基于C++和Python的进程线程CPU使用率监控工具
文章目录
- 0. 概述
- 1. 数据可视化示例
- 2. 设计思路
- 2.1 系统架构
- 2.2 设计优势
- 3. 流程图
- 3.1 C++录制程序
- 3.2 Python解析脚本
- 4. 数据结构说明
- 4.1 `CpuUsageData` 结构体
- 5. C++录制代码解析
- 5.1 主要模块
- 5.2 关键函数
- 5.2.1 `CpuUsageMonitor::Run()`
- 5.2.2 `CpuUsageMonitor::ComputeCpuUsage()`
- 5.2.3 `CpuUsageMonitor::PrintProcessInfo()`
- 5.3 其他重要功能
- 5.3.1 `CpuUsageMonitor::InitializeThreads()`
- 5.3.2 `CpuUsageMonitor::ReadAndStoreProcessName()`
- 6. Python解析代码解析
- 6.1 主要模块
- 6.2 关键函数
- 6.2.1 `parse_cpu_usage_data(record_bytes)`
- 6.2.2 `read_file_header(f)`
- 6.2.3 `read_cpu_usage_bin(filename)`
- 6.2.4 `parse_records_to_dataframe(records, thread_name_map)`
- 6.2.5 `plot_cpu_usage(...)`
0. 概述
本文将介绍一个基于C++和Python实现的进程线程CPU使用率监控工具,用于监控指定进程及其所有线程的CPU使用情况。
编写这个工具的初衷是源于部分嵌入式ARM系统的top工具不支持显示线程级别的CPU使用情况。
该工具分为两部分:
-
C++录制程序:负责实时采集指定进程及其线程的CPU使用数据,并将数据以二进制格式存储到文件中。
-
Python解析脚本:读取二进制数据文件,解析CPU使用数据,并生成可视化图表,帮助用户直观了解CPU使用情况。
本文完整代码可从gitee的thread-monitor获取到,编译和使用方法见README.md
另一种获取CPU使用率的方式:使用 Shell 和 Python 解析 top 输出并记录进程及线程的 CPU 使用率
1. 数据可视化示例
以下是生成的CPU使用率图表示例:
图中,黑色实线表示整个进程的CPU总使用率,蓝色实线和虚线分别表示特定线程的用户态和内核态CPU使用率。
本文测试的dummp_worker实现代码见:使用C++多线程和POSIX库模拟CPU密集型工作
-
C++录制程序终端显示
$ ./thread_cpu_bin -n0.5 dummp_worker -o dump.bin Process Name: dummp_worker Process ID: 19515 Process Priority: 0 Threads (5):Thread Name: dummp_worker, Thread ID: 19515, Priority: 20Thread Name: worker_0, Thread ID: 19516, Priority: -2Thread Name: worker_1, Thread ID: 19517, Priority: -3Thread Name: worker_2, Thread ID: 19518, Priority: -4Thread Name: worker_3, Thread ID: 19519, Priority: -5
-
Python解析脚本终端显示
$ python3 thread_cpu_bin.py dump.bin Process Name: dummp_worker worker_0: Max/Avg User=2%/1.01% | Max/Avg Kernel=3%/2.03% worker_1: Max/Avg User=2%/1.02% | Max/Avg Kernel=3%/2.03% worker_2: Max/Avg User=2%/1.02% | Max/Avg Kernel=3%/2.03% worker_3: Max/Avg User=2%/1.02% | Max/Avg Kernel=3%/2.03%
2. 设计思路
2.1 系统架构
整个系统由两个独立的组件组成:
-
数据采集组件(C++):
- 监控指定进程的CPU使用情况。
- 定期采集每个线程的用户态和内核态CPU时间。
- 将采集到的数据写入二进制文件,便于后续分析。
-
数据解析与可视化组件(Python):
- 读取C++采集的二进制数据文件。
- 解析并转换为结构化数据(如Pandas DataFrame)。
- 计算统计指标(最小值、最大值、平均值)。
- 生成CPU使用率随时间变化的可视化图表。
2.2 设计优势
- 高性能:使用C++进行数据采集,确保低延迟和高效性能。
- 灵活性:Python脚本提供了灵活的数据处理和可视化能力,用户可以根据需要定制分析和展示方式。
- 可扩展性:系统设计模块化,便于未来功能扩展,如添加更多监控指标或支持更多操作系统。
3. 流程图
3.1 C++录制程序
3.2 Python解析脚本
4. 数据结构说明
4.1 CpuUsageData
结构体
在C++录制程序中,定义了一个结构体 CpuUsageData
用于存储每个线程的CPU使用数据。该结构体采用1字节对齐(#pragma pack(push, 1)
),确保数据在二进制文件中的紧凑存储。
#pragma pack(push, 1)
struct CpuUsageData {uint8_t user_percent; // 用户态CPU使用百分比 (0-100)uint8_t kernel_percent; // 内核态CPU使用百分比 (0-100)uint16_t user_ticks; // 用户态CPU时间滴答数uint16_t kernel_ticks; // 内核态CPU时间滴答数uint32_t timestamp; // 时间戳(自纪元以来的秒数)uint32_t thread_id; // 线程ID(完整形式)uint8_t thread_status; // 线程状态(2位)uint8_t extra_flags; // 额外标志(3位)
};
#pragma pack(pop)
字段说明:
user_percent
和kernel_percent
:表示线程在用户态和内核态的CPU使用百分比。user_ticks
和kernel_ticks
:表示线程在用户态和内核态的CPU时间滴答数。timestamp
:记录数据采集的时间点。thread_id
:线程的唯一标识符。thread_status
和extra_flags
:存储线程的状态信息和额外标志位。
5. C++录制代码解析
C++录制程序负责实时监控指定进程及其线程的CPU使用情况,并将数据存储到二进制文件中。以下将详细解析其主要模块和关键函数。
5.1 主要模块
-
信号处理:
- 通过信号处理机制,程序能够优雅地响应用户中断(如
Ctrl+C
),确保资源的正确释放和数据的完整性。
- 通过信号处理机制,程序能够优雅地响应用户中断(如
-
文件和目录管理:
- 使用RAII(资源获取即初始化)类
FileCloser
和DirCloser
,确保文件和目录在使用后自动关闭,防止资源泄漏。
- 使用RAII(资源获取即初始化)类
-
线程管理:
- 动态初始化和更新被监控进程的所有线程信息,包括线程ID、名称和优先级。
-
数据采集与记录:
- 定期采集每个线程的CPU使用情况,并将数据封装到
CpuUsageData
结构体中。 - 将采集到的数据写入二进制文件中,便于后续分析。
- 定期采集每个线程的CPU使用情况,并将数据封装到
5.2 关键函数
5.2.1 CpuUsageMonitor::Run()
程序的主循环,负责定期采集数据并记录。
void Run() {GetThreadCpuTicks();current_total_cpu_time_ = GetTotalCpuTime();StoreCurrentTicksAsPrevious();while (keep_running) {std::this_thread::sleep_for(std::chrono::duration<double>(refresh_delay_));InitializeThreads();GetThreadCpuTicks();current_total_cpu_time_ = GetTotalCpuTime();delta_total_cpu_time_ =current_total_cpu_time_ - previous_total_cpu_time_;if (delta_total_cpu_time_ > 0) {ComputeCpuUsage();}StoreCurrentTicksAsPrevious();}fprintf(stdout, "Exiting gracefully...\n");
}
功能描述:
- 数据采集:调用
GetThreadCpuTicks()
和GetTotalCpuTime()
获取当前的CPU使用数据。 - 数据处理:计算与上次采集之间的CPU时间差,并通过
ComputeCpuUsage()
计算CPU使用百分比。 - 数据记录:将计算后的数据写入二进制文件。
- 循环控制:根据
refresh_delay_
设置的时间间隔进行循环,直到接收到退出信号。
5.2.2 CpuUsageMonitor::ComputeCpuUsage()
计算每个线程的CPU使用百分比,并将数据封装为 CpuUsageData
结构体。
void ComputeCpuUsage() {std::lock_guard<std::mutex> lck(data_mutex_);auto now = std::chrono::system_clock::now();auto epoch = now.time_since_epoch();auto seconds_since_epoch =std::chrono::duration_cast<std::chrono::seconds>(epoch).count();uint32_t timestamp = static_cast<uint32_t>(seconds_since_epoch);std::vector<CpuUsageData> batch_data;for (const auto &thread : threads_) {int64_t user_delta = 0;int64_t kernel_delta = 0;if (previous_ticks_.find(thread) != previous_ticks_.end()) {user_delta =current_ticks_.at(thread).first - previous_ticks_.at(thread).first;kernel_delta = current_ticks_.at(thread).second -previous_ticks_.at(thread).second;}uint32_t user_percent = 0;uint32_t kernel_percent = 0;if (delta_total_cpu_time_ > 0) {user_percent = static_cast<uint32_t>(static_cast<double>(user_delta) /delta_total_cpu_time_ * 100.0);kernel_percent = static_cast<uint32_t>(static_cast<double>(kernel_delta) / delta_total_cpu_time_ * 100.0);}uint8_t thread_status =0; // e.g., 0 = Running, 1 = Sleeping, 2 = Waiting, 3 = Stoppeduint8_t extra_flags = 0; // Can store priority or other flagsauto it = thread_names_.find(thread);std::string thread_name =(it != thread_names_.end()) ? it->second : "unknown";if (thread_name.find("worker") != std::string::npos) {thread_status = 1; // Assume threads with 'worker' in name are sleeping}extra_flags = thread_priorities_[thread] &0x7; // Lower 3 bits of priority as extra flagsint real_thread_id = std::stoi(thread);CpuUsageData data;data.user_percent =user_percent > 100 ? 100 : static_cast<uint8_t>(user_percent);data.kernel_percent =kernel_percent > 100 ? 100 : static_cast<uint8_t>(kernel_percent);data.user_ticks = static_cast<uint16_t>(current_ticks_.at(thread).first);data.kernel_ticks =static_cast<uint16_t>(current_ticks_.at(thread).second);data.timestamp = timestamp;data.thread_id = static_cast<uint32_t>(real_thread_id);data.thread_status = thread_status;data.extra_flags = extra_flags;batch_data.push_back(data);DEBUG_PRINT("Thread ID %u: user_percent=%u, kernel_percent=%u, ""status=%u, flags=%u\n",data.thread_id, data.user_percent, data.kernel_percent,data.thread_status, data.extra_flags);}// Write data to binary fileWriteDataToBinaryFile(batch_data);
}
功能描述:
- CPU时间差计算:计算当前与上次采集之间的用户态和内核态CPU时间差。
- CPU使用百分比:基于CPU时间差计算每个线程的用户态和内核态CPU使用百分比。
- 状态与标志:根据线程名称推断线程状态,并提取线程优先级的低3位作为额外标志。
- 数据封装:将计算结果封装为
CpuUsageData
结构体,并批量写入二进制文件。
5.2.3 CpuUsageMonitor::PrintProcessInfo()
打印进程和所有线程的基本信息,包括名称、ID和优先级。
void PrintProcessInfo() {// Lock the mutex to ensure thread-safe access to shared datastd::lock_guard<std::mutex> lck(data_mutex_);// Retrieve and print process priorityint process_priority = getpriority(PRIO_PROCESS, pid_);if (process_priority == -1 && errno != 0) {fprintf(stderr, "Failed to get process priority: %s\n", strerror(errno));} else {fprintf(stdout, "Process Name: %s\n", process_name_.c_str());fprintf(stdout, "Process ID: %d\n", pid_);fprintf(stdout, "Process Priority: %d\n", process_priority);}// Print thread informationfprintf(stdout, "Threads (%zu):\n", thread_names_.size());for (const auto &entry : thread_names_) {// Get thread ID and nameconst std::string &thread_id_str = entry.first;const std::string &thread_name = entry.second;// Retrieve thread priorityauto priority_it = thread_priorities_.find(thread_id_str);if (priority_it != thread_priorities_.end()) {int thread_priority = priority_it->second;fprintf(stdout, " Thread Name: %s, Thread ID: %s, Priority: %d\n",thread_name.c_str(), thread_id_str.c_str(), thread_priority);} else {fprintf(stdout," Thread Name: %s, Thread ID: %s, Priority: Unknown\n",thread_name.c_str(), thread_id_str.c_str());}}
}
功能描述:
- 进程信息打印:输出进程名称、ID和优先级。
- 线程信息打印:遍历所有线程,输出线程名称、ID和优先级。如果无法获取某个线程的优先级,则标记为“Unknown”。
5.3 其他重要功能
5.3.1 CpuUsageMonitor::InitializeThreads()
初始化被监控进程的所有线程信息,包括线程ID、名称和优先级。
void InitializeThreads() {std::lock_guard<std::mutex> lck(data_mutex_);threads_.clear();thread_names_.clear();thread_priorities_.clear();std::string task_path = "/proc/" + std::to_string(pid_) + "/task";DIR *dir = opendir(task_path.c_str());if (!dir) {fprintf(stderr, "Failed to open directory: %s\n", task_path.c_str());return;}DirCloser dir_closer(dir);struct dirent *ent;while ((ent = readdir(dir)) != nullptr) {std::string tid_str = ent->d_name;if (std::isdigit(tid_str[0])) {threads_.push_back(tid_str);std::string comm_filename = task_path + "/" + tid_str + "/comm";std::ifstream comm_file(comm_filename);if (comm_file.is_open()) {std::string thread_name;if (std::getline(comm_file, thread_name)) {thread_names_[tid_str] = thread_name;} else {fprintf(stderr, "Failed to read thread name for TID %s\n",tid_str.c_str());thread_names_[tid_str] = "unknown";}// Get thread priority and nice valuestd::string stat_filename = task_path + "/" + tid_str + "/stat";std::ifstream stat_file(stat_filename);if (!stat_file.is_open()) {fprintf(stderr, "Failed to open file: %s\n", stat_filename.c_str());continue;}std::string line;if (!std::getline(stat_file, line)) {fprintf(stderr, "Failed to read line from file: %s\n",stat_filename.c_str());continue;}std::istringstream iss(line);std::string temp;int priority = 0, nice_value = 0;// Skip to the priority and nice fieldsfor (int i = 0; i < PRIORITY_FIELD_INDEX; ++i) {if (!(iss >> temp)) {fprintf(stderr, "Error parsing stat file: %s\n",stat_filename.c_str());continue;}}// Read priority and nice valueif (!(iss >> priority >> nice_value)) {fprintf(stderr,"Error parsing priority/nice value from stat file: %s\n",stat_filename.c_str());continue;}// Store prioritythread_priorities_[tid_str] = priority;} else {fprintf(stderr, "Failed to open comm file for TID %s\n",tid_str.c_str());thread_names_[tid_str] = "unknown";}}}
}
功能描述:
- 线程遍历:通过访问
/proc/[pid]/task
目录,遍历所有线程ID。 - 线程名称获取:读取每个线程的
comm
文件,获取线程名称。 - 线程优先级获取:读取每个线程的
stat
文件,解析优先级和nice值。 - 数据存储:将线程名称和优先级存储到相应的映射中,供后续使用。
5.3.2 CpuUsageMonitor::ReadAndStoreProcessName()
读取被监控进程的名称并存储。
void ReadAndStoreProcessName() {std::string comm_filename = "/proc/" + std::to_string(pid_) + "/comm";std::ifstream comm_file(comm_filename);if (comm_file.is_open()) {std::getline(comm_file, process_name_);if (!process_name_.empty()) {DEBUG_PRINT("Process name: %s\n", process_name_.c_str());} else {fprintf(stderr, "Process name is empty for PID %d.\n", pid_);}} else {fprintf(stderr, "Failed to open %s\n", comm_filename.c_str());}
}
功能描述:
- 进程名称获取:通过读取
/proc/[pid]/comm
文件,获取进程名称。 - 数据存储:将进程名称存储到
process_name_
变量中,以便后续打印和记录。
6. Python解析代码解析
Python脚本负责读取C++录制的二进制数据文件,解析CPU使用数据,并生成可视化图表。以下将详细解析其主要模块和关键函数。
6.1 主要模块
-
二进制数据解析:
- 读取C++程序生成的二进制文件,按照预定义的结构体格式解析数据。
- 提取进程名称、线程ID与线程名称的映射。
-
数据处理与分析:
- 将解析后的数据转换为Pandas DataFrame,方便后续分析。
- 计算各线程的CPU使用统计指标(最小值、最大值、平均值)。
-
数据可视化:
- 使用Matplotlib生成CPU使用率随时间变化的图表。
- 支持过滤特定线程、CPU使用类型以及时间范围。
6.2 关键函数
6.2.1 parse_cpu_usage_data(record_bytes)
解析单条CPU使用数据。
def parse_cpu_usage_data(record_bytes):"""Parse a single CpuUsageData record from bytes.Parameters:record_bytes (bytes): 16-byte binary data representing CPU usage.Returns:dict: Parsed fields from the record."""if len(record_bytes) != CPU_USAGE_SIZE:raise ValueError("Record size must be 16 bytes")# Define the struct format: little-endian# B: uint8_t user_percent# B: uint8_t kernel_percent# H: uint16_t user_ticks# H: uint16_t kernel_ticks# I: uint32_t timestamp# I: uint32_t thread_id# B: uint8_t thread_status# B: uint8_t extra_flagsstruct_format = '<BBHHIIBB'unpacked_data = struct.unpack(struct_format, record_bytes)record = {"user_percent": unpacked_data[0],"kernel_percent": unpacked_data[1],"user_ticks": unpacked_data[2],"kernel_ticks": unpacked_data[3],"timestamp": unpacked_data[4],"thread_id": unpacked_data[5],"thread_status": unpacked_data[6],"extra_flags": unpacked_data[7],}return record
功能描述:
- 数据长度校验:确保每条记录为16字节。
- 数据解包:使用
struct.unpack
按照C++定义的结构体格式解析数据。 - 数据存储:将解析后的数据存储到字典中,便于后续处理。
6.2.2 read_file_header(f)
读取并解析二进制文件的头部信息。
def read_file_header(f):"""Read and parse the file header from cpu_usage.bin.Parameters:f (file object): Opened binary file object positioned at the beginning.Returns:tuple: (process_name (str), thread_name_map (dict))"""# Read header_size (4 bytes)header_size_data = f.read(4)if len(header_size_data) < 4:raise ValueError("Failed to read header size.")header_size = struct.unpack("<I", header_size_data)[0]# Read the rest of the headerheader_data = f.read(header_size)if len(header_data) < header_size:raise ValueError("Failed to read complete header.")offset = 0# Read process_name_length (4 bytes)process_name_length = struct.unpack_from("<I", header_data, offset)[0]offset += 4# Read process_nameprocess_name = header_data[offset : offset + process_name_length].decode("utf-8")offset += process_name_length# Read thread_map_size (4 bytes)thread_map_size = struct.unpack_from("<I", header_data, offset)[0]offset += 4thread_name_map = {}for _ in range(thread_map_size):# Read thread_id (4 bytes)thread_id = struct.unpack_from("<I", header_data, offset)[0]offset += 4# Read thread_name_length (4 bytes)thread_name_length = struct.unpack_from("<I", header_data, offset)[0]offset += 4# Read thread_namethread_name = header_data[offset : offset + thread_name_length].decode("utf-8")offset += thread_name_lengththread_name_map[thread_id] = thread_name# Debug: Print the header information# Uncomment the following lines for debugging purposes# print(f"Process Name: {process_name}")# print(f"Thread Name Map: {thread_name_map}")return process_name, thread_name_map
功能描述:
- 头部大小读取:读取前4字节,获取头部大小。
- 进程名称读取:根据进程名称长度,读取进程名称。
- 线程映射读取:读取线程数量及每个线程的ID与名称,存储到字典中。
6.2.3 read_cpu_usage_bin(filename)
读取并解析整个二进制数据文件。
def read_cpu_usage_bin(filename):"""Read and parse the cpu_usage.bin file.Parameters:filename (str): Path to the cpu_usage.bin file.Returns:tuple: (records (list of dict), process_name (str), thread_name_map (dict))"""records = []process_name = "Unknown Process"thread_name_map = {}try:with open(filename, "rb") as f:# Read and parse the headerprocess_name, thread_name_map = read_file_header(f)# Read and parse each CpuUsageData recordwhile True:record_bytes = f.read(CPU_USAGE_SIZE)if not record_bytes or len(record_bytes) < CPU_USAGE_SIZE:breakrecord = parse_cpu_usage_data(record_bytes)records.append(record)except FileNotFoundError:print(f"File {filename} not found.")except Exception as e:print(f"Error reading binary file: {e}")return records, process_name, thread_name_map
功能描述:
- 文件读取:打开二进制文件并读取头部信息。
- 数据采集:循环读取每条CPU使用数据,并调用
parse_cpu_usage_data
解析。 - 数据存储:将所有记录存储到列表中,便于后续转换为DataFrame。
6.2.4 parse_records_to_dataframe(records, thread_name_map)
将解析后的记录转换为Pandas DataFrame。
def parse_records_to_dataframe(records, thread_name_map):"""Convert parsed records to a pandas DataFrame.Parameters:records (list of dict): Parsed CPU usage records.thread_name_map (dict): Mapping from thread ID to thread name.Returns:pandas.DataFrame: DataFrame containing the CPU usage data."""data = pd.DataFrame(records)# Ensure thread_id is integerdata["thread_id"] = data["thread_id"].astype(int)# Map thread_id to thread_namedata["thread_name"] = data["thread_id"].map(thread_name_map).fillna("unknown")# Convert timestamp to datetime (assuming timestamp is seconds since epoch)data["timestamp"] = pd.to_datetime(data["timestamp"], unit="s")return data
功能描述:
- 数据转换:将记录列表转换为Pandas DataFrame。
- 线程名称映射:根据线程ID映射线程名称,填充“unknown”以处理未知线程。
- 时间戳转换:将时间戳转换为可读的日期时间格式。
6.2.5 plot_cpu_usage(...)
生成CPU使用率随时间变化的图表。
def plot_cpu_usage(data,process_name="Unknown Process",filter_thread=None,filter_cpu_type=None,time_range=None,show_summary_info=True,
):"""Plot CPU usage over time for the process and its threads.Parameters:data (pandas.DataFrame): DataFrame containing CPU usage data.process_name (str): Name of the process.filter_thread (str, optional): Filter to include only specific thread names.filter_cpu_type (str, optional): Filter to include only 'user' or 'kernel' CPU usage.time_range (tuple, optional): Tuple of (start_time, end_time) to filter the data.show_summary_info (bool): Whether to display summary information on the plot."""plt.figure(figsize=(14, 10))# Calculate total CPU usage of the processprocess_cpu = calculate_process_cpu(data)# Sort by timestampprocess_cpu = process_cpu.sort_values("timestamp")# Plot total CPU usageplt.plot(process_cpu["timestamp"],process_cpu["total_usage"],label="Process Total CPU Usage",color="black",linewidth=2,)# Apply filters if anyif filter_thread:data = data[data["thread_name"].str.contains(filter_thread, case=False)]if filter_cpu_type:if filter_cpu_type.lower() == "user":data = data[["timestamp", "thread_name", "user_percent"]]elif filter_cpu_type.lower() == "kernel":data = data[["timestamp", "thread_name", "kernel_percent"]]if time_range:start_time, end_time = time_rangedata = data[(data["timestamp"] >= start_time) & (data["timestamp"] <= end_time)]# Sort data by timestampdata = data.sort_values("timestamp")# Set timestamp as index for resamplingdata.set_index("timestamp", inplace=True)# Determine resampling frequency based on dataresample_freq = 'S' # 1 second# Plot CPU usage for each threadfor thread_name in data["thread_name"].unique():subset = data[data["thread_name"] == thread_name]# Resample to ensure continuityuser_usage = subset["user_percent"].resample(resample_freq).mean().interpolate()kernel_usage = subset["kernel_percent"].resample(resample_freq).mean().interpolate()# Plot user_percent as solid lineplt.plot(user_usage.index,user_usage.values,label=f"{thread_name} (User)",linestyle="-", # Solid line for user)# Plot kernel_percent as dashed lineplt.plot(kernel_usage.index,kernel_usage.values,label=f"{thread_name} (Kernel)",linestyle="--", # Dashed line for kernel)plt.xlabel("Time")plt.ylabel("CPU Usage (%)")plt.title(f"CPU Usage Over Time by Thread for Process: {process_name}")plt.gcf().autofmt_xdate()plt.legend(loc="upper left", bbox_to_anchor=(1, 1))plt.grid(True)plt.tight_layout(rect=[0, 0.1, 1, 0.95])if show_summary_info:summary_info = get_summary_table(data.reset_index(), process_name)plt.figtext(0.02,0.01,summary_info,fontsize=9,verticalalignment="bottom",horizontalalignment="left",bbox=dict(facecolor="white", alpha=0.5),)try:plt.savefig("cpu_usage_over_time.png")plt.show()except KeyboardInterrupt:print("\nPlotting interrupted by user. Exiting gracefully.")plt.close()sys.exit(0)
功能描述:
- 总CPU使用率绘制:绘制整个进程的CPU总使用率曲线。
- 线程CPU使用率绘制:为每个线程绘制用户态和内核态的CPU使用率曲线,其中用户态使用实线,内核态使用虚线。
- 过滤与时间范围:支持根据线程名称、CPU使用类型和时间范围进行数据过滤。
- 摘要信息:在图表底部显示每个线程的CPU使用统计信息。
相关文章:
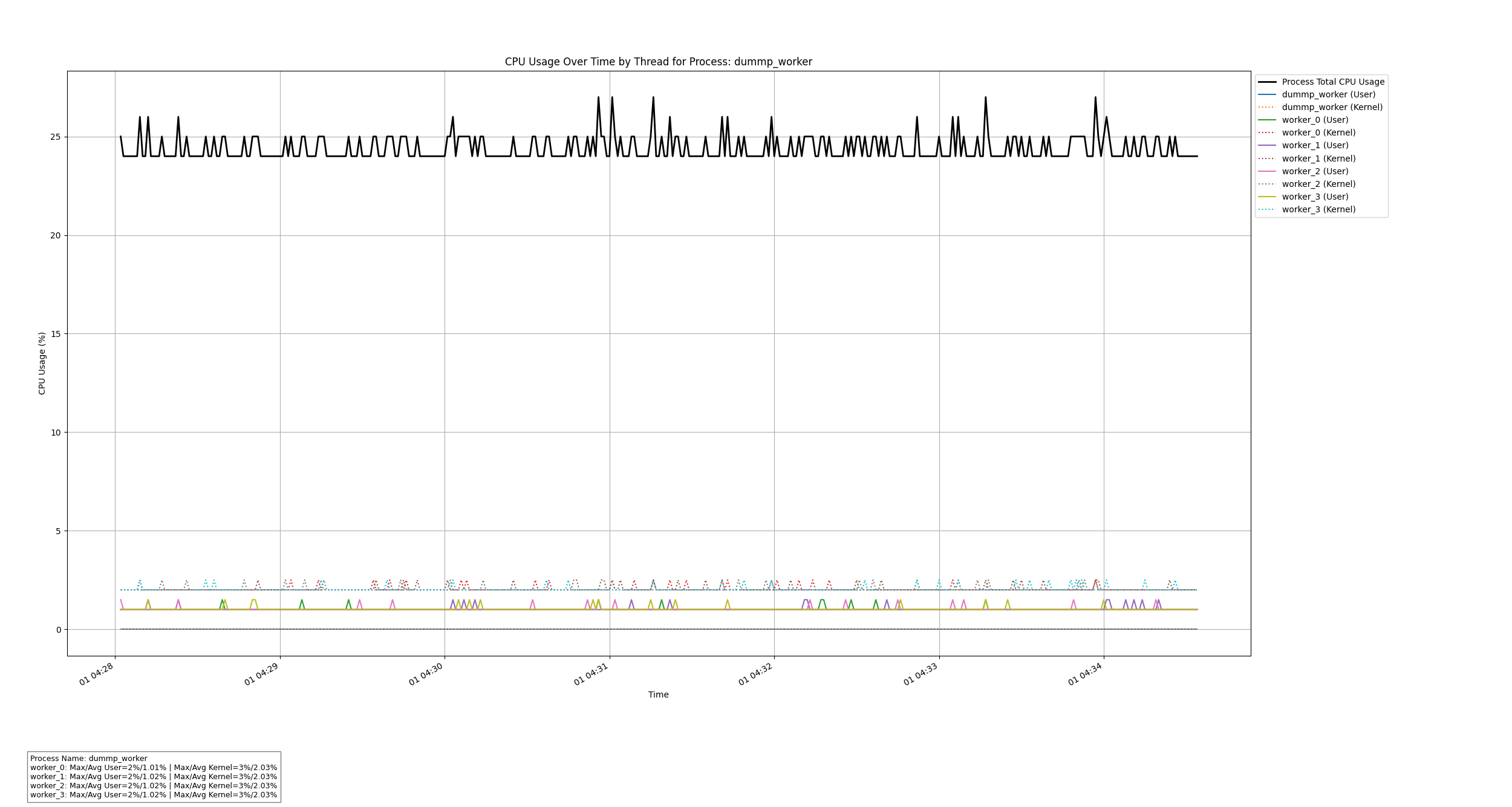
基于C++和Python的进程线程CPU使用率监控工具
文章目录 0. 概述1. 数据可视化示例2. 设计思路2.1 系统架构2.2 设计优势 3. 流程图3.1 C录制程序3.2 Python解析脚本 4. 数据结构说明4.1 CpuUsageData 结构体 5. C录制代码解析5.1 主要模块5.2 关键函数5.2.1 CpuUsageMonitor::Run()5.2.2 CpuUsageMonitor::ComputeCpuUsage(…...
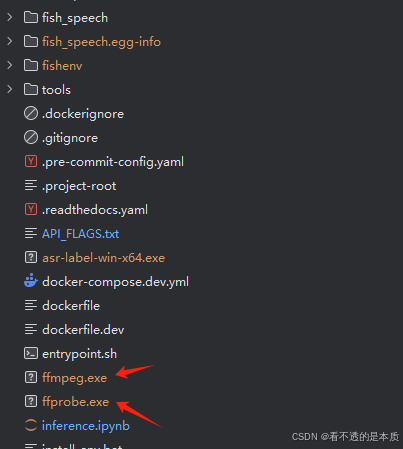
fish-speech语音大模型本地部署
文章目录 fish-speech模型下载编译部署 小结 fish-speech模型 先说下fish-speech模型吧,可以先看下官网。如下: 这就是一个模型,可以根据一个样例声音,构建出自己需要的声音。其实,这个还是有很多用途的;…...
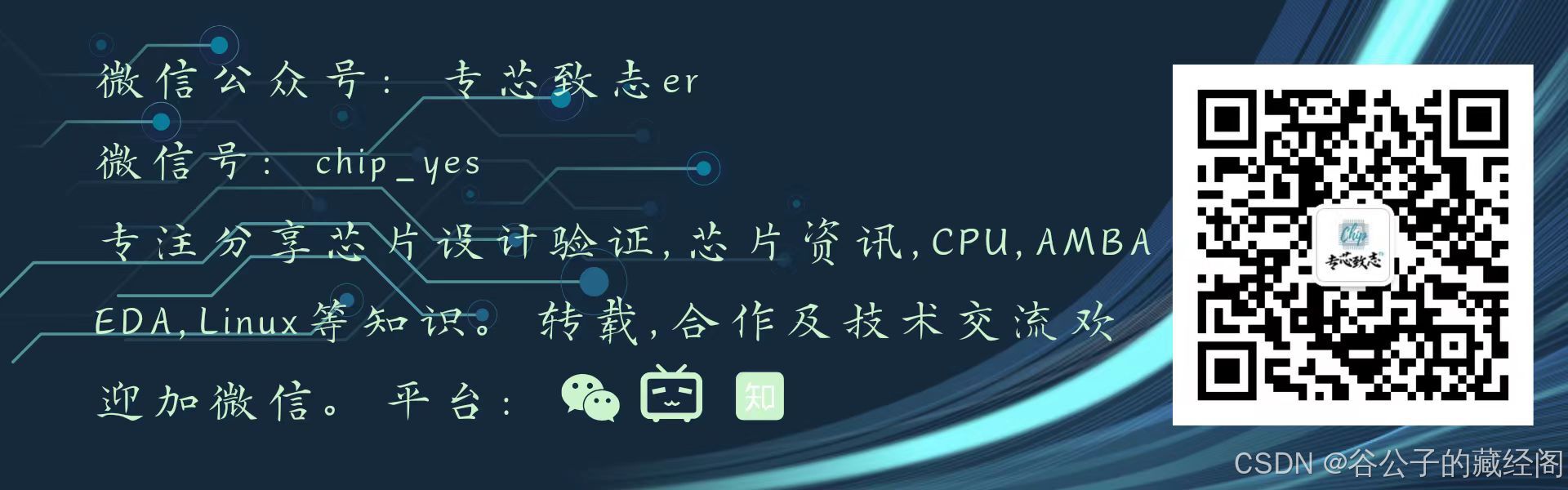
如何写出更牛的验证激励
前言 芯片验证是为了发现芯片中的错误而执行的过程,它是一个破坏性的过程。完备的验证激励可以更有效地发现芯片错误,进而缩短验证周期。合格的验证激励必须能产生所有可能的验证场景(完备性),包括合法和非法的场景,并保持最大的…...
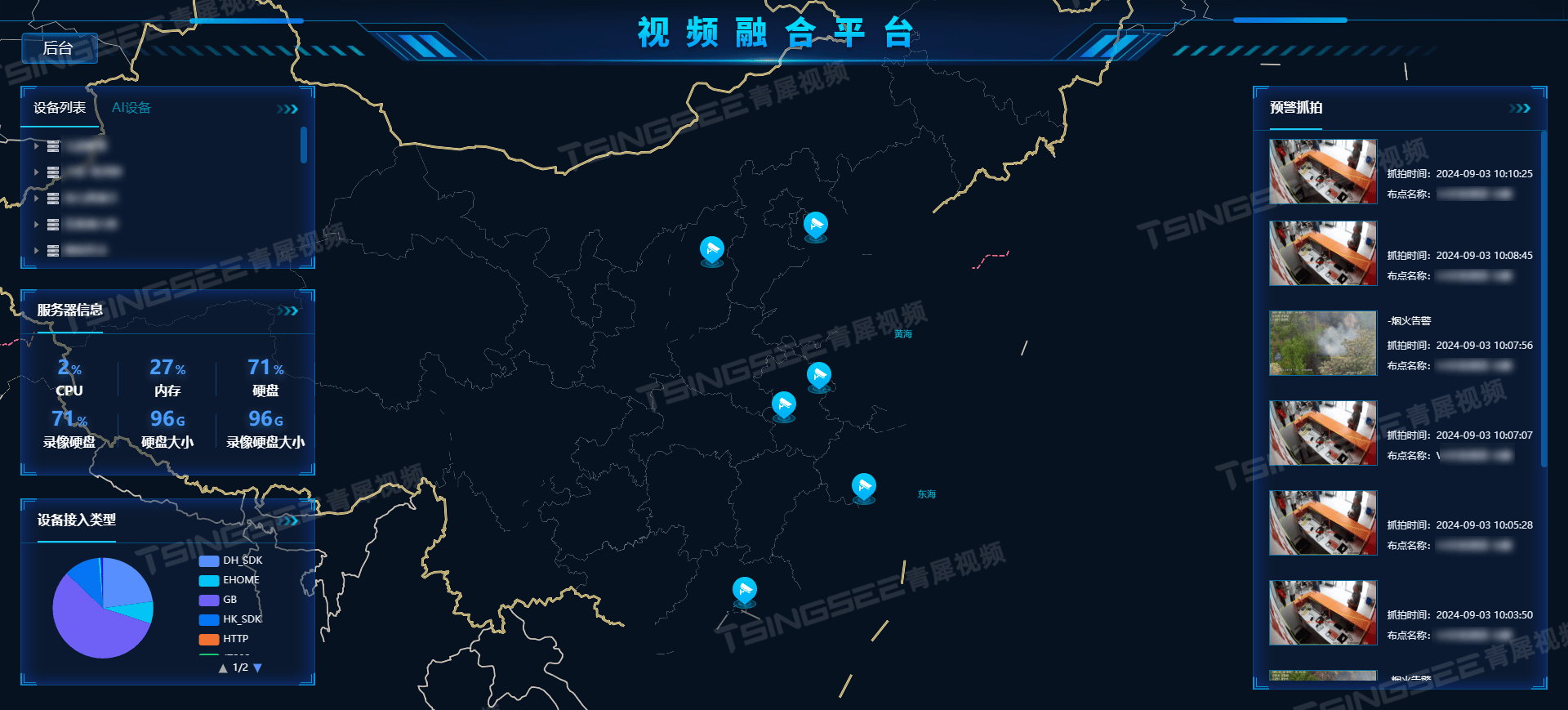
EasyCVR视频汇聚平台:解锁视频监控核心功能,打造高效安全监管体系
随着科技的飞速发展,视频监控技术已成为现代社会安全、企业管理、智慧城市构建等领域不可或缺的一部分。EasyCVR视频汇聚平台作为一款高性能的视频综合管理平台,凭借其强大的视频处理、汇聚与融合能力,在构建智慧安防/视频监控系统中展现出了…...

面对大文件(300G以上)如何加速上传速度
解题思路 采用分片上传,同时每个分片多线程上传可以加速上传速度,上传速度提升10倍左右 在阿里云OSS Go SDK中,bucket.UploadStream 函数并没有直接提供,而是通过 bucket.UploadFile 或者 bucket.PutObject 等函数来实现文件上传…...

基于 Redis 实现消息队列的深入解析
目录 Redis 消息队列简介Redis 消息队列的实现方式 2.1 使用 List 实现简单队列2.2 使用 Pub/Sub 模式实现消息发布与订阅2.3 使用 Stream 实现高级队列 Redis 消息队列的特点与优势Redis 消息队列的应用场景Redis 消息队列的局限性及应对方案总结 Redis 消息队列简介 Redis…...
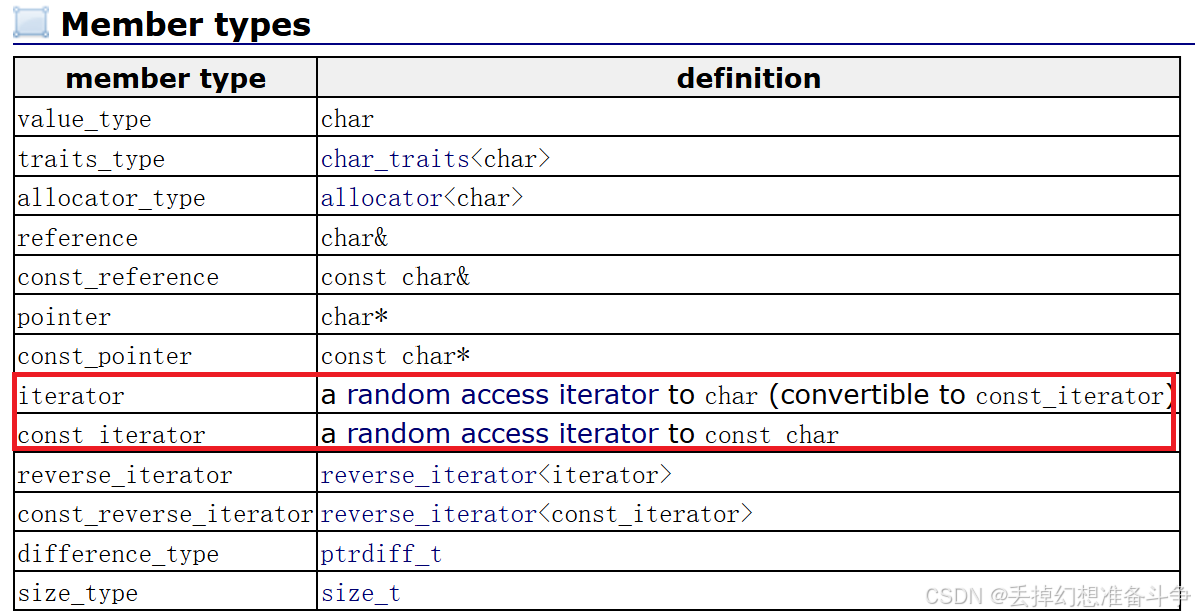
C++(string类的实现)
1. 迭代器、返回capacity、返回size、判空、c_str、重载[]和clear的实现 string类的迭代器的功能就类似于一个指针,所以我们可以直接使用一个指针来实现迭代器,但如下图可见迭代器有两个,一个是指向的内容可以被修改,另一个则是指…...

nrf 24l01使用方法
1、frequency 频率基础频率2.400G HZ RF_CH RF_CH10 CH2.4G0.01G2.41G 2、逻辑通道6个 pipe 时间片不同,占用同一个频率 发送时,只有一个pipe 接受时可以有6个pipe 3、通讯速率 air data rate rf_dr 寄存器设置 有两种速率 2M 1M RF_DR0 1M ,…...

C语言普及难度三题
先热个身,一个长度为10的整型数组,输出元素的差的max和min。 #include<stdio.h> int main() {int m[10],i0,max,min;for(i0;i<10;i){scanf("%d",&m[i]);}minm[0];maxm[0];for (i 0; i <10; i){if(min>m[i]) min m[i];i…...
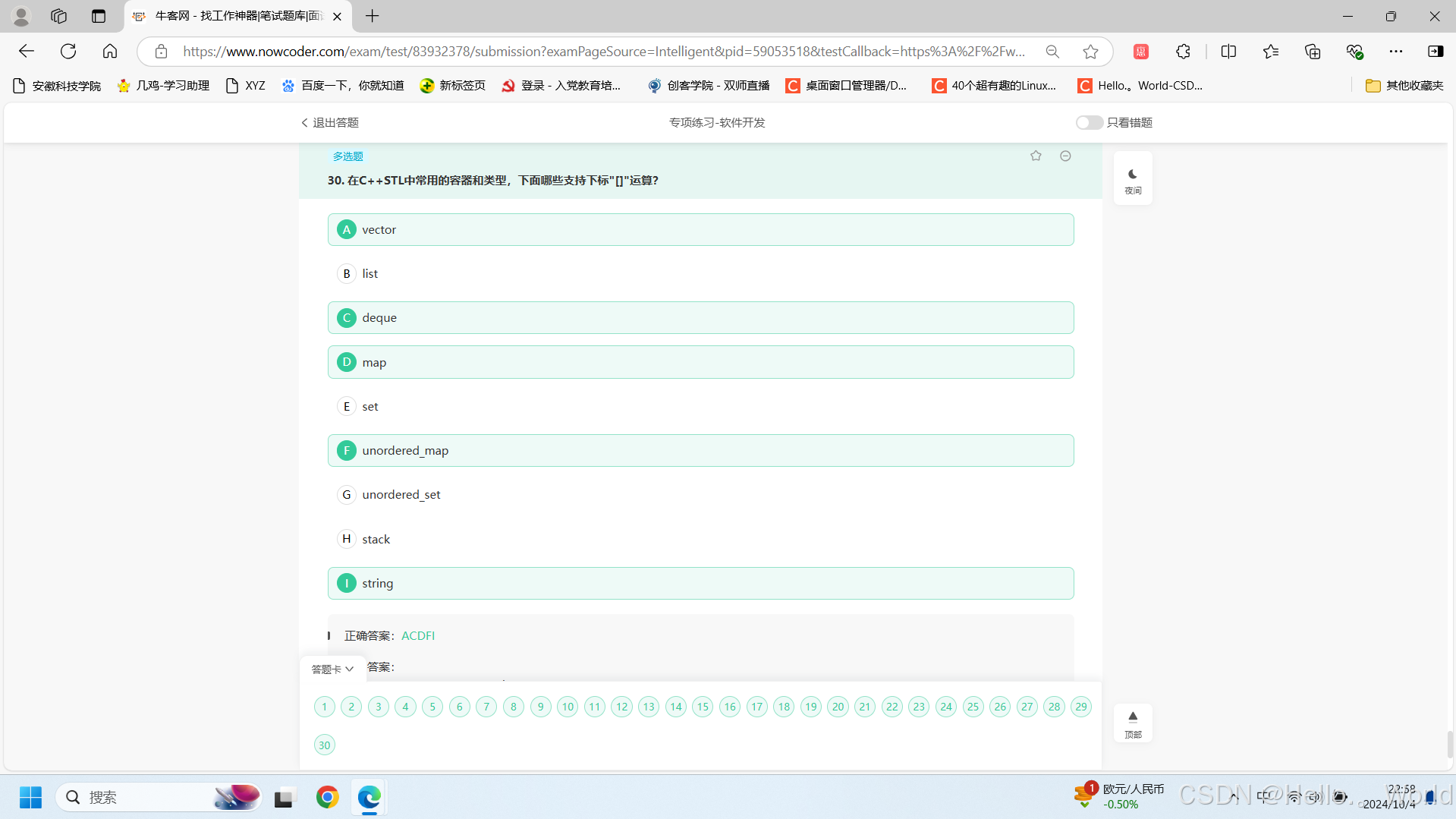
10.4每日作业
C1 C2 C1 C2...
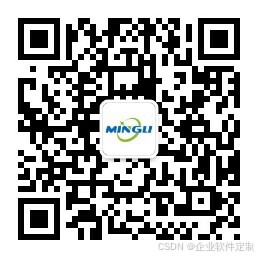
日常工作记录:服务器被攻击导致chattr: command not found
在深夜的寂静中,公司的服务器突然遭遇了一场突如其来的攻击。特别是nginx配置文件无法修改,仿佛预示着不祥的预兆,面对这突如其来的灾难,技术人员迅速响应。 这时候需要chattr,但是执行的chattr -i xxx的时候…...
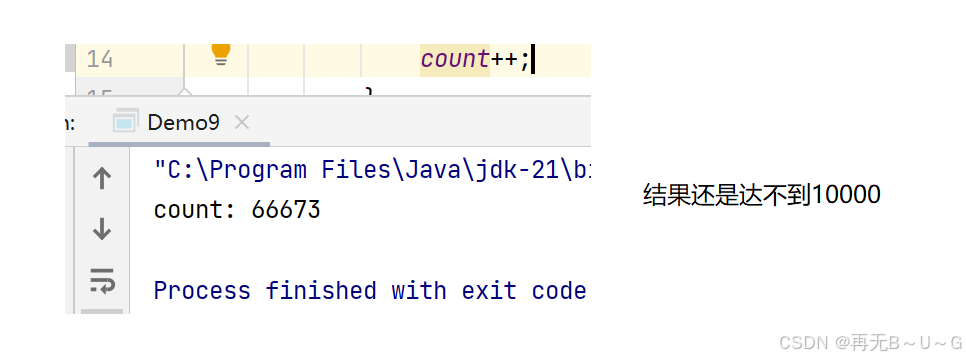
多线程-初阶(1)
本节⽬标 • 认识多线程 • 掌握多线程程序的编写 • 掌握多线程的状态 • 掌握什么是线程不安全及解决思路 • 掌握 synchronized、volatile 关键字 1. 认识线程(Thread) 1.1 概念 1) 线程是什么 ⼀个线程就是⼀个 "执⾏流". 每个线…...
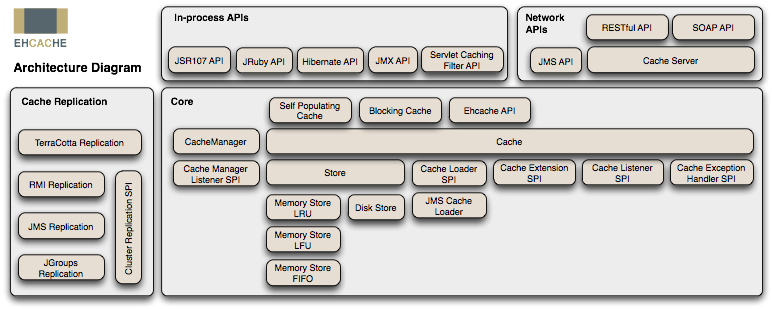
Spring Boot集成encache快速入门Demo
1.什么是encache EhCache 是一个纯 Java 的进程内缓存框架,具有快速、精干等特点,是 Hibernate 中默认的 CacheProvider。 Ehcache 特性 优点 快速、简单支持多种缓存策略:LRU、LFU、FIFO 淘汰算法缓存数据有两级:内存和磁盘&a…...
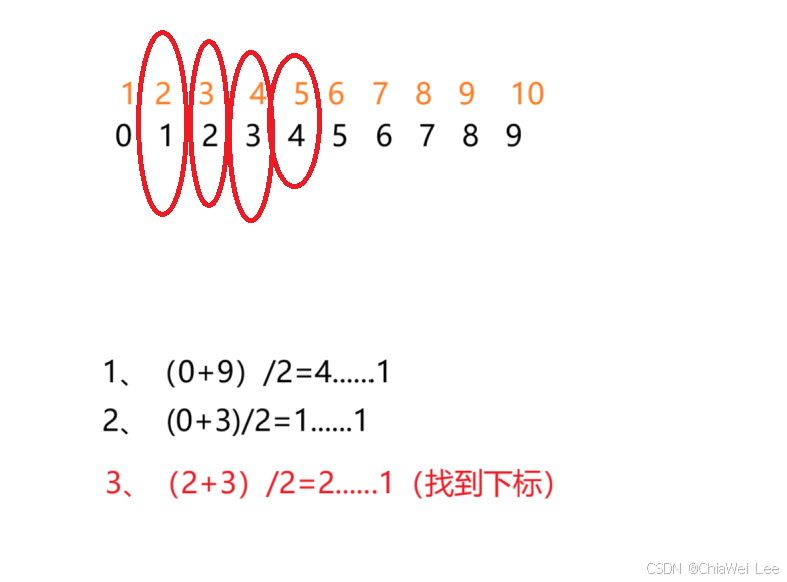
【C语言】数组练习
【C语言】数组练习 练习1:多个字符从两端移动,向中间汇聚练习2、二分查找 练习1:多个字符从两端移动,向中间汇聚 编写代码,演示多个字符从两端移动,向中间汇聚 练习2、二分查找 在⼀个升序的数组中查找指…...
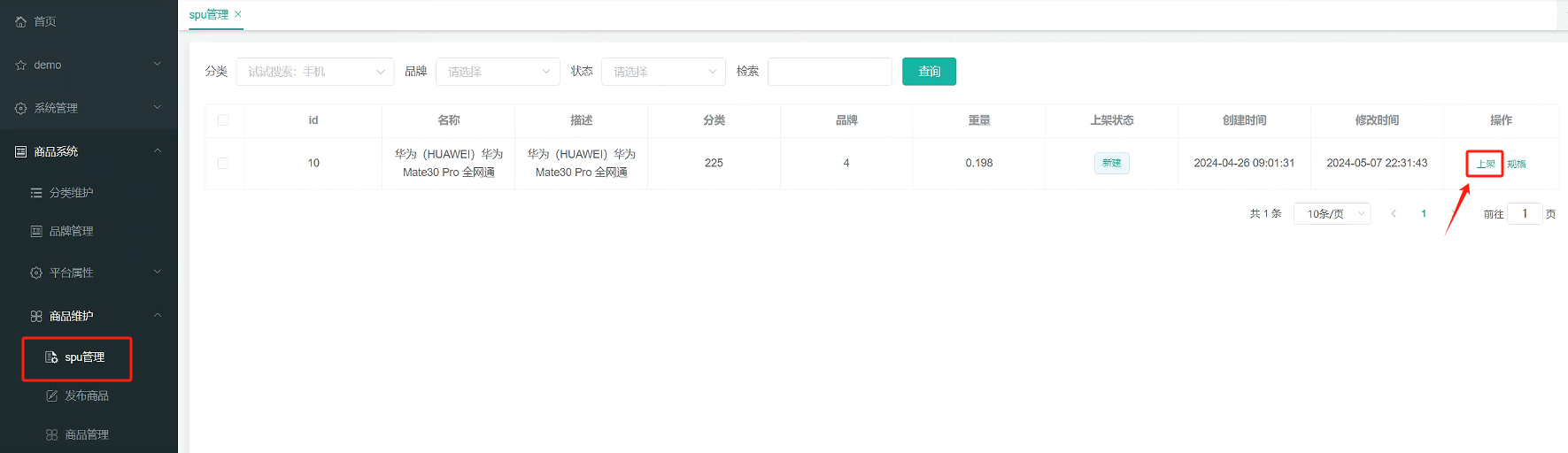
微服务实战——ElasticSearch(保存)
商品上架——ElasticSearch(保存) 0.商城架构图 1.商品Mapping 分析:商品上架在 es 中是存 sku 还是 spu ? 检索的时候输入名字,是需要按照 sku 的 title 进行全文检索的检索使用商品规格,规格是 spu 的…...
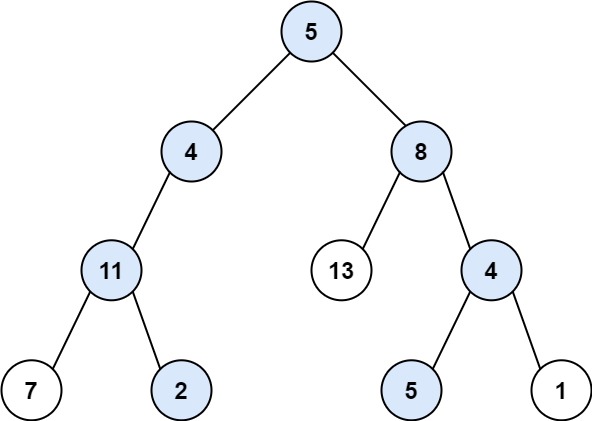
leetcode练习 路径总和II
给你二叉树的根节点 root 和一个整数目标和 targetSum ,找出所有 从根节点到叶子节点 路径总和等于给定目标和的路径。 叶子节点 是指没有子节点的节点。 示例 1: 输入:root [5,4,8,11,null,13,4,7,2,null,null,5,1], targetSum 22 输出&a…...

使用Three.js库创建的简单WebGL应用程序,主要用于展示具有不同透明度和缩放比例的圆环列
上述HTML文档是一个使用Three.js库创建的简单WebGL应用程序,主要用于展示具有不同透明度和缩放比例的圆环列。以下是代码的详细解释: HTML结构: 文档类型声明为HTML5。<html>标签设置了语言属性为英语(lang"en")…...
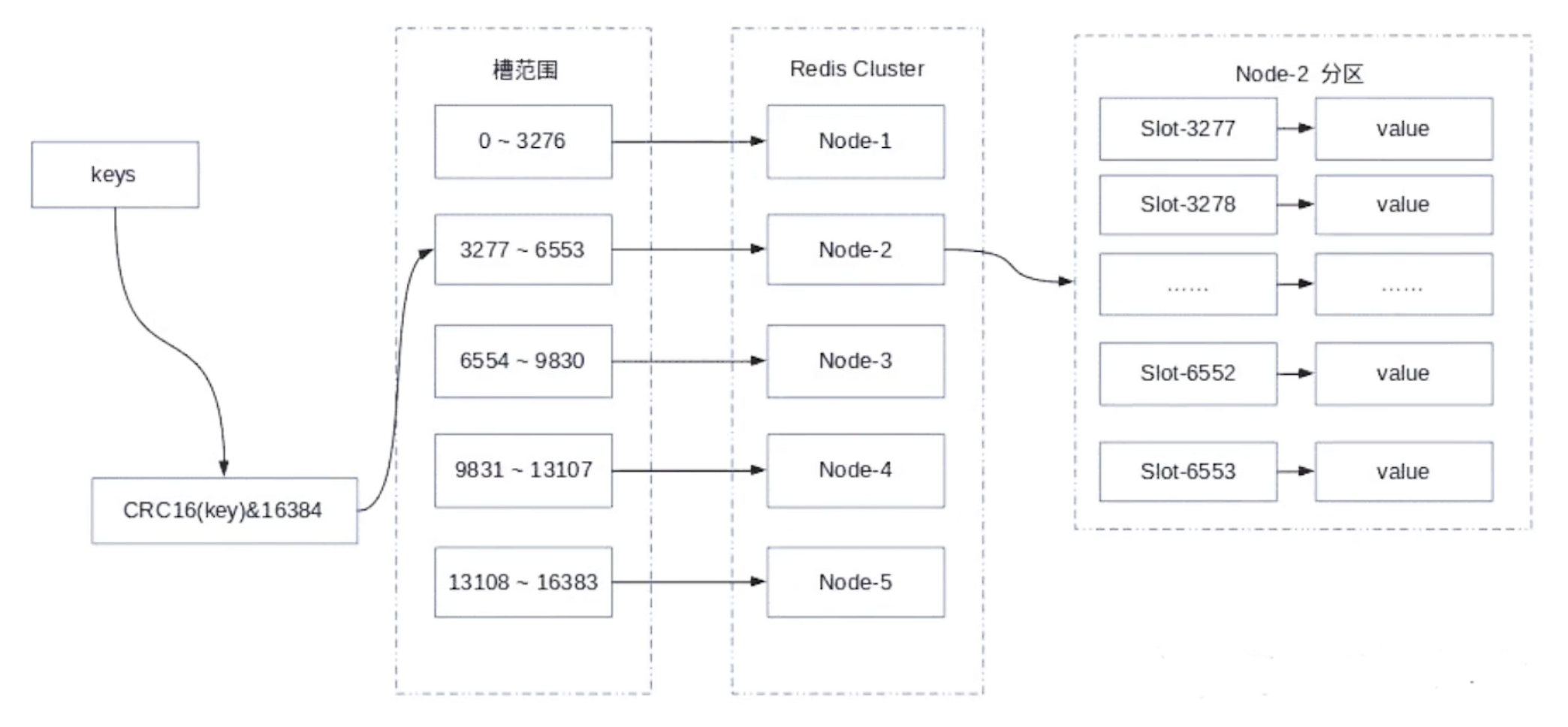
Redis: 集群架构,优缺点和数据分区方式和算法
集群 集群指的就是一组计算机作为一个整体向用户提供一组网络资源 我就举一个简单的例子,比如百度,在北京和你在上海访问的百度是同一个服务器吗?答案肯定是不是的,每一个应用可以部署在不同的地方,但是我们提供的服务…...

负载均衡可以在网络模型的哪一层?
一、网络模型概述 网络模型是用于描述网络通信过程和网络服务的抽象框架。最常见的网络模型有两种:OSI(开放式系统互联)模型和TCP/IP模型。 OSI模型 OSI(Open Systems Interconnection)模型是由国际标准化组织&…...
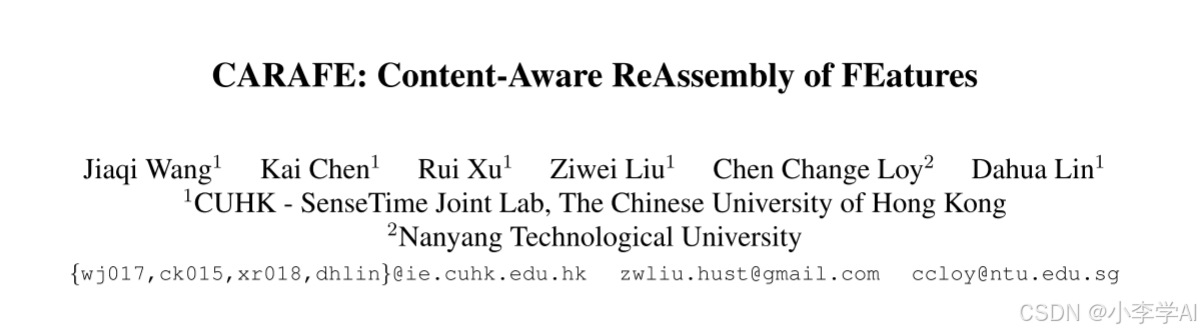
YOLOv11改进 | 上采样篇 | YOLOv11引入CARAFE上采样
1. DySample介绍 1.1 摘要:特征上采样是许多现代卷积网络体系结构(如特征金字塔)中的关键操作。它的设计对于密集预测任务(如对象检测和语义/实例分割)至关重要。在本文中,我们提出了一个通用、轻量级、高效的特征重组算子CARAFE来实现这一目标.CARAFE有几个吸引人的特性…...

【Linux运维】grep命令粗浅学习
文章目录 1 背景介绍1.1 为什么要学习grep?1.2 grep是什么?1.3 grep可以做什么? 2 grep基本语法2.1 命令格式2.2 “PATTERN”部分中的正则表达式语法学习2.3 grep命令参数学习 3 典型案例3.1 匹配非空行,过滤纯空行3.2 匹配IPv4地…...
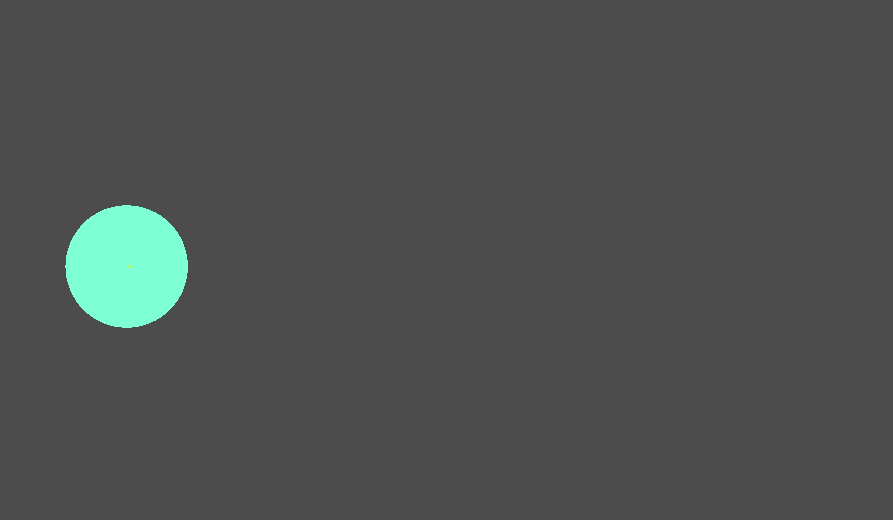
【Godot4.3】匀速和匀变速直线运动粒子
概述 本篇论述,如何用加速度在Godot中控制粒子运动。 匀速和匀变速直线运动的统一 以下是匀变速运动的速度和位移公式: v t v 0 a t x t v 0 t 1 2 a t 2 v_tv_0 at \\ x_tv_0t \frac{1}{2}at^2 vtv0atxtv0t21at2 当a 0 时…...
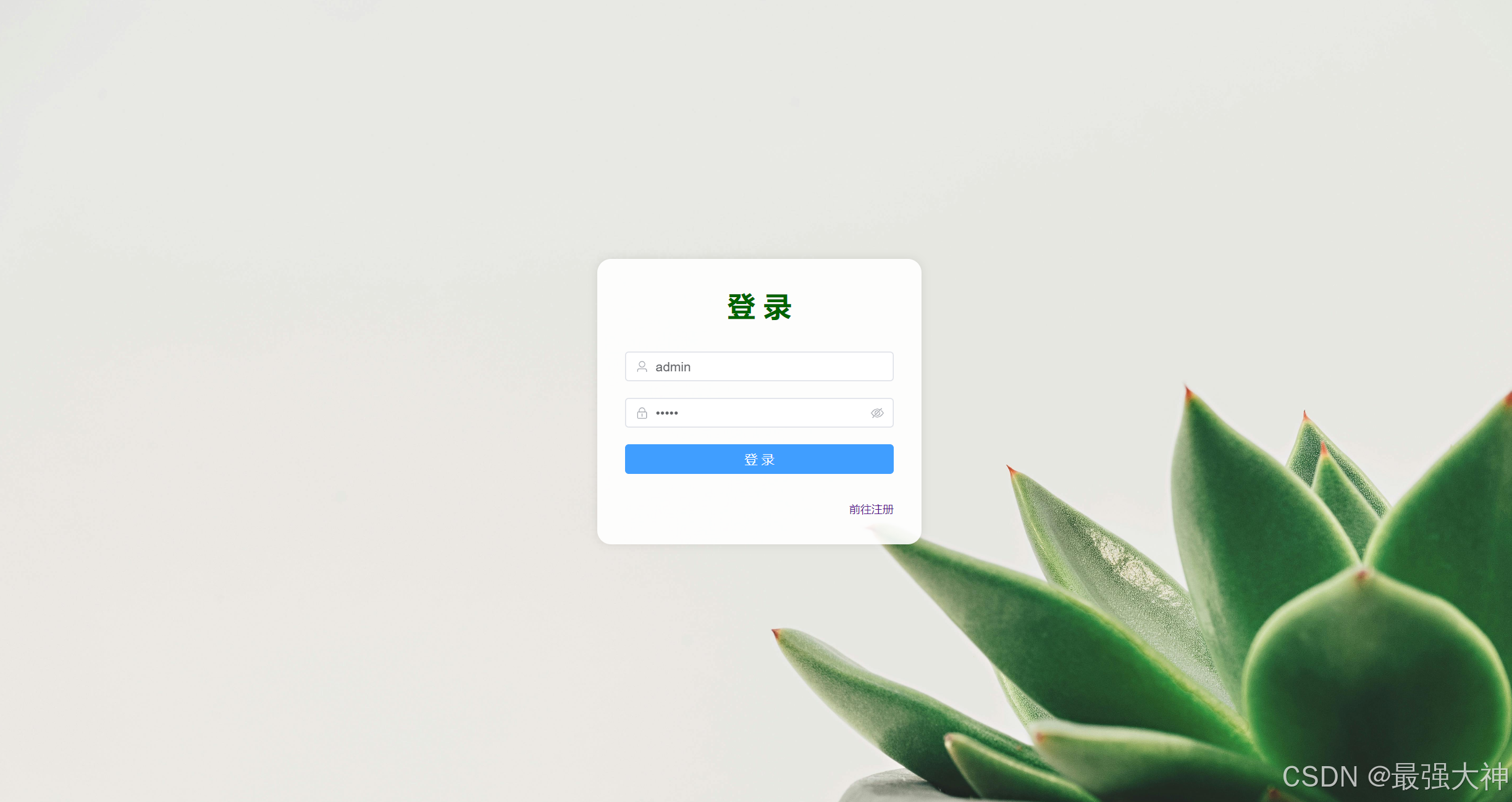
基于Hive和Hadoop的用电量分析系统
本项目是一个基于大数据技术的用电量分析系统,旨在为用户提供全面的电力消耗信息和深入的用电量分析。系统采用 Hadoop 平台进行大规模数据存储和处理,利用 MapReduce 进行数据分析和处理,通过 Sqoop 实现数据的导入导出,以 Spark…...

一个简单的摄像头应用程序4
我们进一步完善了这个app01.py,我们优化了界面使其更人性化,下面介绍中包含了原有的功能及新增的功能: 创建和管理文件夹: create_folder 函数用于创建保存照片和视频的文件夹。 get_next_file_number 函数用于获取文件夹中下一个可用的文件编号。 图像处理: pil_to_cv 函…...
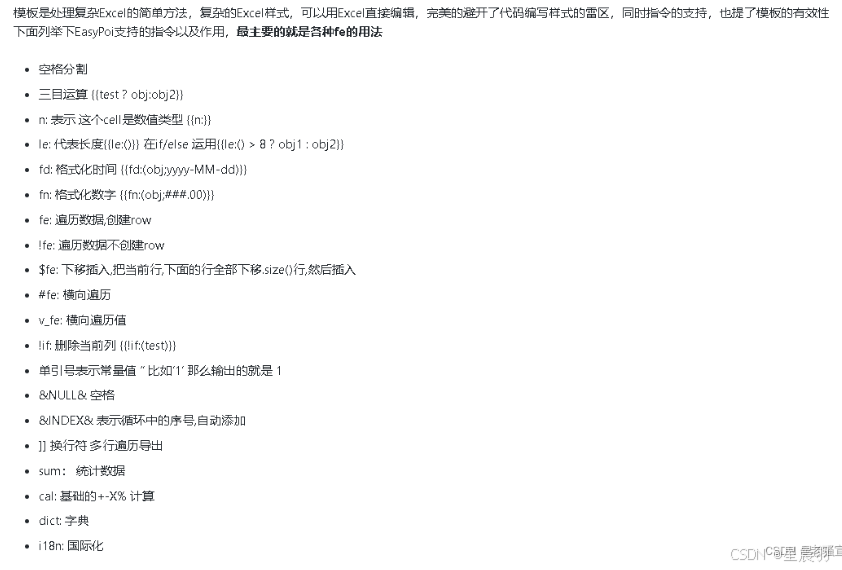
SpringBoot使用EasyPoi根据模板导出word or pdf
1、导出效果 1.1 wrod 1.2 pdf 2、依赖 <!--word--><dependency><groupId>cn.afterturn</groupId><artifactId>easypoi-base</artifactId><version>4.3.0</version></dependency><dependency><groupId>cn.…...
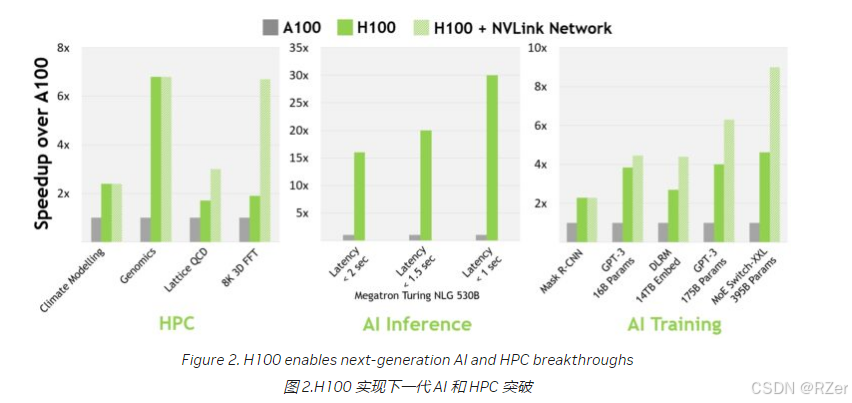
NVIDIA Hopper 架构深入
在 2022 年 NVIDIA GTC 主题演讲中,NVIDIA 首席执行官黄仁勋介绍了基于全新 NVIDIA Hopper GPU 架构的全新 NVIDIA H100 Tensor Core GPU。 文章目录 前言一、NVIDIA H100 Tensor Core GPU 简介二、NVIDIA H100 GPU 主要功能概述1. 新的流式多处理器 (SM) 具有许多性能和效率…...
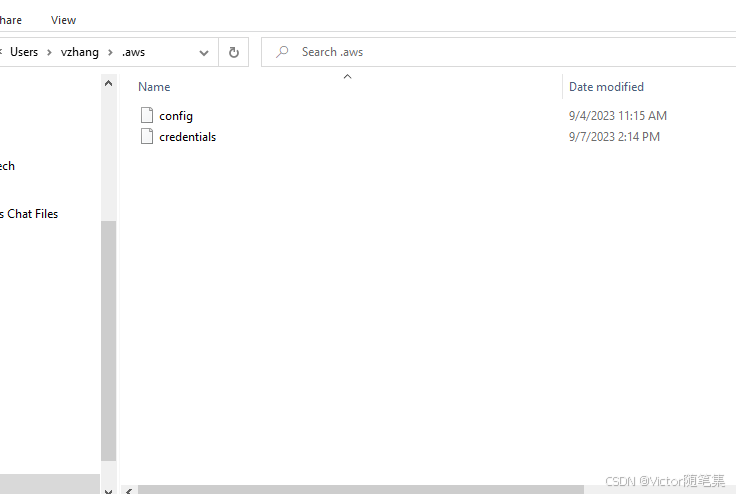
AWS IoT Core for Amazon Sidewalk
目录 1 前言2 AWS IoT2.1 准备条件2.2 创建Credentials2.2.1 创建user2.2.2 配置User 2.3 本地CLI配置Credentials 3 小结 1 前言 在测试Sidewalk时,device发送数据,网关接收到,网关通过网络发送给NS,而此处用到的NS是AWS IoT&am…...
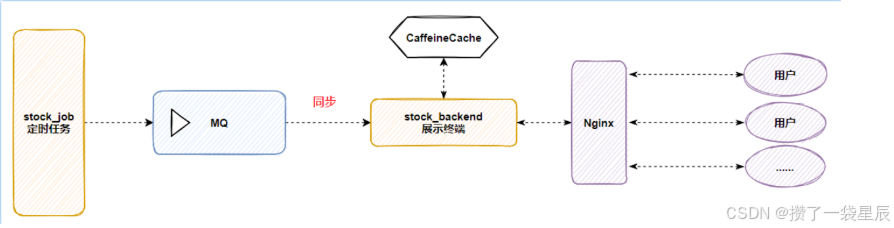
今日指数项目项目集成RabbitMQ与CaffienCatch
今日指数项目项目集成RabbitMQ与CaffienCatch 一. 为什么要集成RabbitMQ 首先CaffeineCatch 是作为一个本地缓存工具 使用CaffeineCatch 能够大大较少I/O开销 股票项目 主要分为两大工程 --> job工程(负责数据采集) , backend(负责业务处理) 由于股票的实时性也就是说 ,…...

C0005.Clion中移动ui文件到新目录后,报错问题的解决
报错问题如下 AutoUic error ------------- "SRC:/confirmwizardpage.cpp" includes the uic file "ui_confirmwizardpage.h", but the user interface file "confirmwizardpage.ui" could not be found in the following directories"SRC…...
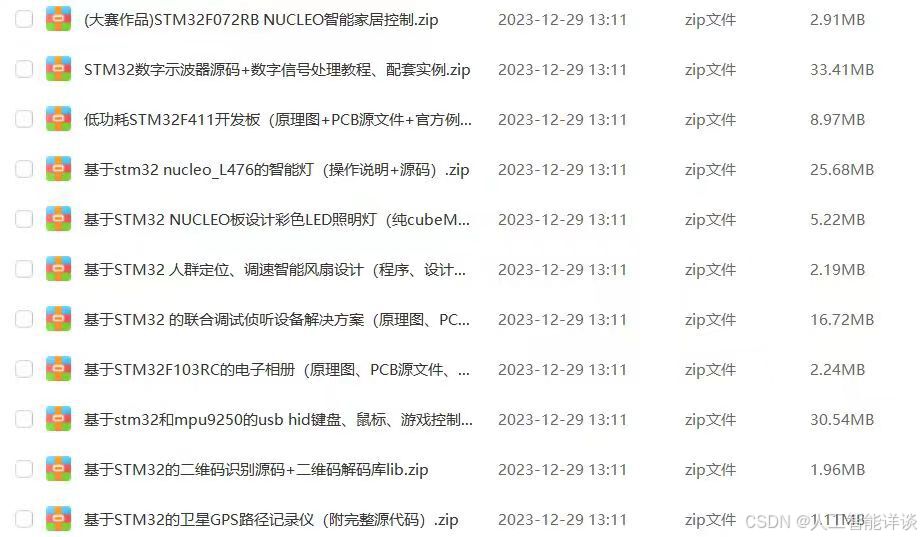
基于STM32的智能家居灯光控制系统设计
引言 本项目将使用STM32微控制器实现一个智能家居灯光控制系统,能够通过按键、遥控器或无线模块远程控制家庭照明。该项目展示了如何结合STM32的外设功能,实现对灯光的智能化控制,提升家居生活的便利性和节能效果。 环境准备 1. 硬件设备 …...