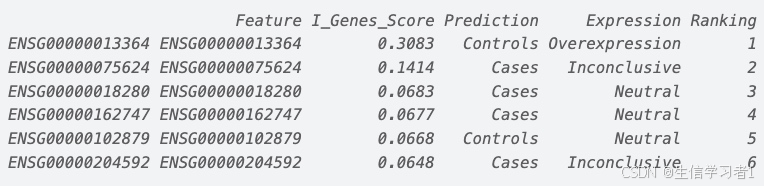
【数据分析】R版IntelliGenes用于生物标志物发现的可解释机器学习
禁止商业或二改转载,仅供自学使用,侵权必究,如需截取部分内容请后台联系作者! 文章目录 介绍流程步骤1. 输入数据2. 特征选择3. 模型训练4. I-Genes 评分计算5. 输出结果 IntelliGenesR 安装包1. 特征选择2. 模型训练和评估3. I-Genes 评分计…...
CSS设置元素的宽度根据其内容自动调整
width: fit-content 是 CSS 中的一个属性值,用于设置元素的宽度根据其内容自动调整,确保宽度刚好容纳内容而不会超出。 效果对比 默认情况(width: auto): 块级元素(如 <div>)会占满父容器…...
蓝桥杯 冶炼金属
原题目链接 🔧 冶炼金属转换率推测题解 📜 原题描述 小蓝有一个神奇的炉子用于将普通金属 O O O 冶炼成为一种特殊金属 X X X。这个炉子有一个属性叫转换率 V V V,是一个正整数,表示每 V V V 个普通金属 O O O 可以冶炼出 …...
在QWebEngineView上实现鼠标、触摸等事件捕获的解决方案
这个问题我看其他博主也写了,要么要会员、要么写的乱七八糟。这里我整理一下,把问题说清楚并且给出代码,拿去用就行,照着葫芦画瓢。 问题 在继承QWebEngineView后,重写mousePressEvent或event函数无法捕获鼠标按下事…...
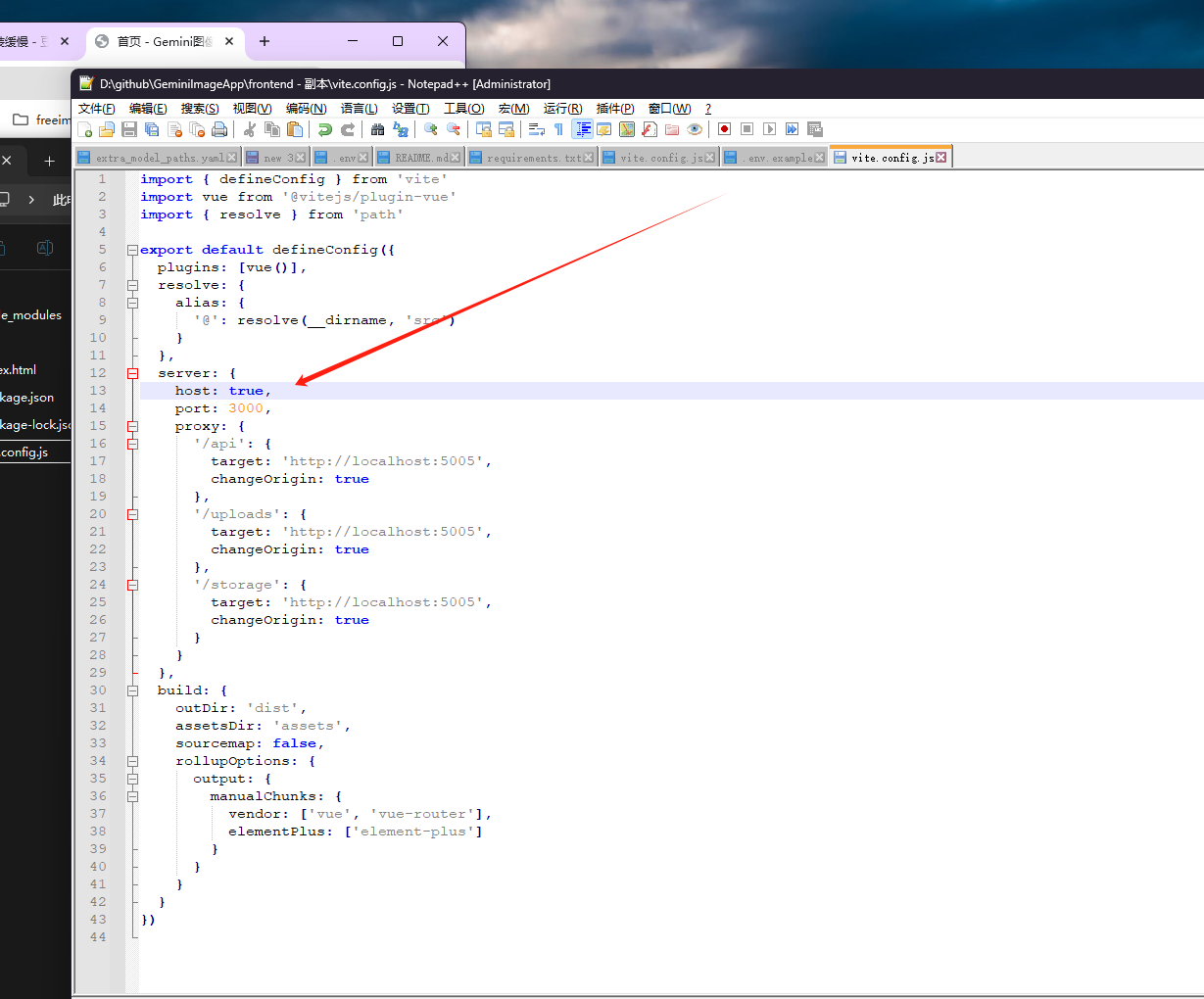
推荐 github 项目:GeminiImageApp(图片生成方向,可以做一定的素材)
推荐 github 项目:GeminiImageApp(图片生成方向,可以做一定的素材) 这个项目能干嘛? 使用 gemini 2.0 的 api 和 google 其他的 api 来做衍生处理 简化和优化了文生图和图生图的行为(我的最主要) 并且有一些目标检测和切割(我用不到) 视频和 imagefx 因为没 a…...
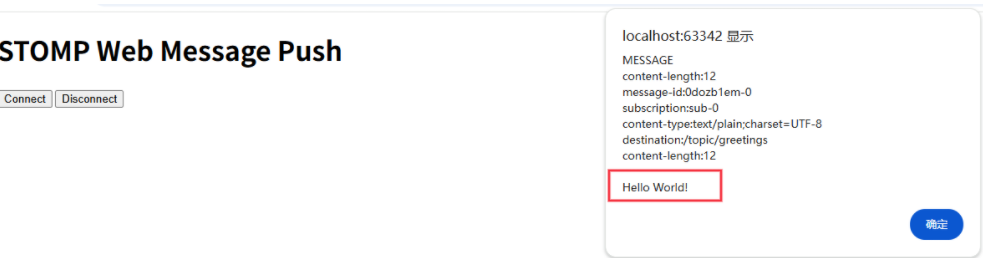
RabbitMQ入门4.1.0版本(基于java、SpringBoot操作)
RabbitMQ 一、RabbitMQ概述 RabbitMQ RabbitMQ最初由LShift和CohesiveFT于2007年开发,后来由Pivotal Software Inc.(现为VMware子公司)接管。RabbitMQ 是一个开源的消息代理和队列服务器,用 Erlang 语言编写。广泛应用于各种分布…...